Abstract
The complex relationships between continuously monitored health signals and therapeutic regimens can be modelled via machine learning. However, the clinical implementation of the models will require changes to clinical workflows. Here we outline ClinAIOps (‘clinical artificial-intelligence operations’), a framework that integrates continuous therapeutic monitoring and the development of artificial intelligence (AI) for clinical care. ClinAIOps leverages three feedback loops to enable the patient to make treatment adjustments using AI outputs, the clinician to oversee patient progress with AI assistance, and the AI developer to receive continuous feedback from both the patient and the clinician. We lay out the central challenges and opportunities in the deployment of ClinAIOps by means of examples of its application in the management of blood pressure, diabetes and Parkinson’s disease. By enabling more frequent and accurate measurements of a patient’s health and more timely adjustments to their treatment, ClinAIOps may substantially improve patient outcomes.
This is a preview of subscription content, access via your institution
Access options
style{display:none!important}.LiveAreaSection-193358632 *{align-content:stretch;align-items:stretch;align-self:auto;animation-delay:0s;animation-direction:normal;animation-duration:0s;animation-fill-mode:none;animation-iteration-count:1;animation-name:none;animation-play-state:running;animation-timing-function:ease;azimuth:center;backface-visibility:visible;background-attachment:scroll;background-blend-mode:normal;background-clip:borderBox;background-color:transparent;background-image:none;background-origin:paddingBox;background-position:0 0;background-repeat:repeat;background-size:auto auto;block-size:auto;border-block-end-color:currentcolor;border-block-end-style:none;border-block-end-width:medium;border-block-start-color:currentcolor;border-block-start-style:none;border-block-start-width:medium;border-bottom-color:currentcolor;border-bottom-left-radius:0;border-bottom-right-radius:0;border-bottom-style:none;border-bottom-width:medium;border-collapse:separate;border-image-outset:0s;border-image-repeat:stretch;border-image-slice:100%;border-image-source:none;border-image-width:1;border-inline-end-color:currentcolor;border-inline-end-style:none;border-inline-end-width:medium;border-inline-start-color:currentcolor;border-inline-start-style:none;border-inline-start-width:medium;border-left-color:currentcolor;border-left-style:none;border-left-width:medium;border-right-color:currentcolor;border-right-style:none;border-right-width:medium;border-spacing:0;border-top-color:currentcolor;border-top-left-radius:0;border-top-right-radius:0;border-top-style:none;border-top-width:medium;bottom:auto;box-decoration-break:slice;box-shadow:none;box-sizing:border-box;break-after:auto;break-before:auto;break-inside:auto;caption-side:top;caret-color:auto;clear:none;clip:auto;clip-path:none;color:initial;column-count:auto;column-fill:balance;column-gap:normal;column-rule-color:currentcolor;column-rule-style:none;column-rule-width:medium;column-span:none;column-width:auto;content:normal;counter-increment:none;counter-reset:none;cursor:auto;display:inline;empty-cells:show;filter:none;flex-basis:auto;flex-direction:row;flex-grow:0;flex-shrink:1;flex-wrap:nowrap;float:none;font-family:initial;font-feature-settings:normal;font-kerning:auto;font-language-override:normal;font-size:medium;font-size-adjust:none;font-stretch:normal;font-style:normal;font-synthesis:weight