Abstract
To study stratification among scientists, we reconstruct the career-long trajectories of 8.2 million scientists worldwide using 12 bibliometric measures of productivity, geographical mobility, collaboration, and research impact. While most previous studies examined these variables in isolation, we study their relationships using Multiple Correspondence and Cluster Analysis. We group authors according to their bibliometric performance and academic age across six macro fields of science, and analyze co-authorship networks and detect collaboration communities of different sizes. We found a stratified structure in terms of academic age and bibliometric classes, with a small top class and large middle and bottom classes in all collaboration communities. Results are robust to community detection algorithms used and do not depend on authors’ gender. These results imply that increased productivity, impact, and collaboration are driven by a relatively small group that accounts for a large share of academic outputs, i.e., the top class. Mobility indicators are the only exception with bottom classes contributing similar or larger shares. We also show that those at the top succeed by collaborating with various authors from other classes and age groups. Nevertheless, they are benefiting disproportionately from these collaborations which may have implications for persisting stratification in academia.
Similar content being viewed by others
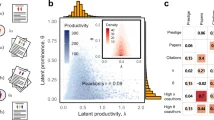
Untangling the network effects of productivity and prominence among scientists
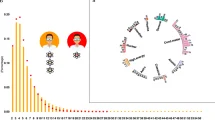
Knowledge and social relatedness shape research portfolio diversification
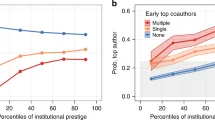
Early coauthorship with top scientists predicts success in academic careers
Introduction
Science is a social enterprise with inequality among its agents (Chompalov et al. 2002; Kozlowski et al. 2022; Shrum et al. 2001, 2007). Factors underpinning social stratification include differences within and between countries in institutional capacity and resources available for research (Castro Torres and Alburez-Gutierrez 2022), and inequalities among scholars according to gender (Akbaritabar and Squazzoni, 2020; Larivière et al. 2013), race and ethnicity (Kozlowski et al. 2022), migration status (Sanliturk et al. 2023; X. Zhao et al. 2023), and social class differences in opportunities to access higher education and research (Bourdieu and Passeron, 1979; Burris 2004; Clauset et al. 2015). Such overrepresentation of specific demographics in privileged positions within scientific systems are indicators of stratification (Alper 1993; Hofstra et al. 2022; Marini and Meschitti 2018). Differences in scholars’ strategies in the search for prestige can also influence inequalities in science (Leahey and Cain 2013). The durability of stratification depends, among other things, on taken-for-granted ideas about the necessity and benefits of hierarchical order—for example in terms of seniority, impact, or recognition. These taken-for-granted ideas also exist in the broader sphere of social and economic affairs. The belief that a market-oriented organization of the economy without state intervention is optimal legitimizes the existence of socioeconomic inequalities within and between societies (Mazzucato 2018; Pikkety 2019), which in turn contributes to sustaining social stratification among nations and individuals (Therborn 2013). In all likelihood, Science as a subfield of these broader social and economic relations, works analogously. Scientific research also is an inherently competitive endeavor, in which individual-based reputational incentives can undermine the motivation to collaborate (Müller 2012; Penman and Goldson 2015; van den Besselaar et al. 2012).
Inequalities in science are often justified by beliefs regarding the meritocratic nature of science and of academic success and the inherent value of truth. Several indicators, such as the number of publications and citations, help fuel these beliefs. While those are increasingly challenged by scholars from different perspectives (Sugimoto and Larivière 2018; Wilsdon et al. 2015), bibliometric measures remain used extensively. Moreover, in the context of assesment, those are mostly used in isolation and their interrelations are ignored.
This paper provides an assessment of stratification across fields of science based on a multivariate analysis of large-scale bibliometric information from 1996 to 2021 and highlights the interrelationships between bibliometric indicators. We argue that these interrelations provide a structural measure of inequalities in the scientific community beyond single variables gaps such as authors’ differences in the number of publications or citations. Because measuring inequalities is only a first step in understanding their potential underlying mechanisms, we make a dataset with country-level measures of scientific stratification publicly available for future research (Akbaritabar and Castro Torres 2024).
Existing inequalities in science
Data on scholars’ collaboration, geographical mobility, productivity, and citations suggest that academia is growing in absolute numbers and expanding geographically. There are more coauthored papers in recent years compared to earlier decades (Abramo et al. 2009; Melkers and Kiopa 2010; Wuchty et al. 2007), and more scholars experienced geographical mobility today than in the past (Sanliturk et al. 2023; Sugimoto et al. 2017; X. Zhao et al. 2023). Likewise, studies have shown that the number of scholarly publications has increased and that digitization has made searching and citing easier (Kozlowski et al. 2024; Lozano et al. 2012). Greater productivity and increased citation capacities enhanced academic works’ visibility and potential impact (Liu et al. 2018; Sinatra et al. 2016). Some of these analyses have pointed out that these rising trends are accompanied by an increased concentration of academic-success indicators among relatively few scholars (Ioannidis et al. 2018) or that increased collaboration and rate of productivity per individual has not increased (Fanelli and Larivière 2016).
According to the 28+ million publications indexed by Scopus (1996–2021), 33% of scholars have contributed to only one research paper throughout their careers, and the median number of authors per paper is two. This suggests that a few highly productive researchers may drive rising trends in scholars’ productivity reported in the literature (Fox and Nikivincze 2021; Ioannidis et al. 2018). Likewise, according to Scopus data, approximately 27.2% of the publications have only one author, and more than 75% are authored by scholars from one country, i.e., strictly national publications. Likewise, most authors (87.5%) have been affiliated with a single country throughout their careers, and 73.5% to a single sub-national region, that therefore experienced little geographical mobility (Akbaritabar et al. 2023; Sanliturk et al. 2023; X. Zhao et al. 2023). Similarly, 36.8% of authors have been actively publishing over only one year. These low shares call for a global investigation into whether claims of increased mobility, collaboration, productivity and impact are widespread phenomena, or remain concentrated among a small group of scholars. Bibliometric research has also shown that academic citations display a skewed distribution where only a very small share of publications, journals, and authors receive disproportionately high citations which has increased recently (Nielsen and Andersen 2021). These studies suggest that bibliometric indicators for academic-success are concentrated on a few countries, institutions, and authors.
