Abstract
Large-scale drug discovery and repurposing is challenging. Identifying the mechanism of action (MOA) is crucial, yet current approaches are costly and low-throughput. Here we present an approach for MOA identification by profiling changes in mitochondrial phenotypes. By temporally imaging mitochondrial morphology and membrane potential, we established a pipeline for monitoring time-resolved mitochondrial images, resulting in a dataset comprising 570,096 single-cell images of cells exposed to 1,068 United States Food and Drug Administration-approved drugs. A deep learning model named MitoReID, using a re-identification (ReID) framework and an Inflated 3D ResNet backbone, was developed. It achieved 76.32% Rank-1 and 65.92% mean average precision on the testing set and successfully identified the MOAs for six untrained drugs on the basis of mitochondrial phenotype. Furthermore, MitoReID identified cyclooxygenase-2 inhibition as the MOA of the natural compound epicatechin in tea, which was successfully validated in vitro. Our approach thus provides an automated and cost-effective alternative for target identification that could accelerate large-scale drug discovery and repurposing.
This is a preview of subscription content, access via your institution
Access options
style{display:none!important}.LiveAreaSection-193358632 *{align-content:stretch;align-items:stretch;align-self:auto;animation-delay:0s;animation-direction:normal;animation-duration:0s;animation-fill-mode:none;animation-iteration-count:1;animation-name:none;animation-play-state:running;animation-timing-function:ease;azimuth:center;backface-visibility:visible;background-attachment:scroll;background-blend-mode:normal;background-clip:borderBox;background-color:transparent;background-image:none;background-origin:paddingBox;background-position:0 0;background-repeat:repeat;background-size:auto auto;block-size:auto;border-block-end-color:currentcolor;border-block-end-style:none;border-block-end-width:medium;border-block-start-color:currentcolor;border-block-start-style:none;border-block-start-width:medium;border-bottom-color:currentcolor;border-bottom-left-radius:0;border-bottom-right-radius:0;border-bottom-style:none;border-bottom-width:medium;border-collapse:separate;border-image-outset:0s;border-image-repeat:stretch;border-image-slice:100%;border-image-source:none;border-image-width:1;border-inline-end-color:currentcolor;border-inline-end-style:none;border-inline-end-width:medium;border-inline-start-color:currentcolor;border-inline-start-style:none;border-inline-start-width:medium;border-left-color:currentcolor;border-left-style:none;border-left-width:medium;border-right-color:currentcolor;border-right-style:none;border-right-width:medium;border-spacing:0;border-top-color:currentcolor;border-top-left-radius:0;border-top-right-radius:0;border-top-style:none;border-top-width:medium;bottom:auto;box-decoration-break:slice;box-shadow:none;box-sizing:border-box;break-after:auto;break-before:auto;break-inside:auto;caption-side:top;caret-color:auto;clear:none;clip:auto;clip-path:none;color:initial;column-count:auto;column-fill:balance;column-gap:normal;column-rule-color:currentcolor;column-rule-style:none;column-rule-width:medium;column-span:none;column-width:auto;content:normal;counter-increment:none;counter-reset:none;cursor:auto;display:inline;empty-cells:show;filter:none;flex-basis:auto;flex-direction:row;flex-grow:0;flex-shrink:1;flex-wrap:nowrap;float:none;font-family:initial;font-feature-settings:normal;font-kerning:auto;font-language-override:normal;font-size:medium;font-size-adjust:none;font-stretch:normal;font-style:normal;font-synthesis:weight