Abstract
Food production data — such as crop, livestock, aquaculture and fisheries statistics — are critical to achieving multiple sustainable development goals. However, the lack of reliable, regularly collected, accessible, usable and spatially disaggregated statistics limits an accurate picture of the state of food production in many countries and prevents the implementation of effective food system interventions. In this Review, we take stock of national and international food production data to understand its availability and limitations. Across databases, there is substantial global variation in data timeliness, granularity (both spatially and by food category) and transparency. Data scarcity challenges are most pronounced for livestock and aquatic food production. These challenges are largely concentrated in Central America, the Middle East and Africa owing to a combination of inconsistent census implementation and a global reliance on self-reporting. Because data scarcity is the result of technical, institutional and political obstacles, solutions must include technological and policy innovations. Fusing traditional and emerging data-gathering techniques with coordinated governance and dedicated long-term financing will be key to overcoming current obstacles to sustained, up-to-date and accurate food production data collection, foundational in promoting and monitoring progress towards healthier and more sustainable food systems worldwide.
Introduction
The production of crops, livestock and aquatic organisms covers more than a third of land1,2 and oceans3, altering Earth systems4 and influencing human health and well-being5. Food production has a central role in determining the extent to which nations can achieve UN Sustainable Development Goal (SDG) targets6, including SDG2 — Zero Hunger. In addition, food production exerts an important influence over numerous other SDGs through its employment of over a billion people7, its large diet-related global burden of disease5, its dominant water footprint8, its contribution to bioenergy9, its substantial greenhouse gas emissions (GHGs)10 and its extensive modifications of natural systems11. A detailed, accurate and up-to-date understanding of the state of food production is foundational to identifying where SDGs are (or are not) being met and serves as a baseline upon which solutions can be built, tested and implemented.
The primary sources of food production data are large-scale censuses (comprehensive data-gathering efforts meant to occur every 5 or 10 years) or surveys (more frequent and less intensive sampling), with complementary remote sensing efforts being used in certain countries. From a perspective of equitable global development, each nation would ideally have the resources to fund, implement and execute these comprehensive data collection efforts; develop robust sampling strategies; collate, standardize and store collected data; and make the final data available to support development, investment and research efforts. Shortcomings in any of these steps can impede the provision, reporting and publication of official food production statistics. In turn, the inaccurate, incomplete or delayed reporting of these data can lead to a distorted understanding of food production patterns and productivity, and could contribute to misinformed and poorly targeted interventions (Fig. 1).
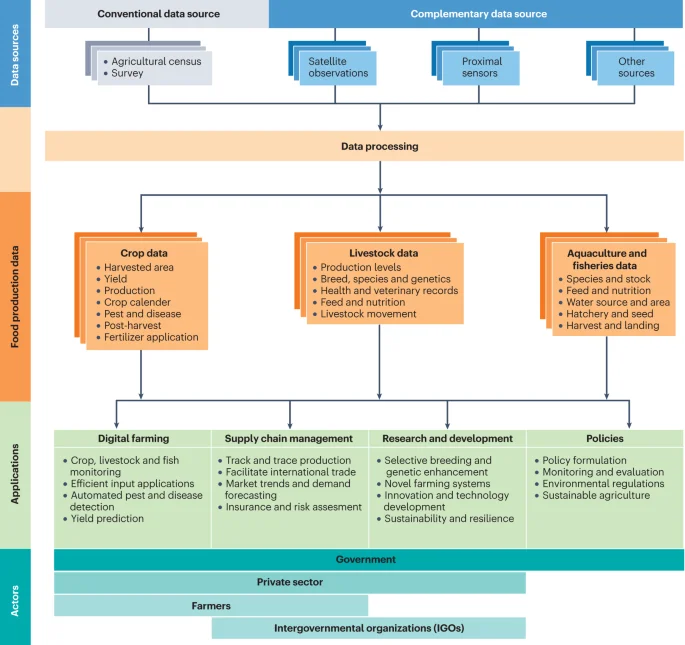
The sources (blue and grey), types (orange), applications (green) and actors (turquoise) of food production data. Food production statistics are diversely sourced and underpin the reliability and accuracy of a suite of decisions and actions related to food security and sustainable development.
Unfortunately, there are substantial gaps in the availability and accessibility of reliable, granular and current data in many regions, with the reliability of food production data also varying widely across products, countries and years. For example, global gridded agricultural products — which inform the efforts of a suite of global assessments and consortia (such as AgMIP12, ISIMIP13, GEOGLAM14 and CGIAR15) — are highly sensitive to the level of disaggregation of underlying food production statistics16,17,18. This scarcity and unequal distribution of quality food production data underscores the critical importance of identifying where and why such deficiencies exist. Quantifying and examining the root causes of insufficient food production data is key to promoting evidence-based understanding and decision-making for sustainable food systems worldwide.
In this Review, we take stock of the current state of global food production data scarcity, defined here as being insufficient in terms of spatial disaggregation (detail), timeliness (recency, temporal coverage and resolution), food item specificity and accessibility. We first quantify the current state of and trends in food production data availability country-by-country for crops, livestock and aquatic organisms. We then examine key technical, institutional and policy obstacles hindering the collection and dissemination of food production data globally. We end by highlighting promising pathways forward for improving global food production data availability and quality. Supporting concerted and creative efforts to address these hotspots of food production data scarcity is critical for enabling holistic progress towards achieving multiple dimensions of sustainable development.
Current state of food production data scarcity
Food production data accuracy often varies widely between countries, food items and years. Across crops, livestock and aquatic production, data have both common traits and distinct challenges in the global data landscape. Identifying these data blind spots in production statistics is an essential first step towards comprehensive and up-to-date data coverage on global food production. This section describes the current state of data availability and deficiencies across different food sectors in order to inform targeted efforts to fill critical data gaps.
Crop production
Information on the location, timing and productivity of crop production is important for various applications, including yield forecasting, land use planning and environmental impact assessment (Fig. 1). There is a varied understanding of global patterns of crop harvest and productivity owing to a reliance on census-based survey data to quantify cultivated extent and productivity. Surveys use varied sampling methodologies and resources, constraining standardization.
National government agencies (such as ministries of agriculture) are typically responsible for collecting, processing and disseminating food production data within their countries19, and many are working to update their methods of collecting and standardizing agricultural statistics systems. To better standardize these efforts across countries, the Food and Agriculture Organization (FAO) of the United Nations coordinates the World Program for the Census of Agriculture (WCA), which provides agricultural census guidelines for different countries and reviews their practices20. FAO compiles the national agricultural census from each country and makes it publicly available through the FAOSTAT21 database, which provides open-source agricultural data from 1961 onwards22. Similarly, EUROSTAT23, the European Union (EU) statistical organization, provides a wide range of socioeconomic and environmental data for member countries of the EU through its open data portal24.
Although substantial progress has been made in gathering and sharing agricultural data through these (and other) national and international efforts, census methodologies and dissemination vary across different countries22,25 (Fig. 2). This difference stems from variations in resources, the importance of agriculture, data needs and the agreements between countries, FAO and EUROSTAT. For example, EUROSTAT can only report data for which the EU has an agreement with the member states. Thus, even if a member state collects far more detailed data, it might not enter into the database as per an agreement with the EUROSTAT agency. The FAO is similarly bound by agreements with individual countries, on whose reports they rely. Partly as a result of this reliance, the categorical and spatial variation in crop area and yield in different agroecological zones is poorly captured in FAOSTAT and other international and global datasets compared with national census portals.
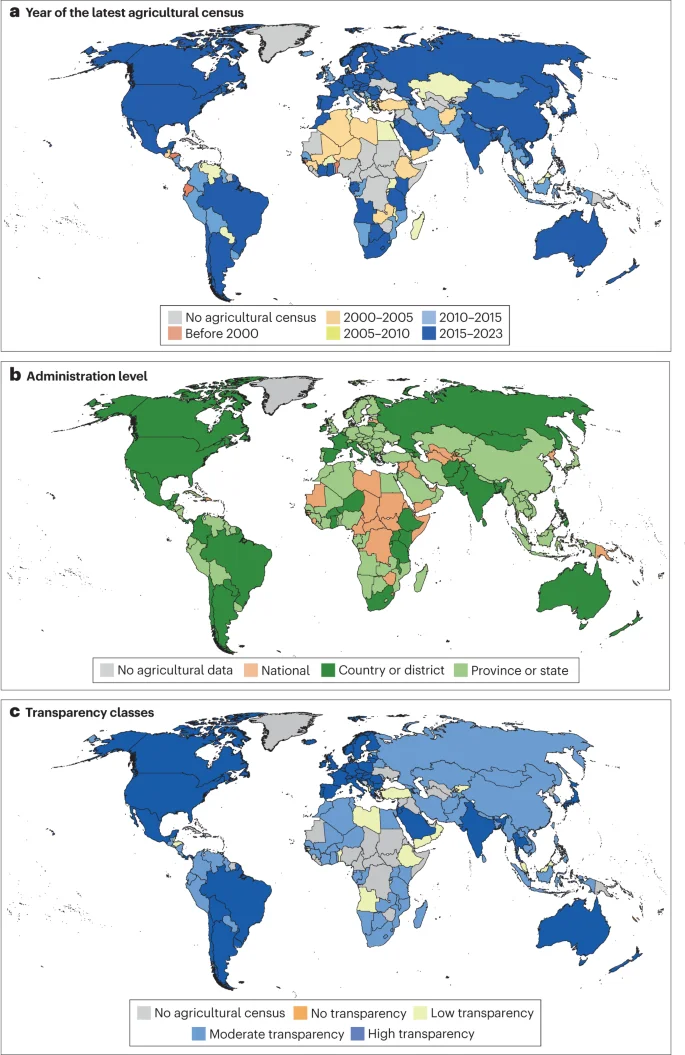
a, The year of the latest publicly available agricultural census, as assessed through the FAO’s World Program for the Census of Agriculture portal. b, The finest administration level of publicly and easily accessible statistics. c, The transparency of the agricultural census, as evaluated based on FAIR (findable, accessible, interoperable and reusable) criteria (Supplementary Information). Agricultural census reports were not considered if there was no metadata as they were not considered publicly available. The persisting data challenges seen in many countries prevent an up-to-date, comprehensive and spatially refined understanding of current food production patterns and trends.
Crop calendars are also an integral component of current and future solutions to agricultural data scarcity. Derived from censuses, models and remote sensing applications26, they define the dates for different stages of crop cultivation, including planting and harvest. Among other uses, crop calendars are mainly utilized when monitoring crop conditions, forecasting and estimating crop yields, and monitoring crop conditions27. Existing crop calendars with global coverage include those produced by the Group on Earth Observations’ Global Agricultural Monitoring (GEOGLAM) Crop Monitor, the US Department of Agriculture — Foreign Agricultural Service (USDA-FAS), the FAO, the European Commission Joint Research Center’s Anomaly hot Spots of Agricultural Production (ASAP), the MIRCA2000 dataset18 and the dataset published by Sacks et al.28. These data are typically provided at the national or subnational level (administrative levels 0 and 1); at this resolution, calendars are unable to capture regional variations at the sub-agroecological zone level that have on-the-ground effects on cropping dates. Resolution for some crops has been enhanced to increase spatial detail29,30,31, but comprehensive higher-resolution crop calendars are limited to a few major staples such as rice, soybean and wheat. Improving the spatial granularity, crop diversity and harvest date accuracy of published crop calendars can strengthen derived agricultural products, policies and food aid mobilization.
