Among the main advancements in AI, seven areas stand out for their potential to revolutionize different sectors: neuromorphic computing, quantum computing for AI, Explainable AI (XAI), AI-augmented design and Creativity, Autonomous Vehicles and Robotics, AI in Cybersecurity and AI for Environmental Sustainability. These technologies promise to enhance current AI capabilities and offer new paradigms in computing and human-machine interaction.
Neuromorphic Computing: Mimicking the Human Brain
Neuromorphic computing represents a radical departure from traditional computing architectures. Taking inspiration from the structure & function of the human brain, neuromorphic chips are designed to emulate how neurons and synapses communicate. This approach offers advantages over conventional hardware, particularly speed and energy efficiency.
The human brain is incredibly efficient and capable of performing complex tasks like image and speech recognition with much less power than current computers require. Neuromorphic chips replicate this efficiency by using systems of artificial neurons and synapses that can operate in parallel and dynamically change their interconnections. This allows them to process information more efficiently and make decisions faster.
Neuromorphic computing has vast applications. In robotics, these chips could enable more responsive and adaptive robots that better mimic natural movements and reactions. AI-driven sensors and devices could significantly reduce power consumption while increasing processing capabilities, ideal for mobile and edge computing devices where power and speed are critical.
Quantum Computing for AI: Unlocking New Possibilities
Quantum computing stands to redefine the limits of data processing. By leveraging the principles of quantum mechanics, quantum computers can process complex problems at speeds unattainable by classical computers. For AI, the implications are profound, especially in fields that require processing vast amounts of data, such as drug discovery and materials science.
Quantum algorithms could dramatically speed up the training of machine learning models and handle problems involving complex systems and large datasets—tasks currently challenging or infeasible for traditional computers. For example, in drug discovery, quantum computers could model molecular interactions at an unprecedented scale and speed, potentially reducing the time and cost of developing new medications.
However, quantum computing for AI is still in its early stages, and several technical challenges remain, including error rates and qubit coherence times. Despite these hurdles, ongoing advancements in quantum technologies are promising, making it a crucial area to watch for future AI applications.
Explainable AI (XAI): Enhancing Trust and Transparency
As AI systems become more advanced, understanding how they make decisions is crucial, especially in sectors like healthcare, finance, and autonomous driving, where decisions have significant consequences. XAI aims to address this by making AI decisions more transparent & understandable to humans.
Techniques like Local Interpretable Model-agnostic Explanations (LIME) and SHapley Additive exPlanations (SHAP) are at the forefront of XAI. These methods help illuminate the reasoning behind AI decisions by explaining the contribution of each input feature to the final decision. For instance, in a medical diagnosis AI, LIME could reveal which symptoms and patient data were most influential in diagnosing a disease, providing insights into the model’s decision-making process.
XAI not only helps validate and improve AI models but also builds trust among users by making AI systems more relatable and less like a “black box.” As AI becomes more prevalent, the demand for transparency will likely increase, making XAI an essential component of future AI systems.
AI-Augmented Design and Creativity
The AI-augmented design represents a synergy between human creativity and computational power, enabling new levels of innovation within creative industries such as architecture, fashion, and graphic design. This technology leverages AI’s capacity to analyze and learn from vast datasets, allowing it to identify patterns and suggest design alternatives that might not be immediately obvious to human designers.
One notable application of AI in this field is generative design, where algorithms can create many design solutions to a single problem, optimizing for materials usage, durability, and aesthetic appeal based on predefined criteria. For instance, Autodesk uses AI in its software to help architects and designers explore different configurations efficiently. AI tools like Adobe’s Sensei also harness machine learning to assist with image editing tasks by automatically tagging photos, enhancing compositions, and suggesting edits.
These advancements enhance productivity and push the boundaries of traditional design, enabling professionals to explore complex and innovative solutions that were previously unattainable.
Autonomous Vehicles and Robotics
The realm of autonomous vehicles and robotics is one of AI’s most visually recognizable applications. This area extends beyond self-driving cars to include drones, autonomous surgical robots, and robots used in logistics and manufacturing. The core AI technologies driving these systems include computer vision, sensor fusion, and DL, which help machines perceive their environment, make informed decisions, and navigate obstacles.
In the automotive industry, companies like Tesla and Waymo are pioneering the use of AI for autonomous driving. These vehicles rely on sophisticated sensors, cameras, and advanced machine learning algorithms to interpret live road data, make split-second driving decisions, and learn from new driving scenarios. The broader implication is the potential reduction in road accidents caused by human error, enhanced mobility for the elderly and disabled, and a significant transformation in urban planning and traffic management.
