Abstract
Accelerating catalyst discovery and development is of paramount importance in addressing the global energy, sustainability and healthcare demands. The past decade has witnessed significant momentum in harnessing data science concepts in catalysis research to aid the aforementioned cause. Here we comprehensively review how catalysis practitioners have leveraged data-driven strategies to solve complex challenges across heterogeneous, homogeneous and enzymatic catalysis. We delineate all studies into deductive or inductive modes, and statistically infer the prevalence of catalytic tasks, model reactions, data representations and choice of algorithms. We highlight frontiers in the field and knowledge transfer opportunities among the catalysis subdisciplines. Our critical assessment reveals a glaring gap in data science exploration in experimental catalysis, which we bridge by elaborating on four pillars of data science, namely descriptive, predictive, causal and prescriptive analytics. We advocate their adoption into routine experimental workflows and underscore the importance of data standardization to spur future research in digital catalysis.
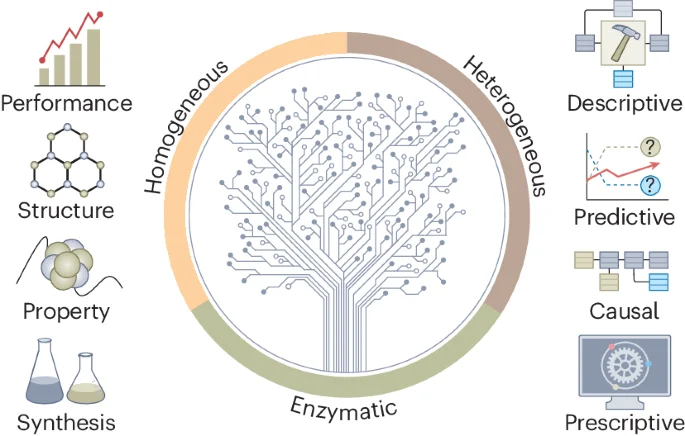
This is a preview of subscription content, access via your institution
Access options
style{display:none!important}.LiveAreaSection-193358632 *{align-content:stretch;align-items:stretch;align-self:auto;animation-delay:0s;animation-direction:normal;animation-duration:0s;animation-fill-mode:none;animation-iteration-count:1;animation-name:none;animation-play-state:running;animation-timing-function:ease;azimuth:center;backface-visibility:visible;background-attachment:scroll;background-blend-mode:normal;background-clip:borderBox;background-color:transparent;background-image:none;background-origin:paddingBox;background-position:0 0;background-repeat:repeat;background-size:auto auto;block-size:auto;border-block-end-color:currentcolor;border-block-end-style:none;border-block-end-width:medium;border-block-start-color:currentcolor;border-block-start-style:none;border-block-start-width:medium;border-bottom-color:currentcolor;border-bottom-left-radius:0;border-bottom-right-radius:0;border-bottom-style:none;border-bottom-width:medium;border-collapse:separate;border-image-outset:0s;border-image-repeat:stretch;border-image-slice:100%;border-image-source:none;border-image-width:1;border-inline-end-color:currentcolor;border-inline-end-style:none;border-inline-end-width:medium;border-inline-start-color:currentcolor;border-inline-start-style:none;border-inline-start-width:medium;border-left-color:currentcolor;border-left-style:none;border-left-width:medium;border-right-color:currentcolor;border-right-style:none;border-right-width:medium;border-spacing:0;border-top-color:currentcolor;border-top-left-radius:0;border-top-right-radius:0;border-top-style:none;border-top-width:medium;bottom:auto;box-decoration-break:slice;box-shadow:none;box-sizing:border-box;break-after:auto;break-before:auto;break-inside:auto;caption-side:top;caret-color:auto;clear:none;clip:auto;clip-path:none;color:initial;column-count:auto;column-fill:balance;column-gap:normal;column-rule-color:currentcolor;column-rule-style:none;column-rule-width:medium;column-span:none;column-width:auto;content:normal;counter-increment:none;counter-reset:none;cursor:auto;display:inline;empty-cells:show;filter:none;flex-basis:auto;flex-direction:row;flex-grow:0;flex-shrink:1;flex-wrap:nowrap;float:none;font-family:initial;font-feature-settings:normal;font-kerning:auto;font-language-override:normal;font-size:medium;font-size-adjust:none;font-stretch:normal;font-style:normal;font-synthesis:weight style;font-variant:normal;font-variant-alternates:normal;font-variant-caps:normal;font-variant-east-asian:normal;font-variant-ligatures:normal;font-variant-numeric:normal;font-variant-position:normal;font-weight:400;grid-auto-columns:auto;grid-auto-flow:row;grid-auto-rows:auto;grid-column-end:auto;grid-column-gap:0;grid-column-start:auto;grid-row-end:auto;grid-row-gap:0;grid-row-start:auto;grid-template-areas:none;grid-template-columns:none;grid-template-rows:none;height:auto;hyphens:manual;image-orientation:0deg;image-rendering:auto;image-resolution:1dppx;ime-mode:auto;inline-size:auto;isolation:auto;justify-content:flexStart;left:auto;letter-spacing:normal;line-break:auto;line-height:normal;list-style-image:none;list-style-position:outside;list-style-type:disc;margin-block-end:0;margin-block-start:0;margin-bottom:0;margin-inline-end:0;margin-inline-start:0;margin-left:0;margin-right:0;margin-top:0;mask-clip:borderBox;mask-composite:add;mask-image:none;mask-mode:matchSource;mask-origin:borderBox;mask-position:0 0;mask-repeat:repeat;mask-size:auto;mask-type:luminance;max-height:none;max-width:none;min-block-size:0;min-height:0;min-inline-size:0;min-width:0;mix-blend-mode:normal;object-fit:fill;object-position:50% 