A comprehensive review of each country’s latest agricultural census for recency, spatial detail and transparency reveals clear regional patterns (Fig. 2). Limitations prevail in Central America, the Middle East and Africa (Fig. 2a), where nations continue to face issues in conducting regular censuses and meeting fundamental agricultural data needs32,33. For many countries in these regions, especially in Africa34,35, these findings align with FAO assessments that indicate steady declines in government capacity to conduct censuses, and in the quality and quantity of national agricultural statistics reporting, since the 1980s. In countries where agricultural censuses are not available or are not carried out regularly (Fig. 2a), these increasingly outdated snapshots of the magnitudes, spatial patterns and temporal trends of crop production risk mismanaging agricultural resources and misinforming interventions in the pursuit of rural development and food security goals.
The degree of spatial disaggregation in crop statistics also varies widely between countries (Fig. 2b), hampering targeted action. Some countries, such as the USA, Brazil, India and Australia, gather and provide agricultural data at fine spatial scales (such as county or district level) and categorical detail (distinguishing individual crops vs. aggregating in crop groups). However, for many countries, publicly available agricultural data is only at coarse administrative levels (such as state, province or national levels). This coarser resolution data can fail to capture the spatial variability of crop production statistics36, especially for countries dominated by smallholder farms37. Even when subnational data exists, the underlying administrative units can change over time owing to renaming, splitting, merging or aggregation. As such, reconciling spatial consistency of statistics through time is required, a challenge if the original unit names change. Further, some countries exercise data privacy, restricting access to microdata critical for administrative-level estimates without agreements or payments. If administrative data risks privacy breaches, it can also be suppressed or combined across units.
The level of agricultural census transparency of each country also varies. This transparency can be assessed using FAIR (findable, accessible, interoperable and reusable)38 principles (Fig. 2c; see Supplementary Information for a detailed description of each criterion and its corresponding score), specifically using country-specific information from the FAO’s WCA portal on metadata, census reports, questionnaires and methodological reports. Encouragingly, most of the countries following WCA guidelines have moderate-to-full transparency, although a few countries (including Ethiopia, Oman, Yemen, Libya and Turkey) have overall low transparency of agricultural census reports.
Despite the existing challenges in data availability and transparency for crop production, multiple global and regional efforts have mapped spatial patterns and temporal trends of cropped areas (Table 1). For example, several global crop-specific harvested area and yield datasets have been developed by combining census statistics with remote sensing data18,39,40,41. These datasets include the most comprehensive global gridded datasets on harvested area and yields for 175 crops (M3-Crops)40 and monthly irrigated and rain-fed cropped areas (MIRCA) for 26 crop classes18. However, these and other global datasets are centred on the year 2000 and are becoming increasingly outdated. Yet, despite the dynamic nature of crop production patterns, most agricultural and environmental assessments still use these ageing datasets owing to a lack of suitable alternatives42,43,44. Updated and current, time-varying and spatially detailed information on cropped areas and yield is urgently needed to support targeted and informed decision-making.
Some ongoing efforts — including the GAEZ45 (Global Agroecological Zones), SPAM16 (Spatial Production Allocation Model) and CropGRIDS datasets — are attempting updates but face constraints from underlying statistics. Emerging remote sensing datasets also attempt to provide updated global cropland extents46,47,48 at fine spatial resolutions. However, accurately estimating actual cropland area and distinguishing crop types remain challenging. Spectral and temporal similarities between cropland and grassland often cause poor cropland identification, especially in less intensified regions such as Africa32,49. The substantial resources required to support ground-truth data collection and computational needs are also considerable constraints on purely remote sensing approaches. At present, a fusion of survey-based census data, modelling and remote sensing offers the most promise for resolving the challenges of comprehensive global crop mapping.
Livestock production
A wide range of data on livestock populations, distributions and production appears readily available (Table 1) (for example, Livestock Data for Decisions). However, the livestock data landscape is far more complex — covering a spectrum of production systems and degrees of intensification — than this apparent widespread availability of data products would suggest. For domesticated livestock species, country-level data on animal numbers and production levels are accessible in FAOSTAT, compiled from a wide range of sources including national censuses, surveys and estimation procedures. FAOSTAT data offer valuable comparability across and between countries and regions, near-global coverage and an annual time series dating back to 1961 for most variables. As such, national FAOSTAT data on livestock production have been, and will continue to be, used in innumerable analyses wherein data comparability, broad or global coverage, and temporal trends are deemed to be important.
Although useful, FAOSTAT data has multiple limitations. Country-level data can mask substantial subnational heterogeneity and rapid local changes between infrequent national surveys. This situation is increasingly relevant for rapidly expanding research and policy applications, for example, in development research, animal health, economics, environmental adaptation and mitigation science50. For more spatially explicit research, the Gridded Livestock of the World (GLW)51,52 dataset is the global standard, mapping populations of cattle, buffalo, horses, sheep, goats, pigs, chickens and ducks in 2010 and 2015. GLW is based on national census data downscaled to a spatial resolution of 5′ (or about 10 km at the equator) and allocated spatially using a set of suitability layers and other spatial predictors50. Beyond GLW, there are few other livestock mapping efforts, excluding those that are highly localized. National livestock census data are key to efforts such as GLW, and although such data are available for many countries, their quality, resolution and timeliness are highly variable, and considerable efforts have to be expended on collation, harmonization and standardization before they can be used53,54. The date of census data collection is also highly variable: the census data in GLW version 4 ranges from the early 1990s to 2019, with all data at the pixel level being harmonized to the national-level FAOSTAT data for the years 2010 (GLW3) and 2015 (GLW4)52.
Other widely used global livestock datasets include the Global Livestock Production Systems (GLPS), the Global Environmental Assessment Model (GLEAM)21,55,56 and the Herrero et al.57,58 dataset on livestock biomass use, production, feed efficiencies and greenhouse gas emissions. Developed for 2003 (ref. 59) and later expanded58, GLPS classifies livestock systems into 11 to 14 types mapped using proxies from a non-spatial livestock classification scheme60. A limitation of GLPS is the lack of detail on mixed crop–livestock systems, partly owing to inconsistencies across crop and livestock datasets. Unlike the GLPS, the farming system mapping by Dixon et al.61 is not derivable from spatial data. The Herrero et al.57 dataset harmonizes livestock populations and milk and meat production data with year 2005 and 2010 FAOSTAT data and spatially downscales biomass use and GHG emissions using plausible feed rations. Beyond these datasets, few (if any) alternatives exist for comparative global or regional assessments. The GLEAM model uses a similar workflow but different methods and resolution, and it does not attempt to harmonize all FAOSTAT statistics. This tool is mainly designed to assist countries in their preparation of nationally determined contributions.
In addition to national censuses, household survey datasets such as the Living Standards Measurement Studies (LSMS) and Rural Household Multi-Indicator Surveys62 (RHoMIS) provide livestock information. Contemporary rounds of LSMS contain a comprehensive livestock module and enable a range of analyses of the contribution of livestock to livelihoods63. RHoMIS uses a modular approach to household data collection, with modules for various agricultural activities (including livestock), and contains data for around 45,000 households across 36 countries. These datasets are useful for analyses that do not require complete coverage, but their sampling designs can limit spatial analysis50.
Major livestock data gaps remain, especially in lower-income and middle-income countries. Ruminant diets comprise diverse feed resources — grasslands, crop residues, supplements and fodder — which have been understood through surveys, but incomplete coverage hinders many types of analyses (including life cycle assessments and environmental footprint accounting). There are also gaps regarding the number and distribution of different animal breeds, GLW3 infers dairy and dual-purpose (milk–meat) production from other data, and there remains a persistent lack of detailed distribution datasets of even broad classes of livestock, such as cattle. Promising opportunities to fill gaps include digital data collection in real time — via mobile and social media and sensor technology — and the use of crowdsourcing and other participatory methods64,65,66, in combination with remote sensing and artificial intelligence tools67.
Aquatic food production
Fish and other aquatic foods are a highly diverse and often understudied food sector, comprising over 3,200 species and species groups caught and farmed in marine, brackish and inland environments68. Moreover, small-scale to industrial producers use a wide range of fishing and farming methods. As a result, monitoring production across the sector requires compiling information from a wide range of actors and governmental agencies. The Coordinating Working Party (CWP) on Fishery Statistics provides standardized definitions, methods and minimum requirements for reporting fisheries and aquaculture statistics at a global scale. Member organizations of CWP on Fisheries Statistics mainly report data through the STATLANT system of questionnaires, with data generally collected through national and regional census-based and sample-based schemes69. FAO reports or calculates estimates for country-level production; when data are not reported or only partially reported, the FAO uses the best available information from alternative sources (including those from regional fishery bodies in the case of capture fisheries) to implement estimates68. The FAO freely provides global fishery and aquaculture data through bulk downloads and the FishStatJ computer application and summarizes this data in the biannual State of Fisheries and Aquaculture report produced by the Committee on Fisheries. Although there has been great progress in the data available on aquatic food production, there are still substantial data gaps for both capture fisheries and aquaculture.
Capture fishery production data are reported for 2,647 species and species groups by marine, brackish and inland habitat type70. Although national statistics generally include finer resolution of where fish are caught, FAO statistics are reported as a catch within one of 19 major fishing regions. The limited geographic resolution of catch obscures the extent of distant water fishing operations — or harvest occurring outside the waters of the fishing country — as fishery production is generally attributed to the fishing vessel flag state irrespective of where the fishing occurs69. Other efforts to spatialize catch include those which build on FAO statistics71,72, and those based on remotely detecting fishing activity3 wherein remote detection stems from thousands of fishing vessels continuously broadcasting their Global Positioning System (GPS) position and identity via the Automatic Identification System (AIS) or national Vessel Monitoring Systems (VMS) on a daily basis.
Fishery catch statistics are generally reported as nominal catch, which represents the live weight equivalent of landed catch. Nominal catch aims to represent the contribution of fisheries to the economy and provision of food. It does not, therefore, include organisms caught and discarded, catch utilized prior to landing (for example, consumed by the crew or used as bait) or landings that are rejected or dumped69. A notable difference in the collection of catch statistics compared with other food subsectors is that catch data is a critical input for stock assessments, which inform management for many industrial fisheries and creates an incentive for collecting quality production data that is unique to fisheries. Catch data collection methods vary across industrial and small-scale fisheries, leading to differences in the comprehensiveness of catch data. Operators in industrial and semi-industrial fisheries often report collected data to a fishing authority as part of licensing and reporting requirements, which forms the basis of census-based schemes69.
Although industrial fisheries are responsible for three-quarters of global catch73, the majority of fishers are engaged in small-scale and artisanal activities, resulting in geographically dispersed catch often governed by local communities. Consequently, estimates of catch by small-scale and industrial producers are often survey-based, with uncertainty around the degree of coverage for this subsector69. Illegal, unregulated and unreported (IUU) catch is also poorly captured by official statistics. Reconstructions of marine catch data estimate that global catch is 50% higher than reported in FAO74, whereas comparisons with household surveys indicate that inland catch is approximately 65% higher than what is reported75. However, FAO global values fall within the uncertainty of reconstructions76. FAO also engages in addressing IUU and smaller-scale catch in other ways, including the Global Record of Fishing Vessels, Refrigerated Transport Vessels and Supply Vessels, and the development of voluntary guidelines. In addition to catch weight, catch value is often important information, but it is not currently included in international statistics70, in the same manner as it is for aquaculture.