style;font-variant:normal;font-variant-alternates:normal;font-variant-caps:normal;font-variant-east-asian:normal;font-variant-ligatures:normal;font-variant-numeric:normal;font-variant-position:normal;font-weight:400;grid-auto-columns:auto;grid-auto-flow:row;grid-auto-rows:auto;grid-column-end:auto;grid-column-gap:0;grid-column-start:auto;grid-row-end:auto;grid-row-gap:0;grid-row-start:auto;grid-template-areas:none;grid-template-columns:none;grid-template-rows:none;height:auto;hyphens:manual;image-orientation:0deg;image-rendering:auto;image-resolution:1dppx;ime-mode:auto;inline-size:auto;isolation:auto;justify-content:flexStart;left:auto;letter-spacing:normal;line-break:auto;line-height:normal;list-style-image:none;list-style-position:outside;list-style-type:disc;margin-block-end:0;margin-block-start:0;margin-bottom:0;margin-inline-end:0;margin-inline-start:0;margin-left:0;margin-right:0;margin-top:0;mask-clip:borderBox;mask-composite:add;mask-image:none;mask-mode:matchSource;mask-origin:borderBox;mask-position:0 0;mask-repeat:repeat;mask-size:auto;mask-type:luminance;max-height:none;max-width:none;min-block-size:0;min-height:0;min-inline-size:0;min-width:0;mix-blend-mode:normal;object-fit:fill;object-position:50% 50%;offset-block-end:auto;offset-block-start:auto;offset-inline-end:auto;offset-inline-start:auto;opacity:1;order:0;orphans:2;outline-color:initial;outline-offset:0;outline-style:none;outline-width:medium;overflow:visible;overflow-wrap:normal;overflow-x:visible;overflow-y:visible;padding-block-end:0;padding-block-start:0;padding-bottom:0;padding-inline-end:0;padding-inline-start:0;padding-left:0;padding-right:0;padding-top:0;page-break-after:auto;page-break-before:auto;page-break-inside:auto;perspective:none;perspective-origin:50% 50%;pointer-events:auto;position:static;quotes:initial;resize:none;right:auto;ruby-align:spaceAround;ruby-merge:separate;ruby-position:over;scroll-behavior:auto;scroll-snap-coordinate:none;scroll-snap-destination:0 0;scroll-snap-points-x:none;scroll-snap-points-y:none;scroll-snap-type:none;shape-image-threshold:0;shape-margin:0;shape-outside:none;tab-size:8;table-layout:auto;text-align:initial;text-align-last:auto;text-combine-upright:none;text-decoration-color:currentcolor;text-decoration-line:none;text-decoration-style:solid;text-emphasis-color:currentcolor;text-emphasis-position:over right;text-emphasis-style:none;text-indent:0;text-justify:auto;text-orientation:mixed;text-overflow:clip;text-rendering:auto;text-shadow:none;text-transform:none;text-underline-position:auto;top:auto;touch-action:auto;transform:none;transform-box:borderBox;transform-origin:50% 50%0;transform-style:flat;transition-delay:0s;transition-duration:0s;transition-property:all;transition-timing-function:ease;vertical-align:baseline;visibility:visible;white-space:normal;widows:2;width:auto;will-change:auto;word-break:normal;word-spacing:normal;word-wrap:normal;writing-mode:horizontalTb;z-index:auto;-webkit-appearance:none;-moz-appearance:none;-ms-appearance:none;appearance:none;margin:0}.LiveAreaSection-193358632{width:100%}.LiveAreaSection-193358632 .login-option-buybox{display:block;width:100%;font-size:17px;line-height:30px;color:#222;padding-top:30px;font-family:Harding,Palatino,serif}.LiveAreaSection-193358632 .additional-access-options{display:block;font-weight:700;font-size:17px;line-height:30px;color:#222;font-family:Harding,Palatino,serif}.LiveAreaSection-193358632 .additional-login>li:not(:first-child)::before{transform:translateY(-50%);content:””;height:1rem;position:absolute;top:50%;left:0;border-left:2px solid #999}.LiveAreaSection-193358632 .additional-login>li:not(:first-child){padding-left:10px}.LiveAreaSection-193358632 .additional-login>li{display:inline-block;position:relative;vertical-align:middle;padding-right:10px}.BuyBoxSection-683559780{display:flex;flex-wrap:wrap;flex:1;flex-direction:row-reverse;margin:-30px -15px 0}.BuyBoxSection-683559780 .box-inner{width:100%;height:100%}.BuyBoxSection-683559780 .readcube-buybox{background-color:#f3f3f3;flex-shrink:1;flex-grow:1;flex-basis:255px;background-clip:content-box;padding:0 15px;margin-top:30px}.BuyBoxSection-683559780 .subscribe-buybox{background-color:#f3f3f3;flex-shrink:1;flex-grow:4;flex-basis:300px;background-clip:content-box;padding:0 15px;margin-top:30px}.BuyBoxSection-683559780 .subscribe-buybox-nature-plus{background-color:#f3f3f3;flex-shrink:1;flex-grow:4;flex-basis:100%;background-clip:content-box;padding:0 15px;margin-top:30px}.BuyBoxSection-683559780 .title-readcube,.BuyBoxSection-683559780 .title-buybox{display:block;margin:0;margin-right:10%;margin-left:10%;font-size:24px;line-height:32px;color:#222;padding-top:30px;text-align:center;font-family:Harding,Palatino,serif}.BuyBoxSection-683559780 .title-asia-buybox{display:block;margin:0;margin-right:5%;margin-left:5%;font-size:24px;line-height:32px;color:#222;padding-top:30px;text-align:center;font-family:Harding,Palatino,serif}.BuyBoxSection-683559780 .asia-link{color:#069;cursor:pointer;text-decoration:none;font-size:1.05em;font-family:-apple-system,BlinkMacSystemFont,”Segoe UI”,Roboto,Oxygen-Sans,Ubuntu,Cantarell,”Helvetica Neue”,sans-serif;line-height:1.05em6}.BuyBoxSection-683559780 .access-readcube{display:block;margin:0;margin-right:10%;margin-left:10%;font-size:14px;color:#222;padding-top:10px;text-align:center;font-family:-apple-system,BlinkMacSystemFont,”Segoe UI”,Roboto,Oxygen-Sans,Ubuntu,Cantarell,”Helvetica Neue”,sans-serif;line-height:20px}.BuyBoxSection-683559780 .access-asia-buybox{display:block;margin:0;margin-right:5%;margin-left:5%;font-size:14px;color:#222;padding-top:10px;text-align:center;font-family:-apple-system,BlinkMacSystemFont,”Segoe UI”,Roboto,Oxygen-Sans,Ubuntu,Cantarell,”Helvetica Neue”,sans-serif;line-height:20px}.BuyBoxSection-683559780 .access-buybox{display:block;margin:0;margin-right:10%;margin-left:10%;font-size:14px;color:#222;opacity:.8px;padding-top:10px;text-align:center;font-family:-apple-system,BlinkMacSystemFont,”Segoe UI”,Roboto,Oxygen-Sans,Ubuntu,Cantarell,”Helvetica Neue”,sans-serif;line-height:20px}.BuyBoxSection-683559780 .price-buybox{display:block;font-size:30px;color:#222;font-family:-apple-system,BlinkMacSystemFont,”Segoe UI”,Roboto,Oxygen-Sans,Ubuntu,Cantarell,”Helvetica Neue”,sans-serif;padding-top:30px;text-align:center}.BuyBoxSection-683559780 .price-buybox-to{display:block;font-size:30px;color:#222;font-family:-apple-system,BlinkMacSystemFont,”Segoe UI”,Roboto,Oxygen-Sans,Ubuntu,Cantarell,”Helvetica Neue”,sans-serif;text-align:center}.BuyBoxSection-683559780 .price-info-text{font-size:16px;padding-right:10px;color:#222;font-family:-apple-system,BlinkMacSystemFont,”Segoe UI”,Roboto,Oxygen-Sans,Ubuntu,Cantarell,”Helvetica Neue”,sans-serif}.BuyBoxSection-683559780 .price-value{font-size:30px;font-family:-apple-system,BlinkMacSystemFont,”Segoe UI”,Roboto,Oxygen-Sans,Ubuntu,Cantarell,”Helvetica Neue”,sans-serif}.BuyBoxSection-683559780 .price-per-period{font-family:-apple-system,BlinkMacSystemFont,”Segoe UI”,Roboto,Oxygen-Sans,Ubuntu,Cantarell,”Helvetica Neue”,sans-serif}.BuyBoxSection-683559780 .price-from{font-size:14px;padding-right:10px;color:#222;font-family:-apple-system,BlinkMacSystemFont,”Segoe UI”,Roboto,Oxygen-Sans,Ubuntu,Cantarell,”Helvetica Neue”,sans-serif;line-height:20px}.BuyBoxSection-683559780 .issue-buybox{display:block;font-size:13px;text-align:center;color:#222;font-family:-apple-system,BlinkMacSystemFont,”Segoe UI”,Roboto,Oxygen-Sans,Ubuntu,Cantarell,”Helvetica Neue”,sans-serif;line-height:19px}.BuyBoxSection-683559780 .no-price-buybox{display:block;font-size:13px;line-height:18px;text-align:center;padding-right:10%;padding-left:10%;padding-bottom:20px;padding-top:30px;color:#222;font-family:-apple-system,BlinkMacSystemFont,”Segoe