Abstract
Climate assessments have largely overlooked the radiative effect of anthropogenic coarse particulate matter (PMcoarse, with an aerodynamic diameter between 2.5 and 10 µm) in China. Despite its similar mass concentration to fine particulate matter (PM2.5), anthropogenic sources of PMcoarse in China have been much less studied and typically underrepresented in models. Here, we present a new model simulation for PMcoarse in China that incorporates various anthropogenic sources. The model successfully captures the magnitude and distribution of observed PMcoarse and recently available aerosol optical depth measurements at near-infrared wavelengths, which are substantially underestimated if anthropogenic PMcoarse is not included. We find that anthropogenic PMcoarse exerts a cooling effect of -0.11 Wm−2 (-0.03 to -0.42 Wm−2) in China by aerosol–radiation interaction, capable of completely offsetting the warming effect from black carbon by 2060 under Dynamic Projection model for Emissions in China (DPEC) 1.1 scenario. We conclude that the radiative effect due to anthropogenic PMcoarse will likely dampen the warming penalty caused by the emission reduction of other aerosols in China and should be incorporated into climate models.
Introduction
Coarse particulate matter (PMcoarse), defined as aerosols with an aerodynamic diameter between 2.5 and 10 µm, encompasses marine sea salt aerosol, volcano ashes, bioaerosol, and fugitive mineral dust originating from both natural and anthropogenic activities1,2. Despite being a severe air pollution issue in China due to its substantial adverse effects on human health3, PMcoarse has received much less attention compared to fine particulate matter (PM2.5) over the past decade. In addition to contributing to the mass of inhalable particle matter (PM10), PMcoarse can influence atmospheric chemistry by providing a reactive surface for heterogeneous reactions and altering cloud water acidity4,5,6. Furthermore, PMcoarse is also a crucial contributor to cloud condensation nuclei and ice nucleating particles, thereby affecting the global climate via modifying the lifetime and properties of clouds1,7. Another significant aspect of its climate impacts is PMcoarse can absorb and scatter radiation, inducing a direct radiative effect (DRE). Unlike PM2.5, which primarily interacts with shortwave radiation, PMcoarse interacts with both shortwave and longwave radiation1,8. This interaction results in a negative DRE in the shortwave and a positive DRE in the longwave at the top of the atmosphere (TOA), potentially leading to atmospheric cooling or warming8,9,10,11,12.
Research on the climate impacts of PMcoarse has predominantly concentrated on marine sea salt aerosol and natural dust, with scant attention given to those from anthropogenic activities13,14. However, a recent study indicates that dust from anthropogenic sources is likely to constitute the majority of PMcoarse mass in China4. While natural dust emissions are primarily caused by wind erosion of soil and are closely related to weather conditions, anthropogenic PMcoarse is directly linked to human activities such as transportation, combustion, and construction sectors15,16. Source appointment analyses of major cities in China suggest that resuspended road dust dominates PMcoarse observations in urban areas17,18,19. A recent study found that PMcoarse dropped abruptly (>50%) in China during the national lockdown period due to COVID-19 restrictions on vehicle traffic, indicating a dominant contribution of road dust to PMcoarse4. Other significant sources include agricultural activities, building construction, and combustions20,21,22. Current national emission inventories typically only consider anthropogenic PMcoarse from combustion and industrial processes, which are minor contributors22,23,24. Emission estimates for other sources are only available for individual cities19,21,25. Models for air quality and climate applications, such as the Coupled Model Intercomparison Project Phase 6 (CIMP6)26, generally do not include these emissions. Consequently, the DRE due to anthropogenic PMcoarse in China is overlooked in current climate assessments.
In this study, we use a three-dimensional chemical transport model (GEOS-Chem), in combination with a newly compiled anthropogenic PMcoarse emission inventory to capture the observed level of PMcoarse in China. The year 2019 was selected for our present-day simulation as it reflects the most recent successful implementation of emission reduction strategies following China’s clean air action, and is not affected by the COVID-19 pandemic that began in 2020. Observations during 2015–2019 show a similar spatial distribution of PMcoarse during the period, with decreasing concentrations reflecting the emission control4. Our study demonstrates that accounting for anthropogenic sources significantly improves the model’s capacity to represent observed level of both PMcoarse mass concentrations and the aerosol optical depth (AOD). The simulated PMcoarse optical properties are subsequently integrated with the rapid radiative transfer model for general circulation models (RRTMG) to investigate its aerosol–radiation effects and radiative forcing.
Results
PMcoarse in China
We have summarized the PMcoarse data, calculated as the difference between PM10 and PM2.5, from the Ministry of Ecology and Environment network for the year 2019. The annual mean concentrations are shown in Fig. 1a. High concentrations of PMcoarse are primarily distributed in northwestern China, where natural desert dust is the dominant source, and over the Beijing–Tianjin–Hebei (BTH) metropolitan region, indicating anthropogenic origins from urban areas. Moderate concentrations (~30 µg m−3) are also found in large areas of South and Northeast China, where natural dust and sea salt aerosol are unlikely to have a significant impact. PMcoarse in eastern China exhibits a weak seasonal variation (Supplementary Fig. 1), not significantly enhanced by natural dust events in spring27, further indicating a dominant anthropogenic source such as from traffic and industrial sectors. We also find a moderate correlation between PMcoarse and CO (correlation coefficient, R = 0.56) in the observation (Supplementary Fig. 2). As CO is primarily emitted by combustion and can be used as a tracer for anthropogenic influence, this correlation provides further evidence that PMcoarse in eastern China is dominated by anthropogenic contributions, consistent with a recent analysis4.
