Abstract
Digital twins, which are considered an effective approach to realize the fusion between virtual and physical spaces, have attracted a substantial amount of attention in the past decade. With their rapid development in recent years, digital twins have been applied in various fields, particularly in industry. However, there are still some gaps to be filled and some limitations to be addressed. Here we provide a brief overview of digital twin advancements in industry and highlight the main pitfalls to avoid and challenges to overcome, to improve the maturity of digital twins and facilitate large-scale industrial applications in the future.
Main
With the continuous development of information technology in recent years (such as network communication technology, sensor technology and intelligent-embedded technology), the virtual space has had a much closer relationship with the physical space than ever before. In this context, digital twins, which are often regarded as living in silico representations of their corresponding physical targets, have grown in popularity. Digital twins can iterate and evolve through the seamless connection and fusion between the virtual and physical spaces1, and this consistency and synchronization can bring many benefits to numerous services, including real-time monitoring, dynamic optimization and accurate prediction2,3,4. The value of digital twins has been recognized by researchers, enterprises and other stakeholders in various fields, including healthcare5,6, agriculture7, urban science8, aerospace engineering9, marine engineering10,11 and even Earth systems12.
Notably, research and application of digital twins in industry are increasingly widespread, as digital twins align with the contemporary demands of smart manufacturing and Industry 4.013. At present, more than 1,000 institutions, including universities, enterprises and research institutes, have carried out related explorations and practice14, which has already covered the entire life cycle of products and manufacturing systems, such as realizing rapid engineering design15, real-time monitoring in the operation process16, intelligent decision-making towards continuous changing manufacturing systems17, prognostics and health management18, and so forth. Different application objects (such as humans, machines and materials), processing technologies and user requirements directly influence the implementation of digital twins, and as a consequence, digital twins should take into account these individual differences of use cases and not always be the same for every context. In addition, there are still some misunderstandings and bottlenecks that hinder further development of digital twins in the industrial field.
In this Perspective, we give an overview of digital twin advancements in industry, followed by a discussion on the pitfalls, challenges and promising research avenues based on the current research status and our own findings from practical applications.
State of the art in digital twins for industrial applications
So far, digital twins have addressed many problems in some industrial application scenarios. Here we give an overview of digital twins in industry from the perspective of theory, technology and applications to better showcase the most recent advancements, understand how digital twins are applied and unravel their value.
Theory and technology
A number of conceptual models of digital twins have been recently proposed to illustrate their components and connotations, as shown in Fig. 1. Figure 1a depicts the three-dimensional digital twin model19, which is one of the most popular conceptual models. Figure 1b is as a more detailed model that takes into account the product, production and performance. Finally, Fig. 1c describes the overall architecture of the digital twin for both products and manufacturing systems, which adds data and services to the three-dimensional digital twin model20. In addition, physical processes and virtual processes are executed alternately during the entire life cycle, as shown in the outer ring of Fig. 1c. The twin model is utilized to support virtual simulations and optimizations, thereby achieving product design, production, testing and optimization at a lower cost and higher efficiency. In addition, the twin model is continuously enriched, modified and updated based on physical information and twin interactions. While these conceptual models differ in terms of framework, they all aim to utilize ultra-fidelity models, rich data and real-time interaction to provide some services for specific objects.
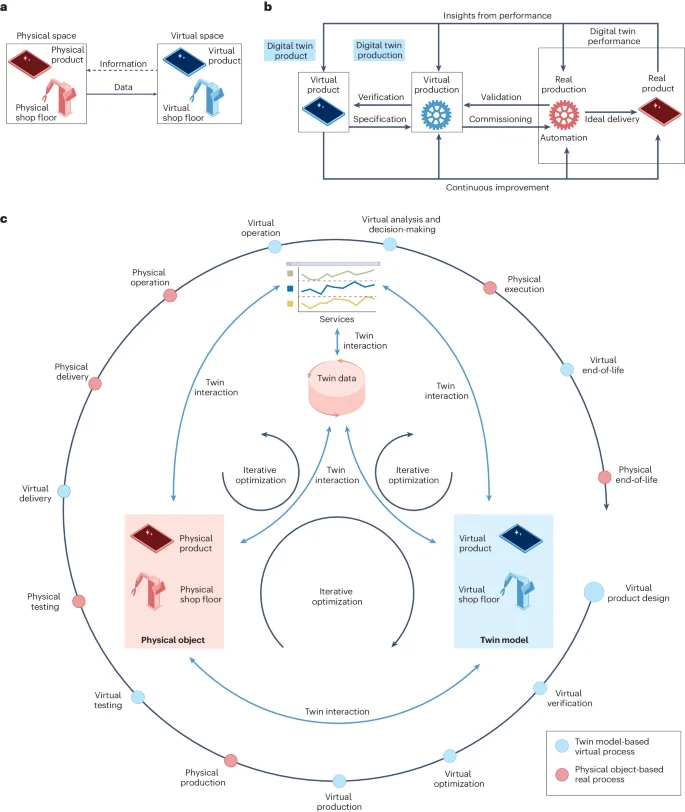
a, The three-dimensional digital twin model is one of the earliest digital twin conceptual models, which includes physical object, virtual model, and the interaction between physical and virtual spaces. b, The Siemens digital twin model demonstrates how digital twins work to improve product, production and performance. c, The five-dimensional digital twin model consists of five dimensions, including physical object, twin model, services, twin data and twin interaction among them.