In light of this evidence, the growth of scientific activities and its geographical expansion require a critical examination of their consequences for inequalities and global stratification. In fact, we know less about the interrelatedness of these trends than we know about them in isolation. Therefore, understanding inequalities in science requires a multidimensional approach. There might be positive or negative correlations, feedback effects, and synergistic connections among bibliometric measures of academic success including individual and collaborative productivity, national and international mobility, and research visibility as measured by citations.
For instance, more collaborations could lead to more citations, which in turn may translate into greater productivity and more opportunities for geographical mobility; greater mobility may expand scholars’ networks, enhancing their potential pool of collaborators. Conversely, mobility and changes of affiliation could also reflect negative conditions such as precarious research contracts and lack of opportunities for a life-long or long-term career. Further, multiple instances of mobility can destabilize one’s network of collaborations (Z. Zhao et al. 2020). The absence or lack of success in any of these realms may negatively affect performance in the others, as well as positive outcomes in any of these realms may boost success in others i.e., Matthew effect (Merton 1968). Social stratification in science will likely emerge from the confluence of successful (and unsuccessful) academic paths in these interrelated realms: productivity, collaboration, geographical mobility, and citations.
Materials and methods
We use 28.5 million articles and review publications indexed in Elsevier’s Scopus between 1996 and 2021. A proper disambiguation of author names is crucial for analysis such as ours that reconstructs publication trajectories over one’s career. Scopus identification numbers (Baas et al. 2020) are one of the few reliable options available (Aman 2018) and were used here to assign papers to authors and to identify groups of authors who publish together in the global network of co-authorship. We limit these publications to all of those written by the authors having identification numbers in Scopus and declared as “disambiguated” by Elsevier which has a 98.3% precision and a 90.6% recall (Baas et al. 2020). In addition to the evaluations by Elsevier (Baas et al. 2020), others have previously shown that Scopus author identification numbers are reliable in comparison to other sources (Aman 2018). We further disambiguate the academic affiliation of authors in this set of publications using the Research Organization Registry’s (ROR) Application Programming Interface (API) and geocode organizations’ addresses to subnational units (Akbaritabar 2021). This reduces our coverage of publications down from 33 to 28.5 million publications by 8.2 million disambiguated authors.
Author level variables and career-long measurement
To categorize scientists into specific groups and identify stratification processes, we reviewed the literature and selected the 12 most-widely used academic performance indicators. The list of indicators is as comprehensive as possible given existing data and it avoids, as much as possible, redundancy across measures. Together, these indicators provide a robust measure of individual-level academic performance. These are the most widely used measures in previous studies which have implemented them mostly in isolation without considering their interrelation.
While our analytical sample includes 8.2 million authors with at least one publication in the Scopus database, we excluded 41,278 authors (0.5%) because their publications have missing metadata. The list below provides each bibliometric indicator’s name and category: productivity, collaboration, mobility, and visibility. These indicators are computed at the author level and comprise all individual publications indexed by Scopus between 1996 and 2021; covering authors’ careers from one up to 25 years.
-
1.
The number of coauthored papers, Num. coauthored pubs. (collaboration/internationalization)
-
2.
The average number of coauthors per paper in career, Avg. collaborations (as a measure for collaboration/internationalization)
-
3.
The number of internationally coauthored publications, Num. intl. pubs (collaboration/internationalization)
-
4.
The number of nationally coauthored publications, Num. national pubs. (collaboration/internationalization)
-
5.
The number of international changes in academic affiliation, Num. intl. moves (mobility)
-
6.
The number of national changes in academic affiliation, Num nat. moves (mobility)
-
7.
The number of affiliated organizations, Num. organizations (mobility)
-
8.
The total number of citations, Total citations (impact/visibility)
-
9.
The average number of citations per paper in career, Avg. citations (impact/visibility)
-
10.
The fractional count of publications, Fractional pubs. (productivity)
-
11.
The number of publications, Total publications (productivity)
-
12.
The number of first-author publications, First author publications (productivity)
To favor comparability among scholars, we standardize most indicators by authors’ academic age (age hereafter), measured as the years since their first publication in our database. However, the average number of coauthors per paper and the average number of citations per paper are not normalized by career age but, rather, the number of papers an author publishes throughout their career. Our goal with these two average measures, used in combination with the other 9 variables, is to further identify the effect of outliers in one’s career, such as highly cited papers or highly collaborative ones. To account for differences across disciplines in publication practices, we categorized researchers separately for each of the six macro fields of science according to the OECD classification by using the field where highest share of their publications appeared: Agricultural Sciences, Natural Sciences, Humanities, Medical and Health Sciences, Engineering and Technology, and Social Sciences.
By default, scholars with only one publication display lower variability across these 12 indicators compared to other groups. Because they published only one article, other measures such as national and international mobility, and the number of organizations are bound to zero and one, respectively. The number of citations, co-authors, and fractional count of papers are also limited to the information of the only published paper. Similarly, scholars who have publications in only one year in our data have lower bounds in these indicators. This limited heterogeneity reduces the influence of this group in our analysis despite their relatively high shares, ranging from 31% in the Natural Sciences to 47% in Engineering and Technology. In the Supplementary information (SI), we show separate figures for scholars with only one year of publication activity (Fig. S3 presents the share of one-year old authors). Instead of excluding this group from the analysis, as the usual practice in the literature, we decided for categorizing them under a specific age group to study the specificities of this understudied group.
Bibliometric variables are extremely skewed and the usual practice in the literature is to exclude outliers. As an example, publications with the highest number of authors are sometimes excluded (Nogrady 2023; Singh Chawla 2019). Here, to better capture non-linear relations across these indicators, and to reduce the influence of outliers, while keeping them in the analysis, all the indicators were categorized into the maximum possible number of categories ensuring relative frequencies of at least 2% in all categories. This categorization method maintains the essential characteristics of the continuous variables while mitigating the impact of outliers on correlation measures. This is achieved by grouping outliers into the lower- and bottom-end categories. This approach to variable coding is beneficial in the context of highly-skewed variables with heavy tails (see Fig. S2), as it allows us to: (i) include extreme values in the analysis, (ii) capture potential non-linear relationships among variables, (iii) preserve the distributional characteristics of each indicator, and (iv) avoids potential biases in correlational analyses due to outlier observations. The resulting number of categories across variables ranges from three for the number of international changes in academic affiliation in Agricultural Sciences (i.e., 95% of authors do not experience international mobility) to ten for the total number of citations in the Natural Sciences and Medical and Health Sciences (i.e., the 10th, 20th, …, 100th percentiles).