50%;offset-block-end:auto;offset-block-start:auto;offset-inline-end:auto;offset-inline-start:auto;opacity:1;order:0;orphans:2;outline-color:initial;outline-offset:0;outline-style:none;outline-width:medium;overflow:visible;overflow-wrap:normal;overflow-x:visible;overflow-y:visible;padding-block-end:0;padding-block-start:0;padding-bottom:0;padding-inline-end:0;padding-inline-start:0;padding-left:0;padding-right:0;padding-top:0;page-break-after:auto;page-break-before:auto;page-break-inside:auto;perspective:none;perspective-origin:50% 50%;pointer-events:auto;position:static;quotes:initial;resize:none;right:auto;ruby-align:spaceAround;ruby-merge:separate;ruby-position:over;scroll-behavior:auto;scroll-snap-coordinate:none;scroll-snap-destination:0 0;scroll-snap-points-x:none;scroll-snap-points-y:none;scroll-snap-type:none;shape-image-threshold:0;shape-margin:0;shape-outside:none;tab-size:8;table-layout:auto;text-align:initial;text-align-last:auto;text-combine-upright:none;text-decoration-color:currentcolor;text-decoration-line:none;text-decoration-style:solid;text-emphasis-color:currentcolor;text-emphasis-position:over right;text-emphasis-style:none;text-indent:0;text-justify:auto;text-orientation:mixed;text-overflow:clip;text-rendering:auto;text-shadow:none;text-transform:none;text-underline-position:auto;top:auto;touch-action:auto;transform:none;transform-box:borderBox;transform-origin:50% 50%0;transform-style:flat;transition-delay:0s;transition-duration:0s;transition-property:all;transition-timing-function:ease;vertical-align:baseline;visibility:visible;white-space:normal;widows:2;width:auto;will-change:auto;word-break:normal;word-spacing:normal;word-wrap:normal;writing-mode:horizontalTb;z-index:auto;-webkit-appearance:none;-moz-appearance:none;-ms-appearance:none;appearance:none;margin:0}.LiveAreaSection-193358632{width:100%}.LiveAreaSection-193358632 .login-option-buybox{display:block;width:100%;font-size:17px;line-height:30px;color:#222;padding-top:30px;font-family:Harding,Palatino,serif}.LiveAreaSection-193358632 .additional-access-options{display:block;font-weight:700;font-size:17px;line-height:30px;color:#222;font-family:Harding,Palatino,serif}.LiveAreaSection-193358632 .additional-login>li:not(:first-child)::before{transform:translateY(-50%);content:””;height:1rem;position:absolute;top:50%;left:0;border-left:2px solid #999}.LiveAreaSection-193358632 .additional-login>li:not(:first-child){padding-left:10px}.LiveAreaSection-193358632 .additional-login>li{display:inline-block;position:relative;vertical-align:middle;padding-right:10px}.BuyBoxSection-683559780{display:flex;flex-wrap:wrap;flex:1;flex-direction:row-reverse;margin:-30px -15px 0}.BuyBoxSection-683559780 .box-inner{width:100%;height:100%;padding:30px 5px;display:flex;flex-direction:column;justify-content:space-between}.BuyBoxSection-683559780 p{margin:0}.BuyBoxSection-683559780 .readcube-buybox{background-color:#f3f3f3;flex-shrink:1;flex-grow:1;flex-basis:255px;background-clip:content-box;padding:0 15px;margin-top:30px}.BuyBoxSection-683559780 .subscribe-buybox{background-color:#f3f3f3;flex-shrink:1;flex-grow:4;flex-basis:300px;background-clip:content-box;padding:0 15px;margin-top:30px}.BuyBoxSection-683559780 .subscribe-buybox-nature-plus{background-color:#f3f3f3;flex-shrink:1;flex-grow:4;flex-basis:100%;background-clip:content-box;padding:0 15px;margin-top:30px}.BuyBoxSection-683559780 .title-readcube,.BuyBoxSection-683559780 .title-buybox{display:block;margin:0;margin-right:10%;margin-left:10%;font-size:24px;line-height:32px;color:#222;text-align:center;font-family:Harding,Palatino,serif}.BuyBoxSection-683559780 .title-asia-buybox{display:block;margin:0;margin-right:5%;margin-left:5%;font-size:24px;line-height:32px;color:#222;text-align:center;font-family:Harding,Palatino,serif}.BuyBoxSection-683559780 .asia-link{color:#069;cursor:pointer;text-decoration:none;font-size:1.05em;font-family:-apple-system,BlinkMacSystemFont,”Segoe UI”,Roboto,Oxygen-Sans,Ubuntu,Cantarell,”Helvetica Neue”,sans-serif;line-height:1.05em6}.BuyBoxSection-683559780 .access-readcube{display:block;margin:0;margin-right:10%;margin-left:10%;font-size:14px;color:#222;padding-top:10px;text-align:center;font-family:-apple-system,BlinkMacSystemFont,”Segoe UI”,Roboto,Oxygen-Sans,Ubuntu,Cantarell,”Helvetica Neue”,sans-serif;line-height:20px}.BuyBoxSection-683559780 ul{margin:0}.BuyBoxSection-683559780 .link-usp{display:list-item;margin:0;margin-left:20px;padding-top:6px;list-style-position:inside}.BuyBoxSection-683559780 .link-usp span{font-size:14px;color:#222;font-family:-apple-system,BlinkMacSystemFont,”Segoe UI”,Roboto,Oxygen-Sans,Ubuntu,Cantarell,”Helvetica Neue”,sans-serif;line-height:20px}.BuyBoxSection-683559780 .access-asia-buybox{display:block;margin:0;margin-right:5%;margin-left:5%;font-size:14px;color:#222;padding-top:10px;text-align:center;font-family:-apple-system,BlinkMacSystemFont,”Segoe