In addition to the conceptual models, reference frameworks have also been recently proposed to provide more detailed guidelines or templates for implementing digital twins toward certain needs. For example, to realize autonomy and real-time supervision, a manufacturing execution system (MES)-integrated digital twin framework was proposed. The framework contains a decision-making level, an MES integration level, and an MES level to deal with error states management during production and reactive disassembly during assembly of products21. The field-synchronized digital twin framework for production scheduling—which includes a physical flowshop system, an input data module, an optimization module, a digital twin module and an operator interface—was proposed to ensure the robustness of the optimal scheduling solutions by considering the uncertainties of a real flowshop22. In addition, a digital twin framework for the simulation and optimization of production systems was proposed to better configure and implement digital twins in the design and operation stages. In this framework, the production twin and machine/process twin were provided with the details about their components and functions3.
Even though conceptual models and reference frameworks are important for providing guidelines for the compositions and the implementation process of digital twins, they alone are not enough, as their specific implementation depends on various supporting technologies.
Twin perception
One of the most important steps of establishing the relationships and realizing the synchronization between the virtual and physical spaces is sensing changes of the environment, machines, people and other elements in the manufacturing systems. This process mainly involves data access, data description, and data transformation from sensors, intelligent terminals and other devices, in which the Industrial Internet of Things (IoT) becomes a valuable choice23,24,25. For example, electric current sensors, accelerometer sensors and acoustic emission sensors could be used to collect the status data of computer numerical control (CNC) machines26. In addition, the data from the CNC system and operation processes—including feed rate, depth of cut, cutter location of the cutting tools, production data, alarm information and so forth—are also needed27,28. The collected data are often integrated into databases or the cloud for further use through data exchange protocols, including transmission control protocol/internet protocol29, open platform communications unified architecture30, MTConnect31 and automation markup language32.
Twin model construction
Twin models are used to depict the key features (such as geometry features, physics features and behavior features) of the manufacturing system and its elements33,34,35. Different modeling methods—including physics-based modeling, data-based modeling and hybrid modeling—have been applied in different scenarios36. The accuracy of physics-based models relies on the understanding of the physical mechanisms and objective laws to a great extent, but this method does not need many data. On the contrary, accurate data-based modeling needs many parameters and many data with high quality. In reality, some mechanisms are not well enough understood and the available data are limited. A hybrid modeling method could take advantage of these two methods, and as such, is regarded as the trend to realize the accurate description of the targets through the fusion of data, knowledge and mechanisms37,38,39.
To meet practical requirements, such as real-time monitoring and dynamic scheduling, multiple models, such as geometric models, mechanical models, material models and discrete-event models, have been constructed40,41,42,43. For example, Söderberg et al. proposed a digital twin model consisting of the geometric representation of the assembly, kinematic relationships, finite-element-analysis functions, Monte Carlo simulation and material properties, thereby supporting the geometry assurance in the design and manufacturing stages44.
Twin data computation
Twin data contain both real-time and historical data on various elements within manufacturing systems, from both physical and virtual spaces. At present, more research is devoted to presenting advanced algorithms or selecting appropriate algorithms oriented to specific requirements, such as the Q-learning algorithm for dynamic scheduling45,46, the particle swarm optimization algorithm for energy optimization47 and the Bayesian network for remaining tool life prediction48. However, research on data-quality improvement, to maximize the value of twin data, is relatively scarce, which cannot be neglected and should be given more attention.
Twin interaction
Twin interaction contains the interactions between the twin model and the physical object, between the twin model and twin data, between the physical object and twin data, between the physical object and services, between the twin model and services, and between twin data and services, thereby forming an organic whole. Serendipitous factors, such as unexpected stochastic disturbance, frequent insertion order and equipment failures, usually make the virtual space inconsistent with practical situations in the industrial scene. This is where twin interactions come into play: the consistency and synchronization between the virtual and physical spaces, which is one of the core features of digital twins, can be realized by virtual–real interaction49. Therefore, some interaction strategies or methods have been studied in the past, for example, the specification-based state replication method50 and the Kalman-filter-as-observer method51. These methods can be mainly divided into two steps. First, the differences between virtual and physical spaces should be uncovered before the virtual space can be updated accordingly. The rule-based consistency-checking method could be used to achieve this goal by comparing the sensed timed event from the physical manufacturing plant with the digital twins-based estimations52. After that, the problem of how to make a virtual space consistent with its corresponding physical space within an acceptable error and time delay can be studied. For example, the anchor point method has been applied to realize synchronization of the manufacturing automation system53.
Applications
On the basis of the developed models and technologies, some explorations and practices have been carried out towards industrial needs. Current applications have shown digital twins’ priorities in the entire product and shop-floor life cycle, as shown in Fig. 2.
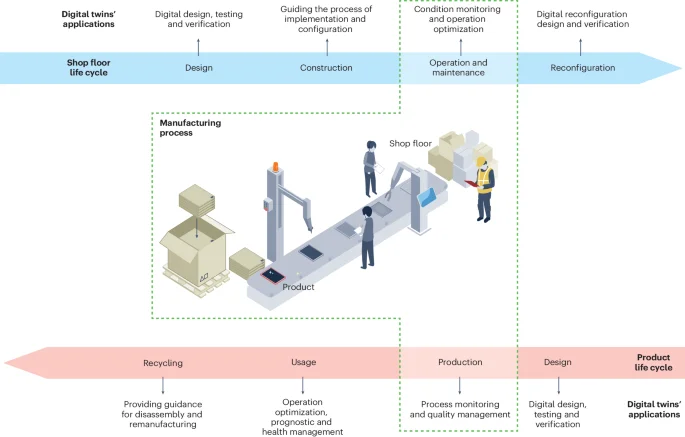
Digital twins can be applied in the entire life cycle of both the product and the shop floor, and form a coupling in the manufacturing process. The main role of digital twins is to assist in the design and implementation of products and shop floors and to guarantee the performance of shop floors and products through integrated simulation, real-time condition monitoring, prediction, optimization and so forth. In the figure, red is used to represent product-related content; blue is used to represent shop floor-related content; and the green dotted box represents the manufacturing process, in which both the products and the corresponding shop floors should be included.