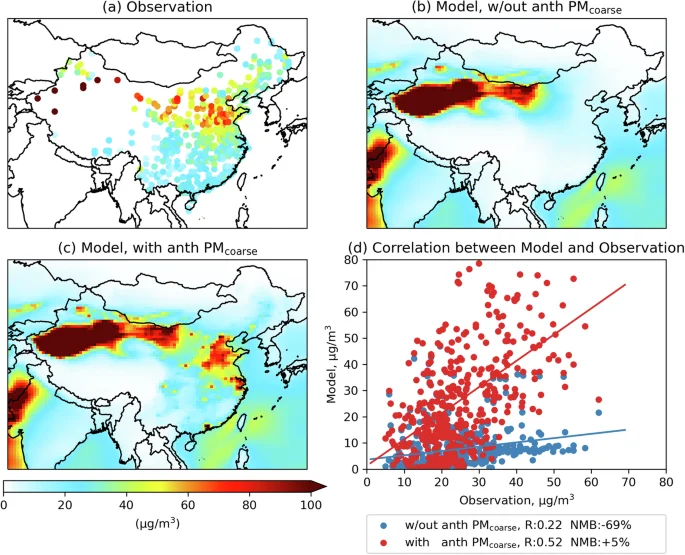
a–c Annual mean concentrations from the Ministry of Ecology and Environment network, standard GEOS-Chem with only natural PMcoarse emissions, and our new GEOS-Chem considering anthropogenic PMcoarse. d Scatterplot of observed and modeled annual mean concentrations. Data during spring (March, April, and May) and over northwestern China (latitude > 35°N and longitude < 105°E) are considered largely affected by natural desert dust and are excluded. Also shown are the correlation coefficient (R), normalized mean bias (NMB) between the model and observations, and the ordinary least squares linear regression lines.
We first simulate PMcoarse using GEOS-Chem version 14.1.1, which only considers the natural source of PMcoarse (see Materials and methods). As shown in Fig. 1b, the simulation captures high PMcoarse levels due to natural dust in northwestern China. However, it yields near-zero values in most areas of eastern China, significantly underestimating the observations. To address this discrepancy, we compile an emission inventory for anthropogenic PMcoarse, incorporating sources from road dust, combustions, industrial processes, and agricultural activities (see Materials and methods). The total anthropogenic emission of PMcoarse for 2019 is estimated to be 24.8 Tg a−1, with road dust being the dominant contributor (90%).
By incorporating anthropogenic sources, we further develop a new PMcoarse simulation for China. As shown in Fig. 1c, this updated simulation successfully represents the observed high PMcoarse concentrations over the BTH metropolitan region and the moderate PMcoarse concentrations over South and Northeast China. Figure 1d presents the correlation between modeled and observed monthly concentrations, in which data from the period (springtime) and region (northwestern China) that are largely affected by natural desert dust have been excluded. The inclusion of anthropogenic sources has significantly improved the model’s performance, elevating the correlation coefficient from 0.22 to 0.52 and reducing the normalized mean bias (NMB) from -69% to 5%. This substantial improvement in the representation of observations demonstrates that our new model can effectively account for the previously overlooked PMcoarse source in current models.
Implications on aerosol optical depth
We further explore the impact of anthropogenic PMcoarse on AOD based on GEOS-Chem aerosol mass and calculated optical properties (see Materials and methods). A frequently used reference wavelength in AOD studies is 550 nm, which aligns closely with the peak solar intensity and is measurable by numerous instruments10,28. However, AOD in the visible spectrum is predominantly influenced by PM2.5 in China, with PMcoarse contributing negligibly29. The influence of PMcoarse becomes more pronounced for AOD at longer wavelengths, such as in the near-infrared range. Supplementary Fig. 3 shows the simulated AOD resulting from anthropogenic PMcoarse. At 550 nm, it contributes a minor fraction (less than 10%) of the total AOD. This contribution escalates to as much as 53% at 1640 nm, and up to 70% at 2313 nm. This result underscores the increasingly significant role of PMcoarse extinction in the near-infrared spectrum.
We compare simulated AOD with the TROPOMI/GRASP product, which provides directly retrieved AOD at both visible and near-infrared wavelengths. This product leverages measurement from the tropospheric monitoring instrument (TROPOMI) on the Sentinel-5 Precursor (S5p) satellite30 and the generalized retrieval of atmosphere and surface properties (GRASP) algorithm31. The model successfully replicates the magnitude and distribution of satellite AOD at 550 nm, which is minimally influenced by anthropogenic PMcoarse (Supplementary Fig. 4). Conversely, the standard model, which only accounts for natural sources of PMcoarse, largely underestimates the satellite AOD at 2313 nm across most regions of China, as shown in Fig. 2. Our updated simulation, which incorporates anthropogenic PMcoarse, exhibits substantial improvement. It successfully reproduces high values (~0.1) in the BTH metropolitan region and moderate values (~0.03) in South China. However, it still underestimates the high values in Northeast China. Figure 2d showcased the frequency distribution of the satellite and modeled AOD at 2313 nm. The inclusion of anthropogenic PMcoarse significantly enhances the simulated frequency towards the satellite distribution but still does not fully replicate the peak, likely due to the underestimate in Northeast China. The bias over Northeast China could be attributed to inaccuracies in the model’s description of emissions, size distribution, PMcoarse shape for this region, or any combination thereof.
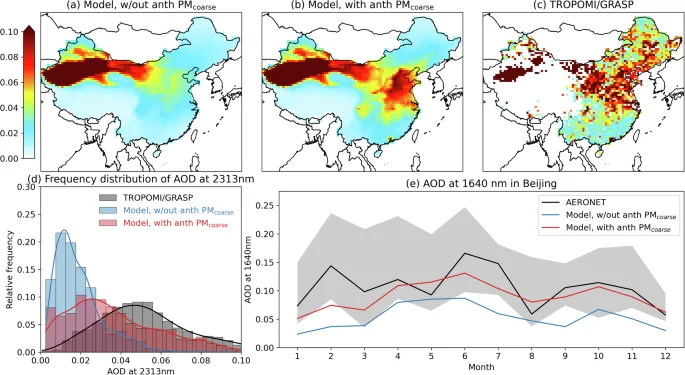
a Modeled AOD at 2313 nm from standard GEOS-Chem without anthropogenic PMcoarse. b Modeled AOD at 2313 nm from our new simulation considering anthropogenic PMcoarse. c Measured AOD at 2313 nm from TROPOMI/GRASP product. d The frequency distribution of measured and simulated AOD at 2313 nm. Data during spring (March, April, and May) and over northwestern China (latitude > 35°N and longitude < 105°E) are excluded in (d) because they are dominated by natural desert dust. All the data shown in a–d are 10-month averaged values covering March to December in 2019, as TROPOMI/GRASP AOD is only available after March 2019. e Monthly median AOD at 1640 nm between model and AERONET measurement at Beijing in 2019. Shadings indicate the range of 25th to 75th percentiles of the measurements.