Similar to wild capture fisheries, data on aquaculture production are largely reported in live or wet weight, with 652 reported species units in FAO statistics70. As of 2020, aquaculture accounted for over half of all aquatic food production (seaweeds included), but the data from 70% of aquaculture-producing countries consisted entirely of FAO-estimated species production (in which data are not reported or only partially reported by the producing country, requiring alternative sources). This level of uncertainty is higher than for wild capture, in which only 22% of values are estimated. Furthermore, the amount and number of organisms farmed are probably underestimated owing to data limitations70. Notably, aquaculture usually is not regulated by a government-issued, standalone regional entity and instead falls into agriculture and/or a fishery agency or body, which can create data gaps and errors.
Aquaculture shares attributes (and resources) of agriculture and wild capture fisheries, including where and how it is produced. Aquaculture can operate on private property (for example, freshwater) or common-use areas (such as oceans). Although not unique to aquaculture, there are also myriad ways to grow different organisms that vary between countries, farms and species, including sourcing seed from the wild (capture-based aquaculture), using different technologies (for example, recirculating systems vs. open pens) and levels of intensity (for example, extensive vs. intensive)77. Although data on production practices is a collective challenge faced by all sectors, where it occurs can introduce unique problems because data collection tends to be a function of a region’s regulatory requirements of reporting. At the most basic level, differences in what is defined as ‘aquaculture’ (FAO has a standard definition, but it does not necessarily match the regional reporting body) or what units are reported (for example, pieces vs. bushels) can differ from one agency to the next, introducing data issues78. As a result, some core measures reported in other sectors are absent from global aquaculture, in particular yield.
Yield provides a unifying measure of scale and productivity over time, but there is a dearth of information for aquaculture compared with agriculture. One major reason for a lack of yield information is probably the result of little to no information concerning the spatial location and extent of existing farms. There is currently no global map of freshwater aquaculture. A map of most marine aquaculture around the world (excluding seaweeds) was published in 2022 (ref. 79), but it does not account for changes over time and is unable to discern active sites (vs. pre-leased or fallowed). Combining production values with spatial estimates from reported (agencies or farmers) or observational sources (such as from remote sensing80) can help fill this gap, especially in areas with high densities of aquatic farms. However, other factors such as feed, feed conversion ratio and grow-out mortality all influence yield and a broader understanding of sustainability but remain extremely heterogeneous and underreported.
FAO fishery and aquaculture production data serves as the backbone for countless peer-reviewed papers, reports and databases. For example, FAO marine fishery data underpins catch reconstructions in the Sea Around Us database73. Many limitations of fisheries and aquaculture production data noted above are related to issues with national reporting that are beyond FAO control. Nevertheless, global fishery and aquaculture data provision can be improved through more detailed metadata, particularly as it relates to data provenance, transparency in assumptions and uncertainty and documenting changes to data through release notes. Tracing data back to its origins is critical for understanding assumptions in the data collection, detecting errors in the data and linking data with other national data sources. Thus, relaying the data provenance in the metadata is a critical first step. In any data management and modelling exercise, there are numerous sources of assumptions and uncertainty that are important for appropriately interpreting data. In the case of fisheries and aquaculture data, more detailed flags on value estimation and reporting as applied to live weight conversion factors would improve transparency of assumptions, whereas reporting measures of uncertainty is important for users to capture uncertainty within their own applications of the data. Finally, as the data is regularly improved over time, it would be valuable for legacy data and data release notes detailing the changes to be maintained in a visible location. This record would facilitate reproducibility of analyses based on older versions of the data and would improve communication of the changes and improvements to the database itself.
Challenges with global food production data
The current state of food production data scarcity is characterized by substantial variation in quality and detail across countries, time and food products. Although there are a growing number of publicly accessible data sources — largely owing to a combination of increased global participation in cross-disciplinary research and development of agricultural and information technologies — technical, institutional and policy barriers remain to increase collection, dissemination and use of food production data globally.
Technical challenges
Food production data is prone to quality issues such as sampling, processing and coverage errors which can substantially undermine the credibility of census reports. Beyond simply creating these datasets, a major challenge for their downstream use is the statistical sampling of the datasets. As surveys will probably continue serving as the primary data source on food production, targeted improvements to survey design, analysis and data-sharing practices are essential to fill key gaps.
Crops
Many publicly accessible agricultural datasets are collected using convenience or opportunistic sampling (or in the case of farmer surveys, from whomever responds). This approach results in datasets that are biased, poor quality and not representative of the data population64. Not enough attention has been given to addressing this issue, with more emphasis on retroactive adjustment than anticipatory choice in the early enumeration stage64. For map-relevant and well-sampled datasets that can be used for proper accuracy assessment and agricultural statistics (for example, production or area), sampling techniques such as simple random sampling, stratified random sampling or systematic sampling must be used81,82. In addition, datasets should be documented with as much detail as possible about the data collection procedures, choices made, expertise of annotators, any quality assessment and control (QA/QC) performed, and other important information influencing data quality and interpretation. For geospatial and remote sensing datasets in particular, large globally distributed datasets of cropland have resulted from land cover and land use mapping initiatives, which typically include cropland as a class. The presence of cropland (broadly defined as land used for growing crops) can in most cases be determined from inspecting high-resolution remote sensing images, but there is still inconsistency around what constitutes cropland across datasets. For example, some datasets define cropland to include tree crops like palm or coffee, whereas others do not83.
These challenges are far greater for data collection with more fine-grained categories than the presence or absence of cropland, such as crop type, cultivation practices (such as tillage, cover cropping and irrigation), nitrogen or other input use, livestock stocking rates or pasture management, pests or disease, and fallow status. Annotators must visit the data locations in situ during the relevant time of the growing season to ground truth the observed category. For some categories — for example, crop type in intercropped fields, level of tillage or cover crop variety — more detailed annotation is needed to effectively use the data in downstream applications. Collection of field-scale yield data is particularly challenging, very expensive and error prone64,84. In some cases, such data are recorded in some form by farmers, farming equipment or equipment companies, but these data are not typically available to the public or research community.
Livestock
Despite the multi-dimensional importance of livestock to the livelihoods of at least 1.3 billion people, the critical role of the sector has never been reflected in development assistance, research outputs or the data landscape. From a data perspective, for instance, data collection approaches in livestock systems have not developed substantially over the past ~40 years (ref. 64), although the methods of data collection have evolved (high-resolution remote sensing, drones, tablets and smartphones).
Despite their advances, higher-resolution remote sensing methods for estimating livestock populations and gathering data on many livestock-related management variables still face considerable challenges. First, defining the nature and extent of grazing systems and the complexity of associated land cover (including pasture, browse, bare land and all gradations in between) is challenging, and difficulties separating land use from land cover lead to a broad range of estimates of the extent of grazing systems locally and globally85. Second, in many lower-income and middle-income countries, mixed crop–livestock systems predominate. These systems involve crops and livestock occupying the same or adjacent areas, and globally, 70% of farms are less than 1 ha in size86, further complicating the robust characterization of the livestock and crop components of small-field mixed systems. Third, unlike with crops, transboundary issues are particularly relevant for pastoral systems (nomadic, transhumant, agro-pastoral). Although there are challenges in assembling meaningful national data on livestock populations in many countries, databases on animal numbers, locations and movements are essential for preparing for, managing and mitigating the risks of certain transboundary diseases that could have high potential impacts, such as foot-and-mouth disease87. Finally, even where data do exist, there can be complex issues concerning legitimacy and accessibility in many situations, highlighting the sociocultural challenges and power asymmetries that can militate effective data sharing88,89.
Fisheries and aquaculture
The wide ranging, mobile and relatively invisible nature of fisheries make accurate and consistent fisheries data collection challenging, time intensive and costly. Numerous stakeholders can be involved in data collection, and data is often recorded using paper-based logs and/or on-board observer programs that suffer from very low fleet coverage90. Furthermore, the huge variety of harvested marine species makes accurate species reporting a challenge. Although FAO capture fishery data includes 2,647 categories, many of the largest categories by volume are highly aggregated. In 2020, 10.5 million tonnes, or approximately 13% of global production, was categorized as “marine fishes not elsewhere included”70. Electronic monitoring is emerging as a promising strategy for comprehensive catch monitoring, including bycatch and discards. However, a review of over 100 electronic monitoring trials and programs has found challenges with data quality, storage and transmission, as well as overall system failure and prohibitively long data review times91.
Vessel tracking systems detect and characterize industrial fishing activity3,92, but their use varies by region and fleet. They are generally used in larger vessels (>24 m) from upper-income and middle-income countries that have adopted AIS measures stricter than the Convention for the Safety of Life at Sea (SOLAS), the international regulation governing AIS, which explicitly exempts fishing vessels. Only around 2% of the world’s 2.8 million fishing vessels and less than 0.4% of vessels under 12 m broadcast their position over AIS93. AIS devices can also be manipulated or turned off, often without penalty, obscuring fishing activity and potential IUU catch94. Additionally, not all AIS messages that are broadcast are recorded owing to variable terrestrial coverage and satellite reception. In areas with high vessel density — such as the South China Sea, Mediterranean Sea and Gulf of Mexico — AIS messages interfere with one another, preventing them from being recorded by satellites. VMS systems, which generally carry strict penalties for tampering, are proprietary and data are rarely shared publicly in usable formats93,94. Additionally, there is no standard format for VMS data, and efforts to merge data from multiple sources face challenges associated with different broadcast intervals, schemas, metadata and units95.
Satellite imagery, although useful for large-scale detection of fishing vessels96,97 and aquaculture farms98, have several limitations and technical challenges specific to marine applications. Orbital mechanics and satellite reception result in variable spatial and temporal coverage, as most public earth observation satellites, including the important Landsat and Copernicus missions, have multi-day revisit frequencies and do not image the open ocean. Small-scale vessels have lower tendency to be detectable in these imagery collections owing to insufficient pixel resolution, and suitable high-resolution imagery (<1 m) from commercial providers such as Maxar and Planet Labs can be cost prohibitive at even moderate spatial scales. Synthetic Aperture Radar (SAR) is a proven method for detecting vessels at sea97 and multiple forms of aquaculture99,100. However, the complexities of SAR images complicate the ability to discern vessel characteristics, such as gear type, and radar signals are reflected by the water’s surface, limiting their utility for sub-surface aquaculture detection. Optical imagery, particularly at high resolution, offers increased potential for detecting and classifying at-sea vessels and aquaculture but is limited by weather (such as clouds) and daylight. Yet, infrared imaging radiometer suite day and night band optical remote sensing images can be an effective source of information capturing vessel lights, especially fisheries that use lights as a harvest strategy (such as for squid)98,101. However, remote observations are not direct measures of production and cannot provide information on species composition unless paired with additional data, such as from logbooks. The most effective approach is to combine a variety of observational sources, but it is also computationally intensive102,103.
A further technical challenge for fishery and aquaculture data is that there is no universally accepted distinction between small-scale and industrial fisheries. Definitions of the sectors are based on a range of characteristics and vary across countries, resulting in inconsistent inclusion of small-scale production in national reporting requirements104,105. Modern fisheries management, which developed largely in response to industrial fishing, has often deprioritized small-scale producers in data collection efforts and exempted them from self-reporting. Similarly, there is no uniform definition of small-scale aquaculture, as production methods and scales differ considerably across regions, and aquaculture development has often proceeded ad hoc in the absence of clearly defined property rights in the ocean. Efforts at defining small-scale aquatic production are complicated by its distributed nature, coupled with limited budgets and capacity for monitoring and reporting106.