UI”,Roboto,Oxygen-Sans,Ubuntu,Cantarell,”Helvetica Neue”,sans-serif;line-height:20px}.BuyBoxSection-683559780 .access-buybox{display:block;margin:0;margin-right:10%;margin-left:10%;font-size:14px;color:#222;opacity:.8px;padding-top:10px;text-align:center;font-family:-apple-system,BlinkMacSystemFont,”Segoe UI”,Roboto,Oxygen-Sans,Ubuntu,Cantarell,”Helvetica Neue”,sans-serif;line-height:20px}.BuyBoxSection-683559780 .price-buybox{display:block;font-size:30px;color:#222;font-family:-apple-system,BlinkMacSystemFont,”Segoe UI”,Roboto,Oxygen-Sans,Ubuntu,Cantarell,”Helvetica Neue”,sans-serif;padding-top:30px;text-align:center}.BuyBoxSection-683559780 .price-buybox-to{display:block;font-size:30px;color:#222;font-family:-apple-system,BlinkMacSystemFont,”Segoe UI”,Roboto,Oxygen-Sans,Ubuntu,Cantarell,”Helvetica Neue”,sans-serif;text-align:center}.BuyBoxSection-683559780 .price-info-text{font-size:16px;padding-right:10px;color:#222;font-family:-apple-system,BlinkMacSystemFont,”Segoe UI”,Roboto,Oxygen-Sans,Ubuntu,Cantarell,”Helvetica Neue”,sans-serif}.BuyBoxSection-683559780 .price-value{font-size:30px;font-family:-apple-system,BlinkMacSystemFont,”Segoe UI”,Roboto,Oxygen-Sans,Ubuntu,Cantarell,”Helvetica Neue”,sans-serif}.BuyBoxSection-683559780 .price-per-period{font-family:-apple-system,BlinkMacSystemFont,”Segoe UI”,Roboto,Oxygen-Sans,Ubuntu,Cantarell,”Helvetica Neue”,sans-serif}.BuyBoxSection-683559780 .price-from{font-size:14px;padding-right:10px;color:#222;font-family:-apple-system,BlinkMacSystemFont,”Segoe UI”,Roboto,Oxygen-Sans,Ubuntu,Cantarell,”Helvetica Neue”,sans-serif;line-height:20px}.BuyBoxSection-683559780 .issue-buybox{display:block;font-size:13px;text-align:center;color:#222;font-family:-apple-system,BlinkMacSystemFont,”Segoe UI”,Roboto,Oxygen-Sans,Ubuntu,Cantarell,”Helvetica Neue”,sans-serif;line-height:19px}.BuyBoxSection-683559780 .no-price-buybox{display:block;font-size:13px;line-height:18px;text-align:center;padding-right:10%;padding-left:10%;padding-bottom:20px;padding-top:30px;color:#222;font-family:-apple-system,BlinkMacSystemFont,”Segoe UI”,Roboto,Oxygen-Sans,Ubuntu,Cantarell,”Helvetica Neue”,sans-serif}.BuyBoxSection-683559780 .vat-buybox{display:block;margin-top:5px;margin-right:20%;margin-left:20%;font-size:11px;color:#222;padding-top:10px;padding-bottom:15px;text-align:center;font-family:-apple-system,BlinkMacSystemFont,”Segoe UI”,Roboto,Oxygen-Sans,Ubuntu,Cantarell,”Helvetica Neue”,sans-serif;line-height:17px}.BuyBoxSection-683559780 .tax-buybox{display:block;width:100%;color:#222;padding:20px 16px;text-align:center;font-family:-apple-system,BlinkMacSystemFont,”Segoe UI”,Roboto,Oxygen-Sans,Ubuntu,Cantarell,”Helvetica Neue”,sans-serif;line-height:NaNpx}.BuyBoxSection-683559780 .button-container{display:flex;padding-right:20px;padding-left:20px;justify-content:center}.BuyBoxSection-683559780 .button-container>*{flex:1px}.BuyBoxSection-683559780 .button-container>a:hover,.Button-505204839:hover,.Button-1078489254:hover,.Button-2737859108:hover{text-decoration:none}.BuyBoxSection-683559780 .btn-secondary{background:#fff}.BuyBoxSection-683559780 .button-asia{background:#069;border:1px solid #069;border-radius:0;cursor:pointer;display:block;padding:9px;outline:0;text-align:center;text-decoration:none;min-width:80px;margin-top:75px}.BuyBoxSection-683559780 .button-label-asia,.ButtonLabel-3869432492,.ButtonLabel-3296148077,.ButtonLabel-1636778223{display:block;color:#fff;font-size:17px;line-height:20px;font-family:-apple-system,BlinkMacSystemFont,”Segoe UI”,Roboto,Oxygen-Sans,Ubuntu,Cantarell,”Helvetica Neue”,sans-serif;text-align:center;text-decoration:none;cursor:pointer}.Button-505204839,.Button-1078489254,.Button-2737859108{background:#069;border:1px solid #069;border-radius:0;cursor:pointer;display:block;padding:9px;outline:0;text-align:center;text-decoration:none;min-width:80px;max-width:320px;margin-top:20px}.Button-505204839 .btn-secondary-label,.Button-1078489254 .btn-secondary-label,.Button-2737859108 .btn-secondary-label{color:#069}
/* style specs end */
Access Nature and 54 other Nature Portfolio journals
Get Nature+, our best-value online-access subscription
$29.99 / 30 days
cancel any time
Subscribe to this journal
Receive 12 digital issues and online access to articles
$119.00 per year
only $9.92 per issue
Buy this article
- Purchase on Springer Link
- Instant access to full article PDF
Prices may be subject to local taxes which are calculated during checkout
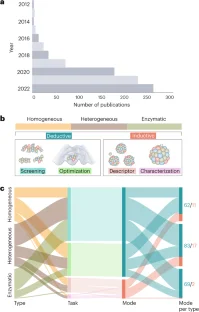
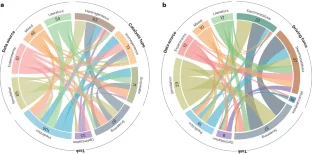
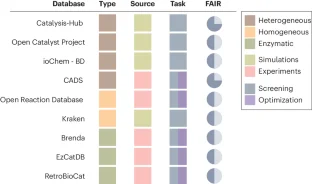
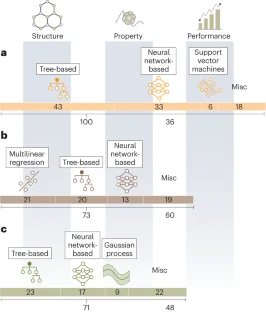
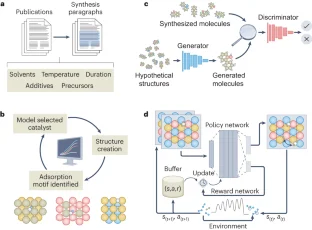
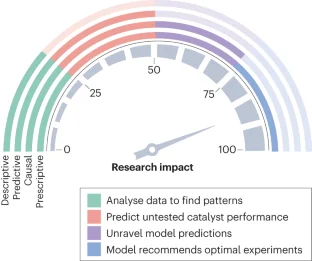
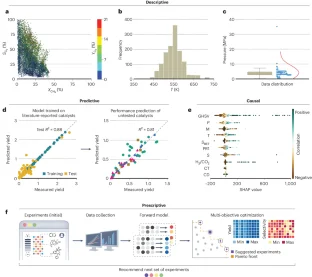
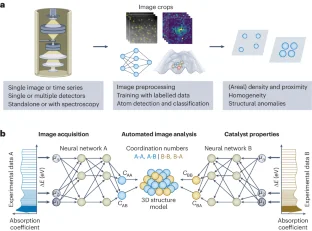
References
-
Vogt, C. & Weckhuysen, B. M. The concept of active site in heterogeneous catalysis. Nat. Rev. Chem. 6, 89–111 (2022).
