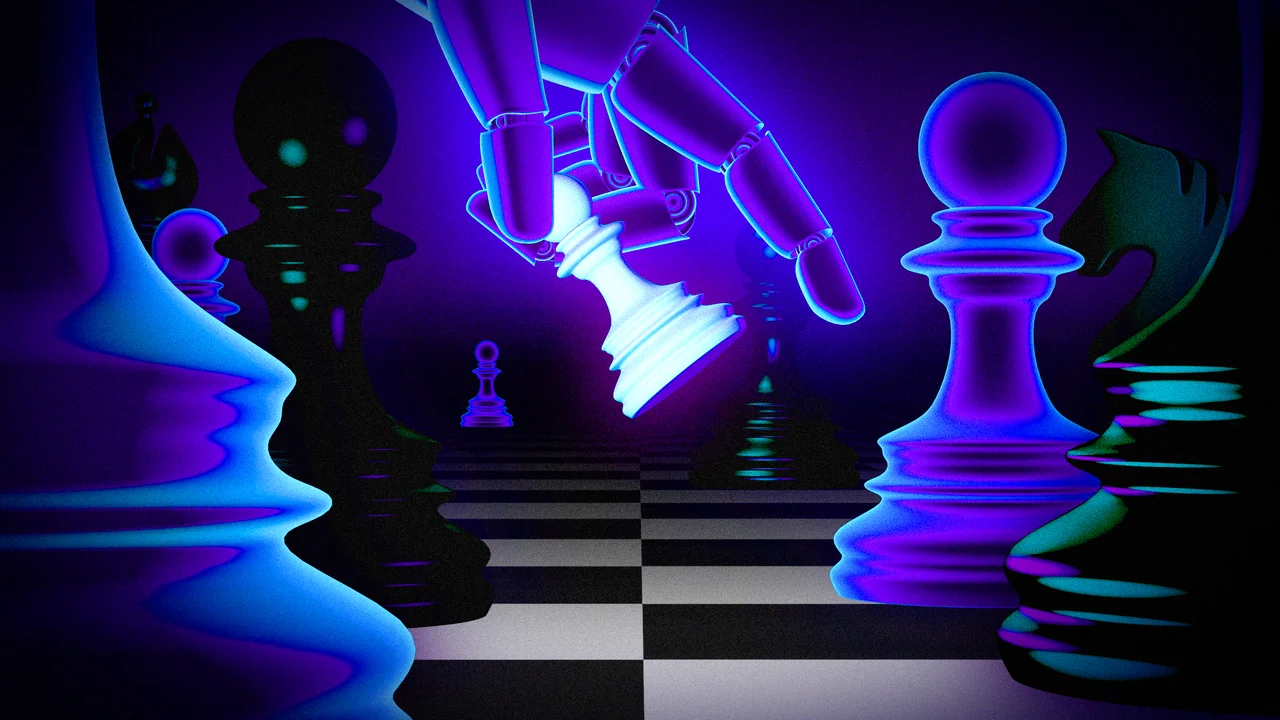
Can an A.I. Make Plans?
Today’s systems struggle to imagine the future—but that may soon change.
March 15, 2024
Last summer, AdamYedidia, a user on a Web forum called LessWrong, published a post titled “Chess as a Case Study in Hidden Capabilities in ChatGPT.” He started by noting that the Internet is filled with funny videos of ChatGPT playing bad chess: in one popular clip, the A.I. confidently and illegally moves a pawn backward. But many of these videos were made using the original version of OpenAI’s chatbot, which was released to the public in late November, 2022, and was based on the GPT-3.5 large language model. Last March, OpenAI introduced an enhanced version of ChatGPT based on the more powerful GPT-4. As the post demonstrated, this new model, if prompted correctly, could play a surprisingly decent game of chess, achieving something like an Elo rating of 1000—better than roughly fifty per cent of ranked players. “ChatGPT has fully internalized the rules of chess,” he asserted. It was “not relying on memorization or other, shallower patterns.”
