Abstract
Brain-age estimation has gained increased attention in the neuroscientific community owing to its potential use as a biomarker of brain health. The difference between estimated and chronological age based on neuroimaging data enables a unique perspective on brain development and aging, with multiple open questions still remaining in the brain-age research field. This Perspective presents an overview of current advancements in the field and envisions the future evolution of the brain-age framework before its potential deployment in hospital settings.
This is a preview of subscription content, access via your institution
Access options
style{display:none!important}.LiveAreaSection-193358632 *{align-content:stretch;align-items:stretch;align-self:auto;animation-delay:0s;animation-direction:normal;animation-duration:0s;animation-fill-mode:none;animation-iteration-count:1;animation-name:none;animation-play-state:running;animation-timing-function:ease;azimuth:center;backface-visibility:visible;background-attachment:scroll;background-blend-mode:normal;background-clip:borderBox;background-color:transparent;background-image:none;background-origin:paddingBox;background-position:0 0;background-repeat:repeat;background-size:auto auto;block-size:auto;border-block-end-color:currentcolor;border-block-end-style:none;border-block-end-width:medium;border-block-start-color:currentcolor;border-block-start-style:none;border-block-start-width:medium;border-bottom-color:currentcolor;border-bottom-left-radius:0;border-bottom-right-radius:0;border-bottom-style:none;border-bottom-width:medium;border-collapse:separate;border-image-outset:0s;border-image-repeat:stretch;border-image-slice:100%;border-image-source:none;border-image-width:1;border-inline-end-color:currentcolor;border-inline-end-style:none;border-inline-end-width:medium;border-inline-start-color:currentcolor;border-inline-start-style:none;border-inline-start-width:medium;border-left-color:currentcolor;border-left-style:none;border-left-width:medium;border-right-color:currentcolor;border-right-style:none;border-right-width:medium;border-spacing:0;border-top-color:currentcolor;border-top-left-radius:0;border-top-right-radius:0;border-top-style:none;border-top-width:medium;bottom:auto;box-decoration-break:slice;box-shadow:none;box-sizing:border-box;break-after:auto;break-before:auto;break-inside:auto;caption-side:top;caret-color:auto;clear:none;clip:auto;clip-path:none;color:initial;column-count:auto;column-fill:balance;column-gap:normal;column-rule-color:currentcolor;column-rule-style:none;column-rule-width:medium;column-span:none;column-width:auto;content:normal;counter-increment:none;counter-reset:none;cursor:auto;display:inline;empty-cells:show;filter:none;flex-basis:auto;flex-direction:row;flex-grow:0;flex-shrink:1;flex-wrap:nowrap;float:none;font-family:initial;font-feature-settings:normal;font-kerning:auto;font-language-override:normal;font-size:medium;font-size-adjust:none;font-stretch:normal;font-style:normal;font-synthesis:weight style;font-variant:normal;font-variant-alternates:normal;font-variant-caps:normal;font-variant-east-asian:normal;font-variant-ligatures:normal;font-variant-numeric:normal;font-variant-position:normal;font-weight:400;grid-auto-columns:auto;grid-auto-flow:row;grid-auto-rows:auto;grid-column-end:auto;grid-column-gap:0;grid-column-start:auto;grid-row-end:auto;grid-row-gap:0;grid-row-start:auto;grid-template-areas:none;grid-template-columns:none;grid-template-rows:none;height:auto;hyphens:manual;image-orientation:0deg;image-rendering:auto;image-resolution:1dppx;ime-mode:auto;inline-size:auto;isolation:auto;justify-content:flexStart;left:auto;letter-spacing:normal;line-break:auto;line-height:normal;list-style-image:none;list-style-position:outside;list-style-type:disc;margin-block-end:0;margin-block-start:0;margin-bottom:0;margin-inline-end:0;margin-inline-start:0;margin-left:0;margin-right:0;margin-top:0;mask-clip:borderBox;mask-composite:add;mask-image:none;mask-mode:matchSource;mask-origin:borderBox;mask-position:0 