In the design phase, compared with traditional design methods, for example, brainstorming, it is much easier to generate new solutions by using high-fidelity twin models and life-cycle data. The models can support virtual testing with a high level of confidence, which thus can greatly reduce the costs and time of physical testing54. In addition, twin models and product life-cycle data could bridge the gap between design and manufacturing to avoid the condition where the design schemes cannot be realized with limited manufacturing capacity. For example, Huang et al. proposed a framework for rapid product development based on digital twins. The digital product prototypes were obtained based on digital trial manufacturing, which contributes to improving the manufacturing process during the design stage, and rotorcraft components were used to validate the performance of the proposed framework54.
In the production or construction phase, the interaction strategy makes it possible to realize the synchronization between the virtual models and practical status, which can support real-time monitoring55. Furthermore, in the virtual space, multiple schemes (for example, logistics schemes and control schemes) could be simulated to support decision-making and to improve efficiency56,57. Instructions could then be given to the elements in the manufacturing systems, creating a closed loop between the virtual and physical spaces. On the basis of this idea, for the uncertain events, information asymmetry and abnormal interference in the manufacturing process of the shop floor, Fang et al. used digital twins to obtain equipment states, production tasks, interference and other relevant information from the physical shop floor. Shop-floor dynamic scheduling was realized through the analysis of processing time, worker skill level, cost, energy consumption and other data. Thus, the scheduling deviation could be effectively reduced and the efficiency and quality of scheduling execution could be improved58.
In the operation and maintenance or usage and recycling phases, rich sensing data reflect the current state of machines, production lines, factories or other objects. By combining historical data, field data and twin models, a more accurate prediction can be made59. When a machine presents a failure, the fault link, the fault location and the fault cause could be determined rapidly by using digital twins. At the same time, maintenance planning and remote maintenance could also be realized, preventing serious consequences and reducing overall costs60,61,62.
The effects of digital twins at each of these stages are clear, and many companies have been working on this space, including GE, Siemens, IBM and Dassault Systems63. However, there are still two major drawbacks that limit the further development of digital twins. One is that some existing pitfalls (see below) have led to the improper use of digital twins, so the full value of digital twins has not been fully realized yet64. In addition, there are some related technological bottlenecks to overcome. Finally, current applications from academic papers mostly focus on laboratories or pilot production lines rather than practical factories. More efforts are needed to move digital twins from theory to practice. Some companies have provided digital twin solutions, but there is still a long way to go for large-scale applications.
Main pitfalls
Overly simplistic or overly complex models
Modeling is mainly used to describe the features of physical objects, which raises a question about how to determine the right level of detail. It goes without saying that the more accurate the models, the better the simulation results. However, overly complex models require a lot of resources (computing costs and time), which can not only be unnecessary but also not satisfy the timeliness requirement in some situations. For example, to minimize the maximum delay time through dynamic job shop scheduling, the material flow, process flow and information flow are the focuses of twin models. Besides, the health status, capacity, location of equipment and process execution are additional factors that should be considered. However, the models of machines’ screws or product structures are not necessary to obtain accurate results and could even be neglected.
Under this condition, some people may ask whether simpler models are recommended instead. The answer is, however, ‘no’. Ideally, we would like to use simple models effectively to solve complex problems, but in reality, it is hard to do so. For example, the three-dimensional models of a CNC machine tool can reflect only its actions, which allows the visualization of the running process. Because the physical features (for example, fatigue wear and corrosion fatigue) are not embodied in three-dimensional models, the prognostics and health management of the machines cannot be assessed. The problems exposed by overly simplistic and overly complex models or elements include ambiguous requirements and unbalanced input–output. Therefore, it is important to accurately clarify user needs and analyze specific problems, thus guiding the trade-off of model construction or reconstruction between complex models and simple models.
Not just big data
Data are essential to bridge the virtual and physical spaces, which can drive models to be in silico representations of their physical counterparts and machines to operate. However, current research pays more attention to big data and neglects the value of small data to some extent. The difference between big data and small data lies in the scale, complexity and processing methods of the data. Big data often require the use of distributed computing and artificial intelligence techniques for processing and analysis, whereas small data can be processed and analyzed using traditional statistical analysis and data-mining methods. In some conditions, small data could beat big data. A small dataset with high quality is more meaningful for specific industrial services than large observational data of unknown provenance. In addition, big data have a relatively poor performance in assessments of uncertainty65. Some dangers of model misspecification and uncertainty for small data could be obtained, which is helpful for calibrating the uncertainty66. Neglecting small data may also cause bias in big data. Big data usually have a better performance in discovering rules but have a relatively poor performance in imperceptible changes. Hence, it is better to integrate the strengths and limitations from both small data and big data approaches into a hybrid approach to support better industrial services. Moreover, in the process of acquiring big data and small data, the strategy of data collection, data transmission, data storage and data analysis should also be seriously evaluated to avoid unprofitable or unnecessary data collection.
Inadequate interaction
Interaction is one of the main features of digital twins compared with traditional offline simulation, and focus should be given to the timeliness and comprehensiveness of the interaction processes. Timeliness reflects the execution time of the interaction. The higher the real-time requirement, the faster the data transmission needs to be. Although some new data transmission technologies (for example, 6G67,68) have been developed, the transmission speed is still limited. Therefore, we should not blindly pursue high interaction timeliness, but choose different interaction strategies according to each situation. For example, some static production elements (such as machine tool bed) do not need to interact with other elements. However, safety-related elements require a high interactive timeliness. Another important consideration is comprehensiveness, which refers to how much elements and links are considered in the process of interaction. Current applications often focus on the interaction between physical and virtual spaces, or between human and machine. However, we believe that other interactions should also be considered. There are many subelements in virtual and physical spaces, and these subelements also need to interact with each other, including interactions among virtual elements (such as models, data and service) and among physical elements (such as the person, machine, material, method and environment), which need more attention from users and researchers. Through comprehensive interactions, the accuracy of models, the value of data and the quality of service can all be improved.