style;font-variant:normal;font-variant-alternates:normal;font-variant-caps:normal;font-variant-east-asian:normal;font-variant-ligatures:normal;font-variant-numeric:normal;font-variant-position:normal;font-weight:400;grid-auto-columns:auto;grid-auto-flow:row;grid-auto-rows:auto;grid-column-end:auto;grid-column-gap:0;grid-column-start:auto;grid-row-end:auto;grid-row-gap:0;grid-row-start:auto;grid-template-areas:none;grid-template-columns:none;grid-template-rows:none;height:auto;hyphens:manual;image-orientation:0deg;image-rendering:auto;image-resolution:1dppx;ime-mode:auto;inline-size:auto;isolation:auto;justify-content:flexStart;left:auto;letter-spacing:normal;line-break:auto;line-height:normal;list-style-image:none;list-style-position:outside;list-style-type:disc;margin-block-end:0;margin-block-start:0;margin-bottom:0;margin-inline-end:0;margin-inline-start:0;margin-left:0;margin-right:0;margin-top:0;mask-clip:borderBox;mask-composite:add;mask-image:none;mask-mode:matchSource;mask-origin:borderBox;mask-position:0 0;mask-repeat:repeat;mask-size:auto;mask-type:luminance;max-height:none;max-width:none;min-block-size:0;min-height:0;min-inline-size:0;min-width:0;mix-blend-mode:normal;object-fit:fill;object-position:50% 50%;offset-block-end:auto;offset-block-start:auto;offset-inline-end:auto;offset-inline-start:auto;opacity:1;order:0;orphans:2;outline-color:initial;outline-offset:0;outline-style:none;outline-width:medium;overflow:visible;overflow-wrap:normal;overflow-x:visible;overflow-y:visible;padding-block-end:0;padding-block-start:0;padding-bottom:0;padding-inline-end:0;padding-inline-start:0;padding-left:0;padding-right:0;padding-top:0;page-break-after:auto;page-break-before:auto;page-break-inside:auto;perspective:none;perspective-origin:50% 50%;pointer-events:auto;position:static;quotes:initial;resize:none;right:auto;ruby-align:spaceAround;ruby-merge:separate;ruby-position:over;scroll-behavior:auto;scroll-snap-coordinate:none;scroll-snap-destination:0 0;scroll-snap-points-x:none;scroll-snap-points-y:none;scroll-snap-type:none;shape-image-threshold:0;shape-margin:0;shape-outside:none;tab-size:8;table-layout:auto;text-align:initial;text-align-last:auto;text-combine-upright:none;text-decoration-color:currentcolor;text-decoration-line:none;text-decoration-style:solid;text-emphasis-color:currentcolor;text-emphasis-position:over right;text-emphasis-style:none;text-indent:0;text-justify:auto;text-orientation:mixed;text-overflow:clip;text-rendering:auto;text-shadow:none;text-transform:none;text-underline-position:auto;top:auto;touch-action:auto;transform:none;transform-box:borderBox;transform-origin:50% 50%0;transform-style:flat;transition-delay:0s;transition-duration:0s;transition-property:all;transition-timing-function:ease;vertical-align:baseline;visibility:visible;white-space:normal;widows:2;width:auto;will-change:auto;word-break:normal;word-spacing:normal;word-wrap:normal;writing-mode:horizontalTb;z-index:auto;-webkit-appearance:none;-moz-appearance:none;-ms-appearance:none;appearance:none;margin:0}.LiveAreaSection-193358632{width:100%}.LiveAreaSection-193358632 .login-option-buybox{display:block;width:100%;font-size:17px;line-height:30px;color:#222;padding-top:30px;font-family:Harding,Palatino,serif}.LiveAreaSection-193358632 .additional-access-options{display:block;font-weight:700;font-size:17px;line-height:30px;color:#222;font-family:Harding,Palatino,serif}.LiveAreaSection-193358632 .additional-login>li:not(:first-child)::before{transform:translateY(-50%);content:””;height:1rem;position:absolute;top:50%;left:0;border-left:2px solid #999}.LiveAreaSection-193358632 .additional-login>li:not(:first-child){padding-left:10px}.LiveAreaSection-193358632 .additional-login>li{display:inline-block;position:relative;vertical-align:middle;padding-right:10px}.BuyBoxSection-683559780{display:flex;flex-wrap:wrap;flex:1;flex-direction:row-reverse;margin:-30px -15px 0}.BuyBoxSection-683559780 .box-inner{width:100%;height:100%;padding:30px 5px;display:flex;flex-direction:column;justify-content:space-between}.BuyBoxSection-683559780 p{margin:0}.BuyBoxSection-683559780 .readcube-buybox{background-color:#f3f3f3;flex-shrink:1;flex-grow:1;flex-basis:255px;background-clip:content-box;padding:0 15px;margin-top:30px}.BuyBoxSection-683559780 .subscribe-buybox{background-color:#f3f3f3;flex-shrink:1;flex-grow:4;flex-basis:300px;background-clip:content-box;padding:0 15px;margin-top:30px}.BuyBoxSection-683559780 .subscribe-buybox-nature-plus{background-color:#f3f3f3;flex-shrink:1;flex-grow:4;flex-basis:100%;background-clip:content-box;padding:0 15px;margin-top:30px}.BuyBoxSection-683559780 .title-readcube,.BuyBoxSection-683559780 .title-buybox{display:block;margin:0;margin-right:10%;margin-left:10%;font-size:24px;line-height:32px;color:#222;text-align:center;font-family:Harding,Palatino,serif}.BuyBoxSection-683559780 .title-asia-buybox{display:block;margin:0;margin-right:5%;margin-left:5%;font-size:24px;line-height:32px;color:#222;text-align:center;font-family:Harding,Palatino,serif}.BuyBoxSection-683559780 .asia-link{color:#069;cursor:pointer;text-decoration:none;font-size:1.05em;font-family:-apple-system,BlinkMacSystemFont,”Segoe UI”,Roboto,Oxygen-Sans,Ubuntu,Cantarell,”Helvetica Neue”,sans-serif;line-height:1.05em6}.BuyBoxSection-683559780 .access-readcube{display:block;margin:0;margin-right:10%;margin-left:10%;font-size:14px;color:#222;padding-top:10px;text-align:center;font-family:-apple-system,BlinkMacSystemFont,”Segoe UI”,Roboto,Oxygen-Sans,Ubuntu,Cantarell,”Helvetica Neue”,sans-serif;line-height:20px}.BuyBoxSection-683559780 ul{margin:0}.BuyBoxSection-683559780 .link-usp{display:list-item;margin:0;margin-left:20px;padding-top:6px;list-style-position:inside}.BuyBoxSection-683559780 .link-usp span{font-size:14px;color:#222;font-family:-apple-system,BlinkMacSystemFont,”Segoe UI”,Roboto,Oxygen-Sans,Ubuntu,Cantarell,”Helvetica Neue”,sans-serif;line-height:20px}.BuyBoxSection-683559780 .access-asia-buybox{display:block;margin:0;margin-right:5%;margin-left:5%;font-size:14px;color:#222;padding-top:10px;text-align:center;font-family:-apple-system,BlinkMacSystemFont,”Segoe UI”,Roboto,Oxygen-Sans,Ubuntu,Cantarell,”Helvetica Neue”,sans-serif;line-height:20px}.BuyBoxSection-683559780 .access-buybox{display:block;margin:0;margin-right:10%;margin-left:10%;font-size:14px;color:#222;opacity:.8px;padding-top:10px;text-align:center;font-family:-apple-system,BlinkMacSystemFont,”Segoe UI”,Roboto,Oxygen-Sans,Ubuntu,Cantarell,”Helvetica Neue”,sans-serif;line-height:20px}.BuyBoxSection-683559780 .price-buybox{display:block;font-size:30px;color:#222;font-family:-apple-system,BlinkMacSystemFont,”Segoe UI”,Roboto,Oxygen-Sans,Ubuntu,Cantarell,”Helvetica Neue”,sans-serif;padding-top:30px;text-align:center}.BuyBoxSection-683559780 .price-buybox-to{display:block;font-size:30px;color:#222;font-family:-apple-system,BlinkMacSystemFont,”Segoe UI”,Roboto,Oxygen-Sans,Ubuntu,Cantarell,”Helvetica Neue”,sans-serif;text-align:center}.BuyBoxSection-683559780 .price-info-text{font-size:16px;padding-right:10px;color:#222;font-family:-apple-system,BlinkMacSystemFont,”Segoe UI”,Roboto,Oxygen-Sans,Ubuntu,Cantarell,”Helvetica Neue”,sans-serif}.BuyBoxSection-683559780 .price-value{font-size:30px;font-family:-apple-system,BlinkMacSystemFont,”Segoe UI”,Roboto,Oxygen-Sans,Ubuntu,Cantarell,”Helvetica Neue”,sans-serif}.BuyBoxSection-683559780 .price-per-period{font-family:-apple-system,BlinkMacSystemFont,”Segoe UI”,Roboto,Oxygen-Sans,Ubuntu,Cantarell,”Helvetica Neue”,sans-serif}.BuyBoxSection-683559780 .price-from{font-size:14px;padding-right:10px;color:#222;font-family:-apple-system,BlinkMacSystemFont,”Segoe UI”,Roboto,Oxygen-Sans,Ubuntu,Cantarell,”Helvetica Neue”,sans-serif;line-height:20px}.BuyBoxSection-683559780 .issue-buybox{display:block;font-size:13px;text-align:center;color:#222;font-family:-apple-system,BlinkMacSystemFont,”Segoe UI”,Roboto,Oxygen-Sans,Ubuntu,Cantarell,”Helvetica Neue”,sans-serif;line-height:19px}.BuyBoxSection-683559780 .no-price-buybox{display:block;font-size:13px;line-height:18px;text-align:center;padding-right:10%;padding-left:10%;padding-bottom:20px;padding-top:30px;color:#222;font-family:-apple-system,BlinkMacSystemFont,”Segoe UI”,Roboto,Oxygen-Sans,Ubuntu,Cantarell,”Helvetica Neue”,sans-serif}.BuyBoxSection-683559780 .vat-buybox{display:block;margin-top:5px;margin-right:20%;margin-left:20%;font-size:11px;color:#222;padding-top:10px;padding-bottom:15px;text-align:center;font-family:-apple-system,BlinkMacSystemFont,”Segoe UI”,Roboto,Oxygen-Sans,Ubuntu,Cantarell,”Helvetica Neue”,sans-serif;line-height:17px}.BuyBoxSection-683559780 .tax-buybox{display:block;width:100%;color:#222;padding:20px 16px;text-align:center;font-family:-apple-system,BlinkMacSystemFont,”Segoe UI”,Roboto,Oxygen-Sans,Ubuntu,Cantarell,”Helvetica Neue”,sans-serif;line-height:NaNpx}.BuyBoxSection-683559780 .button-container{display:flex;padding-right:20px;padding-left:20px;justify-content:center}.BuyBoxSection-683559780 .button-container>*{flex:1px}.BuyBoxSection-683559780 .button-container>a:hover,.Button-505204839:hover,.Button-1078489254:hover,.Button-2737859108:hover{text-decoration:none}.BuyBoxSection-683559780 .btn-secondary{background:#fff}.BuyBoxSection-683559780 .button-asia{background:#069;border:1px solid #069;border-radius:0;cursor:pointer;display:block;padding:9px;outline:0;text-align:center;text-decoration:none;min-width:80px;margin-top:75px}.BuyBoxSection-683559780 .button-label-asia,.ButtonLabel-3869432492,.ButtonLabel-3296148077,.ButtonLabel-1636778223{display:block;color:#fff;font-size:17px;line-height:20px;font-family:-apple-system,BlinkMacSystemFont,”Segoe UI”,Roboto,Oxygen-Sans,Ubuntu,Cantarell,”Helvetica Neue”,sans-serif;text-align:center;text-decoration:none;cursor:pointer}.Button-505204839,.Button-1078489254,.Button-2737859108{background:#069;border:1px solid #069;border-radius:0;cursor:pointer;display:block;padding:9px;outline:0;text-align:center;text-decoration:none;min-width:80px;max-width:320px;margin-top:20px}.Button-505204839 .btn-secondary-label,.Button-1078489254 .btn-secondary-label,.Button-2737859108 .btn-secondary-label{color:#069}
/* style specs end */
Access Nature and 54 other Nature Portfolio journals
Get Nature+, our best-value online-access subscription
$29.99 / 30 days
cancel any time
Subscribe to this journal
Receive 12 digital issues and online access to articles
$99.00 per year
only $8.25 per issue
Buy this article
- Purchase on SpringerLink
- Instant access to full article PDF
Prices may be subject to local taxes which are calculated during checkout
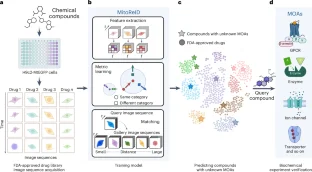
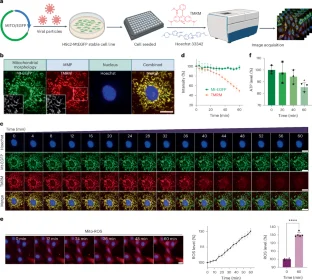
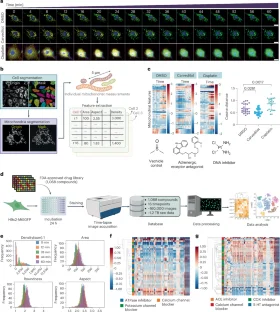
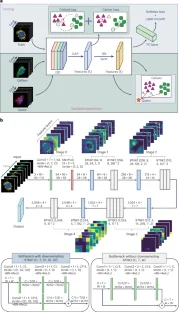
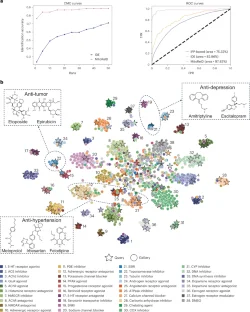
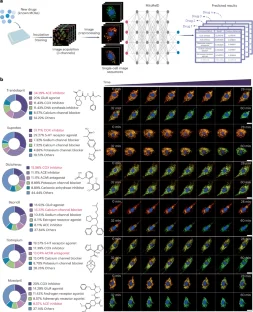
Data availability
The dataset used to train the model and all the model weights are available via Zenodo64 at https://doi.org/10.5281/zenodo.12730131. Molecular structural data were obtained from the PDB database (http://www.rcsb.org/). Annotations of FDA-approved drugs were collected from Drugbank at https://go.drugbank.com/, ChEMBL at https://www.ebi.ac.uk/chembl/ and the Drug Repurposing Hub at https://www.broadinstitute.org/drug-repurposing-hub. Source Data are provided with this paper.
