Abstract
Salmonella enterica serovar Gallinarum (S. Gallinarum) is an avian-specific pathogen responsible for fowl typhoid, a severe systemic disease with high mortality in chickens. This disease poses a substantial burden to the poultry industry, particularly in developing countries like China. However, comprehensive genome datasets on S. Gallinarum are lacking. Here, we present the most extensive S. Gallinarum genome dataset, comprising 574 well-collated samples. This dataset consists of 366 genomes sequenced in our laboratory and 208 publicly available genomes, collected from various continents over the past century. Using in silico prediction, we categorized S. Gallinarum into three distinct biovars. Regarding antimicrobial resistance, 238 strains (41.5%) carried antimicrobial resistance genes (ARGs) with a total of 635 records, while 232 strains (40.4%) exhibited multi-drug resistance. Mobile genomic elements (MGEs) serve as critical drivers for ARGs. Our dataset includes 5,636 MGEs records, with most MGEs belonging to prophages and plasmids. This dataset expands our understanding of the genomic characteristics of S. Gallinarum, providing valuable resources for future genomic studies to improve disease management.
Similar content being viewed by others
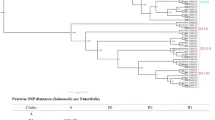
Whole genome sequencing and protein structure analyses of target genes for the detection of Salmonella
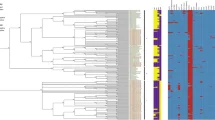
Whole genome sequencing analyses revealed that Salmonella enterica serovar Dublin strains from Brazil belonged to two predominant clades
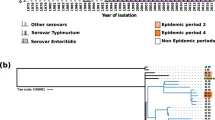
Comparative genomics of Salmonella enterica serovar Enteritidis ST-11 isolated in Uruguay reveals lineages associated with particular epidemiological traits
Background & Summary
Salmonella is a significant pathogen threatening the global public health1,2,3. Many Salmonella serovars can infect various hosts. Salmonella enterica serovar Gallinarum (S. Gallinarum), an avian-restricted pathogen, causes pullorum disease (PD) and fowl typhoid (FT), resulting in high mortality rates and disproportionately affecting the poultry industry in low- and middle-income countries4. S. Gallinarum comprises three common biovars: S. Gallinarum biovar Gallinarum (bvSG), S. Gallinarum biovar Pullorum (bvSP), and S. Gallinarum biovar Duisburg (bvSD)5. Specifically, bvSP causes PD through both horizontal and vertical transmission and is prevalent in many countriess affecting chickens and turkeys6,7. Persistent bvSP infection in young birds within spleen macrophages complicates decontamination efforts. In contrast, bvSG can infect birds of any age, leading to fatal FT disease8. While S. Gallinarum was globally prevalent in the 20th century, eradication programs in most developed countries have significantly reduced its presence9. However, S. Gallinarum remains prevalent in developing countries like China and Brazil due to the diverse poultry industry and breeding patterns, severely constraining the healthy development of the breeding chicken industry10.
Antimicrobial therapy remains the primary treatment for S. Gallinarum infections11. However, the misuse or overuse of antimicrobials has led to the increasingly frequent issue of antibiotic resistance (AMR)12,13,14. Previous studies have shown that S. Gallinarum has developed significant resistance to fluoroquinolones, a class of first-line drugs used for treatment, such as enrofloxacin and ofloxacin15,16. Increasing strains exhibit multi-drug resistance (MDR), which jeopardizes the efficacy of single antibiotic treatments17,18. Mobile genomic elements (MGEs) are key drivers in the acquisition of antimicrobial resistance genes (ARGs), which contribute to antimicrobial resistance in S. Gallinaru19. For example, class 1 integrons have been demonstrated to facilitate AMR acquisition in S. Gallinarum in both in vivo and in vitro experiments20. Plasmids such as IncN, IncX1, and IncQ1, have also been observed among antimicrobial-resistant S. Gallinarum21. However, the role of other MGEs, such as prophages and transposons, in acquiring resistance remains uncertain. Additionally, it is unclear whether other unproven genes contribute to the elevated levels of resistance observed.
