The field of audiomics combines artificial intelligence tools with human sounds, such as a coughs, to evaluate health.Credit: Getty
A team led by Google scientists has developed a machine-learning tool that can help to detect and monitor health conditions by evaluating noises such as coughing and breathing. The artificial intelligence (AI) system1, trained on millions of audio clips of human sounds, might one day be used by physicians to diagnose diseases including COVID-19 and tuberculosis and to assess how well a person’s lungs are functioning.
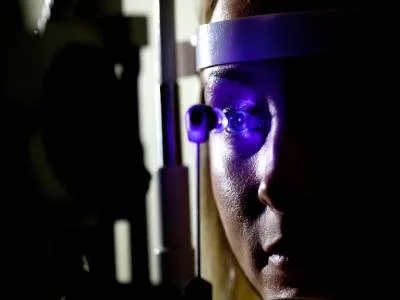
AI detects eye disease and risk of Parkinson’s from retinal images
This is not the first time a research group has explored using sound as a biomarker for disease. The concept gained traction during the COVID-19 pandemic, when scientists discovered that it was possible to detect the respiratory disease through a person’s cough2.
What’s new about the Google system — called Health Acoustic Representations (HeAR) — is the massive data set that it was trained on, and the fact that it can be fine-tuned to perform multiple tasks.
The researchers, who reported the tool earlier this month in a preprint1 that has not yet been peer reviewed, say it’s too early to tell whether HeAR will become a commercial product. For now, the plan is to give interested researchers access to the model so that they can use it in their own investigations. “Our goal as part of Google Research is to spur innovation in this nascent field,” says Sujay Kakarmath, a product manager at Google in New York City who worked on the project.
How to train your model
Most AI tools being developed in this space are trained on audio recordings — for example, of coughs — that are paired with health information about the person who made the sounds. For example, the clips might be labelled to indicate that the person had bronchitis at the time of the recording. The tool comes to associate features of the sounds with the data label, in a training process called supervised learning.
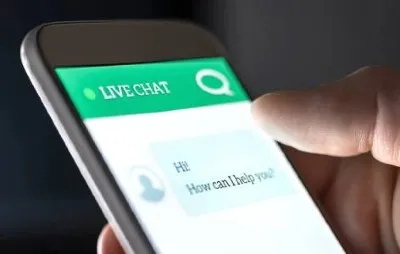
Google AI has better bedside manner than human doctors — and makes better diagnoses
“In medicine, traditionally, we have been using a lot of supervised learning, which is great because you have a clinical validation,” says Yael Bensoussan, a laryngologist at the University of South Florida in Tampa. “The downside is that it really limits the data sets that you can use, because there is a lack of annotated data sets out there.”
Instead, the Google researchers used self-supervised learning, which relies on unlabelled data. Through an automated process, they extracted more than 300 million short sound clips of coughing, breathing, throat clearing and other human sounds from publicly available YouTube videos.
Each clip was converted into a visual representation of sound called a spectrogram. Then the researchers blocked segments of the spectrograms to help the model learn to predict the missing portions. This is similar to how the large language model that underlies chatbot ChatGPT was taught to predict the next word in a sentence after being trained on myriad examples of human text. Using this method, the researchers created what they call a foundation model, which they say can be adapted for many tasks.
An efficient learner
In the case of HeAR, the Google team adapted it to detect COVID-19, tuberculosis and characteristics such as whether a person smokes. Because the model was trained on such a broad range of human sounds, to fine-tune it, the researchers only had to feed it very limited data sets labelled with these diseases and characteristics.
On a scale where 0.5 represents a model that performs no better than a random prediction and 1 represents a model that makes an accurate prediction each time, HeAR scored 0.645 and 0.710 for COVID-19 detection, depending on which data set it was tested on — a better performance than existing models trained on speech data or general audio. For tuberculosis, the score was 0.739.
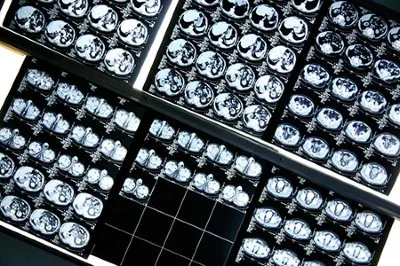
An AI revolution is brewing in medicine. What will it look like?
The fact that the original training data were so diverse — with varying sound quality and human sources — also means that the results are generalizable, Kakarmath says.
Ali Imran, an engineer at the University of Oklahoma in Tulsa, says that the sheer volume of data used by Google lends significance to the research. “It gives us the confidence that this is a reliable tool,” he says.
Imran leads the development of an app named AI4COVID-19, which has shown promise at distinguishing COVID-19 coughs from other types of cough3. His team plans to apply for approval from the US Food and Drug Administration (FDA) so that the app can eventually move to market; he is currently seeking funding to conduct the necessary clinical trials. So far, no FDA-approved tool provides diagnosis through sounds.
The field of health acoustics, or ‘audiomics’, is promising, Bensoussan says. “Acoustic science has existed for decades. What’s different is that now, with AI and machine learning, we have the means to collect and analyse a lot of data at the same time.” She co-leads a research consortium focused on exploring voice as a biomarker to track health.
“There’s an immense potential not only for diagnosis, but also for screening” and monitoring, she says. “We can’t repeat scans or biopsies every week. So that’s why voice becomes a really important biomarker for disease monitoring,” she adds. “It’s not invasive, and it’s low resource.”