Code availability
Codes can be accessed via GitHub at https://github.com/liweim/MitoReID. A stable version of the code used in this work is available via Zenodo65 at https://doi.org/10.5281/zenodo.12726571.
References
-
DiMasi, J. A., Grabowski, H. G. & Hansen, R. W. Innovation in the pharmaceutical industry: new estimates of R&D costs. J. Health Econ. 47, 20–33 (2016).
Google Scholar
-
Swinney, D. C. & Anthony, J. How were new medicines discovered? Nat. Rev. Drug Discov. 10, 507–519 (2011).
Google Scholar
-
Rask-Andersen, M., Almen, M. S. & Schioth, H. B. Trends in the exploitation of novel drug targets. Nat. Rev. Drug Discov. 10, 579–590 (2011).
Google Scholar
-
Lee, H. & Lee, J. W. Target identification for biologically active small molecules using chemical biology approaches. Arch. Pharm. Res. 39, 1193–1201 (2016).
Google Scholar
-
Ha, J. et al. Recent advances in identifying protein targets in drug discovery. Cell Chem. Biol. 28, 394–423 (2021).
Google Scholar
-
Boutros, M., Heigwer, F. & Laufer, C. Microscopy-based high-content screening. Cell 163, 1314–1325 (2015).
Google Scholar
-
Chandrasekaran, S. N. et al. Image-based profiling for drug discovery: due for a machine-learning upgrade? Nat. Rev. Drug Discov. 20, 145–159 (2021).
Google Scholar
-
Caicedo, J. C. et al. Data-analysis strategies for image-based cell profiling. Nat. Methods 14, 849–863 (2017).
Google Scholar
-
Way, G. P. et al. Morphology and gene expression profiling provide complementary information for mapping cell state. Cell Syst. 13, 911–923 e9 (2022).
Google Scholar
-
Funk, L. et al. The phenotypic landscape of essential human genes. Cell 185, 4634–4653 e22 (2022).
Google Scholar
-
Thyme, S. B. et al. Phenotypic landscape of schizophrenia-associated genes defines candidates and their shared functions. Cell 177, 478–491.e20 (2019).
Google Scholar
-
Simm, J. et al. Repurposing high-throughput image assays enables biological activity prediction for drug discovery. Cell Chem. Biol. 25, 611–618.e3 (2018).
Google Scholar
-
Nyffeler, J. et al. Bioactivity screening of environmental chemicals using imaging-based high-throughput phenotypic profiling. Toxicol. Appl. Pharmacol. 389, 114876 (2020).
Google Scholar
-
Pegoraro, G. & Misteli, T. High-throughput imaging for the discovery of cellular mechanisms of disease. Trends Genet. 33, 604–615 (2017).
Google Scholar
-
Bray, M. A. et al. Cell Painting, a high-content image-based assay for morphological profiling using multiplexed fluorescent dyes. Nat. Protoc. 11, 1757–1774 (2016).
Google Scholar
-
Hofmarcher, M. et al. Accurate prediction of biological assays with high-throughput microscopy images and convolutional networks. J. Chem. Inf. Model. 59, 1163–1171 (2019).