With the advancement of whole-genome sequencing (WGS) technology, researchers can now monitor and trace phylogenetic relationships among S. Gallinarum populations at the single-base level, further elucidating the genetic mechanisms underlying epidemiologically important phenotypes like antimicrobial resistance22,23,24. In this study, we collected the most comprehensive and well-annotated genomic dataset of 574 S. Gallinarum isolates, covering the period from 1920 to 2023. We found that S. Gallinarum, with distinct properties, exhibits variations in the types of ARGs, MGEs, and potential functional genes it possesses. We believe the comprehensive S. Gallinarum genome resources provided in this study will significantly aid in the surveillance and prevention of pullorum disease.
Methods
Ethical considerations
The protocols used in this study were approved by the Committee of the Laboratory Animal Center of Zhejiang University (ZJU20190094; ZJU20220295).
Sample and metadata collection
The collection of S. Gallinarum genomes in this study was obtained from publicly accessible datasets and laboratory sources. Firstly, a PubMed (“https://pubmed.ncbi.nlm.nih.gov”) search using the terms “Salmonella Gallinarum” and “Genome” yielded 42 publications on the epidemiology of the S. Gallinarum genome over the past decade. After identifying and excluding one duplicate report, we included 41 unique studies in our analysis. Of these, 22 contained publicly available S. Gallinarum genomic data (Fig. 1a). Next, we used the Enterobase25 (“https://enterobase.warwick.ac.uk”), a comprehensive online resource for bacterial genomics, to search for genome data of all Salmonella serovars labelled as “Gallinarum”. Finally, we combined and streamlined the genome data obtained from both sources, resulting in a dataset comprising 532 S. Gallinarum strains. Notably, 325 of these genomes were sourced from public datasets and had been previously studied in our laboratory. The metadata also includes the “Isolate continent”, “Isolate country”, “Isolate province or state”, and “Isolate year” for each strain collected. Additionally, 42 recently isolated S. Gallinarum strains from deceased chicken embryos in Zhejiang Province, China, were added to the preliminary dataset, bringing the total number of S. Gallinarum genomes to 574.
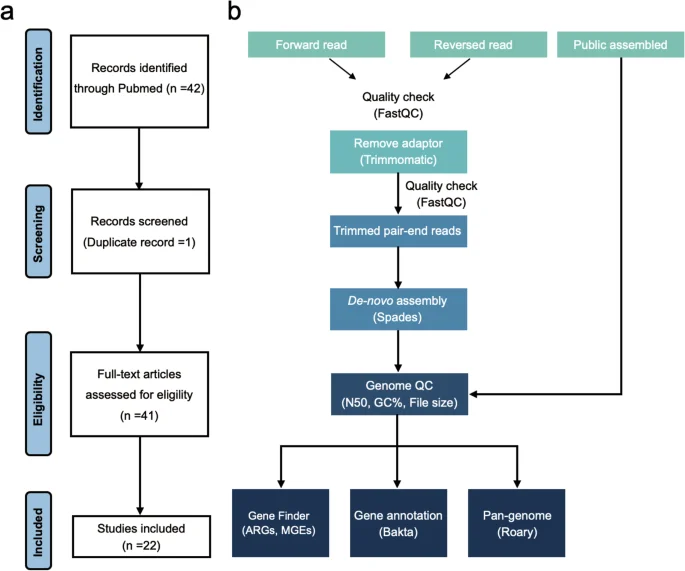
Overall study workflow. (a) Analysis process for the systematic literature review. (b) Processing pipeline for genomic data.
S. Gallinarum isolation and DNA extraction
A total of 734 dead chicken embryo samples were collected from Taishun and Yueqing in Zhejiang Province, China. After thorough autopsies, the liver, intestines, and spleen were extracted and placed individually into 2 mL centrifuge tubes, which contained 1 mL PBS. The organs were then homogenized by grinding. During the initial enrichment phase, we used Buffered Peptone Water (BPW, Haibo Biotechnology Co, Qingdao, China) at a 1:9 dilution ratio (sample in PBS: BPW) and incubated the mixture at 37 °C for 16–18 hours in a rotary incubator set to 180 rpm. For selective enrichment, Tetrathionate Broth Base (TTB, Land Bridge Biotechnology Co, Beijing, China), enhanced with iodine solution and brilliant green solution (both from Land Bridge Biotechnology Co, Beijing, China), was used at a 1:10 ratio (sample in BPW: TTB). The mixture was then incubated at 42 °C for 22–26 hours in a rotary incubator set at 180 rpm. Isolated Salmonella colonies from positive samples were obtained by sub-culturing the selectively enriched samples on Xylose Lysine Deoxycholate (XLD, Land Bridge Technology Co, Beijing, China) agar, followed by an 18–22-hour incubation at 37 °C. Typical and pure colonies were selected after sub-culturing on XLD agar and then transferred into Luria-Bertani (LB) broth. Finally, the transferred bacterial culture was further incubated at 37 °C for 18–22 hours in a rotary incubator set to 180 rpm.