UI”,Roboto,Oxygen-Sans,Ubuntu,Cantarell,”Helvetica Neue”,sans-serif}.BuyBoxSection-683559780 .vat-buybox{display:block;margin-top:5px;margin-right:20%;margin-left:20%;font-size:11px;color:#222;padding-top:10px;padding-bottom:15px;text-align:center;font-family:-apple-system,BlinkMacSystemFont,”Segoe UI”,Roboto,Oxygen-Sans,Ubuntu,Cantarell,”Helvetica Neue”,sans-serif;line-height:17px}.BuyBoxSection-683559780 .tax-buybox{display:block;width:100%;color:#222;padding:20px 16px;text-align:center;font-family:-apple-system,BlinkMacSystemFont,”Segoe UI”,Roboto,Oxygen-Sans,Ubuntu,Cantarell,”Helvetica Neue”,sans-serif;line-height:NaNpx}.BuyBoxSection-683559780 .button-container{display:flex;padding-right:20px;padding-left:20px;justify-content:center}.BuyBoxSection-683559780 .button-container>*{flex:1px}.BuyBoxSection-683559780 .button-container>a:hover,.Button-505204839:hover,.Button-1078489254:hover,.Button-2496381730:hover{text-decoration:none}.BuyBoxSection-683559780 .readcube-button{background:#fff;margin-top:30px}.BuyBoxSection-683559780 .button-asia{background:#069;border:1px solid #069;border-radius:0;cursor:pointer;display:block;padding:9px;outline:0;text-align:center;text-decoration:none;min-width:80px;margin-top:75px}.BuyBoxSection-683559780 .button-label-asia,.ButtonLabel-3869432492,.ButtonLabel-3296148077,.ButtonLabel-1651148777{display:block;color:#fff;font-size:17px;line-height:20px;font-family:-apple-system,BlinkMacSystemFont,”Segoe UI”,Roboto,Oxygen-Sans,Ubuntu,Cantarell,”Helvetica Neue”,sans-serif;text-align:center;text-decoration:none;cursor:pointer}.Button-505204839,.Button-1078489254,.Button-2496381730{background:#069;border:1px solid #069;border-radius:0;cursor:pointer;display:block;padding:9px;outline:0;text-align:center;text-decoration:none;min-width:80px;max-width:320px;margin-top:10px}.Button-505204839 .readcube-label,.Button-1078489254 .readcube-label,.Button-2496381730 .readcube-label{color:#069}
/* style specs end */
Access Nature and 54 other Nature Portfolio journals
Get Nature+, our best-value online-access subscription
$29.99 / 30 days
cancel any time
Subscribe to this journal
Receive 12 digital issues and online access to articles
$99.00 per year
only $8.25 per issue
Rent or buy this article
Prices vary by article type
from$1.95
to$39.95
Prices may be subject to local taxes which are calculated during checkout



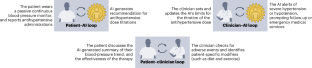
References
-
Tison, G. H. et al. Passive detection of atrial fibrillation using a commercially available smartwatch. JAMA Cardiol. 3, 409–416 (2018).
Google Scholar
-
Dörr, M. et al. The WATCH AF Trial: SmartWATCHes for detection of atrial fibrillation. JACC Clin. Electrophysiol. 5, 199–208 (2019).
Google Scholar
-
Galindo, R. J. et al. Continuous glucose monitors and automated insulin dosing systems in the hospital consensus guideline. J. Diabetes Sci. Technol. 14, 1035–1064 (2020).
Google Scholar
-
Johnston, L., Wang, G., Hu, K., Qian, C. & Liu, G. Advances in biosensors for continuous glucose monitoring towards wearables. Front. Bioeng. Biotechnol. 9, 733810 (2021).
Google Scholar
-
Martens, T. et al. Effect of continuous glucose monitoring on glycemic control in patients with Type 2 diabetes treated with basal insulin: a randomized clinical trial. JAMA 325, 2262–2272 (2021).
Google Scholar
-
Tschider, C. A. Medical device artificial intelligence: the new tort frontier. BYU Law Rev. 46, 1551 (2020).
-
Simon, D. A., Shachar, C. & Glenn Cohen, I. Unsettled liability issues for ‘prediagnostic’ wearables and health-related products. JAMA 328, 1391–1392 (2022).
Google Scholar
-
Benroubi, M. Fear, guilt feelings and misconceptions: barriers to effective insulin treatment in type 2 diabetes. Diabetes Res. Clin. Pract. 93, S97–S99 (2011).