0;mask-repeat:repeat;mask-size:auto;mask-type:luminance;max-height:none;max-width:none;min-block-size:0;min-height:0;min-inline-size:0;min-width:0;mix-blend-mode:normal;object-fit:fill;object-position:50% 50%;offset-block-end:auto;offset-block-start:auto;offset-inline-end:auto;offset-inline-start:auto;opacity:1;order:0;orphans:2;outline-color:initial;outline-offset:0;outline-style:none;outline-width:medium;overflow:visible;overflow-wrap:normal;overflow-x:visible;overflow-y:visible;padding-block-end:0;padding-block-start:0;padding-bottom:0;padding-inline-end:0;padding-inline-start:0;padding-left:0;padding-right:0;padding-top:0;page-break-after:auto;page-break-before:auto;page-break-inside:auto;perspective:none;perspective-origin:50% 50%;pointer-events:auto;position:static;quotes:initial;resize:none;right:auto;ruby-align:spaceAround;ruby-merge:separate;ruby-position:over;scroll-behavior:auto;scroll-snap-coordinate:none;scroll-snap-destination:0 0;scroll-snap-points-x:none;scroll-snap-points-y:none;scroll-snap-type:none;shape-image-threshold:0;shape-margin:0;shape-outside:none;tab-size:8;table-layout:auto;text-align:initial;text-align-last:auto;text-combine-upright:none;text-decoration-color:currentcolor;text-decoration-line:none;text-decoration-style:solid;text-emphasis-color:currentcolor;text-emphasis-position:over right;text-emphasis-style:none;text-indent:0;text-justify:auto;text-orientation:mixed;text-overflow:clip;text-rendering:auto;text-shadow:none;text-transform:none;text-underline-position:auto;top:auto;touch-action:auto;transform:none;transform-box:borderBox;transform-origin:50% 50%0;transform-style:flat;transition-delay:0s;transition-duration:0s;transition-property:all;transition-timing-function:ease;vertical-align:baseline;visibility:visible;white-space:normal;widows:2;width:auto;will-change:auto;word-break:normal;word-spacing:normal;word-wrap:normal;writing-mode:horizontalTb;z-index:auto;-webkit-appearance:none;-moz-appearance:none;-ms-appearance:none;appearance:none;margin:0}.LiveAreaSection-193358632{width:100%}.LiveAreaSection-193358632 .login-option-buybox{display:block;width:100%;font-size:17px;line-height:30px;color:#222;padding-top:30px;font-family:Harding,Palatino,serif}.LiveAreaSection-193358632 .additional-access-options{display:block;font-weight:700;font-size:17px;line-height:30px;color:#222;font-family:Harding,Palatino,serif}.LiveAreaSection-193358632 .additional-login>li:not(:first-child)::before{transform:translateY(-50%);content:””;height:1rem;position:absolute;top:50%;left:0;border-left:2px solid #999}.LiveAreaSection-193358632 .additional-login>li:not(:first-child){padding-left:10px}.LiveAreaSection-193358632 .additional-login>li{display:inline-block;position:relative;vertical-align:middle;padding-right:10px}.BuyBoxSection-683559780{display:flex;flex-wrap:wrap;flex:1;flex-direction:row-reverse;margin:-30px -15px 0}.BuyBoxSection-683559780 .box-inner{width:100%;height:100%;padding:30px 5px;display:flex;flex-direction:column;justify-content:space-between}.BuyBoxSection-683559780 p{margin:0}.BuyBoxSection-683559780 .readcube-buybox{background-color:#f3f3f3;flex-shrink:1;flex-grow:1;flex-basis:255px;background-clip:content-box;padding:0 15px;margin-top:30px}.BuyBoxSection-683559780 .subscribe-buybox{background-color:#f3f3f3;flex-shrink:1;flex-grow:4;flex-basis:300px;background-clip:content-box;padding:0 15px;margin-top:30px}.BuyBoxSection-683559780 .subscribe-buybox-nature-plus{background-color:#f3f3f3;flex-shrink:1;flex-grow:4;flex-basis:100%;background-clip:content-box;padding:0 15px;margin-top:30px}.BuyBoxSection-683559780 .title-readcube,.BuyBoxSection-683559780 .title-buybox{display:block;margin:0;margin-right:10%;margin-left:10%;font-size:24px;line-height:32px;color:#222;text-align:center;font-family:Harding,Palatino,serif}.BuyBoxSection-683559780 .title-asia-buybox{display:block;margin:0;margin-right:5%;margin-left:5%;font-size:24px;line-height:32px;color:#222;text-align:center;font-family:Harding,Palatino,serif}.BuyBoxSection-683559780 .asia-link{color:#069;cursor:pointer;text-decoration:none;font-size:1.05em;font-family:-apple-system,BlinkMacSystemFont,”Segoe UI”,Roboto,Oxygen-Sans,Ubuntu,Cantarell,”Helvetica