DNA extraction and genomic assembly
The DNA was extracted using the Vazyme Fastpure® Bacteria DNA Isolation Mini Kit (Vazyme Biotech Co., Ltd.) and quantified using the NanoDrop1000 system (Thermo Fisher Scientific, USA). DNA libraries were subsequently constructed and sequenced on the Illumina Novaseq. 6000 platform (Novogene, Beijing, China). The quality of the sequencing reads was assessed using FastQC v0.74, and any joint or low-quality reads were removed with Trimmomatic26 v0.39. Genome sequences were assembled by SPAdes27 v3.12.0 with default parameters. We obtained 45 S. Gallinarum genomic sequences from 734 dead chicken embryo samples (isolation rate = 6.1%), but three were duplicates. After removing duplicates, we finally retained 42 unique S. Gallinarum genome sequences.
Genome quality control
All WGS data passed strict quality control according to criteria set by the European Reference Laboratory28. Python3 script was used to assess genome quality with genomics exceeding 500 contigs, and an N50 of less than 30,000 was excluded. Moreover, we considered GC% within the range of 51% to 53% and a file genome length between 4.2 and 5.5 MB. Busco29 v5.5.0 was used to assess genomic completeness with default parameters. Lastly, the bacterial species and S. Gallinarum biovar type were confirmed by KmerFinder v3.2 and SISTR30 v1.1.1. Finally, the assembled dataset includes a total of 574 high-quality sequences.
Genome annotation and Pan-genome analysis
The assembled genomes were annotated using the Bakta31 v1.9.2 with the latest “Full” dataset. Further, the minimum contig size (–min-contig-length) was set to 200, and “–keep-contig-headers” was set to “Ture”. The output of Bakta was then used to calculate the Pan-genome matrix using Roary32 v3.13.0. The results were visualized using Python3 scripts provided by Roary with default parameters. The 7-gene legacy Multilocus Sequence Typing (MLST) was conducted using the MLST software with the “senterica_achtman_2” scheme.
MGE and ARG detecting
We identified four types of MGEs: plasmids, transposons, integrons, and prophages. MOB-suite33 v3.0.3 was used to reconstruct and classify Plasmids. Specifically, we extracted plasmid sequences from assembled fasta files by MOB-Recon, and the plasmid replicon type and mobility were predicted by MOB-Typer. Integrons and transposons in the genomes were detected with BacAnt34 v3.4.0, only focusing on those with a similarity of more than 60% and a coverage greater than 60%. The prophages were identified through the Phaster pipeline35. The genomic data were split into two temporary datasets based on contigs number: one dataset for single contig files and the other for multiple contig files imported into the Phaster pipeline separately with default parameters. The ARGs were detected by ResFinder, with minimal identity and coverage thresholds set to 90%.
Figure 1b illustrates the overall genomic analysis pipeline of this study.
Data Records
-
Publication information: The list of authors, titles, journals, and Digital Object Identifiers (DOIs) for each paper allows authors or subsequent dataset users to track and validate data sources (Supplementary Table 1). Literature serial numbers have no practical significance and are only used for arbitrary sorting and counting functions.
-
Genome meta information: The names, quality information, biovar type, isolation source, isolation time, and sequence type (ST) of all genomes in this dataset are provided in Supplementary Tables 2, 3. Each row represents an individual record, while each column corresponds to a variable, as detailed below:
-
1)
GC%: The percentage of nucleobases in a DNA molecule that are either guanine (G) or cytosine (C).
-
2)
N50: The length of the shortest contig for which contigs of equal or longer length cover at least 50% of the assembled genome data.
-
3)
Total length(bp): The number of nucleobases in a DNA molecule.
-
4)
BUSCO: These results correspond to the genomic completeness assessment, which consists of four components: Complete (C) and single-copy (S), Complete and duplicated (D), Fragmented (F), and Missing (M).
-
5)
Biovar: Biovar of S. Gallinarum.
-
6)
Continent: The sampling site information at the continent level.
-
7)
Country: The sampling site information at the country level.
-
8)
Province or State: The sampling site information at the province or state level.
-
9)
Year: Sampling year.
