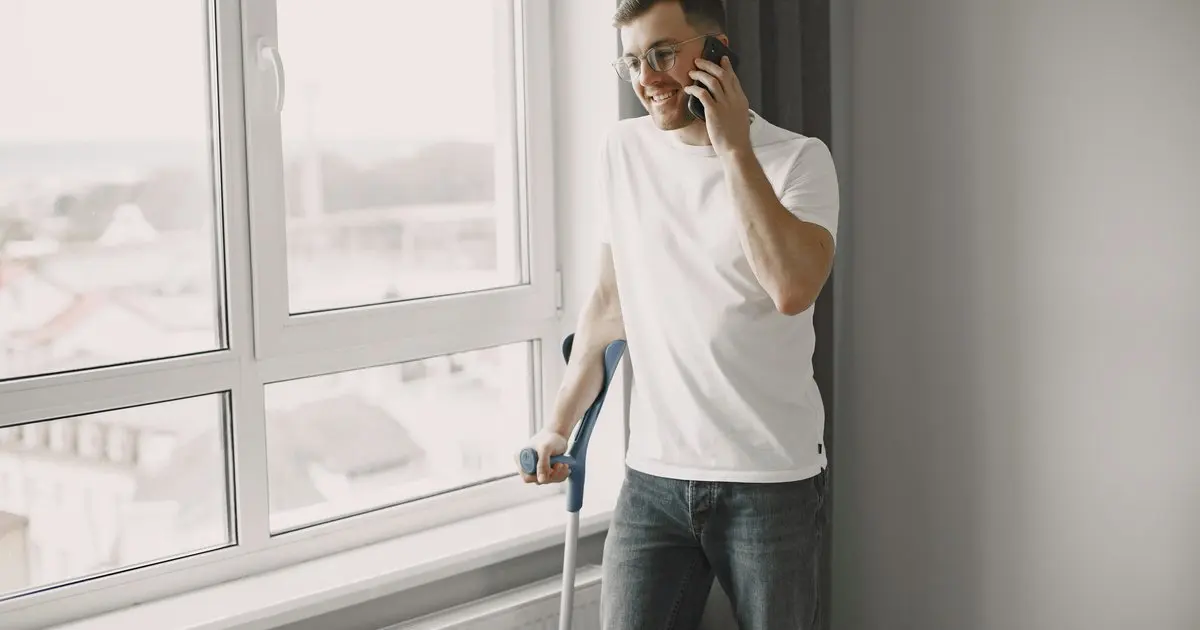
If a clinician you care about counts on AI to help make medical decisions, remind them: Tort law principles hold that doing so means risking liability should a patient sue over harm done.
The reminder comes from researchers in the anesthesiology department at Rutgers New Jersey Medical School. Saad Ali, MD, and co-authors had their commentary published in Biomedical Instrumentation & Technology, a peer-reviewed journal of the Association for the Advancement of Medical Instrumentation (AAMI).
Largely focusing on the “black box” problem and how it exposes clinicians to liability, Ali and colleagues cite last year’s draft guidance from the FDA discussing the information that manufacturers of AI-equipped medical devices should include in their product literature. [1]
Stating that the FDA document is “only a first step and doesn’t address the thorny question of clinician liability,” Ali and colleagues offer three key points that AI-embracing clinicians ought to keep in mind.
1. With the help of machine learning algorithms, AI-equipped devices are likely to become more accurate over time, producing fewer mistakes and lowering false-positive rates. However,
‘Until the use of AI/ML for treatment recommendations by clinicians gets recognized as the standard of care, the best option for clinicians to minimize the risk of medical malpractice liability is to use it as a confirmatory tool to assist with decision-making.’
2. Financial compensation commonly is used by U.S. vaccine manufacturers to pay those who have adverse reactions after receiving vaccines. Manufacturers of AI/ML-enabled devices may be able to use a similar approach to incentivize the use of their products. However,
‘Such an approach may give less incentive to manufacturers to ensure their product’s reliability and safety, and would have little to no beneficial effect on clinicians’ wariness of the products.’
3. Because the training datasets are not unlimited, it is understandable that all AI/ML-enabled medical devices will have a degree of bias. However,
‘Not being transparent about certain limitations can result in a loss of trust. Improvements to product labeling should be made to clearly delineate the training dataset used and provide assessment of potential biases.’
Extending the latter point, the authors add that transparency “seems to be the key to the growth of AI in healthcare, fostering trust among software developers, clinicians and patients.”
Ali and co-authors encourage providers and hospitals to test the outputs of AI-equipped medical devices for themselves before acquiring such devices. They also urge end-users to fully inform patients on potential risks and expected benefits when obtaining patient consent.
New AI-enabled devices continue entering the market at a brisk pace, the authors note. They write:
‘Clinicians who encounter these devices need to be certain that device performance can match the standard of care. Such assurance is needed to prevent fear of malpractice liability from curtailing clinician use of these innovative devices.’
The full article is posted here (behind paywall).
Reference:
- FDA, “Marketing Submission Recommendations for a Predetermined Change Control Plan for Artificial Intelligence/Machine Learning (AI/ML)-Enabled Device Software Functions”: Draft Guidance for Industry and FDA Staff (April 3, 2023)