Neue”,sans-serif;line-height:1.05em6}.BuyBoxSection-683559780 .access-readcube{display:block;margin:0;margin-right:10%;margin-left:10%;font-size:14px;color:#222;padding-top:10px;text-align:center;font-family:-apple-system,BlinkMacSystemFont,”Segoe UI”,Roboto,Oxygen-Sans,Ubuntu,Cantarell,”Helvetica Neue”,sans-serif;line-height:20px}.BuyBoxSection-683559780 ul{margin:0}.BuyBoxSection-683559780 .link-usp{display:list-item;margin:0;margin-left:20px;padding-top:6px;list-style-position:inside}.BuyBoxSection-683559780 .link-usp span{font-size:14px;color:#222;font-family:-apple-system,BlinkMacSystemFont,”Segoe UI”,Roboto,Oxygen-Sans,Ubuntu,Cantarell,”Helvetica Neue”,sans-serif;line-height:20px}.BuyBoxSection-683559780 .access-asia-buybox{display:block;margin:0;margin-right:5%;margin-left:5%;font-size:14px;color:#222;padding-top:10px;text-align:center;font-family:-apple-system,BlinkMacSystemFont,”Segoe UI”,Roboto,Oxygen-Sans,Ubuntu,Cantarell,”Helvetica Neue”,sans-serif;line-height:20px}.BuyBoxSection-683559780 .access-buybox{display:block;margin:0;margin-right:10%;margin-left:10%;font-size:14px;color:#222;opacity:.8px;padding-top:10px;text-align:center;font-family:-apple-system,BlinkMacSystemFont,”Segoe UI”,Roboto,Oxygen-Sans,Ubuntu,Cantarell,”Helvetica Neue”,sans-serif;line-height:20px}.BuyBoxSection-683559780 .price-buybox{display:block;font-size:30px;color:#222;font-family:-apple-system,BlinkMacSystemFont,”Segoe UI”,Roboto,Oxygen-Sans,Ubuntu,Cantarell,”Helvetica Neue”,sans-serif;padding-top:30px;text-align:center}.BuyBoxSection-683559780 .price-buybox-to{display:block;font-size:30px;color:#222;font-family:-apple-system,BlinkMacSystemFont,”Segoe UI”,Roboto,Oxygen-Sans,Ubuntu,Cantarell,”Helvetica Neue”,sans-serif;text-align:center}.BuyBoxSection-683559780 .price-info-text{font-size:16px;padding-right:10px;color:#222;font-family:-apple-system,BlinkMacSystemFont,”Segoe UI”,Roboto,Oxygen-Sans,Ubuntu,Cantarell,”Helvetica Neue”,sans-serif}.BuyBoxSection-683559780 .price-value{font-size:30px;font-family:-apple-system,BlinkMacSystemFont,”Segoe UI”,Roboto,Oxygen-Sans,Ubuntu,Cantarell,”Helvetica Neue”,sans-serif}.BuyBoxSection-683559780 .price-per-period{font-family:-apple-system,BlinkMacSystemFont,”Segoe UI”,Roboto,Oxygen-Sans,Ubuntu,Cantarell,”Helvetica Neue”,sans-serif}.BuyBoxSection-683559780 .price-from{font-size:14px;padding-right:10px;color:#222;font-family:-apple-system,BlinkMacSystemFont,”Segoe UI”,Roboto,Oxygen-Sans,Ubuntu,Cantarell,”Helvetica Neue”,sans-serif;line-height:20px}.BuyBoxSection-683559780 .issue-buybox{display:block;font-size:13px;text-align:center;color:#222;font-family:-apple-system,BlinkMacSystemFont,”Segoe UI”,Roboto,Oxygen-Sans,Ubuntu,Cantarell,”Helvetica Neue”,sans-serif;line-height:19px}.BuyBoxSection-683559780 .no-price-buybox{display:block;font-size:13px;line-height:18px;text-align:center;padding-right:10%;padding-left:10%;padding-bottom:20px;padding-top:30px;color:#222;font-family:-apple-system,BlinkMacSystemFont,”Segoe UI”,Roboto,Oxygen-Sans,Ubuntu,Cantarell,”Helvetica Neue”,sans-serif}.BuyBoxSection-683559780 .vat-buybox{display:block;margin-top:5px;margin-right:20%;margin-left:20%;font-size:11px;color:#222;padding-top:10px;padding-bottom:15px;text-align:center;font-family:-apple-system,BlinkMacSystemFont,”Segoe UI”,Roboto,Oxygen-Sans,Ubuntu,Cantarell,”Helvetica Neue”,sans-serif;line-height:17px}.BuyBoxSection-683559780 .tax-buybox{display:block;width:100%;color:#222;padding:20px 16px;text-align:center;font-family:-apple-system,BlinkMacSystemFont,”Segoe UI”,Roboto,Oxygen-Sans,Ubuntu,Cantarell,”Helvetica Neue”,sans-serif;line-height:NaNpx}.BuyBoxSection-683559780 .button-container{display:flex;padding-right:20px;padding-left:20px;justify-content:center}.BuyBoxSection-683559780 .button-container>*{flex:1px}.BuyBoxSection-683559780 .button-container>a:hover,.Button-505204839:hover,.Button-1078489254:hover,.Button-2737859108:hover{text-decoration:none}.BuyBoxSection-683559780 .btn-secondary{background:#fff}.BuyBoxSection-683559780 .button-asia{background:#069;border:1px solid #069;border-radius:0;cursor:pointer;display:block;padding:9px;outline:0;text-align:center;text-decoration:none;min-width:80px;margin-top:75px}.BuyBoxSection-683559780 .button-label-asia,.ButtonLabel-3869432492,.ButtonLabel-3296148077,.ButtonLabel-1636778223{display:block;color:#fff;font-size:17px;line-height:20px;font-family:-apple-system,BlinkMacSystemFont,”Segoe UI”,Roboto,Oxygen-Sans,Ubuntu,Cantarell,”Helvetica Neue”,sans-serif;text-align:center;text-decoration:none;cursor:pointer}.Button-505204839,.Button-1078489254,.Button-2737859108{background:#069;border:1px solid #069;border-radius:0;cursor:pointer;display:block;padding:9px;outline:0;text-align:center;text-decoration:none;min-width:80px;max-width:320px;margin-top:20px}.Button-505204839 .btn-secondary-label,.Button-1078489254 .btn-secondary-label,.Button-2737859108 .btn-secondary-label{color:#069}
/* style specs end */
Access Nature and 54 other Nature Portfolio journals
Get Nature+, our best-value online-access subscription
$29.99 / 30 days
cancel any time
Subscribe to this journal
Receive 12 digital issues and online access to articles
$99.00 per year
only $8.25 per issue
Buy this article
- Purchase on Springer Link
- Instant access to full article PDF
Prices may be subject to local taxes which are calculated during checkout
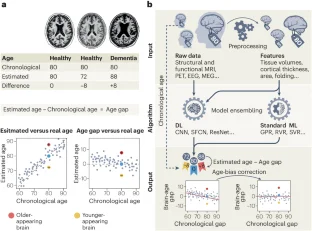
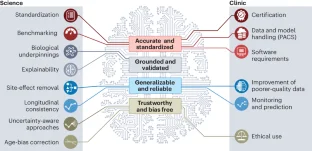
Similar content being viewed by others
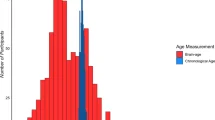
Brain-age in midlife is associated with accelerated biological aging and cognitive decline in a longitudinal birth cohort
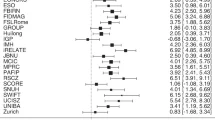
Brain ageing in schizophrenia: evidence from 26 international cohorts via the ENIGMA Schizophrenia consortium
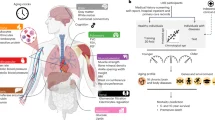
Heterogeneous aging across multiple organ systems and prediction of chronic disease and mortality
References
-
Diebel, L. W. M. & Rockwood, K. Determination of biological age: geriatric assessment vs biological biomarkers. Curr. Oncol. Rep. 23, 104 (2021).
Google Scholar
-
Ferrucci, L. et al. Measuring biological aging in humans: a quest. Aging Cell 19, e13080 (2020).
Google Scholar
-
Belsky, D. W. et al. Quantification of the pace of biological aging in humans through a blood test, the DunedinPoAm DNA methylation algorithm. eLife 9, e54870 (2020).
Google Scholar
-
Hannum, G. et al. Genome-wide methylation profiles reveal quantitative views of human aging rates. Mol. Cell 49, 359–367 (2013).
Google Scholar
-
Horvath, S. DNA methylation age of human tissues and cell types. Genome Biol. 14, R115 (2013).
Google Scholar
-
Tian, Y. E. et al. Heterogeneous aging across multiple organ systems and prediction of chronic disease and mortality. Nat. Med. 29, 1221–1231 (2023).
Google Scholar
-
Cole, J. H., Marioni, R. E., Harris, S. E. & Deary, I. J. Brain age and other bodily ‘ages’: implications for neuropsychiatry. Mol. Psychiatry 24, 266–281 (2019).
Google Scholar
-
Anderton, B. H. Ageing of the brain. Mech. Ageing Dev. 123, 811–817 (2002).
Google Scholar
-
Livingston, G. et al. Dementia prevention, intervention, and care: 2020 report of the Lancet Commission. Lancet 396, 413–446 (2020).
Google Scholar
-
Cole, J. H. & Franke, K. Predicting age using neuroimaging: innovative brain ageing biomarkers. Trends Neurosci. 40, 681–690 (2017).
Google Scholar
-
Baecker, L. et al. Machine learning for brain age prediction: introduction to methods and clinical applications. EBioMedicine 72, 103600 (2021a).
Google Scholar
-
Franke, K. & Gaser, C. Ten years of BrainAGE as a neuroimaging biomarker of brain aging: what insights have we gained? Front. Neurol. 10, 789 (2019).
Google Scholar
-
Mishra, S., Beheshti, I. & Khanna, P. A review of neuroimaging-driven brain age estimation for identification of brain disorders and health conditions. IEEE Rev. Biomed. Eng. 16, 371–385 (2023).
Google Scholar
-
Sone, D. & Beheshti, I. Neuroimaging-based brain age estimation: a promising personalized biomarker in neuropsychiatry. J. Pers. Med. 12, 1850 (2022).
Google Scholar
-
Wrigglesworth, J. et al. Factors associated with brain ageing—a systematic review. BMC Neurol. 21, 312 (2021).