A multidimensional measure of social stratification within scientific communities
We run a Multiple Correspondence Analysis (Le Roux and Rouanet 2004) on the 12 categorized indicators for each macro field of science. Based on the Singular Value Decomposition of the matrix representing the 12 indicators, MCA yields individual-level numerical variables termed factorial axes. These factorial axes summarize the 12 indicators according to their multivariate correlations and relative importance. Due to the high number of categories of the 12 variables, our field-specific MCAs yield more than 50 factorial axes, most of which have very little informational value. We focus on the first three axes because their associated eigenvalues are significantly larger than the others, and therefore capture the most salient differences among scholars’ bibliometric performances (see Fig. S4).
Despite our age standardization, the first factorial axis of all MCAs came out as strongly correlated with scholars’ age and indicators of productivity, visibility, and collaboration. This result is partially due to the specificities of the one-year old group (e.g., reduced heterogeneity and very distinct profiles compared to older scholars), but also underscores the cumulative aspect of academic achievements with age. There is a clear age gradient in the first factorial axis for all age groups, not only the one year old, indicating that the incremental improvements in academic productivity, visibility, and collaboration grow as individuals progress in seniority.
Considering the significance of age in our study, and with the aim of improving comparability, we performed cluster analyses independently for six age groups: One-year-old, two to five, six to nine, 10 to 14, 15 to 20, and 21 to 25. Hence, we conducted 36 hierarchical clustering analyses (six macro fields of science multiplied by six age groups) based on the Ward method followed by a cluster consolidation via the K-means algorithms. Neighboring solutions with five, six, seven, and eight clusters were assessed using the ratio of between to total variance. These assessments led us to focus on a six-cluster solution (see SI). We term these clustering bibliometric classes and we use positional words to label them: bottom, low, mid-low, mid-high, high, and top. The marginal distribution of scholars across bibliometric classes measures the social stratification of science in each field. The differences between bibliometric classes in academic performance indicators capture the extent of hierarchies. We visualize these differences using factorial axes where distance implies differences and proximity implies similarity.
Network analysis of intra- and inter-class collaboration
To investigate whether members of identified bibliometric classes collaborate “within” their own class or with members of other classes and age groups, we construct global bipartite networks of co-authorship among the 8.2 million authors, identify its largest connected (giant) component and detect communities of densely collaborating scientists. In other words, we group authors into scientific communities according to their degrees of proximity in collaboration networks. Scholars that coauthor papers are maximally close, whereas authors without any coauthor in common are maximal distal. To identify communities, we use the Constant Potts Model (CPM) (Reichardt and Bornholdt 2004) and its extension to bipartite networks (Akbaritabar 2021; Akbaritabar and Barbato 2021; Traag et al. 2011) with a varying range of 18 resolution parameters. For robustness checks, we use three additional community detection algorithms from NetworKit (default algorithm, parallel Louvain, and parallel Label Propagation) and cross-check the identified communities. Additionally, we projected the bipartite network to a one-mode one, despite criticisms on such a projection and information loss it brings (Akbaritabar 2021; Akbaritabar and Barbato 2021), to use Leiden algorithm and results were robust and our storyline did not change (see SI).
We examine authors’ distribution across bibliometric classes within these identified scientific communities. For this analysis, we pooled all academic-age groups and compared the distribution of authors within each scientific community according to their academic age and bibliometric class. A side-by-side comparison of the bibliometric classes and academic-age distributions within scientific communities and entropy measures for these two distributions allows for assessing the nature and strength of stratification across scientific communities. Figure S1 presents the steps described above.
Results
We represent social stratification in science and bibliometric classes using the first two MCA axes. We interpret these axes according to the variables’ percentage contribution to the variance, as displayed in Fig. 1. A vertical line is drawn at the mean percentage contribution, i.e., 8.3%. Markers at the right of this vertical line indicate variables with above-average contributions to the axes’ variance. Different markers are used for each macro field of science.
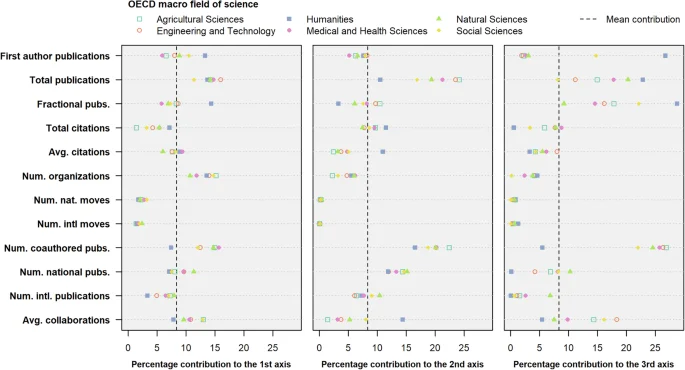
The panels correspond to the first three factorial axes. The X-axis shows the variables’ contribution to the axes’ inertia. Markers’ colors and shapes distinguish the OECD macro field of sciences. The vertical dashed line indicates the average percentage point contribution (100%/12 = 8%).
The variables that contribute the most to the first factorial axis are total publications, number of organizations, number of coauthored publications, average collaborations, and first-authored publications. Field differences are evident in the contribution of these variables to the first axis. For instance, in the Humanities (filled square), “Num. coauthored pubs.” and “Avg. collaborations” have a much lower contribution than “First author publications”, which can be explained by the fact that they are generally a non-collaborative field. The reverse is observed for the Social Sciences (filled diamond), where coauthored papers have a higher contribution to the first axis than first-author publications.