Institutional and policy challenges
Multiple institutional challenges obstruct comprehensive food production data collection and curation. A major challenge related to data collection is the lack of consistency and duplication of effort between various agencies and organizations, including government, research institutions and international non-governmental organizations. In some countries, there is also ambiguity about an institutional mandate for collecting and disseminating food production data107. Data collection efforts are often siloed within individual departments or institutions, and even within the same broader institution (for example, federal government), leading to a lack of coordination and sharing. For example, fisheries and aquaculture are often managed by different agencies or ministries and often not the ministry of agriculture. This management structure creates potential reporting gaps and mismatches in the available information. Furthermore, institutions that are (or could potentially be) in charge of collecting data (for example, agricultural ministries) also often lack the capacity and expertise to adopt innovative methods for data collection or data provisioning, particularly in low-income countries64.
Sharing of food production data between countries and/or organizations is often challenging, worsened by inconsistent data privacy protocols and platforms108. The lack of coordination among national and international organizations results in poorly harmonized agricultural data sources33. Some data collection efforts are integrated with the data users (for example, agricultural statistics agencies), but in many cases, the data collector and the data scientist are also separated, leading to a gap in needs from both sides. There is a need for more cross-institution and cross-disciplinary collaborations throughout the data life cycle to address these gaps. Such collaborations could also increase the likelihood that a dataset is hosted and maintained over long periods of time, for example, beyond the duration of a funded project at a particular institution, and that the dataset is provided in formats interoperable by a wide range of users.
Similarly, policy silos exist within and across countries and institutions in the public and private sectors. These silos make it difficult to aggregate data and derive meaningful insights even within one country109. Policy silos also impact which data are prioritized for collection and which are overlooked110, and contribute to issues related to data privacy and sharing. Different institutions have varying policies around data privacy and sharing, leading to inconsistencies in how data are collected, stored and shared. The lack of coordination and inconsistencies in data collection, management and analysis lead to a fragmented data landscape. For instance, some institutions might collect the personal information of farmers without their consent, whereas others might not collect this information at all. In addition, geo-referencing data is crucial for satellite data analysis, but a lot of critical data is collected without geo-referencing, limiting their utility108. Moreover, some institutions do not share data with other stakeholders owing to concerns about data privacy and security, whereas others might be more open to data sharing. These mismatches further exacerbate the problem of fragmented data, making it difficult to derive comprehensive insights into food production and food security111,112.
Perhaps the most critical obstacle hindering systematic agricultural data collection by government organizations — particularly in sub-Saharan Africa — is the lack of steady and sufficient public funding. Without dedicated long-term financing to develop centralized data infrastructure and standards, data collection efforts remain siloed, sporadic and disjointed across various agencies. As a result, the assembly of high-quality, consistent datasets needed to inform policies and interventions are severely impeded. Indeed, despite growing recognition of the importance of food production data, funding gaps hinder the implementation of mandates on data collection, management and sharing, particularly in low-income and middle-income countries. It also limits the adoption of new technologies, such as remote sensing and artificial intelligence and machine learning, which augment traditional monitoring and assessments. Overcoming funding inconsistencies is the foremost challenge that must be addressed to improve inter-institutional coordination, reduce duplicated efforts, build capacity and establish sustainable data systems. Concerted efforts are needed to secure enduring financial support that enables a more integrated, effective approach to food production data gathering across sub-Saharan Africa and other regions facing data scarcity challenges. No other intervention would be as broadly impactful in overcoming current data deficiencies as establishing the consistent financing required for strengthened systems and collaboration across governmental bodies.
Agricultural research and development organizations accumulate vast amounts of data annually from numerous farmer surveys and field trials. However, despite the substantial efforts and costs involved in collecting these data and the value of these data for research re-use, only minimal infrastructure currently exists to systematically document, share and curate this information. For example, only a small fraction of the data gathered by the wider research community readily adhere to FAIR (findable, accessible, interoperable and reusable) guidelines. Although some of the challenges are institutional, such as limited open access and interoperability112, targeted improvements in data governance could help overcome these hurdles. Specifically, coordinated development and application of data documentation, dissemination and formatting standards, alongside raising awareness of FAIR principles, could greatly enhance preservation and utilization of survey data assets. Initiatives are needed across local, national and international levels to implement improved governance that simultaneously establishes community data sharing norms and builds capacity for proper data curation. By tackling obstacles in unison through governance that advances standards and education, the vast potential of accumulated survey data to inform agricultural research could be more fully realized.
Private sector investments can lead to improved productivity and efficiency, including investments in data collection that benefit the public and private sector99. However, large volumes of data and insights increasing in the hands of the private sector — with no clear policies and regulations around who can collect what data and when — can disadvantage farmers (often smallholders and rural communities) and expose them to exploitation100. Moreover, companies rarely share data publicly, preventing access to relevant and accurate data and limiting the development of comprehensive policies and strategies. Further, contextually irrelevant policies that do not consider the characteristics of the target population often lead to ineffectiveness, unintended consequences, lack of buy-in, waste of resources and inequity113.
For livestock, aquaculture and fisheries, substantial differences in country-specific regulations are ill-equipped to address transboundary issues, such as diseases and climate change, which can substantially affect international trade access and domestic food security87. Successful monitoring and control of transboundary diseases is greatly dependent on governmental cooperation, something that can be made considerably more challenging with incompatible or inconsistent surveillance systems and regulatory frameworks in the countries that tend to be affected87. Furthermore, digital information sharing comes with critical political economy considerations, including data ownership, control and security114. Similarly, national agricultural data governance frameworks do not always reflect the concerns and expectations of farmers regarding ownership, control and privacy115.
Transboundary and diseases-related issues with aquaculture largely mirror those with livestock production, but potentially have a higher concern over the risk of escapees and their potential impact on local ecosystems, including wild capture fisheries116. Fisheries face additional challenges as stocks can span multiple jurisdictions, often requiring regional management approaches. However, as many fish stocks shift owing to climate change, new entities can gain access to fisheries, requiring renegotiation or creating potential conflict117.
Socio-technical levers for data abundance
A variety of technological and policy innovations offer new opportunities for overcoming many of the challenges causing food production data scarcity to persist (Fig. 3). However, the development and deployment of these new approaches require an enabling policy environment with appropriate incentives, regulations and benefits sharing116. As such, bundles of socio-technical innovations — which combine new technologies with coordinated policy — will be necessary for coordinating stakeholder priorities, investments and multi-scalar governance in transforming the food production datascape to the benefit of all117.
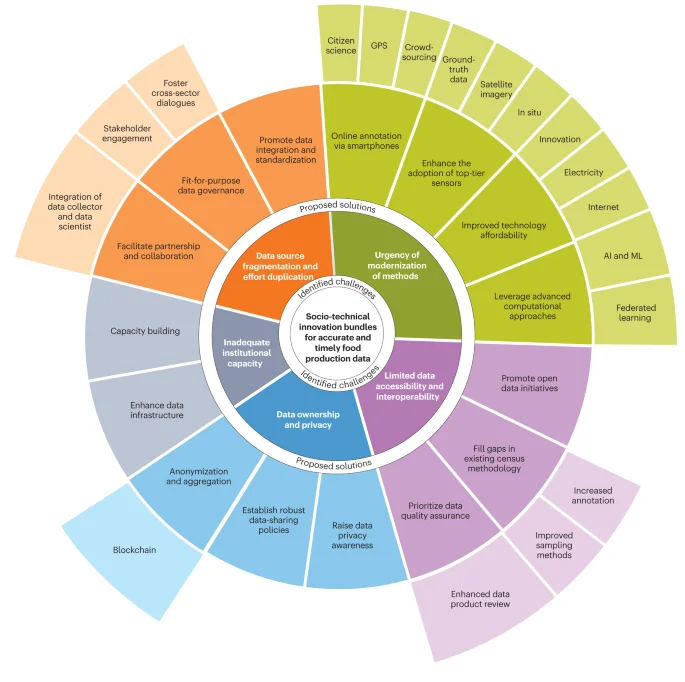
The five key challenges for transforming the food production data landscape (inner wedges) and their proposed solutions which can be utilized, in combination, to form socio-technical innovation bundles to effectively and sustainably address food production data scarcity (outer two wedges). Such coordinated efforts will be crucial for globally ensuring the accuracy and timeliness of food production data. AI, artificial intelligence; GPS, Global Positioning System; ML, machine learning.
Technological opportunities
Crops
Advanced technologies have great potential to assist data collection, but the use of this technology still depends on access to other resources (such as electricity) and governance. Such technologies can increase the scale of agricultural datasets (such as geographic and temporal coverage, and the number of data points). For instance, rapid acquisition and automated analysis of street-level images can be used to efficiently collect samples over a large area at low cost118,119,120. Commercial off-the-shelf drones or micro air vehicles are also being used to efficiently collect observations of agricultural areas at low cost121, although the use of drones in some regions can be complicated by policy or local community restrictions or regulations and important issues of trust.
Citizen science and crowdsourcing initiatives are making use of online annotation and smartphone technologies to collect large-scale agricultural datasets globally83,122. However, these projects require oversight to review novice annotations and ensure high-quality data83. The growth of agricultural technology (agtech) companies also presents opportunities for partnerships that leverage data collected by companies for commercial purposes to be used for scientific research123,124. These strategies can provide more comprehensive and diverse datasets than data collection efforts that rely on traditional data collection mechanisms, such as in situ or farmer surveys. These technologies can also provide more accurate and precise measurements using high-quality, low-cost sensors such as in situ sensors or smartphone GPS, compared with qualitative surveys or farmer recall.
Satellite remote sensing data can also be used to fill data gaps in the status and monitoring of agricultural variables; however, its utility relies heavily on high-quality ground-truth data for model calibration and validation. High-quality in situ datasets detailing the time and location that a particular agricultural category or quantity was observed can be paired with Earth observation datasets that provide environmental and biological covariates for downstream analyses. Machine learning technologies can be used with satellite remote sensing data to build correlative models that predict agricultural characteristics such as crop type, yield or cultivation practices from Earth Observations (such as reflectance in certain wavelengths, precipitation and temperature)125,126,127.
The increasingly resolved information that these technological advances promise also poses substantial challenges in terms of data privacy and ethics. Emerging technologies such as blockchain and federated learning have the potential to address privacy concerns related to agricultural datasets (for example, anonymization) while allowing such datasets to be made available for public research. Blockchain technology can be used to create a secure and transparent system for sharing data while maintaining data privacy through anonymization and ensuring that only authorized parties have access to data128,129,130. Federated learning allows for machine learning models to be trained on distributed datasets without the need for data to be centralized in a single location. This approach allows individual farmers or institutions to keep their data local and have control over its access and use131. Federated learning can also help to mitigate concerns related to data bias by ensuring that models are trained on a diverse range of datasets. Additional techniques for the anonymization of geo-referenced data (such as anonymized spatial coordinates and sample displacement) are being increasingly promoted132.
Livestock
Many of these technologies can also be applied to livestock data, including new and improved methods of data acquisition and automated data analysis for determining livestock populations and characteristics such as species and breed. Two more livestock-specific innovations with substantial potential for providing data of great utility are animal-based methane measurement (in ruminants) and precision livestock farming. Several non-invasive methods are available to measure methane production in animals. These include microbial biomarkers in the rumen that, if heritable, could be used for targeting purposes, GHG emission monitoring systems using hand-held methane sensors, and ingestible methane detection capsules and other sensors that allow continuous monitoring with Wi-Fi133,134,135. However, all these methods currently have some disadvantages such as cost, reliability and/or reproducibility, so wider uptake of these innovations tends to depend to some extent on the development of appropriate validation, calibration and standardization protocols.
Precision livestock farming is another set of innovations based on the application of process engineering principles and techniques to livestock feeding to automatically monitor, model and manage animal feeding at the individual level. The idea has been used to maximize margins for intensive livestock production for many years. However, it is developing rapidly to encompass a wide range of new monitoring and sensor technologies (the Internet of Things) and their application to the major domesticated livestock species136,137,138. For animal-based methane measurement and precision livestock farming, the future issues around data privacy and ownership tend to be just as challenging as for other agricultural data.