This distinction matters. When large language models first vaulted into the public consciousness, scientists and journalists struggled to find metaphors to help explain their eerie facility with text. Many eventually settled on the idea that these models “mix and match” the incomprehensibly large quantities of text they digest during their training. When you ask ChatGPT to write a poem about the infinitude of prime numbers, you can assume that, during its training, it encountered many examples of both prime-number proofs and rhyming poetry, allowing it to combine information from the former with the patterns observed in the latter. (“I’ll start by noting Euclid’s proof, / Which shows that primes aren’t just aloof.”) Similarly, when you ask a large language model, or L.L.M., to summarize an earnings report, it will know where the main points in such documents can typically be found, and then will rearrange them to create a smooth recapitulation. In this view, these technologies play the role of redactor, helping us to make better use of our existing thoughts.
But after the advent of GPT-4—which was soon followed by other next-generation A.I. models, including Google’s PaLM-2 and Anthropic’s Claude 2.1—the mix-and-match metaphor began to falter. As the LessWrong post emphasizes, a large language model that can play solid novice-level chess probably isn’t just copying moves that it encountered while ingesting books about chess. It seems likely that, in some hard-to-conceptualize sense, it “understands” the rules of the game—a deeper accomplishment. Other examples of apparent L.L.M. reasoning soon followed, including acing SAT exams, solving riddles, programming video games from scratch, and explaining jokes. The implications here are potentially profound. During a talk at M.I.T., Sébastien Bubeck, a Microsoft researcher who was part of a team that systematically studied the abilities of GPT-4, described these developments: “If your perspective is, ‘What I care about is to solve problems, to think abstractly, to comprehend complex ideas, to reason on new elements that arrive at me,’ then I think you have to call GPT-4 intelligent,” he said.
Yet intertwined with this narrative of uneasy astonishment is an intriguing counterpoint. There remain some surprisingly simple tasks that continue to stymie L.L.M.s. In his M.I.T. talk, Bubeck described giving GPT-4 the math equation “7 x 4 + 8 x 8 = 92.” He then asked it to modify exactly one number on the left-hand side so that the equation would instead evaluate to 106. For a person, this problem is straightforward: change “7 x 4” to “7 x 6.” But GPT-4 couldn’t figure it out, and provided an answer that was clearly wrong. “The arithmetic is shaky,” Bubeck said.
How can these powerful systems beat us in chess but falter on basic math? This paradox reflects more than just an idiosyncratic design quirk. It points toward something fundamental about how large language models think. Given the predicted importance of these tools in our lives, it’s worth taking a moment to pull on this thread. To better understand what to expect from A.I. systems in the future, in other words, we should start by better understanding what the dominant systems of today still cannot do.
How does the human brain tackle a math problem like the one that Bubeck used to stump GPT-4? In his M.I.T. talk, he described how our thinking might unfold. Once we recognize that our goal is to increase the sum on the right side of the equation by fourteen, we begin searching for promising options on the left side. “I look at the left, I see a seven,” Bubeck said. “Then I have kind of a eureka moment. Ah! Fourteen is seven times two. O.K., so if it’s seven times two, then I need to turn this four into a six.”
To us, this type of thinking is natural—it’s just how we figure things out. We might overlook, therefore, the degree to which such reasoning depends on anticipation. To solve our math problem, we have to look into the future and assess the impact of various changes that we might make. The reason the “7 x 4” quickly catches our attention is that we intuitively simulate what will happen if we increase the number of sevens. “It was through some kind of planning,” Bubeck concluded, of his solution process. “I was thinking ahead about what I’m gonna need.”