The first factorial axis correlates positively with academic age. This is a somewhat unexpected result given that we use indicators standardized by age. In all macro fields of science, there is an age-gradient in the first axis, and the mean coordinate of first and last age-groups are more than one standard deviation apart. There is no age gradient in any of the other axes. Therefore, when considering total publications, the number of organizations, coauthored publications, average collaborations, and first-author publications per year of age, senior scholars surpass their junior counterparts. In other words, the positive correlation between academic age and the first axis suggests that academic success accumulates with age, leading to progressively greater marginal gains. Thus, we labeled the first MCA axis as “Academic age, number of organizations, and individual productivity” despite the fact that age has not been used as an input in the MCA. A large coordinate in this axis represents older academic age, a relatively high number of organizations, and an above-average number of publications, as first-author in collaborations.
The variables that contribute the most to the second factorial axis are total, fractional (for some fields), and coauthored publications. In addition, the total number of citations and the number of national publications also contribute significantly to the second axis. We labeled the second axis as: “Total productivity, visibility, and collaborations.” Finally, the variables that contribute the most to the third factorial axis are first-authored publications, total publications, fractional publications, number of coauthored publications, and average collaborations. There is a large variety among fields of science in variables’ contributions to the third axis, yet, productivity and collaboration measures excel for their large contributions, particularly for the Humanities.
Hence, the organization of scholars according to their bibliometric indicators revolves around two main dimensions: “academic age, number of organizations, and individual productivity” on the one side, and “total productivity, visibility, and collaborations,” on the other. Scholars’ productivity is distinctly comprised in both dimensions. In the first dimension, productivity goes along with age and first-author publication. In the second dimension, productivity is less dependent on age and is associated with collaborations and citations. Interestingly, none of the mobility measures contribute significantly to the first three MCA axes that could stem from the very small share of mobile authors (about 8% in international and 12% in national moves).
Figure 2 displays authors’ distribution by fields of science according to the above-described main dimensions and the bibliometric classes detected via cluster analysis. Existing differences in academic practices (e.g., publication, collaboration, mobility, and citation) across fields of science require axes’ scales be free and prevent scaled comparisons across them. Authors with identical bibliometric measures are grouped and represented as circles to reduce overplotting. Circles’ size is proportional to the number of authors with identical bibliometric profiles. Although we conduct the analysis for all ages and find similar results across those (gray background circles), Fig. 2 highlights the bibliometric stratification of 15 to 20 year old scholars. The top group comprises the most successful authors based on combining our 12 bibliometric measures. The bottom-left includes those at the bottom of academic achievement indicators’ distributions.
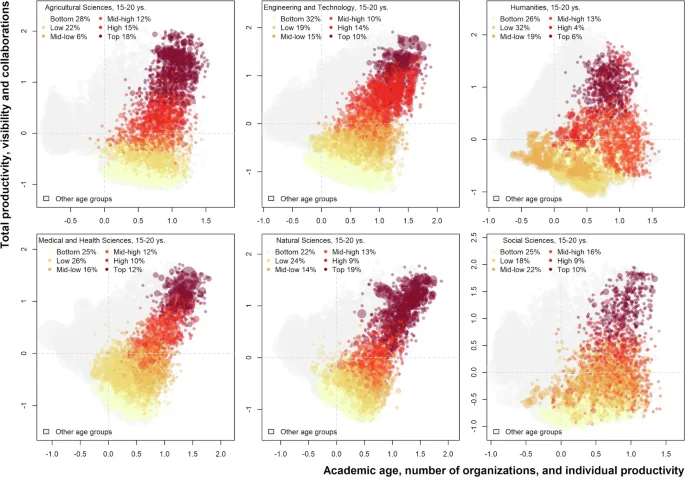
Multiple Correspondence Analysis (MCA) results using the 12 most widely used bibliometric variables allowed identifying six classes of scientists from Bottom, Low, Middle low, Middle high, High, to Top. In all six fields of science and five-year career groups from a minimum of 1 to a maximum of 25 years of publication career indexed in Scopus, we see the same stratified structure appearing. A minority of the top class is identified which consists of less or about 10% (in most fields) of the most successful scientists indicated with dark red colors in the figure. See figures in Supplementary Information (SI) for other academic age groups and disaggregated analysis based on gender of authors to males and females which did not show a change in the reported trends.
The clustering of authors according to their academic achievement is a measure of existing inequalities in these fields of science. Despite disciplinary differences in size and scientific practices, the commonalities in the stratification of authors are notable. In all six fields of science, the top class comprises a minority whose share ranges from a minimum of 6% in Humanities to a maximum of 19% in Natural Sciences. The bottom class ranges from a minimum of 22% in Natural Sciences to a maximum of 32% in Engineering and Technology. On the contrary, the middle- and bottom classes unanimously position towards the bottom left quadrant, meaning they are always worse off in terms of 12 bibliometric measures investigated here.
This structure replicates among other academic-age groups (refer to figures in SI) with the exception of the one-year old. Scholars’ bibliometric stratification is most pronounced within the oldest age group (i.e., 21-to-25 years old) with bibliometric classes comprising more similar shares compared to bibliometric classes among 15-to-20-year-old scholars (refer to Fig. S10). This greater uniformity in the size of bibliometric classes indicates a possible cumulative effect of bibliometric performance over time. The 21-to-25 years old group represents scholars who have been actively publishing in Scopus-indexed journals for over 20 years. Thus, they are likely committed to the principles of scientific production, or at least, to the norms governing publication systems, including their penalties and rewards.
In contrast, a strong pyramidal structure (i.e., very small shares at the top classes) appears among scholars with shorter durations in the publishing system, such as those aged one year or two to five years. This strong pyramidal pattern may stem from their limited exposure to publication systems, hindering the establishment of distinct patterns. Consequently, the correlations, feedback mechanisms, and synergistic effects among bibliometric indicators are yet to manifest fully among these younger scholars.
This multivariate approach to academic performance and bibliometric classes challenges the so-called 20/80 rule, showing that it does not apply to all cases. To illustrate this point, Fig. 3 compares the bottom and top classes’ contribution to the total output in 10 metrics among 15 to 20-year-old scholars. The vertical axes represent the outcome share coming from each class, and the numbers at the top indicate class’ sizes. For example, the bottom class in Agricultural Sciences comprises 28% of the authors in our sample. These scholars contribute less than 5% of the total international publications. The scholars who are in the top class, 18%, instead, contribute more than 55%.