Google Scholar
-
Moor, M. et al. Foundation models for generalist medical artificial intelligence. Nature 616, 259–265 (2023).
Google Scholar
-
Berwick, D. M., Nolan, T. W. & Whittington, J. The triple aim: care, health and cost. Health Aff. 27, 759–769 (2008).
Google Scholar
-
Assadi, A. et al. An integration engineering framework for machine learning in healthcare. Front. Digit. Health 4, 932411 (2022).
Google Scholar
-
Breaux-Shropshire, T. L., Judd, E., Vucovich, L. A., Shropshire, T. S. & Singh, S. Does home blood pressure monitoring improve patient outcomes? A systematic review comparing home and ambulatory blood pressure monitoring on blood pressure control and patient outcomes. Integr. Blood Press. Control 8, 43–49 (2015).
Google Scholar
-
Siontis, K. C., Noseworthy, P. A., Attia, Z. I. & Friedman, P. A. Artificial intelligence-enhanced electrocardiography in cardiovascular disease management. Nat. Rev. Cardiol. 18, 465–478 (2021).
Google Scholar
-
Attia, Z. I. et al. Novel bloodless potassium determination using a signal‐processed single‐lead ECG. J. Am. Heart Assoc. 5, e002746 (2016).
Google Scholar
-
Goud, K. Y. et al. Wearable electrochemical microneedle sensor for continuous monitoring of levodopa: toward Parkinson management. ACS Sens. 4, 2196–2204 (2019).
Google Scholar
-
Powers, R. et al. Smartwatch inertial sensors continuously monitor real-world motor fluctuations in Parkinson’s disease. Sci. Transl. Med. 13, eabd7865 (2021).
Google Scholar
-
Fletcher, R. R., Tam, S., Omojola, O., Redemske, R. & Kwan, J. Wearable sensor platform and mobile application for use in cognitive behavioral therapy for drug addiction and PTSD. Conf. Proc. IEEE Eng. Med. Biol. Soc. 2011, 1802–1805 (2011).
-
Russell-Jones, D., Pouwer, F. & Khunti, K. Identification of barriers to insulin therapy and approaches to overcoming them. Diabetes Obes. Metab. 20, 488–496 (2018).
Google Scholar
-
Przezak, A., Bielka, W. & Molęda, P. Fear of hypoglycemia—an underestimated problem. Brain Behav. 12, e2633 (2022).
Google Scholar
-
Yeh, T., Yeung, M. & Mendelsohn Curanaj, F. A. Managing patients with insulin pumps and continuous glucose monitors in the hospital: to wear or not to wear. Curr. Diab. Rep. 21, 7 (2021).
Google Scholar
-
Waks, J. W. et al. Intermittent anticoagulation guided by continuous atrial fibrillation burden monitoring using dual-chamber pacemakers and implantable cardioverter-defibrillators: results from the Tailored Anticoagulation for Non-Continuous Atrial Fibrillation (TACTIC-AF) pilot study. Heart Rhythm 15, 1601–1607 (2018).
Google Scholar
-
Passman, R. et al. Targeted anticoagulation for atrial fibrillation guided by continuous rhythm assessment with an insertable cardiac monitor: the rhythm evaluation for anticoagulation with continuous monitoring (React.com) pilot study. J. Cardiovasc. Electrophysiol 27, 264–270 (2016).
Google Scholar
-
Wasserlauf, J. et al. Smartwatch performance for the detection and quantification of atrial fibrillation. Circ. Arrhythm. Electrophysiol. 12, e006834 (2019).
Google Scholar
-
Leading science, research and technology leaders join forces to accelerate REACT-AF trial. American Heart Association (29 August 2022).
-
Bienefeld, N. et al. Solving the explainable AI conundrum by bridging clinicians’ needs and developers’ goals. NPJ Digit. Med. 6, 94 (2023).
Google Scholar
-
Attig, C. & Franke, T. Abandonment of personal quantification: a review and empirical study investigating reasons for wearable activity tracking attrition. Comput. Hum. Behav. 102, 223–237 (2020).
Google Scholar
-
Wang, T. et al. Identifying major impact factors affecting the continuance intention of mHealth: a systematic review and multi-subgroup meta-analysis. NPJ Digit. Med. 5, 145 (2022).