Underused artificial intelligence
There is a substantial amount of data coming from machines, humans, materials and the environment in the physical space, which brings the challenge of how to deal with these data during the implementation of digital twins. Notably, artificial intelligence is suitable for mining knowledge from huge amounts of data. Artificial intelligence has been flourishing in the past few years, and while it is still under constant development, it might be mature enough to be applied to some practical scenarios. For instance, digital twins can provide dynamic scheduling, equipment failure prediction, energy consumption optimization and some other services with high quality by the use of artificial intelligence. In process manufacturing, Priyanka et al. combined digital twins with machine learning to predict the risk probability rate of an oil pipeline system and to assess its remaining useful life69. In discrete manufacturing, to reduce the impact caused by uncertainties and unpredictable events, Chen et al. proposed a multi-objective scheduling strategy of a fuzzy flexible job shop under the uncertainty of completion time, delivery date and equipment maintenance cycle, and the hybrid particle swarm optimization algorithm with a variable neighborhood search strategy was used to find the optimal scheduling scheme. The effectiveness and feasibility of the proposed method were illustrated in an automobile supporting factory70.
Apart from data mining, artificial intelligence could also be applied in other aspects during the digital twin implementation. Considering the limitations of computing power on the shop floor, artificial intelligence could help solve the problem of optimally allocating computing resources. Moreover, artificial intelligence could be embodied into edge hardware71, greatly reducing the amount of data transfer and better meeting the timeliness requirement of digital twins. Some recent products based on neural network and large language models, such as ChatGPT, may provide additional possibilities to digital twins.
Main challenges
Accurate model construction and comprehensive validation
It is easy to build an accurate model for simple objects. However, in many situations, the structure of the processing equipment, the technological process and the operating environment all may be very complicated, which brings challenges to modeling. The mechanism for some processes and coupling relationships may not be clear or accurate enough either, making it difficult to build their corresponding models. For example, the degree of tool wear is affected by many factors, such as tool material and shape, working environment and cooling method, cutting parameters (such as cutting speed, feed rate and the material to be machined) and so forth. In addition, the different forms of tool wear, including abrasive wear, adhesive wear, diffusion wear, fatigue wear and chemical wear, also relates to the different factors mentioned above72. It is then hard to depict the process of tool wear accurately. In addition, many challenges lie in the balance between computation cost and model accuracy: taking all of the aforementioned factors into account would require a substantial number of parameters, bringing huge computing costs. It is worth noticing that the models here are not infinitely accurate but valid on the premise of satisfying customer demand. To tackle this challenge, the fusion between physics-based models and data is promising, because the knowledge mined by data can compensate the deficiencies of the unknown mechanism to some extent.
Model validation is the main way to evaluate the model performance and the degree of confidence, and an indispensable step for digital twin applications. One-sided or wrong evaluation results will probably mislead the usage of the models and even cause serious consequences. A comprehensive model validation is needed to assist in judging where the models are suitable for. However, there is currently a lack of related international standards or benchmarks in industry to guide the implementation of model validation.
Data tasks under complex working conditions
Data collection, transmission, storage and processing are the main steps to create the value of twin data, and there are challenges in each of these steps.
Data acquisition
Even though there are some common data-acquisition methods—including configuration software, data-acquisition card, sensors and radio-frequency identification devices—there are still some challenges to be addressed. Some interfaces of machines are not open, and some machines do not even have interface to support data collection. Besides, some machines cannot be installed with sensors, as the installed sensors would affect their performance. In some complex working conditions—for example, high temperature, high pressure, low temperature, dust and high-radiation flux—there are higher requirements for sensors, including security, microminiaturization, high precision, low power consumption and so forth.
Data transmission
As more and more data are collected, higher requirements are put forward for data transmission speed. In addition, data transmission faces the risk of unauthorized access, data leakage and data tampering. Common encryption technologies, including link encryption, node encryption and end-to-end encryption, can ensure secure data transmission to some extent, but their performance in data protection is still limited73.
Data storage
The large volume of data makes the data storage burden increasingly unbearable. The software-defined-storage approach could alleviate the above problems, because it utilizes software to manage and control storage resources, contrasting with traditional hardware-dependent storage solutions. Software-defined storage abstracts storage functionality from hardware, enabling the management and configuration of storage resources through software-defined methods. Additional storage nodes or devices can also be easily incorporated to meet growing storage demands74. However, to realize giant technological leaps, the next generation of transformative optical storage technology looks promising75.
Data processing
Both unstructured and structured data are collected in industrial applications. Hence, how to integrate these data formats, ensure data quality and maximize data value becomes a primary task. Recently, advanced algorithms have been more widely used in the field of manufacturing to solve the problem of classification, prediction and optimization. However, better algorithms are still needed to make full use of collected data to meet the needs of different industrial applications. In addition, the explainability, robustness and fairness of algorithms should be further improved. To run these algorithms, the time consumption has a close relationship with the computing power, which would directly affect the timeliness of digital twins. Even though the technology of multi-core chips could optimize the performance of traditional chips, the improvements are in a limited range.
Explainable artificial intelligence for industrial applications
Many factories, shop floors, production lines and products have a high requirement for safety and reliability, as they may contain dangerous articles (for example, crude oil) that are inflammable or explosive. While artificial intelligence has been used to improve the effects and values of some digital twins’ industrial applications76, it is usually a ‘black box,’ which means that how the models work and the results are obtained may not be entirely clear77. In addition, it is difficult to assign responsibility once accidents occur. Explainable artificial intelligence provides a path to address this problem78,79. However, reaching a consensus on the judgement criteria on ‘explainability’, creating an acceptable benchmark considering subjective and objective factors, and making the trade-off between explainability and performance should be further developed in the future80.