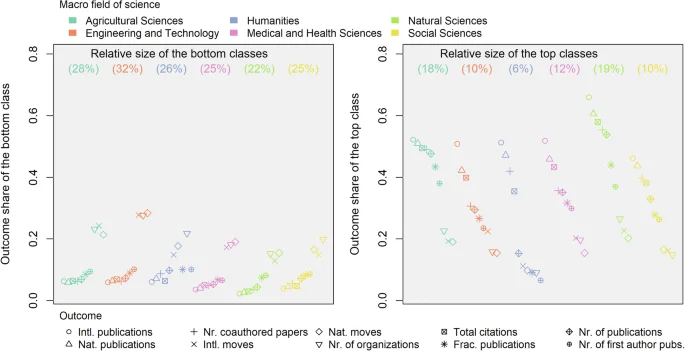
A multivariate approach to academic performance shows that the assumption that 80% of outputs are produced by the top 20% contributors (the so-called 20/80 rule) does not hold for bibliometric variables. The top classes in all macro fields of science account for less than 80% of the total outputs across 10 indicators. Bottom classes’ contributions are meager highlighting the extreme heterogeneity across academic careers. Both, top and bottom classes display similar contributions to geographical and institutional mobility.
Figure 3 shows that bottom classes comprise one fourth of authors in all macro fields and contribute less than 5% of the total in seven out of 10 indicators. The three exceptions are the number of organizations, and national and international moves which are measures of mobility. In fact, the share contribution of the bottom classes to these three outcomes is similar to that of the top class, except in the Humanities where bottom class scholars contribute much larger shares. These similarities indicate that mobility, both geographical and institutional, is associated with both success and failure in bibliometric performance. This is coherent with the literature highlighting positive and negative implications for mobility such as higher impact and less stable network of collaborations (Sugimoto et al. 2017; Z. Zhao et al. 2020).
In contrast, the top classes, between 6% and 19% of authors, lead the contributions to international publications in all macro fields of science. However, even in the Natural Sciences, where their share contribution is the highest, they are far from contributing 80%, meaning that the 20/80 rule does not hold under a multivariate approach to academic performance. The top classes also excel by their contribution to national publications, Coauthored papers, and total citations. Share contributions to other outcomes by the top class are generally lower, particularly for outcomes that imply some mobility or change of institutional affiliation as highlighted above. Figure S5 in the SI displays the shared contribution of all classes for the 10 outcomes.
Another aspect of these bibliometric classes is whether authors from different classes belong to the same research communities identified in the co-authorship network. Figure 4 shows the distribution of authors according to bibliometric classes (Panel A) and academic age groups (Panel B) across 19,970 scientific communities with at least 20 authors (99% of authors and 42.7% of communities). These communities are identified from the collaboration networks measured through co-authorship of publications (see more information in methods section). In panels A and B, scientific communities are represented by horizontal lines sorted from largest (on the top) to smallest and the deciles of the community-size distribution are indicated in the vertical axis. According to these panels, bibliometric-based stratification is similar to stratification based on age, suggesting that collaboration networks comprise authors of all ages and from all bibliometric classes. This similarity of bibliometric-class and academic age compositions is confirmed by Panel C, which displays the empirical density of the community-level entropy of authors’ distribution by bibliometric classes and age groups. We display results for three community detection scenarios out of 18 that were assessed, to maintain the figure’s clarity (see further robustness results including evaluation of authors’ country of affiliation and gender in SI). The fact that all density curves are strongly skewed towards high entropy values (max entropy = 1) confirms our visual assessment of Panels A and B and suggests our results are robust to different community detection scenarios and algorithms.
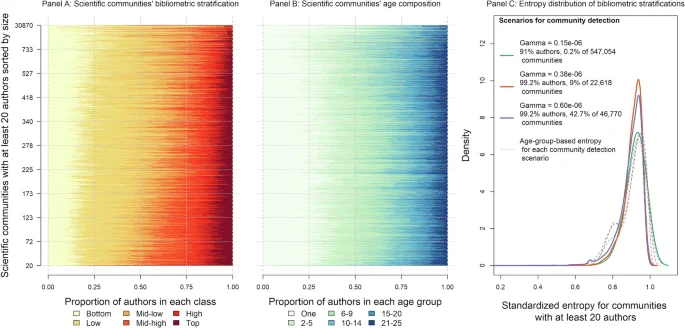
To investigate the trends shown in Fig. 2 further and control the collaboration structure among the classes, we turned to co-authorship networks of the studied 28 million publications. Networks of collaboration in terms of co-authoring scientific publications among 8.2 million authors worldwide allowed us to identify communities of collaboration. We used the Constant Potts Model (CPM) and its extension for bipartite networks with a varying range of 18 thresholds for the resolution parameter to detect communities. In all these detected communities (only 3 shown in the figure to preserve clarity), we investigated the class (A) and age (B) composition of members. Independent from the threshold used, all these communities have a heterogeneous composition of classes and age groups and analysis of entropies of this stratification (C) indicates an inter-class and inter-age collaboration structure among the most and least prolific, collaborative/internationalized, and mobile scientists. SI includes figures with further robustness analysis using three other community detection algorithms, one-mode projection of the network and results using Leiden (Traag et al. 2019) algorithm, and also disaggregated analysis based on gender of authors to males and females which did not show a change in the reported trends.
Discussion
This paper provided a quantitative assessment of the global inequalities in science using bibliometric data across fields of science and research communities. Our results show that a stratified system in terms of bibliometric performance exists in all macro fields of science, and it is as strong as fields’ stratification by academic age. As scholars age (i.e., progress to more senior academic career stages) and maintain consistent participation in publication systems, their positioning within the bibliometric-based academic hierarchy becomes clearer. This clarity evolves potentially due to increased exposure and experience in publishing, highlighting the role of time and continued scholarly activity in shaping bibliometric classes. In addition, we evaluated collaboration ties among classes and whether specific age groups dominate it. We provide the aggregated data to enable future research on the causes and consequences of this stratification (Akbaritabar and Castro Torres 2024).