-
10)
ST: The sequence type of S. Gallinarum.
-
11)
Data source: This indicates whether the strain originated from our laboratory.
-
The ARGs records are provided in Supplementary Table 4, while the MGEs records are included in Supplementary Tables 5, 6. The annotated files produced by “Bakta” and the pan-genome results have been uploaded to Figshare36 at: https://doi.org/10.6084/m9.figshare.26054251.v1.
-
Genome dataset: The dataset for the newly isolated 42 strains of Salmonella Gallinarum is available in the NCBI Sequence Read Archive (SRA) under BioProject PRJNA114371337,38. The “BioSample ID” and “Accession number” for each strain are listed in Supplementary Table 7. The dataset for the 532 Salmonella Gallinarum genomes, including publicly available data from our lab and other sources, is available on Figshare36 at: https://doi.org/10.6084/m9.figshare.26054251.v1.
-
The Sequence files for the 1,733 plasmids from 574 S. Gallinarum are available on Figshare36 at: https://doi.org/10.6084/m9.figshare.26054251.v1.
Technical Validation
Genome quality
The genomic data of the 574 S. Gallinarum in the dataset underwent rigorous quality control. All genomic data were predicted by KmerFinder and SISTR, confirming S. Gallinarum identity, with N50 values exceeding 30,000 (average = 529,910). The genome lengths of the 574 strains ranged from 4,511,649 bp to 5,411,605 bp (average = 4,800,117 bp), and the GC content ranged from 51.25% to 53.15% (average = 52.12%). The anticipated genome size ensured minimal genomic contamination (Fig. 2a–c). Additionally, Busco results indicated that the genomic completeness of the dataset exceeded 98.5%, ensuring the reliability of the data for future analyses (Fig. 2d).
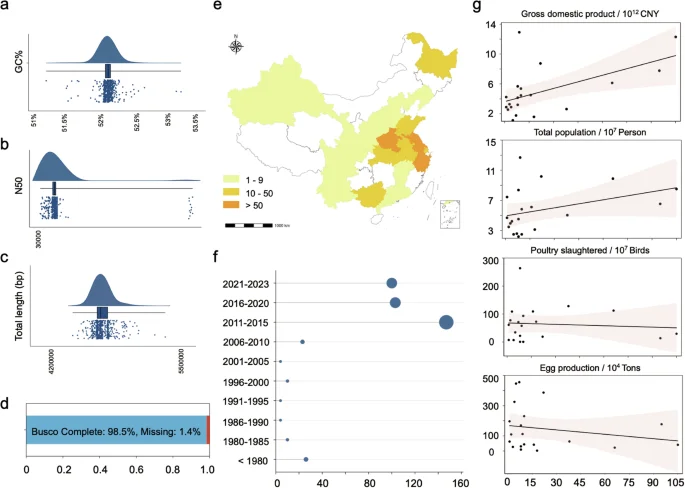
Genomic quality control and geo-temporal distribution of Chinese source Salmonella enterica serovar Gallinarum (S. Gallinarum). (a–c) Quality control of the assembled genome. Where a-c represents GC%, N50, and genome length, respectively. (d) BUSCO analysis showed 98.5% gene completeness in genomes of all three strains, with only 1.4% missing gene orthologs. (e) Geographical distribution of S. Gallinarum in China. The colour indicates the number of isolated bacterial within the province. (f) Temporal distribution of S. Gallinarum in China. Larger circles represent more S. Gallinarum. (g) Correlation analysis was conducted between the number of S. Gallinarum isolates collected from different provinces in China and each province’s total GDP, population size, number of poultry slaughtered, and egg production. The figure depicts points representing individual provinces. The x-axis indicates the number of S. Gallinarum isolates included in the dataset, while the y-axis displays the values for each province’s total GDP, population size, number of poultry slaughtered, and egg production, respectively.
Geographic distribution
In our dataset, most S. Gallinarum isolates were from Asia (435/574), with Europe (61/574), South America (30/574), North America (14/574), and Africa (7/574). For Asia, China is the primary source of S. Gallinarum, with most cases concentrated in the eastern region (Fig. 2e). Regarding the duration of isolation, our dataset indicates a notable prevalence of S. Gallinarum in China after 2010 (Fig. 2f). To determine whether the number of isolates in China is biased, we conducted a correlation analysis between the number of S. Gallinarum isolates from different provinces and the provinces’ latest GDP and total population, respectively. The results indicate that most points fall within the 95% confidence interval of the regression line. Although some points exhibit a bias in the number of S. Gallinarum strains, most of these points have a low sample size (n < 15) (Fig. 2g). Consequently, we hypothesize that the geographic distribution of S. Gallinarum in different provinces of China mirrors the overall national trend. To further eliminate potential bias, conducting larger sampling and increasing the number of available genomes is important.