Google Scholar
-
Madan, C. R. Scan once, analyse many: using large open-access neuroimaging datasets to understand the brain. Neuroinform 20, 109–137 (2022).
Google Scholar
-
Jones, D. T., Lee, J. & Topol, E. J. Digitising brain age. Lancet 400, 988 (2022).
Google Scholar
-
Franke, K., Ziegler, G., Klöppel, S. & Gaser, C. Estimating the age of healthy subjects from T1-weighted MRI scans using kernel methods: exploring the influence of various parameters. NeuroImage 50, 883–892 (2010).
Google Scholar
-
Cole, J. H. et al. Prediction of brain age suggests accelerated atrophy after traumatic brain injury. Ann. Neurol. 77, 571–581 (2015).
Google Scholar
-
Bashyam, V. M. et al. MRI signatures of brain age and disease over the lifespan based on a deep brain network and 14 468 individuals worldwide. Brain 143, 2312–2324 (2020); erratum 144, e12 (2021).
-
Smith, S. M., Vidaurre, D., Alfaro-Almagro, F., Nichols, T. E. & Miller, K. L. Estimation of brain age delta from brain imaging. NeuroImage 200, 528–539 (2019).
Google Scholar
-
de Lange, A.-M. G. et al. Population-based neuroimaging reveals traces of childbirth in the maternal brain. Proc. Natl Acad. Sci. USA 116, 22341–22346 (2019).
Google Scholar
-
Beheshti, I., Mishra, S., Sone, D., Khanna, P. & Matsuda, H. T1-weighted MRI-driven brain age estimation in Alzheimer’s disease and Parkinson’s disease. Aging Dis. 11, 618–628 (2020).
Google Scholar
-
Abrol, A. et al. Deep learning encodes robust discriminative neuroimaging representations to outperform standard machine learning. Nat. Commun. 12, 353 (2021).
Google Scholar
-
Cole, J. H. et al. Brain age predicts mortality. Mol. Psychiatry 23, 1385–1392 (2018).
Google Scholar
-
Franke, K. & Gaser, C. Longitudinal changes in individual BrainAGE in healthy aging, mild cognitive impairment, and Alzheimer’s disease. GeroPsych 25, 235–245 (2012).
Google Scholar
-
Valizadeh, S. A., Hänggi, J., Mérillat, S. & Jäncke, L. Age prediction on the basis of brain anatomical measures. Hum. Brain Mapp. 38, 997–1008 (2017).
Google Scholar
-
Wang, J. et al. Age estimation using cortical surface pattern combining thickness with curvatures. Med. Biol. Eng. Comput. 52, 331–341 (2014).
Google Scholar
-
Dosenbach, N. U. et al. Prediction of individual brain maturity using fMRI. Science 329, 1358–1361 (2010); erratum 330, 756 (2010).
-
Lund, M. J. et al. Brain age prediction using fMRI network coupling in youths and associations with psychiatric symptoms. NeuroImage Clin. 33, 102921 (2022).
Google Scholar
-
Tønnesen, S. et al. Brain age prediction reveals aberrant brain white matter in schizophrenia and bipolar disorder: a multisample diffusion tensor imaging study. Biol. Psychiatry Cogn. Neurosci. Neuroimaging 5, 1095–1103 (2020).
-
Lee, J. et al. Deep learning-based brain age prediction in normal aging and dementia. Nat. Aging 2, 412–424 (2022).
Google Scholar
-
Al Zoubi, O. et al. Predicting age from brain EEG signals—a machine learning approach. Front. Aging Neurosci. 10, 184 (2018).
Google Scholar
-
Xifra-Porxas, A., Ghosh, A., Mitsis, G. D. & Boudrias, M.-H. Estimating brain age from structural MRI and MEG data: insights from dimensionality reduction techniques. NeuroImage 231, 117822 (2021).
Google Scholar
-
Cole, J. H. Multimodality neuroimaging brain-age in UK Biobank: relationship to biomedical, lifestyle, and cognitive factors. Neurobiol. Aging 92, 34–42 (2020).
Google Scholar
-
de Lange, A. G. et al. Multimodal brain-age prediction and cardiovascular risk: the Whitehall II MRI sub-study. NeuroImage 222, 117292 (2020).
Google Scholar
-
Liem, F. et al. Predicting brain-age from multimodal imaging data captures cognitive impairment. Neuroimage 148, 179–188 (2017).
Google Scholar
-
Ray, B. et al. Multimodal brain age prediction with feature selection and comparison. In 43rd Annual International Conference of the IEEE Engineering in Medicine & Biology Society (EMBC) https://doi.org/10.1109/EMBC46164.2021.9631007 (IEEE, 2021).
-
Hu, D. et al. Disentangled-multimodal adversarial autoencoder: application to infant age prediction with incomplete multimodal neuroimages. IEEE Trans. Med. Imaging 39, 4137–4149 (2020).