Our multivariate assessment of bibliometric classes is grounded in the assumption that scholars’ prestige within their respective fields does not rely solely on a single indicator, such as the number of citations or publications. Instead, we assume that scholars’ standing and prestige is based on their performance across multiple indicators. Consequently, the top class includes authors who may not necessarily rank at the highest levels in every individual indicator but possess the most favorable overall academic profiles. Similarly, the middle and lower classes encompass authors with varying degrees of less favorable academic profiles. This conceptualization of academic performance introduces nuances to the conventional 20/80 rule, demonstrating that it does not necessarily apply universally. It emphasizes that individual contributions to a particular output are more intricate than the notion that the top 20% contribute 80% of the outcome. We found that top classes, defined multidimensionally, contribute less than 80% in most of the cases. Bottom classes’ contributions are minimal suggesting the existence of very distinct academic careers. While the causes and implications of these disparities are yet to be examined, we speculate that differential access to resources and additional labor (Zhang et al. 2022) that could be higher among the top class and be perpetuated through additional funding and new resources allocated to them in performance-based funding schemes (Akbaritabar et al. 2021; Zacharewicz et al. 2019) could drive the persisting trends. The positive age pattern of bibliometric stratification suggest that these are no unlikely speculations. Greater exposure to publication systems and continued publishing activities likely serve as reinforcing mechanisms, contributing to the observed patterns of bibliometric stratification advancement over academic age.
Science is transmitted from established scholars to new generations through a mentorship relationship that affects mentees’ future success (Ke et al. 2022; Liénard et al. 2018; Ma et al. 2020). Such supervisor-supervisee relationships inherently have an age component. Hence, we expect that a share of observed scientific collaborations will be among junior and senior scholars. Nevertheless, our results show that the proportion of scholars who exit the system after only one paper amounts to 25% or more of the members of identified communities, which cannot be solely representing the age structure of academia and could be driven by the performance measures described and the hierarchical structure inherent in them that drives a high proportion to exit the system. We emphasize that not all graduate students continue the career paths in research leading to continued publication activity. Nonetheless, the probability of having higher impact and citations in the science system is disproportionately distributed and highly stratified (Nielsen and Andersen 2021).
Our study has a descriptive nature, despite the comprehensive inclusion of all most widely used bibliometric variables, their relationships, while considering academic age differences and fields of science. With the current descriptive setup, it is not possible to evaluate if the observed quantitative stratification signals inequality in access to resources such as research assistants and junior collaborators (Zhang et al. 2022). We do not know much about the type of contracts or positions these studied researchers hold; we only know their academic age. Similarly, the prestige of these academic institutions is not covered in our analysis, as well as the national policies that might affect the resources one accesses. These differences in resources and environment affect the type of research one can do and could lead to a different position on observational data i.e., bibliometric indicators. While our study sheds light on the stratifications because of its elaborated and comprehensive use of all relevant bibliometric variables, we did not have a causal setup and cannot evaluate the underlying causes leading to the reported stratifications and presented arguments on potential causes are based on our speculations.
Bibliometric indicators are widely used in national research assessment exercises (Akbaritabar et al. 2021; Zacharewicz et al. 2019) to determine who should be hired and promoted and whose research should be funded (Sugimoto and Larivière 2018). Based on our analysis, which was possible by adopting a global, multivariate, and multi-method framework to debunk the widely-spread myths about increased productivity, collaboration, internationalization, mobility, and impact among scientists, we call for a further elaborated investigation of these trends. We propose considering academic age, career cohorts and composition of a multitude of bibliometric variables instead of solely relying on one-indicator explanations which might be appealing to attract policy-makers’ attention, but might be detrimental to our understanding of the science system, its social structure, and its inherent stratification and intersectional inequalities (Kozlowski et al. 2022).
Data availability
All data to replicate presented results are publicly accessible under: Aliakbar Akbaritabar, & Castro Torres, A. F. (2024). Replication data for: A global perspective on social stratification in science (1.0). Zenodo. https://doi.org/10.5281/zenodo.12527944.
References
-
Abramo G, D’Angelo CA, Di Costa F (2009) Research collaboration and productivity: Is there correlation? High Educ 57(2):155–171. https://doi.org/10.1007/s10734-008-9139-z
Google Scholar
-
Akbaritabar A (2021) A quantitative view of the structure of institutional scientific collaborations using the example of Berlin. Quant Sci Stud 2(2):753–777. https://doi.org/10.1162/qss_a_00131
Google Scholar
-
Akbaritabar A, Barbato G (2021) An internationalised Europe and regionally focused Americas: A network analysis of higher education studies. Eur J Educ 56(2):219–234. https://doi.org/10.1111/ejed.12446
Google Scholar
-