Google Scholar
-
Perlman, Z. E. et al. Multidimensional drug profiling by automated microscopy. Science 306, 1194–1198 (2004).
-
Lin, J. R., Fallahi-Sichani, M. & Sorger, P. K. Highly multiplexed imaging of single cells using a high-throughput cyclic immunofluorescence method. Nat. Commun. 6, 8390 (2015).
Google Scholar
-
Nunnari, J. & Suomalainen, A. Mitochondria: in sickness and in health. Cell 148, 1145–1159 (2012).
Google Scholar
-
Russell, O. M. et al. Mitochondrial diseases: hope for the future. Cell 181, 168–188 (2020).
Google Scholar
-
Jangili, P. et al. DNA-damage-response-targeting mitochondria-activated multifunctional prodrug strategy for self-defensive tumor therapy. Angew. Chem. Int. Ed. 61, e202117075 (2022).
Google Scholar
-
Carelli, V. & Chan, D. C. Mitochondrial DNA: impacting central and peripheral nervous systems. Neuron 84, 1126–1142 (2014).
Google Scholar
-
Glancy, B. Visualizing mitochondrial form and function within the cell. Trends Mol. Med. 26, 58–70 (2020).
Google Scholar
-
Cretin, E. et al. High-throughput screening identifies suppressors of mitochondrial fragmentation in OPA1 fibroblasts. EMBO Mol. Med. 13, e13579 (2021).
Google Scholar
-
Varkuti, B. H. et al. Neuron-based high-content assay and screen for CNS active mitotherapeutics. Sci. Adv. 6, eaaw8702 (2020).
Google Scholar
-
Chandrasekharan, A. et al. A high-throughput real-time in vitro assay using mitochondrial targeted roGFP for screening of drugs targeting mitochondria. Redox Biol. 20, 379–389 (2019).
Google Scholar
-
Iannetti, E. F. et al. Multiplexed high-content analysis of mitochondrial morphofunction using live-cell microscopy. Nat. Protoc. 11, 1693–1710 (2016).
Google Scholar
-
Pereira, G. C. et al. Drug-induced cardiac mitochondrial toxicity and protection: from doxorubicin to carvedilol. Curr. Pharm. Des. 17, 2113–2129 (2011).
Google Scholar
-
Varga, Z. V. et al. Drug-induced mitochondrial dysfunction and cardiotoxicity. Am. J. Physiol. Heart. Circ. Physiol. 309, H1453–H1467 (2015).
Google Scholar
-
Stringer, C. et al. Cellpose: a generalist algorithm for cellular segmentation. Nat. Methods 18, 100–106 (2021).
Google Scholar
-
Cao, M. et al. Plant exosome nanovesicles (PENs): green delivery platforms. Mater. Horiz. 10, 3879–3894 (2023).
Google Scholar
-
Zhang, D. et al. Microalgae-based oral microcarriers for gut microbiota homeostasis and intestinal protection in cancer radiotherapy. Nat. Commun. 13, 1413 (2022).
Google Scholar
-
Ji, X. et al. Capturing functional two-dimensional nanosheets from sandwich-structure vermiculite for cancer theranostics. Nat. Commun. 12, 1124 (2021).
Google Scholar
-
Zhong, D. et al. Orally deliverable strategy based on microalgal biomass for intestinal disease treatment. Sci. Adv. 7, eabi9265 (2021).
Google Scholar
-
Chen, F. et al. The V-ATPases in cancer and cell death. Cancer Gene Ther. 29, 1529–1541 (2022).
Google Scholar
-
Rizzuto, R. et al. Mitochondria as sensors and regulators of calcium signalling. Nat. Rev. Mol. Cell Biol. 13, 566–578 (2012).
Google Scholar
-
Giorgi, C., Marchi, S. & Pinton, P. The machineries, regulation and cellular functions of mitochondrial calcium. Nat. Rev. Mol. Cell Biol. 19, 713–730 (2018).
Google Scholar
-
Schmitt, N., Grunnet, M. & Olesen, S. P. Cardiac potassium channel subtypes: new roles in repolarization and arrhythmia. Physiol. Rev. 94, 609–653 (2014).
Google Scholar
-
Lei, M. et al. Modernized classification of cardiac antiarrhythmic drugs. Circulation 138, 1879–1896 (2018).