Google Scholar
-
Baecker, L. et al. Brain age prediction: a comparison between machine learning models using region- and voxel-based morphometric data. Hum. Brain Mapp. 42, 2332–2346 (2021b).
Google Scholar
-
Beheshti, I. et al. Predicting brain age using machine learning algorithms: a comprehensive evaluation. IEEE J. Biomed. Health Inf. 26, 1432–1440 (2022).
Google Scholar
-
Lee, W. H. et al. Brain age prediction in schizophrenia: does the choice of machine learning algorithm matter? Psychiatry Res. Neuroimaging 310, 111270 (2021).
Google Scholar
-
Lee, W. H. The choice of machine learning algorithms impacts the association between brain-predicted age difference and cognitive function. Mathematics 11, 1229 (2023).
Google Scholar
-
Cole, J. H. et al. Predicting brain age with deep learning from raw imaging data results in a reliable and heritable biomarker. NeuroImage 163, 115–124 (2017).
Google Scholar
-
Jonsson, B. A. et al. Brain age prediction using deep learning uncovers associated sequence variants. Nat. Commun. 10, 5409 (2019).
Google Scholar
-
Peng, H., Gong, W., Beckmann, C. F., Vedaldi, A. & Smith, S. M. Accurate brain age prediction with lightweight deep neural networks. Med. Image Anal. 68, 101871 (2021).
Google Scholar
-
Hahn, T. et al. An uncertainty-aware, shareable, and transparent neural network architecture for brain-age modeling. Sci. Adv. 8, eabg9471 (2022).
Google Scholar
-
Leonardsen, E. H. et al. Deep neural networks learn general and clinically relevant representations of the ageing brain. NeuroImage 256, 119210 (2022).
Google Scholar
-
Wood, D. A. et al. Accurate brain‐age models for routine clinical MRI examinations. NeuroImage 249, 118871 (2022).
Google Scholar
-
Brown, T. B. et al. Language models are few-shot learners. In 34th Conference on Neural Information Processing Systems (NeurIPS, 2020).
-
Esser, P., Rombach, R., Blattman, A. & Ommer, B. ImageBART: bidirectional context with multinomial diffusion for autoregressive image synthesis. In 35th Conference on Neural Information Processing Systems (NeurIPS, 2021).
-
He, S., Grant, P. E. & Ou, Y. Global–local transformer for brain age estimation. IEEE Trans. Med. Imaging 41, 213–224 (2022).
Google Scholar
-
Cai, H., Gao, Y. & Liu, M. Graph transformer geometric learning of brain networks using multimodal MR images for brain age estimation. IEEE Trans. Med. Imaging 42, 456–466 (2023).
Google Scholar
-
Pinaya, W. H. L. et al. Brain imaging generation with latent diffusion models. Preprint at https://arxiv.org/abs/2209.07162 (2022).
-
Tanveer, M. et al. Deep learning for brain age estimation: a systematic review. Inf. Fusion 96, 130–143 (2023).
Google Scholar
-
Kaufmann, T. et al. Common brain disorders are associated with heritable patterns of apparent aging of the brain. Nat. Neurosci. 22, 1617–1623 (2019).
Google Scholar
-
Popescu, S. G., Glocker, B., Sharp, D. J. & Cole, J. H. Local brain-age: a U-Net model. Front. Aging Neurosci. 13, 761954 (2021).
Google Scholar
-
Le, T. T. et al. A nonlinear simulation framework supports adjusting for age when analyzing BrainAGE. Front. Aging Neurosci. 10, 317 (2018).
Google Scholar
-
Liang, H., Zhang, F. & Niu, X. Investigating systematic bias in brain age estimation with application to post-traumatic stress disorders. Hum. Brain Mapp. 40, 3143–3152 (2019).
Google Scholar
-
de Lange, A.-M. G. & Cole, J. H. Correction procedures in brain-age prediction. Neuroimage Clin. 26, 102229 (2020).
Google Scholar
-
de Lange, A.-M. G. et al. Mind the gap: performance metric evaluation in brain-age prediction. Hum. Brain Mapp. 43, 3113–3129 (2022).
Google Scholar
-
More, S. et al. Brain-age prediction: a systematic comparison of machine learning workflows. NeuroImage 270, 119947 (2023).
Google Scholar
-
Dular, L. & Špiclin, Ž. BASE: brain age standardized evaluation. NeuroImage 285, 120469 (2024).
Google Scholar
-
Dufumier, B. et al. OpenBHB: a large-scale multi-site brain MRI data-set for age prediction and debiasing. NeuroImage 263, 119637 (2022).
Google Scholar
-
Dörfel, R. P. et al. Prediction of brain age using structural magnetic resonance imaging: a comparison of accuracy and test–retest reliability of publicly available software packages. Hum. Brain Mapp. 44, 6139–6148 (2023).
Google Scholar
-
Jirsaraie, R. J. et al. A systematic review of multimodal brain age studies: uncovering a divergence between model accuracy and utility. Patterns 4, 100712 (2023).