Akbaritabar A, Bravo G, & Squazzoni F (2021) The impact of a national research assessment on the publications of sociologists in Italy. Sci. Public Policy. scab013. https://doi.org/10.1093/scipol/scab013
-
Akbaritabar A, & Castro Torres AF (2024) akbaritabar/A-global-perspective-on-social-stratification-in-science: 1.0 (1.0) [Computer software]. Zenodo. https://doi.org/10.5281/zenodo.12527944
-
Akbaritabar A, & Squazzoni F (2020) Gender Patterns of Publication in Top Sociological Journals. Sci, Technol, Hum Values. https://doi.org/10.1177/0162243920941588
-
Akbaritabar A, Theile T, & Zagheni E (2023) Global flows and rates of international migration of scholars. MPIDR Working Paper, 018. https://doi.org/10.4054/MPIDR-WP-2023-018
-
Akbaritabar A, & Castro Torres, AF (2024) Replication data for: A global perspective on social stratification in science (1.0). Zenodo. https://doi.org/10.5281/zenodo.12527944
-
Alper J (1993) The Pipeline Is Leaking Women All the Way Along. Science 260(5106):409–411. https://doi.org/10.1126/science.260.5106.409
Google Scholar
-
Aman V (2018) Does the Scopus author ID suffice to track scientific international mobility? A case study based on Leibniz laureates. Scientometrics 117(2):705–720. https://doi.org/10.1007/s11192-018-2895-3
Google Scholar
-
Baas J, Schotten M, Plume A, Côté G, Karimi R (2020) Scopus as a curated, high-quality bibliometric data source for academic research in quantitative science studies. Quant Sci Stud 1(1):377–386. https://doi.org/10.1162/qss_a_00019
Google Scholar
-
Bourdieu P, & Passeron JC (1979) The Inheritors: French Students and Their Relation to Culture. University of Chicago Press
-
Burris V (2004) The Academic Caste System: Prestige Hierarchies in PhD Exchange Networks. Am Sociol Rev 69(2):239–264. https://doi.org/10.1177/000312240406900205
Google Scholar
-
Castro Torres AF, Alburez-Gutierrez D (2022) North and South: Naming practices and the hidden dimension of global disparities in knowledge production. Proc Natl Acad Sci 119(10):e2119373119. https://doi.org/10.1073/pnas.2119373119
Google Scholar
-
Chompalov I, Genuth J, Shrum W (2002) The organization of scientific collaborations. Res Policy 31(5):749–767
Google Scholar
-
Clauset A, Arbesman S, Larremore DB (2015) Systematic inequality and hierarchy in faculty hiring networks. Sci Adv 1(1):e1400005. https://doi.org/10.1126/sciadv.1400005
Google Scholar
-
Fanelli D, Larivière V (2016) Researchers’ Individual Publication Rate Has Not Increased in a Century. PLOS ONE 11(3):e0149504. https://doi.org/10.1371/journal.pone.0149504
Google Scholar
-
Fox MF, Nikivincze I (2021) Being highly prolific in academic science: Characteristics of individuals and their departments. High Educ 81(6):1237–1255. https://doi.org/10.1007/s10734-020-00609-z
Google Scholar
-
Hofstra B, McFarland DA, Smith S, Jurgens D (2022) Diversifying the Professoriate. Socius 8:23780231221085118. https://doi.org/10.1177/23780231221085118
Google Scholar
-
Ioannidis JPA, Klavans R, Boyack KW (2018) Thousands of scientists publish a paper every five days. Nature 561(7722):167–169. https://doi.org/10.1038/d41586-018-06185-8
Google Scholar
-
Ke Q, Liang L, Ding Y, David SV, Acuna DE (2022) A dataset of mentorship in bioscience with semantic and demographic estimations. Sci Data 9(1):1. https://doi.org/10.1038/s41597-022-01578-x
Google Scholar
-
Kozlowski D, Andersen JP, Larivière V (2024) The decrease in uncited articles and its effect on the concentration of citations. J Assoc Inf Sci Technol 75(2):188–197. https://doi.org/10.1002/asi.24852
Google Scholar
-
Kozlowski D, Larivière V, Sugimoto CR, & Monroe-White T (2022) Intersectional inequalities in science. Proc Natl Acad Sci. 119(2). https://doi.org/10.1073/pnas.2113067119
-
Larivière V, Ni C, Gingras Y, Cronin B, Sugimoto CR (2013) Bibliometrics: Global gender disparities in science. Nature 504(7479):211–213. https://doi.org/10.1038/504211a
Google Scholar
-
Le Roux B, & Rouanet H (2004) Geometric Data Analysis: From correspondence analysis to structured data analysis. Dordrecht
-
Leahey E, Cain CL (2013) Straight from the source: Accounting for scientific success. Soc Stud Sci 43(6):927–951. https://doi.org/10.1177/0306312713484820
Google Scholar
-
Liénard JF, Achakulvisut T, Acuna DE, & David SV (2018) Intellectual synthesis in mentorship determines success in academic careers. Nat. Commun. 9(1), Article 1. https://doi.org/10.1038/s41467-018-07034-y
-
Liu L, Wang Y, Sinatra R, Giles CL, Song C, Wang D (2018) Hot streaks in artistic, cultural, and scientific careers. Nature 559(7714):7714. https://doi.org/10.1038/s41586-018-0315-8
Google Scholar
-
Lozano GA, Larivière V, Gingras Y (2012) The weakening relationship between the impact factor and papers’ citations in the digital age. J Am Soc Inf Sci Technol 63(11):2140–2145. https://doi.org/10.1002/asi.22731
Google Scholar
-
Ma Y, Mukherjee S, & Uzzi B (2020) Mentorship and protégé success in STEM fields. Proc Natl Acad Sci. https://doi.org/10.1073/pnas.1915516117
-
Marini G, Meschitti V (2018) The trench warfare of gender discrimination: Evidence from academic promotions to full professor in Italy. Scientometrics 115(2):989–1006. https://doi.org/10.1007/s11192-018-2696-8
Google Scholar
-
Mazzucato M (2018) The entrepreneurial state: Debunking public vs. private sector myths. Penguin Books
-
Melkers J, Kiopa A (2010) The Social Capital of Global Ties in Science: The Added Value of International Collaboration: The Social Capital of Global Ties in Science. Rev Policy Res 27(4):389–414. https://doi.org/10.1111/j.1541-1338.2010.00448.x
Google Scholar
-
Merton RK (1968) The Matthew Effect in Science. Science 159(3810):56–63. https://doi.org/10.1126/science.159.3810.56
Google Scholar
-
Müller R (2012) Collaborating in Life Science Research Groups: The Question of Authorship. High Educ Policy 25(3):289–311. https://doi.org/10.1057/hep.2012.11
Google Scholar
-
Nielsen MW & Andersen JP (2021) Global citation inequality is on the rise. Proc Natl Acad Sci. 118(7). https://doi.org/10.1073/pnas.2012208118
-
Nogrady B (2023) Hyperauthorship: The publishing challenges for ‘big team’ science. Nature 615(7950):175–177. https://doi.org/10.1038/d41586-023-00575-3