Google Scholar
-
Zheng, Z., Zheng, L. & Yang, Y. A discriminatively learned CNN embedding for person reidentification. In ACM Transactions on Multimedia Computing, Communications, and Applications (TOMM) Vol. 14, 1–20 (ACM, 2017).
-
Luo, H. et al. Bag of tricks and a strong baseline for deep person re-identification. In Proc. IEEE/CVF Conference on Computer Vision and Pattern Recognition Workshops (IEEE, 2019).
-
Carreira, J. & A. Zisserman. Quo vadis, action recognition? A new model and the kinetics dataset. Proc. IEEE Conference on Computer Vision and Pattern Recognition 6299–6308 (IEEE, 2017).
-
Hermans, A., Beyer, L. & Leibe, B. In defense of the triplet loss for person re-identification. Preprint at https://arxiv.org/abs/1703.07737 (2017).
-
Wen, Y. et al. A discriminative feature learning approach for deep face recognition. In European Conference On Computer Vision 499–515 (Springer, 2016).
-
Szegedy, C. et al. Rethinking the inception architecture for computer vision. Proc. IEEE Conference On Computer Vision And Pattern Recognition 2818–2826 (2016).
-
Moon, H. & Phillips, P. J. Computational and performance aspects of PCA-based face-recognition algorithms. Perception 30, 303–321 (2001).
Google Scholar
-
Zheng, L. et al. Scalable person re-identification: a benchmark. In Proc. IEEE International Conference On Computer Vision 1116–1124 (IEEE, 2015).
-
Atanasov, A. G. et al. Natural products in drug discovery: advances and opportunities. Nat. Rev. Drug Discov. 20, 200–216 (2021).
Google Scholar
-
Zhou, J. et al. Graph neural networks: a review of methods and applications. AI Open 1, 57–81 (2020).
Google Scholar
-
Santos, R. et al. A comprehensive map of molecular drug targets. Nat. Rev. Drug Discov. 16, 19–34 (2017).
Google Scholar
-
Corsello, S. M. et al. The drug repurposing hub: a next-generation drug library and information resource. Nat. Med. 23, 405–408 (2017).
Google Scholar
-
Zdrazil, B. et al. The ChEMBL Database in 2023: a drug discovery platform spanning multiple bioactivity data types and time periods. Nucleic Acids Res. 52, D1180–D1192 (2024).
Google Scholar
-
Wishart, D. S. et al. DrugBank 5.0: a major update to the DrugBank database for 2018. Nucleic Acids Res. 46, D1074–D1082 (2018).
Google Scholar
-
MetaXpress v.6.6 https://www.moleculardevices.com/products/cellular-imaging-systems/high-content-analysis/metaxpress (Molecular Devices, 2020).
-
AutoDock v.4.2.6 https://autodock.scripps.edu/ (CCSB, 2014).
-
ChemOffice v.19.0 https://revvitysignals.com/products/research/chemdraw (Revvity Signals, 2019).
-
Goodsell, D. S. et al. RCSB Protein Data Bank: enabling biomedical research and drug discovery. Protein Sci. 29, 52–65 (2020).
Google Scholar
-
PyMOL v.2.5 https://pymol.org/ (Schrödinger, 2021).
-
Zhang, S. et al. Discovery of herbacetin as a novel SGK1 inhibitor to alleviate myocardial hypertrophy. Adv. Sci. 9, e2101485 (2022).
Google Scholar
-
He, K. et al. Deep residual learning for image recognition. Proc. IEEE Conference On Computer Vision And Pattern Recognition 770–778 (IEEE, 2016).
-
Schroff, F., Kalenichenko, D. & Philbin, P. FaceNet: a unified embedding for face recognition and clustering. In 2015 IEEE Conference on Computer Vision and Pattern Recognition (CVPR) (IEEE, 2015).
-
He, K. et al. Delving deep into rectifiers: surpassing human-level performance on imagenet classification. Proc. IEEE International Conference On Computer Vision 1026–1034 (IEEE, 2015).
-
LeCun, Y. et al. Backpropagation applied to handwritten ZIP Code recognition. Neural Comput. 1, 541–551 (1989).