We also compare the simulated AOD with ground-based sun-photometer measurements from the aerosol robotic network (AERONET) in Beijing, the sole location in continental China where AERONET data was available during 2019. As depicted in Supplementary Fig. 4e, both simulations, with and without anthropogenic PMcoarse, can reproduce the magnitude of AERONET monthly median AOD at 440 nm, primarily contributed by PM2.5. The inclusion of anthropogenic PMcoarse slightly reduces the bias (NMB = −7% compared to −14%). AERNOET version 3 data also provides AOD measurements at 1640 nm, enabling a more thorough examination of PMcoarse’s influence. Unlike at 440 nm, the standard model significantly underestimates AERONET AOD at 1640 nm (NMB = −50%), as shown in Fig. 2e. The inclusion of anthropogenic PMcoarse effectively reduces this bias (NMB = −14%). Note that our model resolution of 0.5° × 0.625° might not capture the local conditions of individual AERONET surface sites, which could contribute to the remaining errors. The above comparisons provide further evidence for the missing PMcoarse in current climate assessments and demonstrate that our model simulation can reproduce the general range and distributions of observed AOD.
Radiative forcing by aerosol–radiation interaction
We assess the impact of anthropogenic PMcoarse on the DRE at TOA by integrating GEOS-Chem output with RRTMG (see Materials and methods). Figure 3 presents the DRE of anthropogenic PMcoarse in the present day (2019), which exerts a net annual average cooling effect of −0.13 Wm−2 (−0.04 to −0.61 Wm−2) under clear-sky conditions and −0.11 Wm−2 (−0.03 to −0.42 Wm−2) under all-sky conditions, over continental China. The uncertainty range is calculated from extreme model sensitivities described in Methods. The annual mean cloud fraction in China for 2019 is 21% in GEOS-Chem, resulting in an all-sky DRE 20% lower than the clear-sky DRE. We also calculate the regional mean for eastern China (longitude > 110°E), where most population and anthropogenic sources are concentrated, to be −0.36 Wm−2 (−0.10 to −1.70 Wm−2) and −0.30 Wm−2 (−0.08 to −1.36 Wm−2) for clear- and all-sky conditions, respectively. The total DRE of anthropogenic PMcoarse is primarily driven by the cooling effect in shortwave wavelengths. The warming effect in the longwave wavelengths is about 10% of the cooling effect.
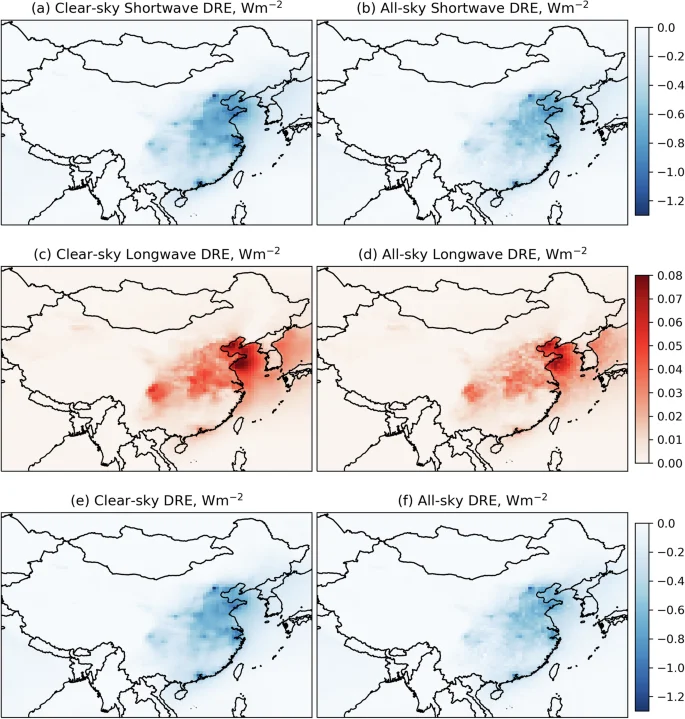
Annual mean values of 2019 simulated by RRTMG are shown for shortwave (a, b), long wave (c, d), and total anthropogenic PMcoarse DRE (e, f) under clear-sky and all-sky conditions.
By conducting an additional simulation for the year 1750, we quantify the radiative forcing by aerosol–radiation interaction (RFari), which signifies a change in DRE from the pre-industrial era to the present day under a fixed climate (see Materials and methods). Figure 4 contrasts the all-sky RFari of anthropogenic PMcoarse with other aerosols. Anthropogenic PMcoarse induces a negative RFari of −0.11 Wm−2 in continental China and −0.30 Wm−2 in eastern China. Although the contribution of anthropogenic PMcoarse to the total RFari is not substantial (~5%), it is noteworthy that over China and eastern China, it could counteract 21% and 36%, respectively, of the warming effect caused by black carbon (BC). BC has been considered a significant warming agent both in China and globally and was the focus of aerosol climate effect studies in the past decade8,32,33.
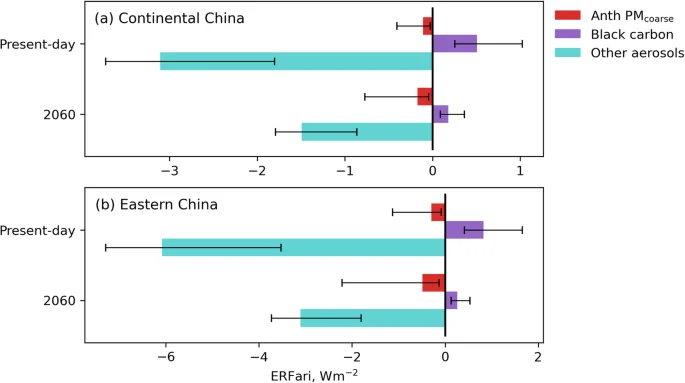
a Mean values of continental China. b Mean values of eastern China. Error bars are calculated from model sensitives as described in Methods. Note the different scales between panels.