Google Scholar
-
Meyerowitz-Katz, G. et al. Rates of attrition and dropout in app-based interventions for chronic disease: systematic review and meta-analysis. J. Med. Internet Res. 22, e20283 (2020).
Google Scholar
-
Helander, E., Kaipainen, K., Korhonen, I. & Wansink, B. Factors related to sustained use of a free mobile app for dietary self-monitoring with photography and peer feedback: retrospective cohort study. J. Med. Internet Res. 16, e109 (2014).
Google Scholar
-
Xu, S., Kim, J., Walter, J. R., Ghaffari, R. & Rogers, J. A. Translational gaps and opportunities for medical wearables in digital health. Sci. Transl. Med. 14, eabn6036 (2022).
Google Scholar
-
Zhang, Y., Suda, N., Lai, L. & Chandra, V. Hello Edge: keyword spotting on microcontrollers. Preprint at https://arxiv.org/abs/1711.07128 (2017).
-
Basaklar, T., Tuncel, Y., An, S. & Ogras, U. Wearable devices and low-power design for smart health applications: challenges and opportunities. In Proc. 2021 IEEE/ACM International Symposium on Low Power Electronics and Design (ISLPED) (eds Li, H. & Augustine, C.) 1 (IEEE, 2021).
-
Sundrani, S. et al. Predicting patient decompensation from continuous physiologic monitoring in the emergency department. NPJ Digit. Med. 6, 60 (2023).
Google Scholar
-
Jackson, C., Shahsahebi, M., Wedlake, T. & DuBard, C. A. Timeliness of outpatient follow-up: an evidence-based approach for planning after hospital discharge. Ann. Fam. Med. 13, 115–122 (2015).
Google Scholar
-
Erb, M. K. et al. mHealth and wearable technology should replace motor diaries to track motor fluctuations in Parkinsonas disease.npj Digit. Med. 3, 6 (2020).
Google Scholar
-
Steinkirchner, A. B. et al. Self-report of chronic diseases in old-aged individuals: extent of agreement with general practitioner medical records in the German AugUR study. J. Epidemiol. Community Health 76, 931–938 (2022).
Google Scholar
-
Pirtošek, Z. et al. Update on the management of Parkinson’s disease for general neurologists. Parkinsonas Dis 2020, 9131474 (2020).
-
Shalash, A., Spindler, M. & Cubo, E. Global perspective on telemedicine for Parkinson’s disease. J. Parkinsons Dis. 11, S11–S18 (2021).
Google Scholar
-
Greenland, J. C., Williams-Gray, C. H. & Barker, R. A. The clinical heterogeneity of Parkinson’s disease and its therapeutic implications. Eur. J. Neurosci. 49, 328–338 (2019).
Google Scholar
-
Drew, B. J. et al. Insights into the problem of alarm fatigue with physiologic monitor devices: a comprehensive observational study of consecutive intensive care unit patients. PLoS ONE 9, e110274 (2014).
Google Scholar
-
Chromik, J. et al. Computational approaches to alleviate alarm fatigue in intensive care medicine: a systematic literature review. Front. Digit. Health 4, 843747 (2022).
Google Scholar
-
Nimri, R. et al. Insulin dose optimization using an automated artificial intelligence-based decision support system in youths with type 1 diabetes. Nat. Med. 26, 1380–1384 (2020).
Google Scholar
-
Zarrinpar, A. et al. Individualizing liver transplant immunosuppression using a phenotypic personalized medicine platform. Sci. Transl. Med. 8, 333ra49 (2016).
Google Scholar
-
Pantuck, A. J. et al. Modulating BET bromodomain inhibitor ZEN-3694 and enzalutamide combination dosing in a metastatic prostate cancer patient using CURATE.AI, an artificial intelligence platform. Adv. Ther. 1, 1800104 (2018).
Google Scholar
-
Kee, T. et al. Harnessing CURATE.AI as a digital therapeutics platform by identifying N-of-1 learning trajectory profiles. Adv. Ther. 2, 1900023 (2019).
Google Scholar
-
Liang, W. et al. Advances, challenges and opportunities in creating data for trustworthy AI. Nat. Mach. Intell. 4, 669–677 (2022).
Google Scholar
-
Zhang, A., Xing, L., Zou, J. & Wu, J. C. Shifting machine learning for healthcare from development to deployment and from models to data. Nat. Biomed. Eng. 6, 1330–1345 (2022).