Abundance of ARGs and MGEs
The dataset includes 635 records on ARGs and 5,706 records on MGEs. For ARGs, 617 records from Asia, 12 from the Americas, and 6 from Europe were identified. Interestingly, we observed a significant increase in the proportion of recently isolated S. Gallinarum (after 2005) carrying beta-lactam and sulfonamide ARGs, while the corresponding proportion of aminoglycoside and trimethoprim ARGs decreased. As the region with the highest number of ARG records, our dataset revealed that beta-lactam and sulfonamide ARGs were prevalent in most provinces of China. In contrast, trimethoprim ARGs were scarce (Fig. 3a).
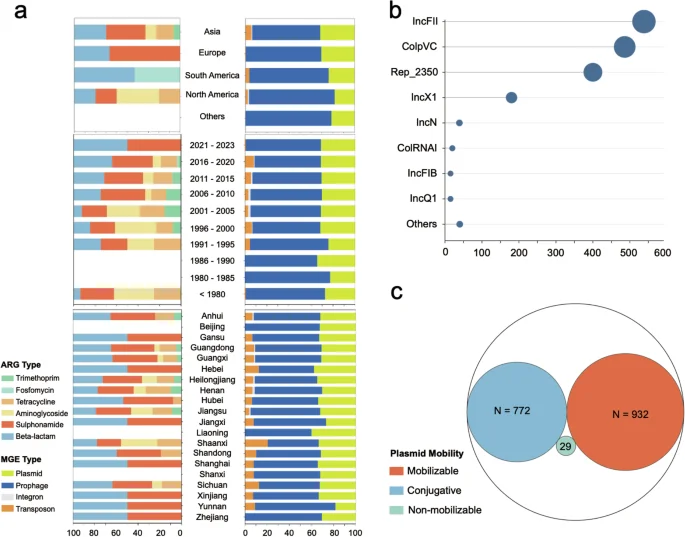
The abundance records of ARGs and MGEs in the dataset. (a) The proportion of abundance records for ARG and MGE in each continent, each year, and each province of China. (b) Predominant replicon types of 1733 plasmid carried by Salmonella enterica serovar Gallinarum. (c) Prediction of mobility of 1733 plasmids using MOB-Typer (Conjugative, Mobilizable, Non-mobilizable).
For MGEs, the records of prophage and plasmid were the highest. Specifically, there were 3,667 records for prophages and 1,733 records for plasmids. Prophages were carried by almost all S. Gallinarum, and plasmid carriage was also high at 97% (557/574). Typing of plasmids further confirmed that IncFII and ColpVC were the most common plasmid types in S. Gallinarum (Fig. 3b). Notably, 53.8% (932/1,733) of the plasmids were predicted to be mobilizable (Fig. 3c).
Pan-genome analysis
Using the combination of Bakta and Roary as a workflow to analyze the pan-genome, we identified the core, soft-core, shell, and cloud genes carried by S. Gallinarum. From a biological significance perspective, the core and soft-core genes in most S. Gallinarum strains encode proteins essential for fundamental biological processes. They could be used in outbreak detection purpose and genomic surveillance39. Non-core genes, such as shell and cloud genes, comprise MGEs and reflect horizontal gene transfer (HGT) between S. Gallinarum strains40. These genes also endow S. Gallinarum with specialized functions, enabling them to thrive in unique environments. We observed a total of 12,461 genes in 574 S. Gallinarum strains. These include core genes (n = 3,604), soft-core genes (n = 467), shell genes (n = 893), and cloud genes (n = 7,497) (Fig. 4a), which might indicate a high frequency of HGT among S. Gallinarum. Furthermore, we compared the unique genes in a single S. Gallinarum biovar type. We found that bvSP has the highest number of unique genes, with 5,941, followed by bvSG (n = 768) and bvSD (n = 120) (Supplementary Table 8).