Figure 4 also provides an estimate of the all-sky RFari in 2060, derived from a simulation based on the Dynamic Projection model for emissions in China (DPEC) current-goals scenario34,35. This scenario reflects China’s future emissions, taking into account both released and upcoming policies on climate and pollution control, associated with the middle-of-the-road socioeconomic pathway (SSP2) and RCP4.5 scenario36. Aerosol concentrations are projected to decrease significantly due to China’s current clean air action plans and its commitment to nationally determined contribution (NDC) under the Paris Agreement to peak greenhouse gases (GHGs) emissions before 2030. We estimate that the overall all-sky RFari in China will drop from −2.71 Wm−2 in 2019 to −1.27 Wm−2 in 2060. The DPEC scenario does not project fugitive road dust, the major source of anthropogenic PMcoarse34,35. China has established a stringent control policy for fugitive road dust, which resulted in a 1/3 decrease of PMcoarse during 2015–2019 in North China4. In the future scenario, we assume that such a control policy will remain, so the emission factor will not change substantially, which is also not likely to be affected by the NDC-adapted energy transition. Instead, road dust emissions might increase due to the continually growing vehicle population in China. On the other side, present control strategies focusing on combustion and industrial processes could effectively reduce emissions of other aerosols, but not PMcoarse, which are mainly from road dust and agriculture activities. This makes the RFari of PMcoarse increase by 70%, reaching −0.17 Wm−2 in 2060. The increase of road dust and decrease of other aerosols result in anthropogenic PMcoarse contributing to 12% of total RFari in China and completely offsetting the positive forcing from BC. Over eastern China, cooling from anthropogenic PMcoarse is projected to exceed the warming effect of BC by 86% in 2060 (−0.49 Wm−2 compared with +0.26 Wm−2).
Discussion
Our study provides compelling evidence that anthropogenic PMcoarse is an important missing component in previous climate assessments of aerosols in China. Our model, which incorporates anthropogenic PMcoarse sources, substantially improves the model’s ability to simulate both PM mass concentrations and AOD, suggesting that the model can, in general, capture the magnitude of anthropogenic PMcoarse in China and its optical properties. We estimate a mean all-sky TOA RFari of -0.11 Wm−2 in China due to anthropogenic PMcoarse, which is projected to rise to −0.17 Wm−2 in 2060 and could completely offset the warming effect from BC. These results indicate a significant missing source of cooling in current models, which should be considered in the future. Furthermore, while there have been concerns that future aerosol emission reductions might induce additional warming in China, our future scenario analysis suggests that a potential increase in vehicle demand could amplify the cooling effect from PMcoarse, thereby mitigating the warming caused by emission reduction.
Unlike PM2.5 and natural PMcoarse, research on anthropogenic PMcoarse is notably sparse, which poses a challenge in further evaluating the uncertainty of our estimated RFari. This is a major limitation of this study. Model assumptions, including those related to emission factors, refractive indices, particle size distribution, and particle shape distribution, might all involve large uncertainties. Some of these factors are not available from current studies and are instead derived from total PMcoarse measurements in our model simulations. This restricts our efforts to further estimate the uncertainties of individual factors contributing to RFari. Measurement support for the aforementioned model assumptions is crucial to further narrow down potential bias in our estimations. With such information available, future studies may extend the scope of research to a global scale as well as the semi-direct climate effect of anthropogenic PMcoarse.
Methods
Measurement data
Surface PM10 and PM2.5 observations are publicly available from the China Ministry of Ecology and Environment network (https://air.cnemc.cn/). The TROPOMI/GRASP product, which uses data measured from the Tropospheric Monitoring Instrument (TROPOMI) on the Sentinel-5 Precursor (S5p) satellite30 and the Generalized Retrieval of Atmosphere and Surface Properties (GRASP) algorithm31, provides global AOD at a spatial resolution of ~10 km and at 10 wavelengths from 340 to 2313 nm after March 2019. The data is publicly available at https://www.grasp-open.com/products/tropomi-data-release/. We use the monthly data and only include the retrievals with a high-quality assurance index. Aerosol robotic network (AERONET) is a global network of sun-photometer measurements37, which reports AOD at 8 wavelengths from 340 to 1640 nm and is available at https://aeronet.gsfc.nasa.gov/new_web/aerosols.html. We use the daily average data of its version 3 AOD product38 at three sites: Beijing (39.977°N,116.381°E), Beijing-CAMS (39.933°N,116.317°E), and XiangHe (39.754°N,116.962°E). We use the average of data from these sites as they all represent the Beijing area and fall into the same model grid box. When comparing with model results, all the above measurement data are averaged on a 0.5° × 0.625° grid to match the model resolution.
GEOS-Chem simulations
GEOS-Chem model version 14.1.139 is used in this study. Model simulations are conducted at a horizontal resolution of 0.5° × 0.625° and 47 vertical levels from the surface to the mesosphere over East Asia (60°–150°E, 10°S–55°N) and driven by MERRA2 (the Modern-Era Retrospective Analysis for Research and Applications, Version 2) assimilated meteorological fields from the NASA Global Modeling and Assimilation office (GMAO). The Multiresolution Emission Inventory for China (MEIC)24 is used for Chinese anthropogenic emissions other than PMcoarse.
We treat anthropogenic PMcoarse as dust in GEOS-Chem, and is transported in three size bins (1.0–1.8, 1.8–3.0, and 3.0–6.0 µm radius)40,41. Natural dust emissions is based on an offline emission inventory with 0.25° × 0.3125° resolution42, which uses the dust entrainment and deposition (DEAD) scheme43 and a high-resolution source function42,44. Particle size distribution (PSD) of dust follows an expression based on brittle fragmentation theory10:
where V is the normalized volume concentration of dust with geometric diameter D, DM is the soil parameters of median diameter by volume, ({{rm{sigma }}}_{s}) is the geometric stand deviation, and λ is the propagation distance of side branches of cracks created during a fragmentation event. As there is no available information for anthropogenic PMcoarse PSD in China, we assume it follows Eq. (1) with parameters constrained by total dust observations in Asia45.