Google Scholar
-
Feng, J. et al. Clinical artificial intelligence quality improvement: towards continual monitoring and updating of AI algorithms in healthcare. NPJ Digit. Med. 5, 66 (2022).
Google Scholar
-
Tang, J. et al. Corrigendum: application of machine-learning models to predict tacrolimus stable dose in renal transplant recipients. Sci. Rep. 8, 46936 (2018).
Google Scholar
-
Liu, R., Li, X., Zhang, W. & Zhou, H.-H. Comparison of nine statistical model based warfarin pharmacogenetic dosing algorithms using the racially diverse International Warfarin Pharmacogenetic Consortium cohort database. PLoS ONE 10, e0135784 (2015).
Google Scholar
-
Zhu, X. et al. A machine learning approach to personalized dose adjustment of lamotrigine using noninvasive clinical parameters. Sci. Rep. 11, 5568 (2021).
Google Scholar
-
Jovanović, M. et al. Application of counter-propagation artificial neural networks in prediction of topiramate concentration in patients with epilepsy. J. Pharm. Pharm. Sci. 18, 856–862 (2015).
Google Scholar
-
Tsichlaki, S., Koumakis, L. & Tsiknakis, M. Type 1 diabetes hypoglycemia prediction algorithms: systematic review. JMIR Diabetes 7, e34699 (2022).
Google Scholar
-
US Food and Drug Administration et al. Proposed Regulatory Framework for Modifications to Artificial Intelligence/Machine Learning (AI/ML)-based Software as a Medical Device (SaMD) (US FDA, 019).
-
Salama, K., Kazmierczak, J. & Schut, D. Practitioners Guide to MLOps: A Framework for Continuous Delivery and Automation of Machine Learning (Google, 2021).
-
Utsumil, Y., Rudovicl, O. O., Petersonl, K., Guerrero, R. & Picardl, R. W. Personalized Gaussian processes for forecasting of Alzheimer’s Disease Assessment Scale-Cognition sub-scale (ADAS-Cog13). Conf. Proc. IEEE Eng. Med. Biol. Soc. 2018, 4007–4011 (2018).
-
Liu, K. et al. Development and validation of a personalized model with transfer learning for acute kidney injury risk estimation using electronic health records. JAMA Netw. Open 5, e2219776 (2022).
Google Scholar
-
Hard, A. et al. Federated learning for mobile keyboard prediction. Preprint at https://arxiv.org/abs/1811.03604 (2018).
-
Agarwal, R. Rehospitalization rates in hypertensive emergency. Hypertension 73, 49–51 (2019).
Google Scholar
-
Miller, J., McNaughton, C., Joyce, K., Binz, S. & Levy, P. Hypertension management in emergency departments. Am. J. Hypertens. 33, 927–934 (2020).
Google Scholar
-
Block, R. C. et al. Conventional pulse transit times as markers of blood pressure changes in humans. Sci. Rep. 10, 16373 (2020).
Google Scholar
-
Nabeel, P. M., Jayaraj, J. & Mohanasankar, S. Single-source PPG-based local pulse wave velocity measurement: a potential cuffless blood pressure estimation technique. Physiol. Meas. 38, 2122–2140 (2017).
Google Scholar
-
Islam, S. M. S. et al. Wearable cuffless blood pressure monitoring devices: a systematic review and meta-analysis. Eur. Heart J. Digit. Health 3, 323–337 (2022).
Google Scholar
-
Zheng, Y.-L., Yan, B. P., Zhang, Y.-T. & Poon, C. C. Y. An armband wearable device for overnight and cuff-less blood pressure measurement. IEEE Trans. Biomed. Eng. 61, 2179–2186 (2014).
Google Scholar
-
AI for Anti-Hypertensive Medication Titration (NIH NCBI, accessed 1 October 2023); https://clinicaltrials.gov/ct2/show/NCT05376683
-
Morawski, K. et al. Association of a Smartphone application with medication adherence and blood pressure control: the MedISAFE-BP randomized clinical trial. JAMA Intern. Med. 178, 802–809 (2018).
Google Scholar
-
Baumann, B. M. et al. Provider self-report and practice: reassessment and referral of emergency department patients with elevated blood pressure. Am. J. Hypertens. 22, 604–610 (2009).