Google Scholar
-
Ye, R., Zhao, J., Wickemeyer, B. B., Toste, F. D. & Somorjai, G. A. Foundations and strategies of the construction of hybrid catalysts for optimized performances. Nat. Catal. 1, 318–325 (2018).
Google Scholar
-
Copéret, C., Chabanas, M., Petroff Saint-Arroman, R. & Basset, J. M. Homogeneous and heterogeneous catalysis: bridging the gap through surface organometallic chemistry. Angew. Chem. Int. Ed. 42, 156–181 (2003).
Google Scholar
-
Ye, R., Hurlburt, T. J., Sabyrov, K., Alayoglu, S. & Somorjai, G. A. Molecular catalysis science: perspective on unifying the fields of catalysis. Proc. Natl Acad. Sci. USA 113, 5159–5166 (2016).
Google Scholar
-
Zhao, B., Han, Z. & Ding, K. The N-H functional group in organometallic catalysis. Angew. Chem. Int. Ed. 52, 4744–4788 (2013).
Google Scholar
-
Sheldon, R. A. & Woodley, J. M. Role of biocatalysis in sustainable chemistry. Chem. Rev. 118, 801–838 (2018).
Google Scholar
-
Munnik, P., de Jongh, P. E. & de Jong, K. P. Recent developments in the synthesis of supported catalysts. Chem. Rev. 115, 6687–6718 (2015).
Google Scholar
-
Bornscheuer, U. T. et al. Engineering the third wave of biocatalysis. Nature 485, 185–194 (2012).
Google Scholar
-
Grunwaldt, J.-D. & Schroer, C. G. Hard and soft X-ray microscopy and tomography in catalysis: bridging the different time and length scales. Chem. Soc. Rev. 39, 4741–4753 (2010).
Google Scholar
-
Meirer, F. & Weckhuysen, B. M. Spatial and temporal exploration of heterogeneous catalysts with synchrotron radiation. Nat. Rev. Mater. 3, 324–340 (2018).
Google Scholar
-
Chen, B. W. J., Xu, L. & Mavrikakis, M. Computational methods in heterogeneous catalysis. Chem. Rev. 121, 1007–1048 (2021).
Google Scholar
-
Durand, D. J. & Fey, N. Computational ligand descriptors for catalyst design. Chem. Rev. 119, 6561–6594 (2019).
Google Scholar
-
Wang, H. et al. Scientific discovery in the age of artificial intelligence. Nature 620, 47–60 (2023).
Google Scholar
-
LeCun, Y., Bengio, Y. & Hinton, G. Deep learning. Nature 521, 436–444 (2015).
Google Scholar
-
Kitchin, J. R. Machine learning in catalysis. Nat. Catal. 1, 230–232 (2018).
Google Scholar
-
Toyao, T. et al. Machine learning for catalysis informatics: recent applications and prospects. ACS Catal. 10, 2260–2297 (2020).
Google Scholar
-
Ma, X., Li, Z., Achenie, L. E. K. & Xin, H. Machine-learning-augmented chemisorption model for CO2 electroreduction catalyst screening. J. Phys. Chem. Lett. 6, 3528–3533 (2015).
Google Scholar
-
Ahneman, D. T., Estrada, J. G., Lin, S., Dreher, S. D. & Doyle, A. G. Predicting reaction performance in C-N cross-coupling using machine learning. Science 360, 186–190 (2018). The application of interpretable machine learning on a high-throughput Buchwald–Hartwig dataset to predict high-performing palladium catalysts and unravel their inhibition mechanism.
Google Scholar
-
Kim, M. et al. Searching for an optimal multi-metallic alloy catalyst by active learning combined with experiments. Adv. Mater. 34, 2108900 (2022).
Google Scholar
-
Shields, B. J. et al. Bayesian reaction optimization as a tool for chemical synthesis. Nature 590, 89–96 (2021). Development of Bayesian optimization on palladium-catalysed Mitsunobu and deoxyfluorination reactions where the algorithm consistently outperformed human decision-making in terms number of experiments and actual yields to optimize the process.
Google Scholar
-
Wu, Z., Kan, S. B. J., Lewis, R. D., Wittmann, B. J. & Arnold, F. H. Machine learning-assisted directed protein evolution with combinatorial libraries. Proc. Natl Acad. Sci. USA 116, 8852–8858 (2019).