Anthropogenic PMcoarse emission in China
We compile a national anthropogenic PMcoarse emission inventory for China including sources from road dust, fuel use, industrial process, agricultural tillage, and harvest of dryland farming. Emissions from stationary combustion, industrial processes, and fuel combustion of vehicles are taken from MEIC, which is 2.4 Tg a−1 in 2019. Emissions from agricultural tillage and harvest of dryland farming are calculated based on the measured relationship between PMcoarse emission and planting area21, the latter is available from the data website of National Bureau of Statistics of China (https://data.stats.gov.cn) for each province. The distribution of agriculture areas within each province is determined based on the spatial distribution of ammonia emissions in MEIC. The calculated national PMcoarse emission from dryland farming is 154 Gg a−1 in 2019. Resuspended road dust emissions from different types of vehicles are calculated as:
where ({E}_{i}) is the annual emission from vehicle type i, including 6 types: small passenger vehicles, middle passenger vehicles, large passenger vehicles, light-duty vehicles, middle-duty trucks, and heavy-duty trucks. VPi is the fleet size of vehicle type i, following the recommendations by Ministry of Ecology and Environment of China46. ({{VKT}}_{i}) is the annual average vehicle-kilometers traveled by vehicle type i, available from the data website of the National Bureau of Statistics of China for each province. EF is the emission factor of fugitive road dust, based on the mean of reported values of different road systems in Beijing47, which show similar values as in another study in Xi’an48. Much higher values and lower values estimated through various approaches are also reported previously19,49,50. We scale the EF by a factor of 5 to match the observed PMcoarse mass concentrations. This scaling is reasonable as current uncertainty of road dust EF is as large as a factor of 1016,50, and our purpose is to represent the observed magnitude of PMcoarse and its AOD rather than focusing on an accurate emission estimate. The scaling may also account for other urban PMcoarse sources that are difficult to calculate, such as from construction sites. Provincial data of ({E}_{i}) calculated with Eq. (2) are then distributed into 1 km resolution within each province based on their population density from GPWv451. The national PMcoarse emission from road dust is estimated to be 22.3 Tg a−1 in 2019. Spatial distributions of the total PMcoarse emissions and those from different sources are shown in Supplementary Fig. 5. Road dust dominates PMcoarse emissions in most area of China even if its EF is not scaled. Anthropogenic PMcoarse emissions are added into the three coarse dust bins based on the PSD mentioned above.
Pre-industrial and future scenarios
To characterize the RFari reflecting change relative to pre-industrial times, we perform a pre-industrial simulation identical to our baseline simulation, but with anthropogenic emissions replaced by those from 1750. We use the historical anthropogenic emissions from the Community Emissions Data System (CEDS) inventory52 for the anthropogenic emissions except for anthropogenic PMcoarse, which is assumed to be zero. Similarly, the future scenario is a simulation identical to our baseline simulation, but using anthropogenic emissions projected for 2060. This elucidates China’s future emissions towards released and upcoming policies both on climate and pollution control, following the Current-goals emission inventory in the Dynamic Projection model for Emissions in China (DPEC) version 1.134,35. DPEC does not have estimates of anthropogenic PMcoarse except for those from fuel consumption sectors, which are included as part of their PM10 emission. For PMcoarse emissions from road dust, we assume its future emission is independent of changes in vehicle types (i.e., changes in energy efficiency or transition to electric vehicles) and keep the EF unchanged. We scale the present-day emissions to 2060 based on the increased total vehicle population in DPEC34, reflecting the influence of fleet size. The vehicle population in DPEC is reported to be 2.64 billion in 2050 for the current goal scenario in DPEC. We calculate 2060 values using this 2050 estimate and assuming an increase rate that same as the average increase rate of present-day to 2050. For PMcoarse emissions from agricultural activities, we scale the present-day emissions to 2060 in the two future scenarios following the change of ammonia emissions from agriculture sectors in DPEC. Anthropogenic emissions for other countries in this 2060 simulation use the SSP2-4.5 (shared socioeconomic pathways) scenario as implemented by Murray et al.53 to GEOS-Chem.
Calculations of AOD, DRE, and RFari
We calculate the optical properties of both natural dust and anthropogenic PMcoarse based on the TAMUdust2020 dust scattering database54,55, available at https://zenodo.org/record/4711247. It considers different particle shapes as an ensemble of 20 irregular hexahedral particles and uses a degree of sphericity (Ѱ) to describe particle shape. We assume Ѱ = 0.71 based on the measured value of mineral particles in China56. Refractive indices are from Global Aerosol Data Set (GADS)57. Optical properties calculated by TAMUdust2020 include extinction efficiency (Qext) with regards to the projected area of ellipsoidal particle, single scattering albedo (SSA), and asymmetry factor (ASM). Optical properties for other aerosols are described in our previous works29,33,58. Qext, SSA, ASM, and PSD are then integrated with GEOS-Chem simulated aerosol mass to calculate column-integrated AOD, SSA, and ASM for 34 different wavelengths (16 at longwave and 14 at shortwave that required by RRTMG, plus 440, 550, 1640, and 2313 nm for the analysis in main text) following Huang et al.59. Aerosols are assumed to be externally mixed in our calculation.
Monthly values of AOD, SSA, and ASM are used as inputs for the RRTMG model, which calculates both longwave and shortwave atmospheric fluxes60. The changes in radiative flux due to aerosols are then calculated by RRTMG, along with other information from GEOS-Chem that described in Heald et al.9, and are considered as aerosol DRE. RFari is calculated as the difference in aerosol DRE between the reference year (2019 or 2060) and the pre-industrial simulation of 1750.