Fisheries and aquaculture
Advances in big data processing, machine learning and vessel tracking data are revolutionizing the ability to provide data on fishing activity at high spatial and temporal resolution. These data now underpin numerous attempts to characterize global industrial fishing activity3,91,139, examine its overlap with target and non-target species140,141, assess conservation actions142,143,144 and reveal illegal fishing97. Similar approaches have also demonstrated successful applications to small-scale fisheries145,146. Data from vessel tracking systems can be supplemented with vessel detections from space-based technologies such as radar, day and nighttime optical imagery96,97,147, radio frequency148, aerial surveys149 and drones150 to better assess fleet size and distribution and monitor IUU fishing.
Onboard vessels, remote electronic monitoring with video cameras can reduce cost and speed of collecting data (especially for non-multispecies fisheries), compared with solely onboard observers and logbooks. Remote electronic monitoring improves coverage of a fleet and enhances compliance around fishing activities and location90. It can also provide these benefits to small-scale fisheries, but it can be less effective in filling in some of the essential data gaps around bycatch and can be affected by species type and haul size (larger catches reduce accuracy)151. However, there is reluctance and lack of adoption owing to issues from perceived intrusion of privacy by the industry, equipment and data storage requirements, and equipment challenges in the harsh marine environment (such as corrosion)147.
In aquaculture, similar hurdles exist for more precision or smart farming data-driven approaches, especially in poorer or more rural regions where access to electricity or the Internet is not reliable152,153,154. Indeed, high-income countries, such as the USA, probably have a greater capacity to improve their aquaculture data more quickly with the right internal coordination and support, especially across the diverse agencies tasked or interested in aquatic commodity data collection78. Ultimately, whether this improved data collection is incorporated into FAO statistics depends on several factors, including financial support and incentives or mandates by local or regional governments to use certain technologies.
Complementary policy innovations
As technology advances and new solutions emerge, policymakers must balance continuity with innovation to sustain effective food production data systems while exploring new approaches to address emerging challenges. A stable and effective policy framework is crucial, but policymakers must also consider new ideas and approaches that build upon existing strengths without undermining existing systems56,155. Thus, it is important to highlight pathways forward for improving global food production data availability and quality.
Collaboration and coordination among countries and institutions are essential to developing comprehensive policies and strategies for food production data collection, management and sharing. However, the proliferation of policy frameworks and actors in the data ecosystem can lead to fragmentation and duplication of efforts, hindering progress towards common goals. Therefore, it is important to ensure policy alignment and harmonization across different levels and sectors to avoid conflicting regulations and to promote a coordinated approach156,157. One potential solution is to invest in greater collaboration and coordination among countries, institutions, initiatives and research fields (agricultural economics, statistics, satellite Earth observation, and emerging artificial intelligence and machine learning) to reduce duplication of efforts and enhance initiatives that often operate independently. Another possible solution is to establish a global platform for data sharing and coordination, enabling countries to share best practices (including standards and benchmarking) and data, which could facilitate the development of comprehensive policies and strategies for food production data management that consider unique contexts and characteristics of different countries and populations158.
In addition to increasing the scope of data collection and monitoring, it is essential to have fit-for-purpose data governance systems in place. In view of the rapidly changing data landscape, the 2021 World Development Report159 on datasets highlights the need for a new social contract between data providers and data users of all types, founded on value (enabling the use and re-use of data for different purposes), trust (the rights and interests of all stakeholders are safeguarded) and equity (the benefits of data are shared equally). These principles of data governance at national and international levels could enforce such a social contract around data. Although several elements of data governance occur primarily at the national level, resolution of some data governance challenges is possible only through international collaboration, such as combating cybercrime, reducing transaction costs by harmonizing legal and technical standards for data protection and interoperability, and surveilling and dealing with transboundary issues.
To ensure that the global platform for data sharing and coordination on food production is effective, it is important to develop a neutral and independent platform that is governed by a diverse set of stakeholders, including representatives from governments, private sector, academia, civil society and farmers’ organizations. The FAO and initiatives such as GODAN (Global Open Data For Agriculture and Nutrition) and GEOGLAM are well-placed to help develop and govern a global platform for food production data, and ensure that the platform is designed to promote open data sharing and collaboration for the public good, and not for the benefit of any single entity. By leveraging their networks and expertise, they are also situated to establish best practices and standards for data sharing and to facilitate the development of policies and strategies for data management that consider the unique contexts and characteristics of different countries and populations. Further, these organizations can also facilitate the responsible sharing and use of the vast amounts of data (such as producer surveys and field trials) that a host of national and international research institutes (like CGIAR) continue to collect through hundreds of projects worldwide. These data are often collected for the purposes of a specific project but hold great, unrealized value for contributing to a more complete understanding of food production systems. Similarly, an effective global platform should also reduce redundancy, build on existing infrastructure largely within FAO, increase accessibility through digital transformations such as a smartphone-based app, and explore the feasibility of user-uploaded content including crowdsourcing and citizen science.
There is widespread consensus on the need for greatly expanded investment in data collection, collation and curation to support decision-making in the food sector. For the SDGs160 specifically, a comprehensive set of regulatory standards is urgently needed, along with the development of the necessary physical infrastructure (such as low-cost mobile broadband) and strengthened public institutions. Indeed, without rigorous monitoring of the entire food system, it is difficult to identify when course corrections will be needed and hold different food system actors accountable161. National and international funding agencies are increasingly making the publication of data an integral part of work plans and project evaluations, which is one step in the right direction. This effort could be made even more useful if the major research and R4D funders helped to promote standardized data collection, documentation and accessibility procedures, or even made them a requirement for funding.
The privacy and confidentiality of food production data must be balanced with catering to the economic interests of the stakeholders wishing to access and use those data113. Of the three elements of a fit-for-purpose social contract on data159 perhaps the most foundational is trust, which is a critical component of scalable innovation116. There is much that policymakers can do to foster trust. Setting up wide-ranging national and international dialogues on data governance would help in understanding the concerns and needs of stakeholders. These dialogues would need to be carried out in ways that are sensitive to sociocultural differences and the existence of varying power dynamics in different contexts88. There is also the need to evaluate existing regulatory frameworks and how they can be improved, at both national and international levels113. There is no doubt that achieving the balancing act between ensuring the legitimacy and security of food production data and generating added value from their use for all stakeholders involved will be very difficult to achieve. Nevertheless, striving towards such balance is vital if the full power of data and the digitalization of our food system are to be realized for maximal public good.
Summary and future perspectives
Detailed and up-to-date food production statistics are key to realizing food system sustainability and achieving multiple SDGs, but substantial data gaps persist across the crop, livestock and aquatic food sectors. This stock-take of food production data demonstrates pronounced sectoral (livestock and fisheries) and regional (Central America, sub-Saharan Africa, Middle East) deficiencies, with information being inadequate to guide a comprehensive and spatially detailed understanding of the current state of food production. A lack of political will, insufficient capacity and funding, and ineffective or inadequate international support are driving these data gaps. At best, these information deficiencies can lead to ineffective interventions and, at worst, can contribute to misinformed actions that erode the sustainability of food systems.
Agricultural, livestock and fisheries censuses will probably continue to be the primary source of information in the food production sector, and so ensuring their accuracy and reliability is essential. Restricted data accessibility and a lack of transparency in many countries are key hindrances in the global food production data landscape. Irregular intervals in census occurrence, diverse methodologies followed by each nation and a lack of standardization make harmonizing food production statistics difficult, and issues of data privacy, particularly related to access to microdata, further exacerbate challenges of accessibility and interoperability. In tandem, diverse institutional and policy obstacles — including inadequate institutional capacity, transboundary issues and policy silos — hinder the progress of collecting, disseminating and utilizing food production data in a consistent manner globally. The lack of dedicated and sustained long-term public funding remains a fundamental obstacle to systematic food production data collection.
Leveraging advanced technologies can offer an effective means of data collection and dissemination. Estimates derived from satellite remote sensing, in situ sensors and digital monitoring using artificial intelligence, machine learning and other modelling techniques can be a promising complement for filling data gaps in censuses. Complements to censuses become especially important in times of crisis such as wars or pandemics, which can delay or halt on-the-ground data collection entirely. However, the need for intensive computational resources and the ethical and privacy concerns associated with these approaches must be critically considered to ensure their responsible use. Issues of data privacy should be addressed through clear data collection and sharing policies and the application of emerging technologies such as blockchain and federated learning. Enabling institutional arrangements and policy environments will also be essential to promote collaboration and partnership among stakeholders, to foster responsible data governance and to ensure equitable benefits sharing across all food system actors. Successfully addressing all of these key factors contributing to food production data scarcity will represent a fundamental and monumental step towards realizing the full potential of food systems for achieving multiple sustainability goals.
Data availability
The data used to support the findings of this article are available in the Supplementary Information.
References
-
Loizou, E., Karelakis, C., Galanopoulos, K. & Mattas, K. The role of agriculture as a development tool for a regional economy. Agric. Syst. 173, 482–490 (2019).
Google Scholar
-
Foley, J. A. et al. Solutions for a cultivated planet. Nature 478, 337–342 (2011).
Google Scholar
-
Kroodsma, D. A. et al. Tracking the global footprint of fisheries. Science 359, 904–908 (2018).
Google Scholar
-
Carpenter, S. R., Booth, E. G. & Kucharik, C. J. Extreme precipitation and phosphorus loads from two agricultural watersheds. Limnol. Oceanogr. 63, 1221–1233 (2018).
Google Scholar
-
Willett, W. et al. Food in the Anthropocene: the EAT-Lancet Commission on healthy diets from sustainable food systems. Lancet 393, 447–492 (2019).
Google Scholar
-
Zhang, J., Wang, S., Zhao, W., Meadows, M. E. & Fu, B. Finding pathways to synergistic development of Sustainable Development Goals in China. Humanit. Soc. Sci. Commun. 9, 21 (2022).
Google Scholar
-
Searchinger, T. et al. Creating a Sustainable Food Future. World Resources Report 2013–14: Interim Findings (World Resources Institute, 2020).
-
Hoekstra, A. Y. & Mekonnen, M. M. The water footprint of humanity. Proc. Natl Acad. Sci. USA 109, 3232–3237 (2012).
Google Scholar
-
Saleem, M. Possibility of utilizing agriculture biomass as a renewable and sustainable future energy source. Heliyon 8, e08905 (2022).
Google Scholar
-
Poore, J. & Nemecek, T. Reducing food’s environmental impacts through producers and consumers. Science 360, 987–992 (2018).
Google Scholar
-
Curtis, P. G., Slay, C. M., Harris, N. L., Tyukavina, A. & Hansen, M. C. Classifying drivers of global forest loss. Science 361, 1108–1111 (2018).
Google Scholar
-
Agricultural Model Intercomparison and Improvement Project (AgMIP) https://doi.org/10.15482/USDA.ADC/1212378 (2015).
-
Warszawski, L. et al. The Inter-Sectoral Impact Model Intercomparison Project (ISI–MIP): project framework. Proc. Natl Acad. Sci. USA 111, 3228–3232 (2014).
Google Scholar
-
Becker-Reshef, I., Justice, C., Whitcraft, A. K. & Jarvis, I. GEOGLAM: a GEO initiative on global agricultural monitoring. In IGARSS 2018 — 2018 IEEE International Geoscience and Remote Sensing Symposium 8155–8157 (2018).