Security of digital assets
Twin models and twin data have become important digital assets as business values are often embodied in these models and data. For example, the twin models of CNC machines contain the information of structure, material, hydraulic system, cooling system, lubrication system and so forth. Therefore, the security of digital assets is essential. If the security issues are not well addressed, it is difficult to realize the industrial chain coordination (meaning, cooperation across different enterprises). Recently, some promising security technologies have been developed. Blockchain is one of the potential security solutions for disseminating digital assets across the stakeholders through the characteristics of decentralization, encryption technology and distributed ledger81,82,83. However, more efforts are still needed for developing blockchain and addressing other security issues (for instance, digital assets attacked by an outside party).
Common industrial software and platform
Mature industrial software and platforms could greatly enhance digital twin applications. At present, some companies have developed tools to support digital twin implementation, such as ThingWorx, 3DEXPERIENCE, TwinBuilder, AWS IoT TwinMaker, Azure Digital Twins and Simcenter84. However, the software usually cannot meet the needs of researchers, developers and enterprises84. Often, the functions are rather limited, as companies develop their software based on their main foundation and strengths. In addition, file formats between different software are generally incompatible. To realize digital twins in industry more widely85, a common industrial software or platform is needed, which would break the bespoke mode. In this process, how to balance the benefits of different software companies is also a huge challenge. Therefore, this vision requires the efforts of the entire digital twin community.
Standard of digital twins in industry
An international standard is the foundation for large-scale industrial application of digital twins. Although standardization work has started (for example, ISO 23247, IPC 2551 and ISO/IEC JTC 1/SC 41), different organizations have not reached an agreement on the understanding of digital twins yet, which may result in compatibility and interoperability problems. Therefore, cooperation among standards organizations is needed in the future to maintain consistency and avoid redundancies. Moreover, some existing standards about the technologies used in digital twins could be still adopted. For example, ISO 29002, IEC 61987 and ISO 13372:2012 could be used in digital twins’ industrial applications to maintain compatibility. Standards on software, development processes and implementation processes toward different industrial scenarios are still absent.
Potential ethical and privacy concerns
The introduction of digital twins inevitably raises privacy and ethical concerns for users, as digital twins would contain multiple data and models of the whole manufacturing systems, including private data from individuals. Individuals may be concerned about models and data being abused and misused, thus requesting ownership and control of data and models. In addition, some new cybercriminal activities may also emerge86. Finally, some biases may occur because of incomplete data or selected algorithms, which would further lead to one-sided conclusions and unreasonable decisions. To address the aforementioned issues, related technologies, policies, laws and regulations need to be developed. For example, appropriate information transparency plays an important role in increasing trust of digital twins. Only when the potential ethical and privacy concerns have been well addressed can digital twin applications be widely accepted and trusted.
Conclusion and outlook
Despite the considerable advancements in industrial applications in recent years, the overall maturity of digital twins remains relatively low due to insufficient recognition, inaccurate models, incomplete data, inadequate interaction, immature commercial software and a fragmentary standard system. In addition, the development of digital twins in industry faces persistent technical challenges that need to be overcome to drive further progress.
Digital twins are not isolated from other advanced technologies (such as artificial intelligence), but instead, have a close relationship with them. In the future, digital twins will potentially combine the theory of reliability, complexity and systems engineering to further improve their application effect and better solve systemic problems of complex objects. In addition, to make digital twins sustainable, mature business models, such as a technology provider model and an application service provider model, should be developed. Policy support is also one of the important driving forces for researchers and enterprises at the same time. In a word, to realize digital twins, collaborative efforts from all stakeholders involved are essential.
References
-
Tao, F. & Qi, Q. Make more digital twins. Nature 573, 490–491 (2019).
Google Scholar
-
Lei, Z. et al. Digital twin based monitoring and control for DC–DC converters. Nat. Commun. 14, 5604 (2023).
Google Scholar
-
Ricondo, I., Porto, A. & Ugarte, M. A digital twin framework for the simulation and optimization of production systems. Procedia CIRP 104, 762–767 (2021).
Google Scholar
-
Hernandez-Boussard, T. et al. Digital twins for predictive oncology will be a paradigm shift for precision cancer care. Nat. Med. 27, 2065–2066 (2021).
Google Scholar
-
Coorey, G., Figtree, G. A., Fletcher, D. F. & Redfern, J. The health digital twin: advancing precision cardiovascular medicine. Nat. Rev. Cardiol. 18, 803–804 (2021).
Google Scholar
-
Coorey, G. et al. The health digital twin to tackle cardiovascular disease—a review of an emerging interdisciplinary field. npj Digit. Med. 5, 126 (2022).
Google Scholar
-
Shrivastava, C., Berry, T., Cronje, P., Schudel, S. & Defraeye, T. Digital twins enable the quantification of the trade-offs in maintaining citrus quality and marketability in the refrigerated supply chain. Nat. Food 3, 413–427 (2022).
Google Scholar
-
Schrotter, G. & Hürzeler, C. The digital twin of the city of Zurich for urban planning. J. Photogramm. Remote Sens. Geoinf. Sci. 88, 99–112 (2020).
-
Aydemir, H., Zengin, U. & Durak, U. The digital twin paradigm for aircraft review and outlook. In AIAA SciTech Forum 0553 (AIAA, 2020).
-
Coraddu, A. et al. Data-driven ship digital twin for estimating the speed loss caused by the marine fouling. Ocean Eng. 186, 106063 (2019).