Google Scholar
-
Lu, H. et al. Machine learning-aided engineering of hydrolases for PET depolymerization. Nature 604, 662–667 (2022).
Google Scholar
-
Ulissi, Z. W., Medford, A. J., Bligaard, T. & Nørskov, J. K. To address surface reaction network complexity using scaling relations machine learning and DFT calculations. Nat. Commun. 8, 14621 (2017).
Google Scholar
-
Li, F. et al. Deep learning-based kcat prediction enables improved enzyme-constrained model reconstruction. Nat. Catal. 5, 662–672 (2022). A deep learning methodology to predict enzyme turnover numbers of metabolic enzymes from any organism merely from substrate structures and protein sequences.
Google Scholar
-
Holeňa, M. & Baerns, M. Feedforward neural networks in catalysis: a tool for the approximation of the dependency of yield on catalyst composition and for knowledge extraction. Catal. Today 81, 485–494 (2003). Amongst the earliest reports on applied machine learning in catalysis, wherein a feedforward neural network was used to predict propene yield based on the catalyst composition.
Google Scholar
-
Baumes, L., Farrusseng, D., Lengliz, M. & Mirodatos, C. Using artificial neural networks to boost high-throughput discovery in heterogeneous catalysis. QSAR Comb. Sci. 23, 767–778 (2004).
Google Scholar
-
Burello, E., Farrusseng, D. & Rothenberg, G. Combinatorial explosion in homogeneous catalysis: screening 60,000 cross-coupling reactions. Adv. Synth. Catal. 346, 1844–1853 (2004).
Google Scholar
-
Corma, A. et al. Optimisation of olefin epoxidation catalysts with the application of high-throughput and genetic algorithms assisted by artificial neural networks (softcomputing techniques). J. Catal. 229, 513–524 (2005).
Google Scholar
-
Venkatasubramanian, V. The promise of artificial intelligence in chemical engineering: is it here, finally? AIChE J. 65, 466–478 (2019).
Google Scholar
-
Pyzer-Knapp, E. O. et al. Accelerating materials discovery using artificial intelligence, high performance computing and robotics. NPJ Comput. Mater. 8, 84 (2022).
Google Scholar
-
Pedregosa, F. et al. Scikit-learn: machine learning in Python. J. Mach. Learn. Res. 12, 2825–2830 (2011).
-
RDKit; https://www.rdkit.org/
-
Chanussot, L. et al. Open catalyst 2020 (OC20) dataset and community challenges. ACS Catal. 11, 6059–6072 (2021). The most extensive database consisting of close to 1.3 million density DFT relaxations across a wide swath of materials, surfaces and adsorbates (nitrogen, carbon and oxygen chemistries) for application in heterogeneous catalysis.
Google Scholar
-
Kearnes, S. M. et al. The open reaction database. J. Am. Chem. Soc. 143, 18820–18826 (2021).
Google Scholar
-
Yano, J. et al. The case for data science in experimental chemistry: examples and recommendations. Nat. Rev. Chem. 6, 357–370 (2022).
Google Scholar
-
Schlexer Lamoureux, P. et al. Machine learning for computational heterogeneous catalysis. ChemCatChem 11, 3581–3601 (2019).
Google Scholar
-
Medford, A. J., Kunz, M. R., Ewing, S. M., Borders, T. & Fushimi, R. Extracting knowledge from data through catalysis informatics. ACS Catal. 8, 7403–7429 (2018).
Google Scholar
-
Maldonado, A. G. & Rothenberg, G. Predictive modeling in homogeneous catalysis: a tutorial. Chem. Soc. Rev. 39, 1891–1902 (2010).
Google Scholar
-
Mazurenko, S., Prokop, Z. & Damborsky, J. Machine learning in enzyme engineering. ACS Catal. 10, 1210–1223 (2020).
Google Scholar
-
Suvarna, M. & Pérez-Ramírez, J. Dataset: Embracing Data Science in Catalysis Research (Zenodo, 2024); https://doi.org/10.5281/zenodo.10640876
-
Zahrt, A. F. et al. Prediction of higher-selectivity catalysts by computer-driven workflow and machine learning. Science 363, eaau5631 (2019). The study models multiple conformations of more than 800 prospective catalysts for the coupling reaction of imines and thiols, and trained machine learning algorithms on a subset of experimental results, to achieve highly accurate predictions of enantioselectivities.
Google Scholar
-
Nguyen, T. N. et al. High-throughput experimentation and catalyst informatics for oxidative coupling of methane. ACS Catal. 10, 921–932 (2020).
Google Scholar
-
Tran, K. & Ulissi, Z. W. Active learning across intermetallics to guide discovery of electrocatalysts for CO2 reduction and H2 evolution. Nat. Catal. 1, 696–703 (2018). A fully automated screening method developed by integrating machine learning and optimization algorithms to guide DFT calculations, for in silico prediction of electrocatalyst performance for CO2 reduction and H2 evolution.
Google Scholar
-
Wang, G. et al. Accelerated discovery of multi-elemental reverse water–gas shift catalysts using extrapolative machine learning approach. Nat. Commun. 14, 5861 (2023).
Google Scholar
-
Amar, Y., Schweidtmann, A. M., Deutsch, P., Cao, L. & Lapkin, A. Machine learning and molecular descriptors enable rational solvent selection in asymmetric catalysis. Chem. Sci. 10, 6697–6706 (2019).
Google Scholar
-
Rinehart, N. I. et al. A machine-learning tool to predict substrate-adaptive conditions for Pd-catalyzed C-N couplings. Science 381, 965–972 (2023).
Google Scholar
-
Schweidtmann, A. M. et al. Machine learning meets continuous flow chemistry: automated optimization towards the pareto front of multiple objectives. Chem. Eng. J. 352, 277–282 (2018).
Google Scholar
-
O’Connor, N. J., Jonayat, A. S. M., Janik, M. J. & Senftle, T. P. Interaction trends between single metal atoms and oxide supports identified with density functional theory and statistical learning. Nat. Catal. 1, 531–539 (2018).