-
Sellitti, S. Evaluation of CGIAR Platform for Big Data in Agriculture (CGIAR, 2021).
-
Yu, Q. et al. A cultivated planet in 2010 — part 2: the global gridded agricultural-production maps. Earth Syst. Sci. Data 12, 3545–3572 (2020).
Google Scholar
-
Fischer, G. et al. Global Agro-Ecological Zones v4 — Model Documentation (IIASA/FAO, 2021).
-
Portmann, F. T., Siebert, S. & Döll, P. MIRCA2000 — global monthly irrigated and rainfed crop areas around the year 2000: a new high-resolution data set for agricultural and hydrological modeling. Glob. Biogeochem. Cycles 24, GB1011 (2010).
-
Weersink, A., Fraser, E., Pannell, D., Duncan, E. & Rotz, S. Opportunities and challenges for big data in agricultural and environmental analysis. Annu. Rev. Resour. Econ. 10, 19–37 (2018).
Google Scholar
-
Global Review of Agricultural Census Methodologies and Results (2006–2015) World Programme for the Census of Agriculture 2010 (FAO, 2021).
-
FAOSTAT Statistical Database (FAO, 2024); https://www.fao.org/faostat/en/#data.
-
Conducting Agricultural Censuses and Surveys FAO Statistical Development Series No. 6) (Food and Agriculture Organization of the United Nations, 1996); https://www.fao.org/economic/the-statistics-division-ess/world-census-of-agriculture/conducting-of-agricultural-censuses-and-surveys/en/.
-
Statistical Office of the European Union (EUROSTAT, 2023); https://ec.europa.eu/eurostat.
-
Lahti, L., Huovari, J., Kainu, M. & Biecek, P. Retrieval and analysis of Eurostat open data with the eurostat package. The R Journal 9, 385–392 (2017).
Google Scholar
-
World Programme For The Census Of Agriculture 2020 Vol. 1 (FAO, 2017).
-
Maria, D., Michele, M. & Felix, R. Development of a National and Sub-National Crop Calendars Data Set Compatible with Remote Sensing Derived Land Surface Phenology (European Union, 2018).
-
Fritz, S. et al. A comparison of global agricultural monitoring systems and current gaps. Agric. Syst. 168, 258–272 (2019).
Google Scholar
-
Sacks, W. J., Deryng, D., Foley, J. A. & Ramankutty, N. Crop planting dates: an analysis of global patterns. Glob. Ecol. Biogeogr. 19, 607–620 (2010).
Google Scholar
-
Becker-Reshef, I. et al. Crop type maps for operational global agricultural monitoring. Sci. Data 10, 172 (2023).
Google Scholar
-
Kotsuki, S. & Tanaka, K. SACRA — a method for the estimation of global high-resolution crop calendars from a satellite-sensed NDVI. Hydrol. Earth Syst. Sci. 19, 4441–4461 (2015).
Google Scholar
-
Laborte, A. G. et al. RiceAtlas, a spatial database of global rice calendars and production. Sci. Data 4, 170074 (2017).
Google Scholar
-
See, L. et al. Improved global cropland data as an essential ingredient for food security. Glob. Food Secur. 4, 37–45 (2015).
Google Scholar
-
Global Strategy to Improve Agricultural and Rural Statistics: Report of the Friends of the Chair on Agricultural Statistics (World Bank, 2010).
-
Independent External Evaluation of the United Nations Food and Agricultural Organization (Food and Agricultural Organization of the United Nations, 2005); https://www.fao.org/3/J6667E/J6667E.pdf.
-
Independent External Evaluation of FAO’s Role and Work in Statistics (Food and Agriculture Organization of the United Nations, 2008); https://www.fao.org/3/bd418e/bd418e.pdf.
-
Iizumi, T. et al. Historical changes in global yields: major cereal and legume crops from 1982 to 2006. Glob. Ecol. Biogeogr. 23, 346–357 (2014).
Google Scholar
-
Gangopadhyay, P. K., Shirsath, P. B., Dadhwal, V. K. & Aggarwal, P. K. A new two-decade (2001–2019) high-resolution agricultural primary productivity dataset for India. Sci. Data 9, 730 (2022).
Google Scholar
-
Wilkinson, M. D. et al. Comment: The FAIR Guiding Principles for scientific data management and stewardship. Sci Data 3, 160018 (2016).
Google Scholar
-
Leff, B., Ramankutty, N. & Foley, J. A. Geographic distribution of major crops across the world. Glob. Biogeochem. Cycles 18, GB1009 (2004).
Google Scholar
-
Monfreda, C., Ramankutty, N. & Foley, J. A. Farming the planet: 2. Geographic distribution of crop areas, yields, physiological types, and net primary production in the year 2000. Glob. Biogeochem. Cycles 22, GB1022 (2008).
Google Scholar
-
Ramankutty, N., Evan, A. T., Monfreda, C. & Foley, J. A. Farming the planet: 1. Geographic distribution of global agricultural lands in the year 2000. Glob. Biogeochem. Cycles 22, GB1003 (2008).
Google Scholar
-
Deutsch, C. A. et al. Increase in crop losses to insect pests in a warming climate. Science 361, 916–919 (2018).
Google Scholar
-
Lombardozzi, D. L., Bonan, G. B., Levis, S. & Lawrence, D. M. Changes in wood biomass and crop yields in response to projected CO2, O3, nitrogen deposition, and climate. J. Geophys. Res. Biogeosci. 123, 3262–3282 (2018).
Google Scholar
-
Rolle, M., Tamea, S. & Claps, P. Improved large-scale crop water requirement estimation through new high-resolution reanalysis dataset. In EGU General Assembly (2020).
-
Fischer, G. et al. Global Agro-Ecological Zones (GAEZ v3.0) (FAO/IIASA, 2012).
-
Bartholomé, E. & Belward, A. S. GLC2000: a new approach to global land cover mapping from Earth observation data. Int. J. Remote Sens. 26, 1959–1977 (2005).
Google Scholar
-
Potapov, P. et al. Global maps of cropland extent and change show accelerated cropland expansion in the twenty-first century. Nat. Food 3, 19–28 (2022).
Google Scholar
-
Klein Goldewijk, K., Beusen, A., van Drecht, G. & de Vos, M. The HYDE 3.1 spatially explicit database of human-induced global land-use change over the past 12,000 years. Glob. Ecol. Biogeogr. 20, 73–86 (2011).
Google Scholar
-
Kerner, H. et al. How accurate are existing land cover maps for agriculture in sub-Saharan Africa? Preprint at https://doi.org/10.48550/arXiv.2307.02575 (2023).
-
Meisner, J. et al. A time-series approach to mapping livestock density using household survey data. Sci. Rep. 12, 13310 (2022).
Google Scholar
-
Gilbert, M. et al. Global distribution data for cattle, buffaloes, horses, sheep, goats, pigs, chickens and ducks in 2010. Sci. Data 5, 180227 (2018).
Google Scholar
-
Gilbert, M. et al. Global Cattle Distribution in 2015 (5 Minutes of Arc) (Harvard Dataverse, accessed 11 July 2023); https://doi.org/10.7910/DVN/LHBICE.
-
Da Re, D. et al. Downscaling livestock census data using multivariate predictive models: sensitivity to modifiable areal unit problem. PLoS One 15, e0221070 (2020).
Google Scholar
-
Nicolas, G. et al. Using random forest to improve the downscaling of global livestock census data. PLoS One 11, e0150424 (2016).
Google Scholar
-
MacLeod, M. et al. Greenhouse Gas Emissions from Pig and Chicken Supply Chains: a Global Life Cycle Assessment (FAO, 2013).
-
Opio, C. et al. Greenhouse Gas Emission from Ruminant Supply Chains (FAO, 2013).
-
Herrero, M. et al. Biomass use, production, feed efficiencies, and greenhouse gas emissions from global livestock systems. Proc. Natl Acad. Sci. USA 110, 20888–20893 (2013).
Google Scholar
-
Robinson, T. P. et al. Global Livestock Production Systems (FAO and ILRI, 2011).
-
Kruska, R. L., Reid, R. S., Thornton, P. K., Henninger, N. & Kristjanson, P. M. Mapping livestock-oriented agricultural production systems for the developing world. Agric. Syst. 77, 39–63 (2003).
Google Scholar
-
Seré Rabé, C. & Steinfeld, H. World Livestock Production Systems: Current Status, Issues and Trends (FAO, 1996).
-
Dixon, J. A., Gibbon, D. P. & Gulliver, A. Farming Systems and Poverty: Improving Farmers’ Livelihoods in a Changing World (FAO, 2001).
-
Hammond, J. et al. The Rural Household Multi-Indicator Survey (RHoMIS) for rapid characterisation of households to inform climate smart agriculture interventions: description and applications in East Africa and Central America. Agric. Syst. 151, 225–233 (2017).
Google Scholar
-
Zane, G. & Pica-Ciamarra, U. The contribution of livestock to household livelihoods in Tanzania and Uganda: measuring tradable and non-tradable livestock outputs. Trop. Anim. Health Prod. 53, 304 (2021).
Google Scholar
-
Carletto, C. Better data, higher impact: improving agricultural data systems for societal change. Eur. Rev. Agric. Econ. 48, 719–740 (2021).
Google Scholar
-
Carletto, C., Dillon, A. & Zezza, A. in Handbook of Agricultural Economics Vol. 5 (eds Barrett, C. B. & Just, D. R.) 4407–4480 (Elsevier, 2021).
-
Duncan, A. J., Lukuyu, B., Mutoni, G., Lema, Z. & Fraval, S. Supporting participatory livestock feed improvement using the Feed Assessment Tool (FEAST). Agron. Sustain. Dev. 43, 34 (2023).
Google Scholar
-
Fritz, S. et al. A global dataset of crowdsourced land cover and land use reference data. Sci. Data 4, 170075 (2017).
Google Scholar
-
The State of World Fisheries and Aquaculture (SOFIA) (Food and Agriculture Organization of the United Nations, 2022); https://www.fao.org/3/cc0461en/online/sofia/2022/world-fisheries-aquaculture.html.
-
Food and Agriculture Organization of the United Nations. Coordinating Working Party on Fishery Statistics (CWP) Handbook (FAO, 2020).
-
Fishery and Aquaculture Statistics. Global Production by Production Source 1950–2020 (FishStatJ) (Food and Agricultural Organization of the United Nations, 2022); https://www.fao.org/fishery/en/topic/166235?lang=en.
-
Watson, R. A. A database of global marine commercial, small-scale, illegal and unreported fisheries catch 1950–2014. Sci. Data 4, 170039 (2017).
Google Scholar
-
Zeller, D. et al. Still catching attention: Sea Around Us reconstructed global catch data, their spatial expression and public accessibility. Mar. Policy 70, 145–152 (2016).
Google Scholar
-
Pauly, D., Zeller, D. & Palomares, M.L.D. (eds) Sea Around Us Concepts, Design and Data (Sea Around Us, 2020); seaaroundus.org.
-
Pauly, D. & Zeller, D. Catch reconstructions reveal that global marine fisheries catches are higher than reported and declining. Nat. Commun. 7, 10244 (2016).
Google Scholar
-
Fluet-Chouinard, E., Funge-Smith, S. & McIntyre, P. B. Global hidden harvest of freshwater fish revealed by household surveys. Proc. Natl Acad. Sci. 115, 7623–7628 (2018).
Google Scholar
-
Ye, Y. et al. FAO’s statistic data and sustainability of fisheries and aquaculture: comments on Pauly and Zeller (2017). Mar. Policy 81, 401–405 (2017).
Google Scholar
-
Klinger, D. H. et al. Moving beyond the fished or farmed dichotomy. Mar. Policy 38, 369–374 (2013).