Google Scholar
-
Mauro, F. & Kana, A. A. Digital twin for ship life-cycle: a critical systematic review. Ocean Eng. 269, 113479 (2023).
Google Scholar
-
Bauer, P., Stevens, B. & Hazeleger, W. A digital twin of Earth for the green transition. Nat. Clim. Change 11, 80–83 (2021).
Google Scholar
-
Uhlemann, T. H.-J., Lehmann, C. & Steinhilper, R. The digital twin: realizing the cyber-physical production system for Industry 4.0. Procedia CIRP 61, 335–340 (2017).
Google Scholar
-
Wang, K. et al. A review of the technology standards for enabling digital twin. Digit. Twin 2, 4 (2022).
Google Scholar
-
Lo, C. K., Chen, C. H. & Zhong, R. Y. A review of digital twin in product design and development. Adv. Eng. Inform. 48, 101297 (2021).
Google Scholar
-
Pei, F. Q., Tong, Y. F., Yuan, M. H., Ding, K. & Chen, X. H. The digital twin of the quality monitoring and control in the series solar cell production line. J. Manuf. Syst. 59, 127–137 (2021).
Google Scholar
-
Magnanini, M. C. & Tolio, T. A. M. A model-based digital twin to support responsive manufacturing systems. CIRP Ann. 70, 353–356 (2021).
Google Scholar
-
Toothman, M. et al. A digital twin framework for prognostics and health management. Comput. Ind. 150, 103948 (2023).
Google Scholar
-
Michael, G. & Vickers, J. in Transdisciplinary Perspectives on Complex Systems: New Findings and Approaches (eds Kahlen, F. J. et al.) 85–113 (Springer, 2017).
-
Tao, F. et al. Digital twin-driven product design, manufacturing and service with big data. Int. J. Adv. Manuf. Technol. 94, 3563–3576 (2018).
Google Scholar
-
Negri, E., Berardi, S., Fumagalli, L. & Macchi, M. MES-integrated digital twin frameworks. J. Manuf. Syst. 56, 58–71 (2020).
Google Scholar
-
Negri, E. et al. Field-synchronized digital twin framework for production scheduling with uncertainty. J. Intell. Manuf. 32, 1207–1228 (2021).
Google Scholar
-
Souza, V., Cruz, R., Silva, W., Lins, S. & Lucena, V. A digital twin architecture based on the Industrial Internet of Things technologies. In 2019 IEEE International Conference on Consumer Electronics (ICCE) 1–2 (IEEE, 2019).
-
Gopal, L. et al. Digital twin and IOT technology for secure manufacturing systems. Meas. Sens. 25, 100661 (2023).
Google Scholar
-
Redelinghuys, A. J. H., Basson, A. H. & Kruger, K. A six-layer architecture for the digital twin: a manufacturing case study implementation. J. Intell. Manuf. 31, 1383–1402 (2020).
Google Scholar
-
Ghosh, A. K., Ullah, A. S., Teti, R. & Kubo, A. Developing sensor signal-based digital twins for intelligent machine tools. J. Ind. Inf. Integr. 24, 100242 (2021).
-
Cai, Y., Starly, B., Cohen, P. & Lee, Y.-S. Sensor data and information fusion to construct digital-twins virtual machine tools for cyber-physical manufacturing. Procedia Manuf. 10, 1031–1042 (2017).
Google Scholar
-
Shahriar, M. R. et al. MTComm based virtualization and integration of physical machine operations with digital-twins in cyber-physical manufacturing cloud. In 2018 5th IEEE International Conference on Cyber Security and Cloud Computing (CSCloud)/2018 4th IEEE International Conference on Edge Computing and Scalable Cloud (EdgeCom) 46–51 (IEEE, 2018).
-
Wang, K. J., Lee, Y. H. & Angelica, S. Digital twin design for real-time monitoring—a case study of die cutting machine. Int. J. Prod. Res. 59, 6471–6485 (2021).
Google Scholar
-
Luo, W., Hu, T., Zhang, C. & Wei, Y. Digital twin for CNC machine tool: modeling and using strategy. J. Ambient Intell. Hum. Comput. 10, 1129–1140 (2019).
Google Scholar
-
Urbina Coronado, P. D. et al. Part data integration in the shop floor digital twin: mobile and cloud technologies to enable a manufacturing execution system. J. Manuf. Syst. 48, 25–33 (2018).
Google Scholar
-
Bao, J., Guo, D., Li, J. & Zhang, J. The modelling and operations for the digital twin in the context of manufacturing. Enterp. Inf. Syst. 13, 534–556 (2019).
Google Scholar
-
Koulouris, A., Misailidis, N. & Petrides, D. Applications of process and digital twin models for production simulation and scheduling in the manufacturing of food ingredients and products. Food Bioprod. Process. 126, 317–333 (2021).
Google Scholar
-
Melesse, T. Y., Pasquale, V. D. & Riemma, S. Digital twin models in industrial operations: state-of-the-art and future research directions. IET Collab. Intell. Manuf. 3, 37–47 (2021).
Google Scholar
-
Tao, F., Xiao, B., Qi, Q., Cheng, J. & Ji, P. Digital twin modeling. J. Manuf. Syst. 64, 372–389 (2022).
Google Scholar
-
Rasheed, A., San, O. & Kvamsdal, T. Digital twin: values, challenges and enablers from a modeling perspective. IEEE Access 8, 21980–22012 (2020).
Google Scholar
-
Hürkamp, A. et al. Combining simulation and machine learning as digital twin for the manufacturing of overmolded thermoplastic composites. J. Manuf. Mater. Process 4, 92 (2020).