Google Scholar
-
Jirsaraie, R. J. et al. Benchmarking the generalizability of brain age models: challenges posed by scanner variance and prediction bias. Hum. Brain Mapp. 44, 1118–1128 (2023).
Google Scholar
-
Vidal-Pineiro, D. et al. Individual variations in ‘brain age’ relate to early-life factors more than to longitudinal brain change. eLife 10, e69995 (2021).
Google Scholar
-
Antonopoulos, G. et al. A systematic comparison of VBM pipelines and their application to age prediction. NeuroImage 279, 120292 (2023).
Google Scholar
-
Korbmacher, M. et al. Considerations on brain age predictions from repeatedly sampled data across time. Brain Behav. 13, e3219 (2023).
Google Scholar
-
Bashyam, V. M. et al. Deep generative medical image harmonization for improving cross‐site generalization in deep learning predictors. J. Magn. Reson. Imaging 55, 908–916 (2022).
Google Scholar
-
Pomponio, R. et al. Harmonization of large MRI datasets for the analysis of brain imaging patterns throughout the lifespan. NeuroImage 208, 116450 (2020).
Google Scholar
-
Dular, L. & Špiclin, Ž. in Predictive Intelligence in Medicine. PRIME 2021 Lecture Notes in Computer Science Vol. 12928 (eds Rekik, I. et al.) 243–254 (Springer, 2021); https://doi.org/10.1007/978-3-030-87602-9_23
-
Elliott, M. L. et al. Brain-age in midlife is associated with accelerated biological aging and cognitive decline in a longitudinal birth cohort. Mol. Psychiatry 26, 3829–3838 (2021).
Google Scholar
-
Gautherot, M. et al. Longitudinal analysis of brain-predicted age in amnestic and non-amnestic sporadic early-onset Alzheimer’s disease. Front. Aging Neurosci. 13, 729635 (2021).
Google Scholar
-
Gaser, C. et al. BrainAGE in mild cognitive impaired patients: predicting the conversion to Alzheimer’s disease. PLoS ONE 8, e67346 (2013).
Google Scholar
-
Lombardi, A. et al. Explainable deep learning for personalized age prediction with brain morphology. Front. Neurosci. 15, 674055 (2021).
Google Scholar
-
Yin, C. et al. Anatomically interpretable deep learning of brain age captures domain-specific cognitive impairment. Proc. Natl Acad. Sci. USA 120, e2214634120 (2023).
Google Scholar
-
Hesse, L. S., Dinsdale, N. K. & Namburete, A. I. L. Prototype learning for explainable brain age prediction. Preprint at https://arxiv.org/abs/2306.09858 (2023).
-
Palma, M., Tavakoli, S., Brettschneider, J. & Nichols, T. E. Quantifying uncertainty in brain-predicted age using scalar-on-image quantile regression. NeuroImage 219, 116938 (2020).
Google Scholar
-
Wei, R. et al. Brain age gap in neuromyelitis optica spectrum disorders and multiple sclerosis. J. Neurol. Neurosurg. Psychiatry 94, 31–37 (2023).
Google Scholar
-
Dafflon, J. et al. An automated machine learning approach to predict brain age from cortical anatomical measures. Hum. Brain Mapp. 41, 3555–3566 (2020).
Google Scholar
-
Butler, E. R. et al. Pitfalls in brain age analyses. Hum. Brain Mapp. 42, 4092–4101 (2021).
Google Scholar
-
Wagen, A. Z. et al. Life course, genetic, and neuropathological associations with brain age in the 1946 British Birth Cohort: a population-based study. Lancet Healthy Longev. 3, e607–e616 (2022).
Google Scholar
-
Bethlehem, R. A. I. et al. Brain charts for the human lifespan. Nature 604, 525–533 (2022).
Google Scholar
-
Marquand, A. F., Rezek, I., Buitelaar, J. & Beckmann, C. F. Understanding heterogeneity in clinical cohorts using normative models: beyond case-control studies. Biol. Psychiatry 80, 552–561 (2016).
Google Scholar
-
Marquand, A. F. et al. Conceptualizing mental disorders as deviations from normative functioning. Mol. Psychiatry 24, 1415–1424 (2019).
Google Scholar
-
Valdes-Hernandez, P. A. et al. Toward MR protocol-agnostic, unbiased brain age predicted from clinical-grade MRIs. Sci. Rep. 13, 19570 (2023).
Google Scholar
-
Billot, B. et al. SynthSeg: segmentation of brain MRI scans of any contrast and resolution without retraining. Med. Image Anal. 86, 102789 (2023).
Google Scholar
-
Pérez-García, F., Sparks, R. & Ourselin, S. TorchIO: a Python library for efficient loading, preprocessing, augmentation and patch-based sampling of medical images in deep learning. Comput. Methods Prog. Biomed. 208, 106236 (2021).