Google Scholar
-
Froehlich, H. E. et al. Piecing together the data of the US marine aquaculture puzzle. J. Environ. Manag. 308, 114623 (2022).
Google Scholar
-
Clawson, G. et al. Mapping the spatial distribution of global mariculture production. Aquaculture 553, 738066 (2022).
Google Scholar
-
Ottinger, M., Bachofer, F., Huth, J. & Kuenzer, C. Mapping aquaculture ponds for the coastal zone of Asia with Sentinel-1 and Sentinel-2 time series. Remote Sens. 14, 153 (2021).
Google Scholar
-
Olofsson, P. et al. Good practices for estimating area and assessing accuracy of land change. Remote Sens. Environ. 148, 42–57 (2014).
Google Scholar
-
Stehman, S. V. & Foody, G. M. Key issues in rigorous accuracy assessment of land cover products. Remote Sens. Environ. 231, 111199 (2019).
Google Scholar
-
Laso Bayas, J. C. et al. A global reference database of crowdsourced cropland data collected using the Geo-Wiki platform. Sci. Data 4, 170136 (2017).
Google Scholar
-
Gourlay, S., Kilic, T. & Lobell, D. B. A new spin on an old debate: errors in farmer-reported production and their implications for inverse scale-productivity relationship in Uganda. J. Dev. Econ. 141, 102376 (2019).
Google Scholar
-
Phelps, L. N. & Kaplan, J. O. Land use for animal production in global change studies: defining and characterizing a framework. Glob. Chang. Biol. 23, 4457–4471 (2017).
Google Scholar
-
Lowder, S. K., Sánchez, M. V. & Bertini, R. Which farms feed the world and has farmland become more concentrated? World Dev. 142, 105455 (2021).
Google Scholar
-
van Andel, M., Tildesley, M. J. & Gates, M. C. Challenges and opportunities for using national animal datasets to support foot‐and‐mouth disease control. Transbound. Emerg. Dis. 68, 1800–1813 (2021).
Google Scholar
-
Abebe, R. et al. Narratives and counternarratives on data sharing in Africa. In Proc. 2021 ACM Conference on Fairness, Accountability, and Transparency 329–341 (2021).
-
Bradley, D. et al. Opportunities to improve fisheries management through innovative technology and advanced data systems. Fish Fish. 20, 564–583 (2019).
Google Scholar
-
van Helmond, A. T. M. et al. Electronic monitoring in fisheries: lessons from global experiences and future opportunities. Fish Fish. 21, 162–189 (2020).
Google Scholar
-
Seto, K. L. et al. Fishing through the cracks: the unregulated nature of global squid fisheries. Sci. Adv. 9, eadd8125 (2023).
Google Scholar
-
Taconet, M. et al. Global Atlas of AIS-Based Fishing Activity: Challenges and Opportunities (FAO, 2019).
-
Welch, H. et al. Hot spots of unseen fishing vessels. Sci. Adv. 8, eabq2109 (2023).
Google Scholar
-
Orofino, S., McDonald, G., Mayorga, J., Costello, C. & Bradley, D. Opportunities and challenges for improving fisheries management through greater transparency in vessel tracking. ICES J. Mar. Sci. 80, 675–689 (2023).
Google Scholar
-
Shepperson, J. L. et al. A comparison of VMS and AIS data: the effect of data coverage and vessel position recording frequency on estimates of fishing footprints. ICES J. Mar. Sci. 75, 988–998 (2018).
Google Scholar
-
Kroodsma, D. A. et al. Revealing the Global Longline Fleet with Satellite Radar (2022).
-
Park, J. et al. Illuminating dark fishing fleets in North Korea. Sci. Adv. 6, eabb1197 (2023).
Google Scholar
-
Ottinger, M., Clauss, K. & Kuenzer, C. Large-scale assessment of coastal aquaculture ponds with Sentinel-1 time series data. Remote Sens. 9, 440 (2017).
Google Scholar
-
Piñeiro, V. et al. A scoping review on incentives for adoption of sustainable agricultural practices and their outcomes. Nat. Sustain. 3, 809–820 (2020).
Google Scholar
-
Rotz, S. et al. The politics of digital agricultural technologies: a preliminary review. Sociol. Ruralis 59, 203–229 (2019).
Google Scholar
-
Xu, Y. et al. Mapping aquaculture areas with multi-source spectral and texture features: a case study in the Pearl River basin (Guangdong), China. Remote Sens. 13, 4320 (2021).
Google Scholar
-
Cochrane, K. (ed.) Illuminating Hidden Harvests: the Contributions of Small-Scale Fisheries to Sustainable Development (FAO, Duke Univ. & World Fish, 2023).
-
Halim, A. et al. Developing a functional definition of small-scale fisheries in support of marine capture fisheries management in Indonesia. Marine Policy 100, 238–248 (2018).
Google Scholar
-
Smith, H. & Basurto, X. Defining small-scale fisheries and examining the role of science in shaping perceptions of who and what counts: a systematic review. Front. Mar. Sci. 6, 236 (2019).
Google Scholar
-
Carletto, C., Jolliffe, D. & Banerjee, R. From tragedy to renaissance: improving agricultural data for better policies. J. Dev. Stud. 51, 133–148 (2015).
Google Scholar
-
Agarwal, S., Singh, V. & Gandhi, R. Could a data sharing protocol be agriculture’s missing link? The Chicago Council on Global Affairs https://globalaffairs.org/commentary-and-analysis/blogs/could-data-sharing-protocol-be-agricultures-missing-link (2021).
-
Fisher, A. & Fukuda-Parr, S. Introduction — data, knowledge, politics and localizing the SDGs. J. Hum. Dev. Capab. 20, 375–385 (2019).
Google Scholar
-
Montenegro de Wit, M. & Canfield, M. Feeding the world, byte by byte’: emergent imaginaries of data productivism. J. of Peasant Stud. https://doi.org/10.1080/03066150.2023.2232997 (2023).
-
Wolfert, S., Ge, L., Verdouw, C. & Bogaardt, M.-J. Big data in smart farming — a review. Agric. Syst. 153, 69–80 (2017).
Google Scholar
-
Spanaki, K., Karafili, E. & Despoudi, S. AI applications of data sharing in agriculture 4.0: a framework for role-based data access control. Int. J. Inf. Manag. 59, 102350 (2021).
Google Scholar
-
Brinkerhoff, D. W. & Brinkerhoff, J. M. Public–private partnerships: perspectives on purposes, publicness, and good governance. Public Adm. Dev. 31, 2–14 (2011).
Google Scholar
-
Wiggins, S., Kirsten, J. & Llambí, L. The future of small farms. World Dev. 38, 1341–1348 (2010).
Google Scholar
-
Jouanjean, M.-A., Casalini, F., Wiseman, L. & Gray, E. Issues Around Data Governance in the Digital Transformation of Agriculture: The Farmers’ Perspective (OECD, 2020).
-
Jensen, Ø., Dempster, T., Thorstad, E. B., Uglem, I. & Fredheim, A. Escapes of fishes from Norwegian sea-cage aquaculture: causes, consequences and prevention. Aquacult. Environ. Interact. 1, 71–83 (2010).
-
Pinsky, M. L. et al. Preparing ocean governance for species on the move. Science 360, 1189–1191 (2018).
Google Scholar
-
Herrero, M. et al. Innovation can accelerate the transition towards a sustainable food system. Nat. Food 1, 266–272 (2020).
Google Scholar
-
Barrett, C. B. et al. Bundling innovations to transform agri-food systems. Nat. Sustain. 3, 974–976 (2020).
Google Scholar
-
Paliyam, M., Nakalembe, C., Liu, K., Nyiawung, R. & Kerner, H. Street2sat: a machine learning pipeline for generating ground-truth geo-referenced labeled datasets from street-level images. In ICML 2021 Workshop on Tackling Climate Change with Machine Learning (ICML, 2021).
-
Yan, Y. & Ryu, Y. Exploring Google Street View with deep learning for crop type mapping. ISPRS J. Photogramm. Remote Sens. 171, 278–296 (2021).
Google Scholar
-
d’Andrimont, R., Yordanov, M., Martinez-Sanchez, L. & Van der Velde, M. Monitoring crop phenology with street-level imagery using computer vision. Comput. Electron. Agric. 196, 106866 (2022).
Google Scholar
-
van der Merwe, D., Burchfield, D. R., Witt, T. D., Price, K. P. & Sharda, A. Drones in agriculture. Adv. Agron. 162, 1–30 (2020).
Google Scholar
-
d’Andrimont, R. et al. Crowdsourced street-level imagery as a potential source of in-situ data for crop monitoring. Land 7, 127 (2018).
Google Scholar
-
Kerner, H. R. et al. Phenological normalization can improve in-season classification of maize and soybean: a case study in the central US Corn Belt. Sci. Remote Sens. 6, 100059 (2022).
Google Scholar
-
Wang, S. et al. Mapping crop types in southeast India with smartphone crowdsourcing and deep learning. Remote Sens. 12, 2957 (2020).
Google Scholar
-
Tseng, G., Kerner, H., Nakalembe, C. & Becker-Reshef, I. Learning to predict crop type from heterogeneous sparse labels using meta-learning. In Proc. IEEE/CVF Conference on Computer Vision and Pattern Recognition 1111–1120 (2021).
-
Muruganantham, P., Wibowo, S., Grandhi, S., Samrat, N. H. & Islam, N. A systematic literature review on crop yield prediction with deep learning and remote sensing. Remote Sens. 14, 1990 (2022).
Google Scholar
-
Deines, J. M., Wang, S. & Lobell, D. B. Satellites reveal a small positive yield effect from conservation tillage across the US Corn Belt. Environ. Res. Lett. 14, 124038 (2019).
Google Scholar
-
Ferrag, M. A., Shu, L., Yang, X., Derhab, A. & Maglaras, L. Security and privacy for green IoT-based agriculture: review, blockchain solutions, and challenges. IEEE Access 8, 32031–32053 (2020).
Google Scholar
-
Rahman, M. U., Baiardi, F. & Ricci, L. Blockchain smart contract for scalable data sharing in IoT: a case study of smart agriculture. In 2020 IEEE Global Conference on Artificial Intelligence and Internet of Things (GCAIoT) 1–7 (IEEE, 2020).
-
Gobezie, T. B. & Biswas, A. Break barriers in soil data stewardship by rewarding data generators. Nat. Rev. Earth Environ. 4, 353–354 (2023).
Google Scholar
-
Durrant, A. et al. The role of cross-silo federated learning in facilitating data sharing in the agri-food sector. Comput. Electron. Agric. 193, 106648 (2022).
Google Scholar
-
UNSC. Spatial anonymization: guidance note for the Inter-Secretariat Working Group on Household Surveys. https://unstats.un.org/unsd/statcom/52nd-session/documents/BG-3l-Spatial_Anonymization-E.pdf (2021).
-
Tedeschi, L. O. et al. Quantification of methane emitted by ruminants: a review of methods. J. Anim. Sci. 100, skac197 (2022).
Google Scholar
-
Ramayo-Caldas, Y. et al. Identification of rumen microbial biomarkers linked to methane emission in Holstein dairy cows. J. Anim. Breed. Genet. 137, 49–59 (2020).
Google Scholar
-
Han, C. S. et al. Invited review: Sensor technologies for real-time monitoring of the rumen environment. J. Dairy Sci. 105, 6379–6404 (2022).
-
Tullo, E., Finzi, A. & Guarino, M. Review: Environmental impact of livestock farming and precision livestock farming as a mitigation strategy. Sci. Total Environ. 650, 2751–2760 (2019).