Sensitivity analysis to quantify the uncertainty of DRE
Due to the lack of information on anthropogenic PMcoarse, it is challenging to quantify the uncertainty of DRE by accounting for individual model processes. Alternatively, we performed two sensitivity simulations (high and low cases) to explore the uncertainty associated with present-day PMcoarse extinction, the dominant factor controlling its DRE impact. In both simulations, we scaled anthropogenic PMcoarse AOD based on comparisons with TROPOMI/GRASP measurements. In the high case simulation, we increased anthropogenic PMcoarse AOD at 2313 nm in China to align with 1.5 times the TROPOMI/GRASP measurements. AOD at other wavelengths was also adjusted based on the modeled wavelength dependency of anthropogenic PMcoarse extinctions. We imposed a limit on the scale factors to prevent the generation of unrealistic values (>2), and excluded AOD in Northwest China, which is significantly influenced by natural desert dust. In the low-case simulation, we reduced the anthropogenic PMcoarse AOD to match half of the values measured by TROPOMI/GRASP. This scaling generally incorporates biases from emissions, optical properties, and other model processes. Given that satellite retravels of AOD typically have a bias of less than ±50%, these two cases should encompass the uncertainty from observations. The uncertainty range of DRE was calculated as half of the difference between the maximum and minimum from these two simulations. Some other factors, such as uncertainties in other aerosol species, aerosol vertical distribution, and single scatter albedo, were not taken into account in the uncertainty estimation.
For BC and other aerosols, we majorly considered the uncertainty due to aerosol extinction and absorption efficiency. For BC, we assumed ranges for its possible refractive indices, absorption enhancement due to coating, geometric median diameter, and standard deviation of size distribution to be 1.75–0.53i to 1.95–0.79i, 1–2, 20–200 nm, and 1.3–2.2, based on our previous summary of measurements33,61. Other aerosols mainly include sulfate, nitrate, ammonium, and organic aerosols. Uncertainties due to natural aerosols were not calculated as they do not contribute to ERFari. We assumed the uncertainty range of the real part of refractive indices, geometric median diameter, and standard deviation of the size distribution for each aerosol to be ±0.03, ±50%, and ±20% for each aerosol species, reflecting the observed range4,37. We performed 1000 Mie calculations for each aerosol species at 550 nm by randomly choosing the above parameters within their ranges. We obtained the 1-sigma uncertainties (error bars in Fig. 4) from the calculated range of aerosol extinction and absorption efficiency, as well as the resulting range of DRE. We used the same uncertainty estimate for DRE and ERFari, so uncertainties of emissions in different scenarios were not considered.
Data availability
PM measurements at surface sites of China’s Ministry of Ecology and Environment network used for deriving PMcoarse concentrations in the study are available at https://quotsoft.net/air/. TROPOMI/GRASP product and AERONET version 3 data used for comparing with modeled AOD in the study are publicly available at Platform for GRASP open source code (https://www.grasp-open.com/products/tropomi-data-release/) and the AERONET official website (https://aeronet.gsfc.nasa.gov/new_web/webtool_inv_v3.html), respectively.
Code availability
Version 14.1.1 of GEOS-Chem model used in this study is preserved at https://doi.org/10.5281/zenodo.7696651, available via MIT License and developed openly at https://github.com/geoschem/. RRTMG model used for shortwave and longwave radiative transfer calculations is open access at https://github.com/AER-RC/RRTMG_SW and https://github.com/AER-RC/RRTMG_LW, respectively.
References
-
Adebiyi, A. et al. A review of coarse mineral dust in the Earth system. Aeolian Res. https://doi.org/10.1016/j.aeolia.2022.100849 (2023).
-
Zhang, X. Y. et al. Atmospheric aerosol compositions in China: spatial/temporal variability, chemical signature, regional haze distribution and comparisons with global aerosols. Atmos. Chem. Phys. 12, 779–799 (2012).
Google Scholar
-
Chen, R. et al. Associations between coarse particulate matter air pollution and cause-specific mortality: a nationwide analysis in 272 Chinese cities. Environ. Health Perspect. 127, 017008 (2019).
Google Scholar
-
Zhai, S. et al. Coarse particulate matter air quality in East Asia: implications for fine particulate nitrate. Atmos. Chem. Phys. 23, 4271–4281 (2023).
Google Scholar
-
Usher, C. R., Michel, A. E. & Grassian, V. H. Reactions on mineral dust. Chem. Rev. 103, 4883–4940 (2003).
Google Scholar
-
Shah, V., Jacob, D. J., Moch, J. M., Wang, X. & Zhai, S. Global modeling of cloudwater acidity, rainwater acidity, and acid inputs to ecosystems. Atmos. Chem. Phys. Discuss. 2020, 1–42 (2020).
-
Allen, R. J. et al. Observationally constrained aerosol–cloud semi-direct effects. npj Clim. Atmos. Sci. 2, 16 (2019).
Google Scholar
-
Short-lived Climate Forcers. In Climate Change 2021—The Physical Science Basis: Working Group I Contribution to the Sixth Assessment Report of the Intergovernmental Panel on Climate Change, C. Intergovernmental Panel on Climate, ed. (Cambridge University Press, 2023), pp. 817-922.
-
Heald, C. L. et al. Contrasting the direct radiative effect and direct radiative forcing of aerosols. Atmos. Chem. Phys. 14, 5513–5527 (2014).
Google Scholar
-
Kok, J. F. et al. Smaller desert dust cooling effect estimated from analysis of dust size and abundance. Nat. Geosci. 10, 274–278 (2017).
Google Scholar
-
Paulot, F. et al. Revisiting the impact of sea salt on climate sensitivity. Geophys. Res. Lett. 47, e2019GL085601 (2020).
Google Scholar
-
Adebiyi, A. A. & Kok, J. F. Climate models miss most of the coarse dust in the atmosphere. Sci. Adv. 6, eaaz9507 (2020).
Google Scholar
-
Ginoux, P., Prospero, J. M., Gill, T. E., Hsu, N. C. & Zhao, M. Global-scale attribution of anthropogenic and natural dust sources and their emission rates based on MODIS Deep Blue aerosol products. Rev. Geophys. https://doi.org/10.1029/2012RG000388 (2023).
-
Shi, Y. et al. Representing anthropogenic dust in E3SMv1: Implementation, evaluation, and assessment of their radiative forcing. https://doi.org/10.22541/essoar.168988430.03348279/v1 (2023).
-
Xia, W. et al. Double trouble of air pollution by anthropogenic dust. Environ. Sci. Technol. 56, 761–769 (2022).
Google Scholar
-
Kakavas, S. & Pandis, S. N. Effects of urban dust emissions on fine and coarse PM levels and composition. Atmos. Env. https://doi.org/10.1016/j.atmosenv.2020.118006 (2021).