Google Scholar
-
National Academies of Sciences, Engineering, and Medicine, Policy and Global Affairs, Committee on Women in Science, Engineering, and Medicine & Committee on Improving the Representation of Women and Underrepresented Minorities in Clinical Trials and Research. Improving Representation in Clinical Trials and Research: Building Research Equity for Women and Underrepresented Groups (National Academies Press, 2022).
-
Hoel, A. W. et al. Under-representation of women and ethnic minorities in vascular surgery randomized controlled trials. J. Vasc. Surg. 50, 349–354 (2009).
Google Scholar
-
Adam, G. et al. Machine learning approaches to drug response prediction: challenges and recent progress. NPJ Precis. Oncol. 4, 19 (2020).
Google Scholar
-
Goyal, M., Ospel, J. M., Kappelhof, M. & Ganesh, A. Challenges of outcome prediction for acute stroke treatment decisions. Stroke 52, 1921–1928 (2021).
Google Scholar
-
Okumura, K. et al. Comparing patient and physician risk tolerance for bleeding events associated with anticoagulants in atrial fibrillation-evidence from the United States and Japan. Value in Health Regional Issues 6, 65–72 (2015).
Google Scholar
-
Wiktorski, T. Challenges in causal inference from personal monitoring devices. In Proc. Federated Conference on Computer Science and Information Systems (ed. Jassem, K.) 99–102 (PTI, 2018).
-
Causality in digital medicine. Nat. Commun. 12, 5471 (2021).
-
Nogueira, A. R., Gama, J. & Ferreira, C. A. Improving prediction with causal probabilistic variables. In Proc. Advances in Intelligent Data Analysis XVIII (eds Berthold, M., Feelders, A. & Krempl, G.) 379–390 (Springer, 2020).
-
Deng, C., Ji, X., Rainey, C., Zhang, J. & Lu, W. Integrating machine learning with human knowledge. iScience 23, 101656 (2020).
Google Scholar
-
Taylor, L. et al. Using virtual representations in mHealth application interventions for health-related behaviour change: a systematic review. Cogent Psychol. 9, 2069906 (2022).
Google Scholar
-
El-Gayar, O., Ofori, M. & Nawar, N. On the efficacy of behavior change techniques in mHealth for self-management of diabetes: a meta-analysis. J. Biomed. Inform. 119, 103839 (2021).
Google Scholar
-
Jakob, R. et al. Factors influencing adherence to mHealth apps for prevention or management of noncommunicable diseases: systematic review. J. Med. Internet Res. 24, e35371 (2022).
Google Scholar
-
Everett, E. M. et al. A longitudinal view of disparities in insulin pump use among youth with type 1 diabetes: the SEARCH for Diabetes in Youth Study. Diabetes Technol. Ther. 25, 131–139 (2023).
Google Scholar
-
Davis, K. & Guterman, S. Rewarding excellence and efficiency in Medicare payments. Milbank Q. 85, 449–468 (2007).
Google Scholar
-
Crowson, M. G. & Chan, T. C. Y. Machine learning as a catalyst for value-based health care. J. Med. Syst. 44, 139 (2020).
Google Scholar
Acknowledgements
The authors thank A. Karargyris for helpful feedback.
Author information
Authors and Affiliations
Contributions
All authors discussed the content, reviewed and edited the manuscript, and agreed to the final version of the manuscript.
Corresponding authors
Ethics declarations
Competing interests
The authors declare no competing interests.
Peer review
Peer review information
Nature Biomedical Engineering thanks David Bates, Randall Moorman and Xiangrong Liu for their contributions to the peer review of this work.
Additional information
Publisher’s note Springer Nature remains neutral with regard to jurisdictional claims in published maps and institutional affiliations.
Rights and permissions
Springer Nature or its licensor (e.g. a society or other partner) holds exclusive rights to this article under a publishing agreement with the author(s) or other rightsholder(s); author self-archiving of the accepted manuscript version of this article is solely governed by the terms of such publishing agreement and applicable law.
Reprints and Permissions
About this article
Cite this article
Chen, E., Prakash, S., Janapa Reddi, V. et al. A framework for integrating artificial intelligence for clinical care with continuous therapeutic monitoring.
Nat. Biomed. Eng (2023). https://doi.org/10.1038/s41551-023-01115-0
-
Received: 15 March 2023
-
Accepted: 26 September 2023
-
Published: 06 November 2023
-
DOI: https://doi.org/10.1038/s41551-023-01115-0