-
Tripura, T., Desai, A. S., Adhikari, S. & Chakraborty, S. Probabilistic machine learning based predictive and interpretable digital twin for dynamical systems. Comput. Struct. 281, 107008 (2023).
Google Scholar
-
Balu, A., Sarkar, S., Ganapathysubramanian, B. & Krishnamurthy, A. Physics-aware machine learning surrogates for real-time manufacturing digital twin. Manuf. Lett. 34, 71–74 (2022).
Google Scholar
-
Tabar, R. S., Wärmefjord, K., Söderberg, R. & Lindkvist, L. Efficient spot welding sequence optimization in a geometry assurance digital twin. J. Mech. Des. 142, 102001 (2020).
Google Scholar
-
Namiot, D., Pokusaev, O., Kupriyanovsky, V. & Zhabitskii, M. Digital twins and discrete-event simulation systems. Int. J. Open Inf. Technol. 9, 70–75 (2021).
-
Morabito, L., Ippolito, M., Pastore, E., Alfieri, A. & Montagna, F. A discrete event simulation based approach for digital twin implementation. IFAC Pap. 54, 414–419 (2021).
-
Ganguli, R. & Adhikari, S. The digital twin of discrete dynamic systems: initial approaches and future challenges. Appl. Math. Model. 77, 1110–1128 (2020).
Google Scholar
-
Söderberg, R., Wärmefjord, K., Carlson, J. S. & Lindkvist, L. Toward a digital twin for real-time geometry assurance in individualized production. CIRP Ann. 66, 137–140 (2017).
Google Scholar
-
Yan, Q., Wang, H. & Wu, F. Digital twin-enabled dynamic scheduling with preventive maintenance using a double-layer Q-learning algorithm. Comput. Oper. Res. 144, 105823 (2022).
Google Scholar
-
Guo, X., Peng, G. & Meng, Y. A modified Q-learning algorithm for t path planning in a digital twin assembly system. Int. J. Adv. Manuf. Technol. 119, 3951–3961 (2022).
Google Scholar
-
Chen, R., Shen, H. & Lai, Y. A metaheuristic optimization algorithm for energy efficiency in digital twins. Internet Things Cyber Phys. Syst. 2, 159–169 (2022).
Google Scholar
-
Bazaz, S. M., Lohtander, M. & Varis, J. The prediction method of tool life on small lot turning process—development of digital twin for production. Procedia Manuf. 51, 288–295 (2020).
Google Scholar
-
Zhang, H., Qi, Q., Ji, W. & Tao, F. An update method for digital twin multi-dimension models. Robot Comput. Integr. Manuf. 80, 102481 (2023).
Google Scholar
-
Eckhart, M. & Ekelhart, A. A specification-based state replication approach for digital twins. In Proc. 2018 Workshop on Cyber-Physical Systems Security and Privacy 36–47 (Association for Computing Machinery, 2018).
-
Akbarian, F., Fitzgerald, E. & Kihl, M. Synchronization in digital twins for industrial control systems. Preprint at https://arxiv.org/abs/2006.03447 (2020).
-
Seok, M. G., Tan, W. J., Cai, W. & Park, D. Digital-twin consistency checking based on observed timed events with unobservable transitions in smart manufacturing. IEEE Trans. Ind. Inform. 19, 6208–6219 (2023).
Google Scholar
-
Talkhestani, B. A., Jazdi, N., Schloegl, W. & Weyrich, M. Consistency check to synchronize the digital twin of manufacturing automation based on anchor points. Procedia CIRP 72, 159–164 (2018).
Google Scholar
-
Huang, S., Wang, G., Lei, D. & Yan, Y. Toward digital validation for rapid product development based on digital twin: a framework. Int. J. Adv. Manuf. Technol. 119, 2509–2523 (2022).
Google Scholar
-
Qamsane, Y. et al. A unified digital twin framework for real-time monitoring and evaluation of smart manufacturing systems. In 2019 IEEE 15th International Conference on Automation Science and Engineering (CASE) 1394–1401 (IEEE, 2019).
-
Agostino, Í. R. S., Broda, E., Frazzon, E. M. & Freitag, M. in Scheduling in Industry 4.0 and Cloud Manufacturing (eds Sokolov, B. et al.) 39–60 (Springer, 2020); https://doi.org/10.1007/978-3-030-43177-8_3
-
Aheleroff, S., Xu, X., Zhong, R. Y. & Lu, Y. Digital twin as a service (DTaaS) in Industry 4.0: an architecture reference model. Adv. Eng. Inform. 47, 101225 (2021).
Google Scholar
-
Fang, Y. et al. Digital-twin-based job shop scheduling toward smart manufacturing. IEEE Trans. Ind. Inform. 15, 6425–6435 (2019).
Google Scholar
-
Liu, D., Du, Y., Chai, W., Lu, C. & Cong, M. Digital twin and data-driven quality prediction of complex die-casting manufacturing. IEEE Trans. Ind. Inform. 18, 8119–8128 (2022).
Google Scholar
-
Aivaliotis, P., Georgoulias, K. & Chryssolouris, G. The use of digital twin for predictive maintenance in manufacturing. Int. J. Comput. Integr. Manuf. 32, 1067–1080 (2019).
Google Scholar
-
Errandonea, I., Beltrán, S. & Arrizabalaga, S. Digital twin for maintenance: a literature review. Comput. Ind. 123, 103316 (2020).
Google Scholar
-
van Dinter, R., Tekinerdogan, B. & Catal, C. Predictive maintenance using digital twins: a systematic literature review. Inf. Softw. Technol. 151, 107008 (2022).
Google Scholar
-
Ribeiro da Silva, E., Assad Neto, A. & Nielsen, C. P. in The Future of Smart Production for SMEs: A Methodological and Practical Approach Towards Digitalization in SMEs (eds Madsen, O. et al.) 343–348 (Springer, 2023).