Google Scholar
-
Li, W., Yu, M. & Wang, Y. Data for Deep Learning Large-Scale Drug Discovery and Repurposing (Zenodo, 2024); https://doi.org/10.5281/zenodo.12730131
-
Li, W. liweim/MitoReID: v1.0 (Zenodo, 2024); https://doi.org/10.5281/zenodo.12726571
Acknowledgements
We are grateful for the support from ZJU PII-Molecular Devices Joint Laboratory. We thank Zhejiang Lab for providing high-performance GPU servers for deep learning research. Images in the illustration were created using BioRender.com. Funding: National Key Research and Development Program of China (grant no. 2023YFC3502801 to Y.W.), National Natural Science Foundation of China (grant no. 82173941 to Y.W.), Fundamental Research Funds for Central Universities (grant no. 226-2024-00001 to Y.W.), ‘Pioneer’ and ‘Leading Goose’ R&D Program of Zhejiang (grant no. 2024C01020 to W.L.), Innovation Team and Talents Cultivation Program of National Administration of Traditional Chinese Medicine (grant no. ZYYCXTD-D-202002 to Y.W.).
Author information
Authors and Affiliations
Contributions
X.Z., Y.W. and Y.C. conceived the study. Y.W., X.Z., M.Y., Y.Y. and Y.Z. designed the experimental scheme. M.Y. collected the data. W.L. performed image data processing, and the model training and prediction. M.Y. and W.L. wrote the original draft of the manuscript, whereas Y.W., X.Z., V.M.L., L.X. and Y.C reviewed and edited it.
Corresponding authors
Ethics declarations
Competing interests
The authors declare that they have no competing interests.
Peer review
Peer review information
Nature Computational Science thanks Paul Czodrowski, Shibiao Wan, and the other, anonymous, reviewer(s) for their contribution to the peer review of this work. Primary Handling Editor: Kaitlin McCardle, in collaboration with the Nature Computational Science team.
Additional information
Publisher’s note Springer Nature remains neutral with regard to jurisdictional claims in published maps and institutional affiliations.
Extended data
Extended Data Fig. 1 Application of MitoReID in natural compounds to identify epicatechin as a cyclooxygenase-2 inhibitor.
(a) Flowchart illustrating the process for predicting MOAs of natural compounds from Traditional Chinese Medicine (TCM). (b) Predicted results for five natural compounds. The blue bars represent predicted outcomes that have been reported in other studies. Abbreviations: AChR, acetylcholine receptor; ACE, angiotensin converting enzyme; GluR, glucocorticoid receptor; SNRI, serotonin-norepinephrine reuptake inhibitor. (c) Molecular structure of epicatechin. (d) The inhibitory effects of epicatechin on COX-2. (e) A schematic diagram illustrating the binding between epicatechin and COX-2, generated through molecular docking. (f) The result of cellular thermal shift assay (CETSA). (g) The result of surface plasmon resonance (SPR) experiments. KD, dissociation constant; Ka, association rate constant; Kd, dissociation rate constant.
Source data
Supplementary information
Supplementary Information
Supplementary Notes 1–5, Figs. 1–6 and Tables 1–3.
Reporting Summary
Supplementary Data 1
Predicted results of eight novel drugs with known MOA.
Supplementary Data 2
Predicted MOAs of 60 natural compounds.
Supplementary Data 3
Drug annotation list.
Source data
Source Data Fig. 2
Unprocessed images for Fig. 2b,c,e,f, and statistical source data for Fig. 2d–f.
Source Data Fig. 3
Unprocessed images for Fig. 3a,c, and statistical source data for Fig. 3c,e,f,g.
Source Data Fig. 5
Statistical source data for Fig.5a,b.
Source Data Fig. 6
Unprocessed images and statistical Source Data for Fig. 6b.
Source Data Extended Data Fig. 1
Statistical source data for Extended Fig.1b,d,g, and unprocessed gels for Extended Fig. 1f.
Rights and permissions
Springer Nature or its licensor (e.g. a society or other partner) holds exclusive rights to this article under a publishing agreement with the author(s) or other rightsholder(s); author self-archiving of the accepted manuscript version of this article is solely governed by the terms of such publishing agreement and applicable law.
Reprints and permissions
About this article
Cite this article
Yu, M., Li, W., Yu, Y. et al. Deep learning large-scale drug discovery and repurposing.
Nat Comput Sci (2024). https://doi.org/10.1038/s43588-024-00679-4
-
Received: 03 October 2023
-
Accepted: 17 July 2024
-
Published: 21 August 2024
-
DOI: https://doi.org/10.1038/s43588-024-00679-4