Google Scholar
-
Iglesias et al. Joint super-resolution and synthesis of 1 mm isotropic MP-RAGE volumes from clinical MRI exams with scans of different orientation, resolution and contrast. NeuroImage 237, 118206 (2021).
Google Scholar
-
Lin, H. et al. Low-field magnetic resonance image enhancement via stochastic image quality transfer. Med. Image Anal. 87, 102807 (2023).
Google Scholar
-
Kimberly, W. T. et al. Brain imaging with portable low-field MRI. Nat. Rev. Bioeng. 1, 617–630 (2023).
Google Scholar
-
Silva, S., Altmann, A., Gutman, B. & Lorenzi, M. in Domain Adaptation and Representation Transfer, and Distributed and Collaborative Learning. DART DCL 2020 2020. Lecture Notes in Computer Science Vol. 12444 (eds Albarqouni, S. et al.) 201–210 (Springer, 2020); https://doi.org/10.1007/978-3-030-60548-3_20
-
Cheshmi, S. S., Mahyar, A., Soroush, A., Rezvani, Z. & Farahani, B. Brain age estimation using structural MRI: a clustered federated learning approach. In 2023 IEEE International Conference on Omni-layer Intelligent Systems (COINS) 1–6 (IEEE, 2023).
-
Li, T., Sahu, A. K., Talwalkar, A. & Smith, V. Federated learning: challenges, methods, and future directions. IEEE Signal Process Mag. 37, 50–60 (2020).
-
Han, L. K. M. et al. Brain aging in major depressive disorder: results from the ENIGMA major depressive disorder working group. Mol. Psychiatry 26, 5124–5139 (2021).
Google Scholar
-
Han, L. K. M. et al. A large-scale ENIGMA multisite replication study of brain age in depression. Neuroimage Rep. 2, 100149 (2022).
Google Scholar
-
Constantinides, C. et al. Brain ageing in schizophrenia: evidence from 26 international cohorts via the ENIGMA schizophrenia consortium. Mol. Psychiatry 28, 1201–1209 (2023).
Google Scholar
-
Bell, C. G. et al. DNA methylation aging clocks: challenges and recommendations. Genome Biol. 20, 249 (2019).
Google Scholar
-
Largent, E. A., Stites, S. D., Harkins, K. & Karlawish, J. ‘That would be dreadful’: the ethical, legal, and social challenges of sharing your Alzheimer’s disease biomarker and genetic testing results with others. J. Law Biosci. 8, lsab004 (2021).
Google Scholar
-
Snowdon, D. A. Healthy aging and dementia: findings from the Nun study. Ann. Intern. Med. 139, 450–454 (2003).
Google Scholar
-
Cabeza, R. et al. Maintenance, reserve and compensation: the cognitive neuroscience of healthy ageing. Nat. Rev. Neurosci. 19, 701–710 (2018).
Google Scholar
-
Stern, Y., Barnes, C. A., Grady, C., Jones, R. N. & Raz, N. Brain reserve, cognitive reserve, compensation, and maintenance: operationalization, validity, and mechanisms of cognitive resilience. Neurobiol. Aging 83, 124–129 (2019).
Google Scholar
Acknowledgements
We gratefully acknowledge R. Dahnke for providing insightful comments on the paper and helping with the visualization. C.G. and P.K. were supported by Carl Zeiss Stiftung as a part of the IMPULS project (IMPULS P2019-01-006), the Federal Ministry of Science and Education (BMBF) under the frame of ERA PerMed (Pattern-Cog ERAPERMED2021-127) and the Marie Skłodowska-Curie Innovative Training Network (SmartAge 859890 H2020-MSCA-ITN2019).
Author information
Authors and Affiliations
Contributions
All authors discussed the content, reviewed and edited the entire paper.
Corresponding author
Ethics declarations
Competing interests
J.H.C. is an advisor to and shareholder in BrainKey and ClaritasHealthPTE.
Peer review
Peer review information
Nature Computational Science thanks Iman Beheshti and the other, anonymous, reviewer(s) for their contribution to the peer review of this work. Primary Handling Editor: Ananya Rastogi, in collaboration with the Nature Computational Science team.
Additional information
Publisher’s note Springer Nature remains neutral with regard to jurisdictional claims in published maps and institutional affiliations.
Rights and permissions
Springer Nature or its licensor (e.g. a society or other partner) holds exclusive rights to this article under a publishing agreement with the author(s) or other rightsholder(s); author self-archiving of the accepted manuscript version of this article is solely governed by the terms of such publishing agreement and applicable law.
Reprints and permissions
About this article
Cite this article
Gaser, C., Kalc, P. & Cole, J.H. A perspective on brain-age estimation and its clinical promise.
Nat Comput Sci (2024). https://doi.org/10.1038/s43588-024-00659-8
-
Received: 11 August 2023
-
Accepted: 12 June 2024
-
Published: 24 July 2024
-
DOI: https://doi.org/10.1038/s43588-024-00659-8