-
Wanasinghe, T. R. et al. Digital twin for the oil and gas industry: overview, research trends, opportunities, and challenges. IEEE Access 8, 104175–104197 (2020).
Google Scholar
-
Faraway, J. J. & Augustin, N. H. When small data beats big data. Stat. Probab. Lett. 136, 142–145 (2018).
Google Scholar
-
Yu, J., Song, Y., Tang, D. & Dai, J. A digital twin approach based on nonparametric Bayesian network for complex system health monitoring. J. Manuf. Syst. 58, 293–304 (2021).
Google Scholar
-
Dang, S. et al. What should 6G be? Nat. Electron. 3, 20–29 (2020).
Google Scholar
-
Saad, W., Bennis, M. & Chen, M. A vision of 6G wireless systems: applications, trends, technologies, and open research problems. IEEE Netw. 34, 134–142 (2020).
Google Scholar
-
Priyanka, E. B., Thangavel, S., Gao, X.-Z. & Sivakumar, N. S. Digital twin for oil pipeline risk estimation using prognostic and machine learning techniques. J. Ind. Inf. Integr. 26, 100272 (2022).
-
Chen, Z., Zou, J. & Wang, W. Digital twin-oriented collaborative optimization of fuzzy flexible job shop scheduling under multiple uncertainties. Sādhanā 48, 78 (2023).
Google Scholar
-
Singh, R. & Gill, S. S. Edge AI: a survey. Internet Things Cyber Phys. Syst. 3, 71–92 (2023).
Google Scholar
-
Olortegui-Yume, J. A. & Kwon, P. Y. Tool wear mechanisms in machining. Int. J. Mach. Mach. Mater. 2, 316–334 (2007).
-
Suo, S. et al. Encryption technology in information system security. In 3rd International Conference on Mechatronics Engineering and Information Technology (ICMEIT 2019) 495–499 (Springer, 2019).
-
Darabseh, A. et al. SDStorage: a software defined storage experimental framework. In 2015 IEEE International Conference on Cloud Engineering 341–346 (IEEE, 2015).
-
Gu, M., Li, X. & Cao, Y. Optical storage arrays: a perspective for future big data storage. Light. Sci. Appl. 3, e177 (2014).
Google Scholar
-
Lv, Z. & Xie, S. Artificial intelligence in the digital twins: state of the art, challenges, and future research topics. Digit. Twin 1, 12 (2022).
Google Scholar
-
Carabantes, M. Black-box artificial intelligence: an epistemological and critical analysis. AI Soc. 35, 309–317 (2020).
Google Scholar
-
Blazek, P. J. & Lin, M. M. Explainable neural networks that simulate reasoning. Nat. Comput. Sci. 1, 607–618 (2021).
Google Scholar
-
Lundberg, S. M. et al. From local explanations to global understanding with explainable AI for trees. Nat. Mach. Intell. 2, 56–67 (2020).
Google Scholar
-
Gunning, D. et al. XAI—explainable artificial intelligence. Sci. Robot. 4, eaay7120 (2019).
Google Scholar
-
Khan, A., Shahid, F., Maple, C., Ahmad, A. & Jeon, G. Toward smart manufacturing using spiral digital twin framework and twinchain. IEEE Trans. Ind. Inform. 18, 1359–1366 (2022).
Google Scholar
-
Suhail, S. et al. Blockchain-based digital twins: research trends, issues, and future challenges. ACM Comput. Surv. 54, 240:1–240:34 (2022).
Google Scholar
-
Yaqoob, I. et al. Blockchain for digital twins: recent advances and future research challenges. IEEE Netw. 34, 290–298 (2020).
Google Scholar
-
Tao, F. et al. makeTwin: a reference architecture for digital twin software platform. Chin. J. Aeronaut. https://doi.org/10.1016/j.cja.2023.05.002 (2023).
Google Scholar
-
Niederer, S. A. et al. Scaling digital twins from the artisanal to the industrial. Nat. Comput. Sci. 1, 313–320 (2021).
Google Scholar
-
Suhail, S., Jurdak, R. & Hussain, R. Security attacks and solutions for digital twins. Preprint at https://doi.org/10.48550/arXiv.2202.12501 (2023).
Acknowledgements
This work is financially supported by the National Natural Science Foundation of China (NSFC) under grants 52120105008 and 52275471, the National Key Research and Development Program of China under grant 2020YFB1708400, and the New Cornerstone Science Foundation through the XPLORER PRIZE.
Author information
Authors and Affiliations
Contributions
All authors contributed to the writing and editing of this paper.
Corresponding author
Ethics declarations
Competing interests
The authors declare no competing interests.
Peer review
Peer review information
Nature Computational Science thanks Michael Grieves, George Q. Huang and Jay Lee for their contribution to the peer review of this work. Primary Handling Editor: Fernando Chirigati, in collaboration with the Nature Computational Science team.
Additional information
Publisher’s note Springer Nature remains neutral with regard to jurisdictional claims in published maps and institutional affiliations.
Rights and permissions
Springer Nature or its licensor (e.g. a society or other partner) holds exclusive rights to this article under a publishing agreement with the author(s) or other rightsholder(s); author self-archiving of the accepted manuscript version of this article is solely governed by the terms of such publishing agreement and applicable law.
Reprints and permissions
About this article
Cite this article
Tao, F., Zhang, H. & Zhang, C. Advancements and challenges of digital twins in industry.
Nat Comput Sci 4, 169–177 (2024). https://doi.org/10.1038/s43588-024-00603-w
-
Received: 30 July 2023
-
Accepted: 06 February 2024
-
Published: 26 March 2024
-
Issue Date: March 2024
-
DOI: https://doi.org/10.1038/s43588-024-00603-w