Google Scholar
-
Penman D, Goldson S (2015) Competition to collaboration: Changing the dynamics of science. J R Soc N. Z 45(2):118–121. https://doi.org/10.1080/03036758.2015.1011172
Google Scholar
-
Pikkety T (2019) Capital et idéologie (1st ed.). Seuil
-
Reichardt J, Bornholdt S (2004) Detecting fuzzy community structures in complex networks with a Potts model. Phys Rev Lett 93(21):218701. https://doi.org/10.1103/PhysRevLett.93.218701
Google Scholar
-
Sanliturk E, Zagheni E, Dańko MJ, Theile T, Akbaritabar A (2023) Global patterns of migration of scholars with economic development. Proc Natl Acad Sci 120(4):e2217937120. https://doi.org/10.1073/pnas.2217937120
Google Scholar
-
Shrum W, Chompalov I, Genuth J (2001) Trust, Conflict and Performance in Scientific Collaborations. Soc Stud Sci 31(5):681–730. https://doi.org/10.1177/030631201031005002
Google Scholar
-
Shrum W, Genuth J, Carlson WB, Chompalov I, & Bijker WE (2007) Structures of Scientific Collaboration. MIT Press
-
Sinatra R, Wang D, Deville P, Song C, Barabasi A-L(2016) Quantifying the evolution of individual scientific impact. Science 354(6312):aaf5239. https://doi.org/10.1126/science.aaf5239
Google Scholar
-
Singh Chawla D (2019) Hyperauthorship: Global projects spark surge in thousand-author papers. Nature. https://doi.org/10.1038/d41586-019-03862-0
-
Sugimoto CR, & Larivière V (2018) Measuring Research: What Everyone Needs to Know. Oxford University Press
-
Sugimoto CR, Robinson-Garcia N, Murray DS, Yegros-Yegros A, Costas R, Larivière V (2017) Scientists have most impact when they’re free to move. Nature 550(7674):29–31. https://doi.org/10.1038/550029a
Google Scholar
-
Therborn G (2013) The killing fields of inequality. Polity
-
Traag VA, Van Dooren P, Nesterov Y (2011) Narrow scope for resolution-limit-free community detection. Phys Rev E 84(1):016114. https://doi.org/10.1103/PhysRevE.84.016114
Google Scholar
-
Traag VA, Waltman L, & van Eck NJ (2019) From Louvain to Leiden: Guaranteeing well-connected communities. Sci Rep 9(1), 5233. https://doi.org/10.1038/s41598-019-41695-z
-
van den Besselaar P, Hemlin S, van der Weijden I (2012) Collaboration and Competition in Research. High Educ Policy 25(3):263–266. https://doi.org/10.1057/hep.2012.16
Google Scholar
-
Wilsdon J, Allen L, Belfiore E, Campbell P, Curry S, Hill S, Jones R, Kain R, Kerridge S, Thelwall M, Tinkler J, Viney I, Wouters P, Hill J, & Johnson B (2015) The Metric Tide: Report of the Independent Review of the Role of Metrics in Research Assessment and Management. https://doi.org/10.13140/RG.2.1.4929.1363
-
Wuchty S, Jones BF, Uzzi B (2007) The Increasing Dominance of Teams in Production of Knowledge. Science 316(5827):1036–1039. https://doi.org/10.1126/science.1136099
Google Scholar
-
Zacharewicz T, Lepori B, Reale E, Jonkers K (2019) Performance-based research funding in EU Member States—A comparative assessment. Sci Public Policy 46(1):105–115. https://doi.org/10.1093/scipol/scy041
Google Scholar
-
Zhang S, Wapman KH, Larremore DB, Clauset A (2022) Labor advantages drive the greater productivity of faculty at elite universities. Sci Adv 8(46):eabq7056. https://doi.org/10.1126/sciadv.abq7056
Google Scholar
-
Zhao X, Akbaritabar A, Kashyap R, Zagheni E (2023) A gender perspective on the global migration of scholars. Proc Natl Acad Sci 120(10):e2214664120. https://doi.org/10.1073/pnas.2214664120
Google Scholar
-
Zhao Z, Bu Y, & Li J (2020) Does the mobility of scientists disrupt their collaboration stability? J. Info Sci. 016555152094874. https://doi.org/10.1177/0165551520948744
Acknowledgements
We thank Cassidy R. Sugimoto for helpful comments on an earlier version of this manuscript. This study has received access to the bibliometric data through the project “Kompetenznetzwerk Bibliometrie” and we acknowledge their funder Bundesministerium für Bildung und Forschung (grant number 16WIK2101A). AFCT received support from the Catalonian Goverment (grant number 2021 BP 00027). Open Access funding enabled and organized by Projekt DEAL.
Author information
Authors and Affiliations
Contributions
AA: Conceptualization, Methodology, Software, Validation, Data Curation, Formal analysis, Investigation, Writing – Original Draft, Writing – Review & Editing, Visualization. AFCT: Conceptualization, Methodology, Software, Validation, Formal analysis, Investigation, Writing – Original Draft, Writing – Review & Editing, Visualization. VL: Conceptualization, Writing – Original Draft, Writing – Review & Editing.
Corresponding author
Ethics declarations
Competing interests
The authors declare no competing interests.
Ethical approval
Not applicable.
Informed consent
Not applicable.
Additional information
Publisher’s note Springer Nature remains neutral with regard to jurisdictional claims in published maps and institutional affiliations.
Supplementary information
Supplementary Information
Rights and permissions
Open Access This article is licensed under a Creative Commons Attribution 4.0 International License, which permits use, sharing, adaptation, distribution and reproduction in any medium or format, as long as you give appropriate credit to the original author(s) and the source, provide a link to the Creative Commons licence, and indicate if changes were made. The images or other third party material in this article are included in the article’s Creative Commons licence, unless indicated otherwise in a credit line to the material. If material is not included in the article’s Creative Commons licence and your intended use is not permitted by statutory regulation or exceeds the permitted use, you will need to obtain permission directly from the copyright holder. To view a copy of this licence, visit http://creativecommons.org/licenses/by/4.0/.
Reprints and permissions
About this article
Cite this article
Akbaritabar, A., Castro Torres, A.F. & Larivière, V. A global perspective on social stratification in science.
Humanit Soc Sci Commun 11, 914 (2024). https://doi.org/10.1057/s41599-024-03402-w
-
Received: 07 October 2023
-
Accepted: 27 June 2024
-
Published: 13 July 2024
-
DOI: https://doi.org/10.1057/s41599-024-03402-w