-
Liu, Z. et al. Seasonal and diurnal variation in particulate matter (PM10 and PM2.5) at an urban site of Beijing: analyses from a 9-year study. Environ. Sci. Pollut. Res. 22, 627–642 (2015).
Google Scholar
-
Han, B. et al. Source apportionment of ambient PM10 in urban areas of Wuxi, China. Front. Environ. Sci. Eng. China 5, 552–563 (2011).
Google Scholar
-
Zhang, J. et al. Vehicular non-exhaust particulate emissions in Chinese megacities: source profiles, real-world emission factors, and inventories. Environ. Pollut. 266, 115268 (2020).
Google Scholar
-
Yan, H. et al. Field evaluation of the dust impacts from construction sites on surrounding areas: a city case study in China. Sustainability 11, 1906 (2019).
Google Scholar
-
Chen, W., Tong, D. Q., Zhang, S., Zhang, X. & Zhao, H. Local PM(10) and PM(2.5) emission inventories from agricultural tillage and harvest in northeastern China. J. Environ. Sci. 57, 15–23 (2017).
Google Scholar
-
Lei, Y., Zhang, Q., He, K. B. & Streets, D. G. Primary anthropogenic aerosol emission trends for China, 1990–2005. Atmos. Chem. Phys. 11, 931–954 (2011).
Google Scholar
-
Li, M. et al. Anthropogenic emission inventories in China: a review. Natl Sci. Rev. 4, 834–866 (2017).
Google Scholar
-
Zheng, B. et al. Trends in China’s anthropogenic emissions since 2010 as the consequence of clean air actions. Atmos. Chem. Phys. 18, 14095–14111 (2018).
Google Scholar
-
Wu, X. et al. Real-world emissions and fuel consumption of diesel buses and trucks in Macao: from on-road measurement to policy implications. Atmos. Environ. 120, 393–403 (2015).
Google Scholar
-
Eyring, V. et al. Overview of the Coupled Model Intercomparison Project Phase 6 (CMIP6) experimental design and organization. Geosci. Model Dev. 9, 1937–1958 (2016).
Google Scholar
-
Shao, Y. & Dong, C. H. A review on East Asian dust storm climate, modelling and monitoring. Glob. Planet. Change 52, 1–22 (2006).
Google Scholar
-
Ridley, D. A., Heald, C. L., Kok, J. F. & Zhao, C. An observationally constrained estimate of global dust aerosol optical depth. Atmos. Chem. Phys. 16, 15097–15117 (2016).
Google Scholar
-
Zhai, S. et al. Relating geostationary satellite measurements of aerosol optical depth (AOD) over East Asia to fine particulate matter (PM2.5): insights from the KORUS-AQ aircraft campaign and GEOS-Chem model simulations. Atmos. Chem. Phys. 21, 16775–16791 (2021).
Google Scholar
-
Veefkind, J. P. et al. TROPOMI on the ESA Sentinel-5 Precursor: a GMES mission for global observations of the atmospheric composition for climate, air quality and ozone layer applications. Remote Sens. Environ. 120, 70–83 (2012).
Google Scholar
-
Dubovik, O. et al. A comprehensive description of multi-term LSM for applying multiple a priori constraints in problems of atmospheric remote sensing: GRASP algorithm, concept, and applications. Front. Remote Sens. https://doi.org/10.3389/frsen.2021.706851 (2021).
-
Bond, T. C. & Bergstrom, R. W. Light absorption by carbonaceous particles: an investigative review. Aerosol Sci. Technol. 40, 27–67 (2006).
Google Scholar
-
Wang, X. et al. Exploiting simultaneous observational constraints on mass and absorption to estimate the global direct radiative forcing of black carbon and brown carbon. Atmos. Chem. Phys. 14, 10989–11010 (2014).
Google Scholar
-
Tong, D. et al. Dynamic projection of anthropogenic emissions in China: methodology and 2015–2050 emission pathways under a range of socio-economic, climate policy, and pollution control scenarios. Atmos. Chem. Phys. 20, 5729–5757 (2020).
Google Scholar
-
Cheng, J. et al. Pathways of China’s PM(2.5) air quality 2015-2060 in the context of carbon neutrality. Natl Sci. Rev. 8, nwab078 (2021).
Google Scholar
-
Ipcc. Climate Change 2021: The Physical Science Basis. Contribution of Working Group I to the Sixth Assessment Report of the Intergovernmental Panel on Climate Change (Cambridge University Press, 2021). https://doi.org/10.1017/9781009157896.
-
Dubovik, O. et al. Variability of absorption and optical properties of key aerosol types observed in worldwide locations. J. Atmos. Sci. 59, 590–608 (2002).
Google Scholar
-
Giles, D. M. et al. Advancements in the Aerosol Robotic Network (AERONET) Version 3 database—automated near-real-time quality control algorithm with improved cloud screening for Sun photometer aerosol optical depth (AOD) measurements. Atmos. Meas. Tech. 12, 169–209 (2019).
Google Scholar
-
Community, T. I. G.-C. U. geoschem/GCClassic: GEOS-Chem Classic 14.1.1 (Zenodo) (2023).
-
Duncan Fairlie, T., Jacob, D. J. & Park, R. J. The impact of transpacific transport of mineral dust in the United States. Atmos. Environ. 41, 1251–1266 (2007).
Google Scholar
-
Ridley, D. A., Heald, C. L., Pierce, J. R. & Evans, M. J. Toward resolution-independent dust emissions in global models: Impacts on the seasonal and spatial distribution of dust. Geophys. Res. Lett. 40, 2873–2877 (2013).
Google Scholar
-
Meng, J. et al. Grid-independent high-resolution dust emissions (v1.0) for chemical transport models: application to GEOS-Chem (12.5.0). Geosci. Model Dev. 14, 4249–4260 (2021).
Google Scholar
-
Zender, C. S., Bian, H. & Newman, D. Mineral Dust Entrainment and Deposition (DEAD) model: Description and 1990s dust climatology. J. Geophys. Res. https://doi.org/10.1029/2002JD002775 (2003).
-
Ginoux, P. et al. Sources and distributions of dust aerosols simulated with the GOCART model. J. Geophys. Res. 106, 20255–20273 (2001).