Google Scholar
-
Chase, L. E. & Fortina, R. Environmental and economic responses to precision feed management in dairy cattle diets. Agriculture https://doi.org/10.3390/agriculture13051032 (2023).
-
Mackenzie, S. in Smart Livestock Nutrition 311–336 (Springer, 2023).
-
Sala, E. et al. The economics of fishing the high seas. Sci. Adv. 4, eaat2504 (2023).
Google Scholar
-
White, T. D. et al. Predicted hotspots of overlap between highly migratory fishes and industrial fishing fleets in the northeast Pacific. Sci. Adv. 5, eaau3761 (2023).
Google Scholar
-
Queiroz, N. et al. Global spatial risk assessment of sharks under the footprint of fisheries. Nature 572, 461–466 (2019).
Google Scholar
-
White, T. D. et al. Assessing the effectiveness of a large marine protected area for reef shark conservation. Biol. Conserv. 207, 64–71 (2017).
Google Scholar
-
McDermott, G. R., Meng, K. C., McDonald, G. G. & Costello, C. J. The blue paradox: preemptive overfishing in marine reserves. Proc. Natl Acad. Sci. 116, 5319–5325 (2019).
Google Scholar
-
Cabral, R. B. et al. Rapid and lasting gains from solving illegal fishing. Nat. Ecol. Evol. 2, 650–658 (2018).
Google Scholar
-
Behivoke, F. et al. Estimating fishing effort in small-scale fisheries using GPS tracking data and random forests. Ecol. Indic. 123, 107321 (2021).
Google Scholar
-
Tilley, A., Dos Reis Lopes, J. & Wilkinson, S. P. PeskAAS: a near-real-time, open-source monitoring and analytics system for small-scale fisheries. PLoS One 15, e0234760 (2020).
Google Scholar
-
Snapir, B., Waine, T. W. & Biermann, L. Maritime vessel classification to monitor fisheries with SAR: demonstration in the North Sea. Remote Sens. 11, 353 (2019).
Google Scholar
-
Sarda, K., CaJacob, D., Orr, N. & Zee, R. Making the invisible visible: precision RF-emitter geolocation from space by the Hawkeye 360 Pathfinder mission. In 32nd Annual AIAA/USU Conference on Small Satellites (AIAA, USU, 2018).
-
Iacarella, J. C. et al. Application of AIS- and flyover-based methods to monitor illegal and legal fishing in Canada’s Pacific marine conservation areas. Conserv. Sci. Pract. 5, e12926 (2023).
Google Scholar
-
Prayudi, A., Sulistijono, I. A., Risnumawan, A. & Darojah, Z. Surveillance system for illegal fishing prevention on UAV imagery using computer vision. In 2020 International Electronics Symposium (IES) 385–391 (2020).
-
Bartholomew, D. C. et al. Remote electronic monitoring as a potential alternative to on-board observers in small-scale fisheries. Biol. Conserv. 219, 35–45 (2018).
Google Scholar
-
Antonucci, F. & Costa, C. Precision aquaculture: a short review on engineering innovations. Aquacult. Int. 28, 41–57 (2020).
Google Scholar
-
Rastegari, H. et al. Internet of Things in aquaculture: a review of the challenges and potential solutions based on current and future trends. Smart Agric. Technol. 4, 100187 (2023).
Google Scholar
-
Cervantes-Godoy, D. et al. The Future of Food and Agriculture: Trends and Challenges Vol. 4 (FAO, 2014).
-
Turnheim, B. et al. Evaluating sustainability transitions pathways: bridging analytical approaches to address governance challenges. Glob. Environ. Chang. 35, 239–253 (2015).
Google Scholar
-
Dawes, S. S. Stewardship and usefulness: policy principles for information-based transparency. Gov. Inf. Q. 27, 377–383 (2010).
Google Scholar
-
Xie, W. et al. Crop switching can enhance environmental sustainability and farmer incomes in China. Nature https://doi.org/10.1038/s41586-023-05799-x (2023).
-
Kochupillai, M., Kahl, M., Schmitt, M., Taubenböck, H. & Zhu, X. X. Earth observation and artificial intelligence: understanding emerging ethical issues and opportunities. IEEE Geosci. Remote Sens. Mag. 10, 90–124 (2022).
Google Scholar
-
World Bank. World Development Report 2021: Data for Better Lives (World Bank, 2021).
-
Sachs, J. D. et al. Six transformations to achieve the sustainable development goals. Nat. Sustain. 2, 805–814 (2019).
-
Fanzo, J. et al. Viewpoint: Rigorous monitoring is necessary to guide food system transformation in the countdown to the 2030 global goals. Food Policy 104, 102163 (2021).
Google Scholar
-
Cassidy, E. S., West, P. C., Gerber, J. S. & Foley, J. A. Redefining agricultural yields: from tonnes to people nourished per hectare. Environ. Res. Lett. 8, 034015 (2013).
Google Scholar
-
Iizumi, T. & Sakai, T. The global dataset of historical yields for major crops 1981–2016. Sci. Data 7, 97 (2020).
Google Scholar
-
Franke, J. A. et al. The GGCMI phase 2 experiment: global gridded crop model simulations under uniform changes in CO2, temperature, water, and nitrogen levels. Geosci. Model Dev. 13, 2315–2336 (2020).
Google Scholar
-
Jägermeyr, J. et al. Climate impacts on global agriculture emerge earlier in new generation of climate and crop models. Nat. Food 2, 873–885 (2021).
Google Scholar
-
Müller, C. et al. The Global Gridded Crop Model Intercomparison phase 1 simulation dataset. Sci. Data 6, 50 (2019).
Google Scholar
-
The Global Yield Gap and Water Productivity Atlas (GYGA) (Yield Gap, 2022); http://www.yieldgap.org.
-
Mueller, N. D. et al. Closing yield gaps through nutrient and water management. Nature 490, 254–257 (2012).
Google Scholar
-
Jackson, N. D., Konar, M., Debaere, P. & Estes, L. Probabilistic global maps of crop-specific areas from 1961 to 2014. Environ. Res. Lett. 14, 094023 (2019).
Google Scholar
-
Ray, D. K. et al. Climate change has likely already affected global food production. PLoS One 14, e0217148 (2019).
Google Scholar
-
International Food Policy Research Institute. Global spatially-disaggregated crop production statistics data for 2000 version 3.0.7. https://doi.org/10.7910/DVN/A50I2T (2019).
-
International Food Policy Research Institute (IFPRI), International Institute for Applied Systems Analysis (IIASA). Global Spatially-disaggregated crop production statistics data for 2005 version 3.2. https://doi.org/10.7910/DVN/DHXBJX (2016).
-
International Food Policy Research Institute. Global spatially-disaggregated crop production statistics data for 2010 version 2.0. https://doi.org/10.7910/DVN/PRFF8V (2019).
-
West, P. C. et al. Leverage points for improving global food security and the environment. Science 345, 325–328 (2014).
Acknowledgements
K.F.D. and E.A.K. were supported by US Department of Agriculture Grant no. 2022-67019-37180 and the University of Alabama Global Water Security Center. E.A.K. also acknowledges support from the Consortium of Universities for the Advancement of Hydrologic Sciences (CUAHSI) Hydroinformatics Fellowship. H.A.A. acknowledges support from the University of Delaware — Delaware Environmental Institute Fellows programme. H.E.F. recognizes support from the University of California, Santa Barbara. J.A.G. was supported by the United States National Science Foundation (NSF) grant no. 2121238. S.H. was supported by NSF Digital Transformation of Development (grant no. 2125913) and NSF Graduate Research Fellowship Program (grant no. 1752814). P.M. acknowledges support from the University of Delaware Doctoral Fellowship for Excellence programme.
Author information
Authors and Affiliations
Contributions
K.F.D. led the Review. E.A.K. and K.F.D. conceived the Review and designed all analyses. E.A.K., S.H., P.M., D.K.R. and S.S. drafted the crop production section. M.H. and P.T. drafted the livestock and policy innovation sections. T.C., H.E.F. and J.A.G. drafted the fisheries and aquaculture section. H.K., C.N. and H.A.A. drafted the technology section. E.A.K., H.A.A., S.H. and K.F.D. compiled and edited the initial manuscript. E.A.K. collected the food production data. E.A.K. and S.H. produced graphical representations of the results. All authors contributed to writing and revising the paper.
Corresponding authors
Ethics declarations
Competing interests
The authors declare no competing interests.
Peer review
Peer review information
Nature Reviews Earth & Environment thanks Leslie Roberson and the other, anonymous, reviewer(s) for their contribution to the peer review of this work.
Additional information
Publisher’s note Springer Nature remains neutral with regard to jurisdictional claims in published maps and institutional affiliations.
Related links
AgMIP: https://agmip.org/
CGIAR: https://www.cgiar.org/
CropGRIDS: https://essd.copernicus.org/preprints/essd-2023-130/
EU ASAP: https://gkhub.earthobservations.org/packages/bf817-dc879
EUROSTAT: https://ec.europa.eu/eurostat/web/main/data/database
FAOSTAT: https://www.fao.org/faostat/en/#data/QCL
FishStat: https://www.fao.org/fishery/en/fishstat/collections
GAEZ: https://gaez.fao.org/
GEOCLAM: https://earthobservations.org/geoglam.php
GEOCLAM Crop Monitor: https://cropmonitor.org/
GGCMI: https://agmip.org/aggrid-ggcmi/
GLEAM: https://www.fao.org/gleam/en/
GLPS: https://data.apps.fao.org/map/catalog/static/search?keyword=Global%20livestock%20production%20systems
GLPS: https://dataverse.harvard.edu/dataset.xhtml?persistentId=doi:10.7910/DVN/WPDSZE
GLW: https://data.apps.fao.org/catalog/dataset/glw
GODAN: https://www.godan.info/
Gridded Livestock of the World: https://data.apps.fao.org/catalog/dataset/glw
GYGA: https://www.yieldgap.org/
ISIMIP: https://www.isimip.org/
Livestock Data for Decisions: https://www.livestockdata.org/type/datasets
LSMS: https://www.worldbank.org/en/programs/lsms
M3-Crops: http://www.earthstat.org/harvested-area-yield-175-crops/
MIRCA: https://gkhub.earthobservations.org/packages/bf817-dc879
PCAM: https://databank.illinois.edu/datasets/IDB-7439710
RHoMIS: https://www.rhomis.org/
RiceAtlas: https://dataverse.harvard.edu/dataset.xhtml?persistentId=doi:10.7910/DVN/JE6R2R
SAGE: https://sage.nelson.wisc.edu/data-and-models/datasets/crop-calendar-dataset/
SPAM: https://mapspam.info/
USDA-FAS: https://ipad.fas.usda.gov/ogamaps/cropmapsandcalendars.aspx
WCA: https://www.fao.org/world-census-agriculture/wcarounds/wca2020/en/
Supplementary information
Supplementary Information
Rights and permissions
Springer Nature or its licensor (e.g. a society or other partner) holds exclusive rights to this article under a publishing agreement with the author(s) or other rightsholder(s); author self-archiving of the accepted manuscript version of this article is solely governed by the terms of such publishing agreement and applicable law.
Reprints and permissions
About this article
Cite this article
Kebede, E.A., Abou Ali, H., Clavelle, T. et al. Assessing and addressing the global state of food production data scarcity.
Nat Rev Earth Environ (2024). https://doi.org/10.1038/s43017-024-00516-2
-
Accepted: 08 January 2024
-
Published: 20 February 2024
-
DOI: https://doi.org/10.1038/s43017-024-00516-2