Google Scholar
-
Foppa, L. et al. Materials genes of heterogeneous catalysis from clean experiments and artificial intelligence. MRS Bull. 46, 1016–1026 (2021).
Google Scholar
-
Zhao, S. et al. Enantiodivergent Pd-catalyzed C-C bond formation enabled through ligand parameterization. Science 362, 670–674 (2018).
Google Scholar
-
Timoshenko, J., Lu, D., Lin, Y. & Frenkel, A. I. Supervised machine-learning-based determination of three-dimensional structure of metallic nanoparticles. J. Phys. Chem. Lett. 8, 5091–5098 (2017). Application of deep learning to solve metal catalyst from XANES, broadly applicable to the determination of nanoparticle structures in operando studies and generalizable to other nanoscale systems.
Google Scholar
-
Zheng, C. et al. Automated generation and ensemble-learned matching of X-ray absorption spectra. NPJ Comput. Mater. 4, 12 (2018).
Google Scholar
-
Mitchell, S. et al. Automated image analysis for single-atom detection in catalytic materials by transmission electron microscopy. J. Am. Chem. Soc. 144, 8018–8029 (2022).
Google Scholar
-
Büchler, J. et al. Algorithm-aided engineering of aliphatic halogenase WelO5* for the asymmetric late-stage functionalization of soraphens. Nat. Commun. 13, 371 (2022).
Google Scholar
-
Wulf, C. et al. A unified research data infrastructure for catalysis research – challenges and concepts. ChemCatChem 13, 3223–3236 (2021).
Google Scholar
-
Mendes, P. S. F., Siradze, S., Pirro, L. & Thybaut, J. W. Open data in catalysis: from today’s big picture to the future of small data. ChemCatChem 13, 836–850 (2021).
Google Scholar
-
Marshall, C. P., Schumann, J. & Trunschke, A. Achieving digital catalysis: strategies for data acquisition, storage and use. Angew. Chem. Int. Ed. 62, e202302971 (2023).
Google Scholar
-
Zavyalova, U., Holena, M., Schlögl, R. & Baerns, M. Statistical analysis of past catalytic data on oxidative methane coupling for new insights into the composition of high-performance catalysts. ChemCatChem 3, 1935–1947 (2011).
Google Scholar
-
Odabasi, C., Gunay, M. E. & Yildrim, R. Knowledge extraction for water gas shift reaction over noble metal catalysts from publications in the literature between 2002 and 2012. Int. J. Hydrog. Energy 39, 5733–5746 (2014).
Google Scholar
-
Suvarna, M., Araújo, T. P. & Pérez-Ramírez, J. A generalized machine learning framework to predict the space-time yield of methanol from thermocatalytic CO2 hydrogenation. Appl. Catal. B Environ. 315, 121530 (2022).
Google Scholar
-
Mamun, O., Winther, K. T., Boes, J. R. & Bligaard, T. High-throughput calculations of catalytic properties of bimetallic alloy surfaces. Sci. Data 6, 76 (2019).
Google Scholar
-
Jinnouchi, R. & Asahi, R. Predicting catalytic activity of nanoparticles by a DFT-aided machine-learning algorithm. J. Phys. Chem. Lett. 8, 4279–4283 (2017).
Google Scholar
-
Berman, H. M. et al. The protein data bank. Nucleic Acids Res. 28, 235–242 (2000).
Google Scholar
-
Mitchell, A. L. et al. InterPro in 2019: improving coverage, classification and access to protein sequence annotations. Nucleic Acids Res. 47, D351–D360 (2019).
Google Scholar
-
UniProt Consortium. UniProt: a worldwide hub of protein knowledge. Nucleic Acids Res. 47, D506–D515 (2019).
Google Scholar
-
Schomburg, I., Chang, A. & Schomburg, D. BRENDA, enzyme data and metabolic information. Nucleic Acids Res. 30, 47–49 (2002).
Google Scholar
-
Nagano, N. EzCatDB: the enzyme catalytic-mechanism database. Nucleic Acids Res. 33, D407–D412 (2005).
Google Scholar
-
Finnigan, W. et al. RetroBioCat as a computer-aided synthesis planning tool for biocatalytic reactions and cascades. Nat. Catal. 4, 98–104 (2021).
Google Scholar
-
Winther, K. T. et al. Catalysis-Hub.org, an open electronic structure database for surface reactions. Sci. Data 6, 75 (2019).
Google Scholar
-
Álvarez-Moreno, M. et al. Managing the computational chemistry big data problem: the ioChem-BD platform. J. Chem. Inf. Model. 55, 95–103 (2015).
Google Scholar
-
Gensch, T. et al. A comprehensive discovery platform for organophosphorus ligands for catalysis. J. Am. Chem. Soc. 144, 1205–1217 (2022).
Google Scholar
-
Bengio, Y., Courville, A. & Vincent, P. Representation learning: a review and new perspectives. IEEE Trans. Pattern Anal. Mach. Intell. 35, 1798–1828 (2013).
Google Scholar
-
Schmidt, J., Marques, M. R. G., Botti, S. & Marques, M. A. L. Recent advances and applications of machine learning in solid-state materials science. NPJ Comput. Mater. 5, 83 (2019).
Google Scholar
-
Sanchez-Lengeling, B. & Aspuru-Guzik, A. Inverse molecular design using machine learning: generative models for matter engineering. Science 361, 360–365 (2018).
Google Scholar
-
Mitchell, J. B. O. Machine learning methods in chemoinformatics. WIREs Comput. Mol. Sci. 4, 468–481 (2014).
Google Scholar
-
Wigh, D. S., Goodman, J. M. & Lapkin, A. A. A review of molecular representation in the age of machine learning. WIREs Comput. Mol. Sci. 12, e1603 (2022).
Google Scholar
-
Krenn, M., Häse, F., Nigam, A., Friederich, P. & Aspuru-Guzik, A. Self-referencing embedded strings (SELFIES): a 100% robust molecular string representation. Mach. Learn. Sci. Technol. 1, 045024 (2020).