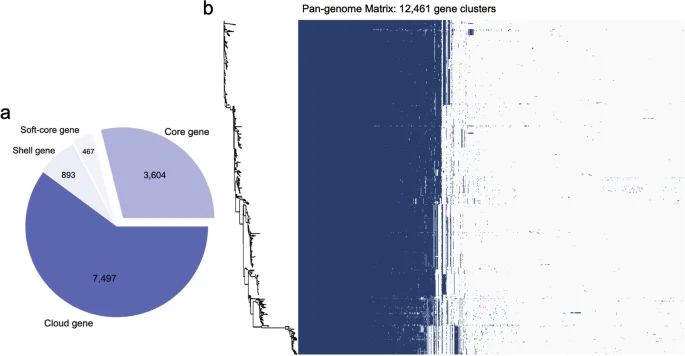
Results of pan-genomic analysis. (a) Presence of different genes in the pan-genome analysis. The total number of genes is 12,461, which contains the Core gene (n = 3,604), Soft-core gene (n = 467), Shell gene (n = 893), and Cloud gene (n = 7,497). (b) The Salmonella enterica serovar Gallinarum phylogenetic tree constructed based on the Pan-genome is shown on the left. The colours in the heat map on the right indicate the presence or absence of genes, with dark blue meaning gene present and blank meaning absent.
Usage Notes
The data are shared under Creative Commons Attribution 4.0 International (CC BY 4.0). The full text of the license is available at: https://creativecommons.org/licenses/by/4.0/.
Code availability
The open-source software used in this study includes:
BacAnt (https://github.com/xthua/bacant),
Abricate (https://github.com/tseemann/abricate),
SISTR (https://github.com/phac-nml/sistr_cmd),
FastQC (https://github.com/s-andrews/FastQC),
Trimmomatic (https://github.com/timflutre/trimmomatic),
MLST (https://cge.food.dtu.dk/services/MLST/),
R (https://www.r-project.org),
Phaster (http://phaster.ca),
KmerFinder (https://bitbucket.org/genomicepidemiology/kmerfinder),
MOB-suite (https://github.com/phac-nml/mob-suite),
Spades (https://github.com/ablab/spades),
Roary (https://sanger-pathogens.github.io/Roary),
Busco (https://busco.ezlab.org),
Bakta (https://github.com/oschwengers/bakta),
Python3 script for genomic quality control was uploaded at: https://github.com/tjiaa/Genome_QC.
References
-
Ke, Y. et al. Genomic investigation and nationwide tracking of pediatric invasive nontyphoidal Salmonella in China. mLife 3, 156–160 (2024).
Google Scholar
-
Wang, Z. et al. Salmonellosis outbreak archive in China: data collection and assembly. Sci Data 11, 244 (2024).
Google Scholar
-
Wang, Z. et al. Nationwide trends and features of human salmonellosis outbreaks in China. Emerging Microbes & Infections 13, 2372364 (2024).
Google Scholar
-
Shivaprasad, H. L. Fowl typhoid and pullorum disease: -EN–FR–ES-. Rev. Sci. Tech. OIE 19, 405–424 (2000).
Google Scholar
-
Batista, D. F. A. et al. Identification and characterization of regions of difference between the Salmonella Gallinarum biovar Gallinarum and the Salmonella Gallinarum biovar Pullorum genomes. Infection, Genetics and Evolution 30, 74–81 (2015).
Google Scholar
-
Kang, X. et al. Integrated OMICs approach reveals energy metabolism pathway is vital for Salmonella Pullorum survival within the egg white. mSphere e00362–24, https://doi.org/10.1128/msphere.00362-24 (2024).
-
El-Saadony, M. T. et al. The control of poultry salmonellosis using organic agents: an updated overview. Poultry Science 101, 101716 (2022).
Google Scholar
-
Kabir, S. M. L. Avian Colibacillosis and Salmonellosis: A Closer Look at Epidemiology, Pathogenesis, Diagnosis, Control and Public Health Concerns. IJERPH 7, 89–114 (2010).
Google Scholar
-
De Carli, S. et al. Molecular and phylogenetic analyses of Salmonella Gallinarum trace the origin and diversification of recent outbreaks of fowl typhoid in poultry farms. Veterinary Microbiology 212, 80–86 (2017).
Google Scholar
-
Zhou, X., Kang, X., Zhou, K. & Yue, M. A global dataset for prevalence of Salmonella Gallinarum between 1945 and 2021. Sci Data 9, 495 (2022).
Google Scholar
-
Zhou, X. et al. Genome degradation promotes Salmonella pathoadaptation by remodeling fimbriae-mediated proinflammatory response. National Science Review 10, nwad228 (2023).