In robotics, AI enables more precise and safer surgical procedures through robots that can assist or even autonomously perform surgeries under the supervision of human medical staff. For instance, the da Vinci Surgical System provides a magnified 3D high-definition vision system & tiny wristed instruments that bend & rotate far more than the human hand, enhancing surgical precision and control.
AI in Cybersecurity
As digital infrastructures become more complex & integral to operations across industries, the importance of robust cybersecurity measures escalates. AI in cybersecurity is a fast-evolving field aiming to defend against and mitigate the effects of increasingly sophisticated and frequent cyber attacks. AI-driven cybersecurity systems can monitor network traffic for unusual patterns, detect anomalies that signify potential threats, and automatically respond to incidents in real time.
ML models can be trained on cybersecurity, network, and even physical security information data to identify potential threats quickly. For example, Darktrace uses AI algorithms that detect and respond to cyber threats across diverse digital environments. This AI capability is crucial for preemptive threat detection and response, significantly reducing the time between threat detection and mitigation compared to traditional methods.
AI for Environmental Sustainability
AI’s potential to aid in environmental sustainability is vast and varied. AI can optimize energy use in large-scale systems through data collection and deep learning, predict weather patterns more accurately, and even monitor wildlife and ecosystems with unprecedented precision. In agriculture, AI-driven technologies can predict crop yields, monitor soil conditions, and optimize resource use (water, fertilizers) to enhance productivity while minimizing environmental impact.
On a larger scale, AI is instrumental in climate modeling and simulation to help scientists better understand climate change and its impacts. Google’s AI for Social Good program leverages machine learning to predict the locations of solar panels to assess solar potential and optimize energy use. Furthermore, AI is used in smart grids to balance electricity supply and demand, ensuring more efficient energy use across urban landscapes.
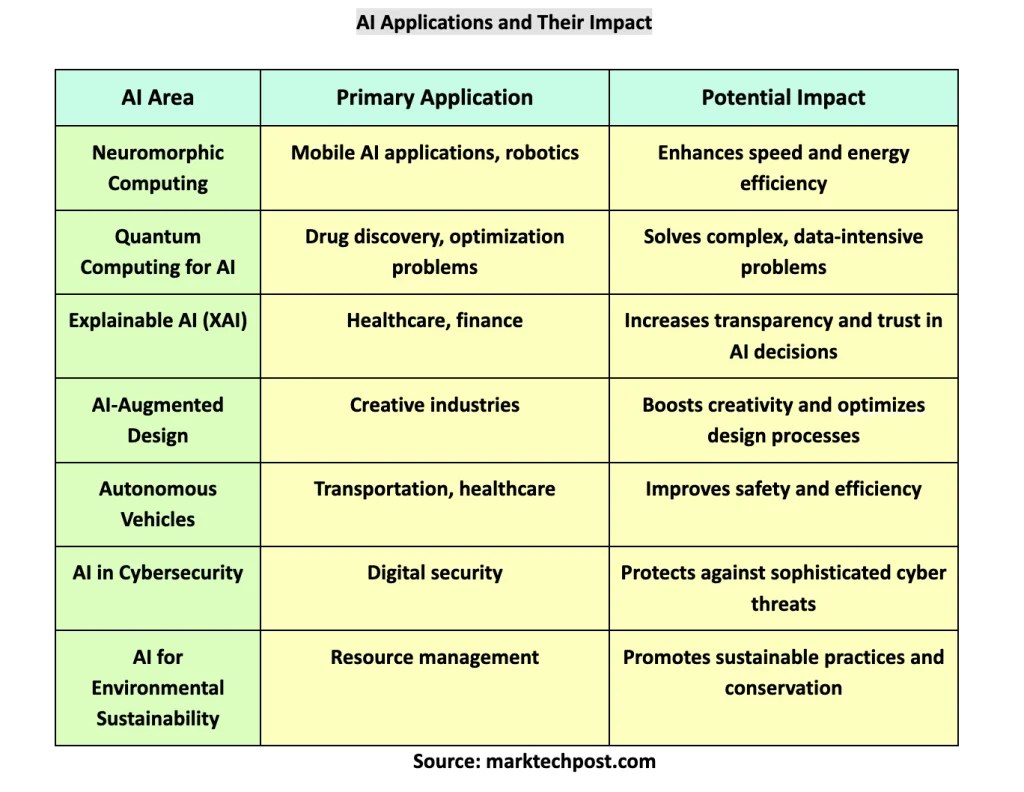
Sources
Sana Hassan, a consulting intern at Marktechpost and dual-degree student at IIT Madras, is passionate about applying technology and AI to address real-world challenges. With a keen interest in solving practical problems, he brings a fresh perspective to the intersection of AI and real-life solutions.