Google Scholar
-
Kononova, O. et al. Text-mined dataset of inorganic materials synthesis recipes. Sci. Data 6, 203 (2019).
Google Scholar
-
Olivetti, E. A. et al. Data-driven materials research enabled by natural language processing and information extraction. Appl. Phys. Rev. 7, 041317 (2020).
Google Scholar
-
Kim, E. et al. Materials synthesis insights from scientific literature via text extraction and machine learning. Chem. Mater. 29, 9436–9444 (2017).
Google Scholar
-
Jensen, Z. et al. A machine learning approach to zeolite synthesis enabled by automatic literature data extraction. ACS Cent. Sci. 5, 892–899 (2019).
Google Scholar
-
Luo, Y. et al. MOF synthesis prediction enabled by automatic data mining and machine learning. Angew. Chem. Int. Ed. 61, e202200242 (2022).
Google Scholar
-
Zheng, Z., Zhang, O., Borgs, C., Chayes, J. T. & Yaghi, O. M. ChatGPT chemistry assistant for text mining and the prediction of MOF synthesis. J. Am. Chem. Soc. 145, 18048–18062 (2023).
Google Scholar
-
Suvarna, M., Vaucher, A. C., Mitchell, S., Laino, T. & Pérez-Ramírez, J. Language models and protocol standardization guidelines for accelerating synthesis planning in heterogeneous catalysis. Nat. Commun. 14, 7964 (2023).
Google Scholar
-
Lai, N. S. et al. Artificial intelligence (AI) workflow for catalyst design and optimization. Ind. Eng. Chem. Res. 62, 17835–17848 (2023).
Google Scholar
-
Probst, D. et al. Biocatalysed synthesis planning using data-driven learning. Nat. Commun. 13, 964 (2022).
Google Scholar
-
Moon, J. et al. Active learning guides discovery of a champion four-metal perovskite oxide for oxygen evolution electrocatalysis. Nat. Mater. 23, 108–115 (2024).
Google Scholar
-
Zhong, M. et al. Accelerated discovery of CO2 electrocatalysts using active machine learning. Nature 581, 178–183 (2020). Discovery of Cu-Al electrocatalysts, though DFT aided machine learning, to efficiently reduce CO2 to ethylene with a Faradaic efficiency of 80%.
Google Scholar
-
Torres, J. A. G. et al. A multi-objective active learning platform and web app for reaction optimization. J. Am. Chem. Soc. 144, 19999–20007 (2022).
Google Scholar
-
Greenhalgh, J. C., Fahlberg, S. A., Pfleger, B. F. & Romero, P. A. Machine learning-guided acyl-ACP reductase engineering for improved in vivo fatty alcohol production. Nat. Commun. 12, 5825 (2021).
Google Scholar
-
Tallorin, L. et al. Discovering de novo peptide substrates for enzymes using machine learning. Nat. Commun. 9, 5253 (2018).
Google Scholar
-
Schwaller, P. et al. Molecular transformer: a model for uncertainty-calibrated chemical reaction prediction. ACS Cent. Sci. 5, 1572–1583 (2019).
Google Scholar
-
Anstine, D. M. & Isayev, O. Generative models as an emerging paradigm in the chemical sciences. J. Am. Chem. Soc. 145, 8736–8750 (2023).
Google Scholar
-
Gómez-Bombarelli, R. et al. Automatic chemical design using a data-driven continuous representation of molecules. ACS Cent. Sci. 4, 268–276 (2018). A method to convert discrete representations of molecules into multidimensional continuous representations for generating compounds in silico.
Google Scholar
-
Repecka, D. et al. Expanding functional protein sequence spaces using generative adversarial networks. Nat. Mach. Intell. 3, 324–333 (2021).
Google Scholar
-
Hawkins-Hooker, A. et al. Generating functional protein variants with variational autoencoders. PLoS Comput. Biol. 17, e1008736 (2021).
Google Scholar
-
Johnson, S. R. et al. Computational scoring and experimental evaluation of enzymes generated by neural networks. Preprint at https://www.biorxiv.org/content/10.1101/2023.03.04.531015v1 (2023).
-
Schilter, O., Vaucher, A., Schwaller, P. & Laino, T. Designing catalysts with deep generative models and computational data. A case study for Suzuki cross coupling reactions. Digit. Discov. 2, 728–735 (2023).
Google Scholar
-
Kreutter, D., Schwaller, P. & Reymond, J.-L. Predicting enzymatic reactions with a molecular transformer. Chem. Sci. 12, 8648–8659 (2021).
Google Scholar
-
Popova, M., Isayev, O. & Tropsha, A. Deep reinforcement learning for de novo drug design. Sci. Adv. 4, eaap7885 (2018).
Google Scholar
-
Zhou, Z., Li, X. & Zare, R. N. Optimizing chemical reactions with deep reinforcement learning. ACS Cent. Sci. 3, 1337–1344 (2017). A fully automated deep reinforcement learning to optimize chemical reactions where the model iteratively records the results of a chemical reaction and chooses new experimental conditions to improve the reaction outcome.
Google Scholar
-
Lan, T. & An, Q. Discovering catalytic reaction networks using deep reinforcement learning from first-principles. J. Am. Chem. Soc. 143, 16804–16812 (2021).
Google Scholar
-
Song, Z. et al. Adaptive design of alloys for CO2 activation and methanation via reinforcement learning Monte Carlo tree search algorithm. J. Phys. Chem. Lett. 14, 3594–3601 (2023).
Google Scholar
-
Suvarna, M., Preikschas, P. & Pérez-Ramírez, J. Identifying descriptors for promoted rhodium-based catalysts for higher alcohol synthesis via machine learning. ACS Catal. 12, 15373–15385 (2022).
Google Scholar
-
Smith, A., Keane, A., Dumesic, J. A., Huber, G. W. & Zavala, V. M. A machine learning framework for the analysis and prediction of catalytic activity from experimental data. Appl. Catal. B Environ. 263, 118257 (2020).