Google Scholar
-
Huang, L. et al. Impact of COVID-19-related nonpharmaceutical interventions on diarrheal diseases and zoonotic Salmonella. hLife 2, 246–256 (2024).
Google Scholar
-
Li, Y. et al. A nontyphoidal Salmonella serovar domestication accompanying enhanced niche adaptation. EMBO Mol Med 14, e16366 (2022).
Google Scholar
-
Wang, H. et al. Paving the way for precise diagnostics of antimicrobial resistant bacteria. Front. Mol. Biosci. 9, 976705 (2022).
Google Scholar
-
Jia, C. et al. Avian-restrict Salmonella transition to endemicity is accompanied by localized resistome adaptation. Preprint at https://doi.org/10.1101/2024.07.26.605275 (2024).
-
Barrow, P. A. & Neto, O. C. F. Pullorum disease and fowl typhoid—new thoughts on old diseases: a review. Avian Pathology 40, 1–13 (2011).
Google Scholar
-
Li, Y. et al. Higher tolerance of predominant Salmonella serovars circulating in the antibiotic-free feed farms to environmental stresses. Journal of Hazardous Materials 438, 129476 (2022).
Google Scholar
-
Tang, B. et al. Genome-based risk assessment for foodborne Salmonella enterica from food animals in China: A One Health perspective. International Journal of Food Microbiology 390, 110120 (2023).
Google Scholar
-
Jia, C. et al. Mobilome-driven partitions of the resistome in Salmonella. mSystems 8, e00883–23 (2023).
Google Scholar
-
Gong, J. et al. Antimicrobial resistance, presence of integrons and biofilm formation of Salmonella Pullorum isolates from eastern China (1962–2010). Avian Pathology 42, 290–294 (2013).
Google Scholar
-
Hu, Y. et al. Loss and Gain in the Evolution of the Salmonella enterica Serovar Gallinarum Biovar Pullorum Genome. mSphere 4, e00627–18 (2019).
Google Scholar
-
Li, Y. et al. Genome-Based Assessment of Antimicrobial Resistance and Virulence Potential of Isolates of Non-Pullorum/Gallinarum Salmonella Serovars Recovered from Dead Poultry in China. Microbiol Spectr 10, e00965–22 (2022).
Google Scholar
-
Pan, H. et al. Comprehensive Assessment of Subtyping Methods for Improved Surveillance of Foodborne Salmonella. Microbiol Spectr 10, e02479–22 (2022).
Google Scholar
-
Van Dijk, E. L., Auger, H., Jaszczyszyn, Y. & Thermes, C. Ten years of next-generation sequencing technology. Trends in Genetics 30, 418–426 (2014).
Google Scholar
-
Alikhan, N.-F., Zhou, Z., Sergeant, M. J. & Achtman, M. A genomic overview of the population structure of Salmonella. PLoS Genet 14, e1007261 (2018).
Google Scholar
-
Bolger, A. M., Lohse, M. & Usadel, B. Trimmomatic: a flexible trimmer for Illumina sequence data. Bioinformatics 30, 2114–2120 (2014).
Google Scholar
-
Bankevich, A. et al. SPAdes: A New Genome Assembly Algorithm and Its Applications to Single-Cell Sequencing. Journal of Computational Biology 19, 455–477 (2012).
Google Scholar
-
Ellington, M. J. et al. The role of whole genome sequencing in antimicrobial susceptibility testing of bacteria: report from the EUCAST Subcommittee. Clinical Microbiology and Infection 23, 2–22 (2017).
Google Scholar
-
Simão, F. A., Waterhouse, R. M., Ioannidis, P., Kriventseva, E. V. & Zdobnov, E. M. BUSCO: assessing genome assembly and annotation completeness with single-copy orthologs. Bioinformatics 31, 3210–3212 (2015).
Google Scholar
-
Yoshida, C. E. et al. The Salmonella In Silico Typing Resource (SISTR): An Open Web-Accessible Tool for Rapidly Typing and Subtyping Draft Salmonella Genome Assemblies. PLoS ONE 11, e0147101 (2016).
Google Scholar
-
Schwengers, O. et al. Bakta: rapid and standardized annotation of bacterial genomes via alignment-free sequence identification: Find out more about Bakta, the motivation, challenges and applications, here. Microbial Genomics 7, (2021).