Google Scholar
-
Zhang, L., Kok, J. F., Henze, D. K., Li, Q. & Zhao, C. Improving simulations of fine dust surface concentrations over the western United States by optimizing the particle size distribution. Geophys. Res. Lett. 40, 3270–3275 (2013).
Google Scholar
-
Technical instructions on building on-road vehicle emission inventory. Ministry of Ecology and Environment of China (2021).
-
Wang, H. et al. Temporal-spatial distributions of road silt loadings and fugitive road dust emissions in Beijing from 2019 to 2020. J. Environ. Sci. 132, 56–70 (2023).
Google Scholar
-
Chen, S. et al. Fugitive road dust PM(2.5) emissions and their potential health impacts. Environ. Sci. Technol. 53, 8455–8465 (2019).
Google Scholar
-
Alves, C. A. et al. Chemical profiling of PM10 from urban road dust. Sci. Total Environ. 634, 41–51 (2018).
Google Scholar
-
Casotti Rienda, I. & Alves, C. A. Road dust resuspension: a review. Atmos. Res. 261, 105740 (2021).
Google Scholar
-
Center for International Earth Science Information Network—CIESIN—Columbia University. Gridded Population of the World, Version 4 (GPWv4): Population Density Adjusted to Match 2015 Revision UN WPP Country Totals, Revision 11. NASA Socioeconomic Data and Applications Center (SEDAC) (2018).
-
Hoesly, R. M. et al. Historical (1750–2014) anthropogenic emissions of reactive gases and aerosols from the Community Emissions Data System (CEDS). Geosci. Model Dev. 11, 369–408 (2018).
Google Scholar
-
Murray, L. T., Leibensperger, E. M., Orbe, C., Mickley, L. J. & Sulprizio, M. GCAP 2.0: a global 3-D chemical-transport model framework for past, present, and future climate scenarios. Geosci. Model Dev. 14, 5789–5823 (2021).
Google Scholar
-
Saito, M., Yang, P., Ding, J. & Liu, X. A comprehensive database of the optical properties of irregular aerosol particles for radiative transfer simulations. J. Atmos. Sci. https://doi.org/10.1175/jas-d-20-0338.1 (2021).
-
Saito, M. & Yang, P. Advanced bulk optical models linking the backscattering and microphysical properties of mineral dust aerosol. Geophys. Res. Lett. 48, e2021GL095121 (2021).
Google Scholar
-
Okada, K., Heintzenberg, J., Kai, K. & Qin, Y. Shape of atmospheric mineral particles collected in three Chinese arid-regions. Geophys. Res. Lett. 28, 3123–3126 (2001).
Google Scholar
-
Koepke, P., M. Hess, I. Schult, & E. P. Shettle (1997). Global Aerosol Data Set, Report No. 243. Max-Planck-Institut für Meteorologie.
-
Wang, X. et al. Exploring the observational constraints on the simulation of brown carbon. Atmos. Chem. Phys. 18, 635–653 (2018).
Google Scholar
-
Huang, Y., Kok, J. F., Saito, M. & Muñoz, O. Single-scattering properties of ellipsoidal dust aerosols constrained by measured dust shape distributions. Atmos. Chem. Phys. 23, 2557–2577 (2023).
Google Scholar
-
Lacis, A. A. & Oinas, V. A description of the correlated k distribution method for modeling nongray gaseous absorption, thermal emission, and multiple scattering in vertically inhomogeneous atmospheres. J. Geophys. Res. 96, 9027–9063 (1991).
Google Scholar
-
Wang, X. et al. Deriving brown carbon from multiwavelength absorption measurements: method and application to AERONET and Aethalometer observations. Atmos. Chem. Phys. 16, 12733–12752 (2016).
Google Scholar
Acknowledgements
This work was supported by the City University of Hong Kong’s New Research Initiatives and Strategic Research Grant (grant Nos. 9610470 and 7005922). LS was supported by the National Natural Science Foundation of China (4227050413). The authors wish to acknowledge GRASP and CloudFlight teams for GRASP/S5p datasets obtained in the framework of ESA S5p + Innovation AOD/BRDF project (https://www.grasp-sas.com/projects/aod-brdf_sentinel-5p-innovation/). The authors would like to acknowledge the efforts of the AERONET site managers and investigators in making the data available for analysis. We thank the helpful discussions with Yuzhong Zhang at Westlake University and Zhen Leng at the Hong Kong Polytechnic University.
Author information
Authors and Affiliations
Contributions
X.W. devised the research, developed, and performed the model simulations. S.Z. contributed to data analysis of surface observations. L.S. contributed to data processing and analysis of future emission scenarios. X.W. wrote the paper with contributions from all co-authors.
Corresponding author
Ethics declarations
Competing interests
The authors declare no competing interests.
Additional information
Publisher’s note Springer Nature remains neutral with regard to jurisdictional claims in published maps and institutional affiliations.
Supplementary information
Supplementary Information
Rights and permissions
Open Access This article is licensed under a Creative Commons Attribution-NonCommercial-NoDerivatives 4.0 International License, which permits any non-commercial use, sharing, distribution and reproduction in any medium or format, as long as you give appropriate credit to the original author(s) and the source, provide a link to the Creative Commons licence, and indicate if you modified the licensed material. You do not have permission under this licence to share adapted material derived from this article or parts of it. The images or other third party material in this article are included in the article’s Creative Commons licence, unless indicated otherwise in a credit line to the material. If material is not included in the article’s Creative Commons licence and your intended use is not permitted by statutory regulation or exceeds the permitted use, you will need to obtain permission directly from the copyright holder. To view a copy of this licence, visit http://creativecommons.org/licenses/by-nc-nd/4.0/.
Reprints and permissions
About this article
Cite this article
Wang, X., Zhai, S. & Shen, L. Cooling from aerosol–radiation interaction of anthropogenic coarse particles in China.
npj Clim Atmos Sci 7, 220 (2024). https://doi.org/10.1038/s41612-024-00773-4
-
Received: 03 June 2024
-
Accepted: 12 September 2024
-
Published: 20 September 2024
-
DOI: https://doi.org/10.1038/s41612-024-00773-4