Google Scholar
-
Vellayappan, K. et al. Impacts of catalyst and process parameters on Ni-catalyzed methane dry reforming via interpretable machine learning. Appl. Catal. B Environ. 330, 122593 (2023).
Google Scholar
-
Roh, J. et al. Interpretable machine learning framework for catalyst performance prediction and validation with dry reforming of methane. Appl. Catal. B Environ. 343, 123454 (2024).
Google Scholar
-
McCullough, K., Williams, T., Mingle, K., Jamshidi, P. & Lauterbach, J. High-throughput experimentation meets artificial intelligence: a new pathway to catalyst discovery. Phys. Chem. Chem. Phys. 22, 11174–11196 (2020).
Google Scholar
-
Suzuki, K. et al. Statistical analysis and discovery of heterogeneous catalysts based on machine learning from diverse published data. ChemCatChem 11, 4537–4547 (2019).
Google Scholar
-
Butler, K. T., Davies, D. W., Cartwright, H., Isayev, O. & Walsh, A. Machine learning for molecular and materials science. Nature 559, 547–555 (2018).
Google Scholar
-
Oviedo, F., Ferres, J. L., Buonassisi, T. & Butler, K. T. Interpretable and explainable machine learning for materials science and chemistry. Acc. Mater. Res. 3, 597–607 (2022).
Google Scholar
-
Esterhuizen, J. A., Goldsmith, B. R. & Linic, S. Interpretable machine learning for knowledge generation in heterogeneous catalysis. Nat. Catal. 5, 175–184 (2022).
Google Scholar
-
Wu, K. & Doyle, A. G. Parameterization of phosphine ligands demonstrates enhancement of nickel catalysis via remote steric effects. Nat. Chem. 9, 779–784 (2017).
Google Scholar
-
Weng, B. et al. Simple descriptor derived from symbolic regression accelerating the discovery of new perovskite catalysts. Nat. Commun. 11, 3513 (2020).
Google Scholar
-
Ouyang, R., Curtarolo, S., Ahmetcik, E., Scheffler, M. & Ghiringhelli, L. M. SISSO: a compressed-sensing method for identifying the best low-dimensional descriptor in an immensity of offered candidates. Phys. Rev. Mater. 2, 083802 (2018).
Google Scholar
-
Foppa, L. et al. Data-centric heterogeneous catalysis: identifying rules and materials genes of alkane selective oxidation. J. Am. Chem. Soc. 145, 3427–3442 (2023).
Google Scholar
-
Li, Z., Ma, X. & Xin, H. Feature engineering of machine-learning chemisorption models for catalyst design. Catal. Today 280, 232–238 (2017).
Google Scholar
-
Lundberg, S. M. et al. From local explanations to global understanding with explainable AI for trees. Nat. Mach. Intell. 2, 56–67 (2020).
Google Scholar
-
Timoshenko, J. et al. Linking the evolution of catalytic properties and structural changes in copper-zinc nanocatalysts using operando EXAFS and neural-networks. Chem. Sci. 11, 3727–3736 (2020).
Google Scholar
-
Wilkinson, M. D. et al. The FAIR guiding principles for scientific data management and stewardship. Sci. Data 3, 160018 (2016).
Google Scholar
-
Scheffler, M. et al. FAIR data enabling new horizons for materials research. Nature 604, 635–642 (2022).
Google Scholar
-
Abolhasani, M. & Kumacheva, E. The rise of self-driving labs in chemical and materials sciences. Nat. Synth. 2, 483–492 (2023). A review of self-driving labs through the integration of machine learning, lab automation and robotics to accelerate digital data curation and enable data-driven discoveries in chemical sciences.
Google Scholar
-
MacLeod, B. P. et al. Self-driving laboratory for accelerated discovery of thin-film materials. Sci. Adv. 6, eaaz8867 (2020).
Google Scholar
Acknowledgements
This study was created as part of NCCR Catalysis (grant no. 180544), a National Centre of Competence in Research funded by the Swiss National Science Foundation. We thank C. Ko, M. E. Usteri, T. Zou and P. Preikschas for fruitful discussions on the manuscript and help with illustrations.
Author information
Authors and Affiliations
Contributions
M.S. and J.P.-R. conceived the project. M.S. led the data collection and analysis efforts, and wrote the manuscript. J.P.-R. supervised the project, wrote the manuscript, and managed resources and funding. Both authors provided input to the manuscript and approved the final version.
Corresponding author
Ethics declarations
Competing interests
The authors declare no competing interests.
Additional information
Publisher’s note Springer Nature remains neutral with regard to jurisdictional claims in published maps and institutional affiliations.
Supplementary information
Supplementary Information
Supplementary Notes 1 and 2, Tables 1–3 and Fig. 1.
Source data
41929_2024_1150_MOESM2_ESM.xlsx
Source Data Fig. 1a Raw data for creating timeline plot of research trends in data-driven catalysis. Source Data Fig. 1c Raw data for creating alluvial plots linking catalysis subdisciplines with different tasks and modes. Source Data Fig. 2a Raw data for network plot mapping relations of deductive tasks based on catalysis type. Source Data Fig. 2b Raw data for network plot mapping relations of deductive tasks based on driving. Source Data Fig. 4 Raw data for establishing structure-property-performance relations through ML.
Rights and permissions
Springer Nature or its licensor (e.g. a society or other partner) holds exclusive rights to this article under a publishing agreement with the author(s) or other rightsholder(s); author self-archiving of the accepted manuscript version of this article is solely governed by the terms of such publishing agreement and applicable law.
Reprints and permissions
About this article
Cite this article
Suvarna, M., Pérez-Ramírez, J. Embracing data science in catalysis research.
Nat Catal (2024). https://doi.org/10.1038/s41929-024-01150-3
-
Received: 08 December 2023
-
Accepted: 29 February 2024
-
Published: 23 April 2024
-
DOI: https://doi.org/10.1038/s41929-024-01150-3