-
Page, A. J. et al. Roary: rapid large-scale prokaryote pan genome analysis. Bioinformatics 31, 3691–3693 (2015).
Google Scholar
-
Robertson, J. & Nash, J. H. E. MOB-suite: software tools for clustering, reconstruction and typing of plasmids from draft assemblies. Microbial Genomics 4, (2018).
-
Hua, X. et al. BacAnt: A Combination Annotation Server for Bacterial DNA Sequences to Identify Antibiotic Resistance Genes, Integrons, and Transposable Elements. Front. Microbiol. 12, 649969 (2021).
Google Scholar
-
Arndt, D. et al. PHASTER: a better, faster version of the PHAST phage search tool. Nucleic Acids Res 44, W16–W21 (2016).
Google Scholar
-
Jia, C. SG_Data. Figshare https://doi.org/10.6084/m9.figshare.26054251.v1 (2024).
-
NCBI, Sequence Read Archive https://identifiers.org/ncbi/insdc.sra:SRP526582 (2024).
-
WGS data of 42 strains of Salmonella Gallinarum isolated from Taishun and Yueqing, Zhejiang Province, China. Genbank https://identifiers.org/bioproject:PRJNA1143713 (2024)
-
Peñil-Celis, A. et al. Mobile genetic elements define the non-random structure of the Salmonella enterica serovar Typhi pangenome. mSystems e00365–24, https://doi.org/10.1128/msystems.00365-24 (2024).
-
Arnold, B. J., Huang, I.-T. & Hanage, W. P. Horizontal gene transfer and adaptive evolution in bacteria. Nat Rev Microbiol 20, 206–218 (2022).
Google Scholar
Acknowledgements
This work was supported by the National Program on the Key Research Project of China (2022YFC2604201), the Zhejiang Provincial Natural Science Foundation of China (LZ24C180002; LR19C180001), the Hainan Provincial Joint Project of Sanya Yazhou Bay Science and Technology City (2021JJLH0083), the Zhejiang Provincial Key R&D Program of China (2023C03045, 2022C02024) and the Open Project Program of the Jiangsu Key Laboratory of Zoonosis (R1902), and the European Union’s Horizon 2020 Research and Innovation Programme under Grant Agreement No. 861917 – SAFFI.
Author information
Authors and Affiliations
Contributions
Chenghao Jia: Conceptualization, Resources, Data curation, Formal Analysis, Methodology, Investigation, Supervision, Visualization, Validation, and Writing – original draft. Linlin Huang and Haiyang Zhou: Data curation, Formal Analysis, Investigation, Validation, and Visualization. Qianzhe Cao and Zining Wang: Formal Analysis and Investigation. Yan Li and Fang He: Writing – review & editing. Min Yue: Funding acquisition, Project administration, Supervision, Validation, Writing – review & editing.
Corresponding author
Ethics declarations
Competing interests
The authors declare no competing interests.
Additional information
Publisher’s note Springer Nature remains neutral with regard to jurisdictional claims in published maps and institutional affiliations.
Supplementary information
Supplementary Table1
Supplementary Table2
Supplementary Table3
Supplementary Table4
Supplementary Table5
Supplementary Table6
Supplementary Table7
Supplementary Table8
Rights and permissions
Open Access This article is licensed under a Creative Commons Attribution-NonCommercial-NoDerivatives 4.0 International License, which permits any non-commercial use, sharing, distribution and reproduction in any medium or format, as long as you give appropriate credit to the original author(s) and the source, provide a link to the Creative Commons licence, and indicate if you modified the licensed material. You do not have permission under this licence to share adapted material derived from this article or parts of it. The images or other third party material in this article are included in the article’s Creative Commons licence, unless indicated otherwise in a credit line to the material. If material is not included in the article’s Creative Commons licence and your intended use is not permitted by statutory regulation or exceeds the permitted use, you will need to obtain permission directly from the copyright holder. To view a copy of this licence, visit http://creativecommons.org/licenses/by-nc-nd/4.0/.
Reprints and permissions
About this article
Cite this article
Jia, C., Huang, L., Zhou, H. et al. A global genome dataset for Salmonella Gallinarum recovered between 1920 and 2024.
Sci Data 11, 1094 (2024). https://doi.org/10.1038/s41597-024-03908-7
-
Received: 03 July 2024
-
Accepted: 19 September 2024
-
Published: 07 October 2024
-
DOI: https://doi.org/10.1038/s41597-024-03908-7