We deploy this cognitive strategy constantly in our daily lives. When holding a serious conversation, we simulate how different replies might shift the mood—just as, when navigating a supermarket checkout, we predict how slowly the various lines will likely progress. Goal-directed behavior more generally almost always requires us to look into the future to test how much various actions might move us closer to our objectives. This holds true whether we’re pondering life’s big decisions, such as whether to move or have kids, or answering the small but insistent queries that propel our workdays forward, such as which to-do-list item to tackle next.
Presumably, for an artificial intelligence to achieve something like human cognition, it would also need to master this kind of planning. In “2001: A Space Odyssey,” the self-aware supercomputer HAL 9000 refuses Dave’s request to “open the pod bay doors” because, we can assume, it simulates the possible consequences of this action and doesn’t like what it discovers. The ability to consider the future is inextricable from our colloquial understanding of real intelligence. All of which points to the importance of GPT-4’s difficulty with Bubeck’s math equation. The A.I.’s struggle here was not a fluke. As it turns out, a growing body of research finds that these cutting-edge systems consistently fail at the fundamental task of thinking ahead.
Consider, for example, the research paper that Bubeck was presenting in his M.I.T. talk. He and his team at Microsoft Research ran a pre-release version of GPT-4 through a series of systematic intelligence tests. In most areas, the model’s performance was “remarkable.” But tasks that involved planning were a notable exception. The researchers provided GPT-4 with the rules of Towers of Hanoi, a simple puzzle game in which you move disks of various sizes between three rods, shifting them one at a time without ever placing a larger disk above a smaller one. They then asked the model to tackle a straightforward instance of the game that can be solved in five moves. GPT-4 provided an incorrect answer. As the researchers noted, success in this puzzle requires you to look ahead, asking whether your current move might lead you to a future dead end.
In another example, the researchers asked GPT-4 to write a short poem in which the last line uses the same words as the first, but in reverse order. Furthermore, they specified that all of the lines of the poem needed to make sense in both grammar and content. For example:
Humans can easily handle this task: the above poem, terrible as it is, satisfies the prompt and took me less than a minute to compose. GPT-4, on the other hand, stumbled. When Bubeck’s team asked it to attempt the assignment, the chatbot started its poem with the line “I heard his voice across the crowd”—an ill-advised decision that led, inevitably, to the nonsensical concluding line “Crowd the across voice his heard I.” To succeed in this poem-writing challenge, you need to think about writing your last line before you compose your first. GPT-4 wasn’t able to peer into the future that way. “The model relies on a local and greedy process of generating the next word, without any global or deep understanding of the task or the output,” the researchers wrote.
Bubeck’s team wasn’t the only one to explore the planning struggle. In December, a paper presented at Neural Information Processing Systems, a prominent artificial-intelligence conference, asked several L.L.M.s to tackle “commonsense planning tasks,” including rearranging colored blocks into stacks ordered in specific ways and coming up with efficient schedules for shipping goods through a network of cities and connecting roads. In all cases, the problems were designed to be easily solvable by people, but also to require the ability to look ahead to understand how current moves might alter what’s possible later. Of the models tested, GPT-4 performed best; even it was able to achieve only a twelve-per-cent success rate.
These problems with planning aren’t superficial. They can’t be fixed by making L.L.M.s bigger, or by changing how they’re trained. They reflect something fundamental about the way these models operate.
A system like GPT-4 is outrageously complicated, but one way to understand it is as a supercharged word predictor. You feed it input, in the form of text, and it outputs, one at a time, a string of words that it predicts will extend the input in a rational manner. (If you give a large language model the input “Mary had a little,” it will likely output “lamb.”) A.I. applications like ChatGPT are wrapped around large language models such as GPT-4. To generate a long response to your prompt, ChatGPT repeatedly invokes its underlying model, growing the output one word at a time.
To choose their words, language models start by running their input through a series of pattern recognizers, arranged into sequential layers. As the text proceeds through this exegetical assembly line, the model incrementally builds up a sophisticated internal representation of what it’s being asked about. It might help to imagine that the model has a vast checklist containing billions of possible properties; as the input text is processed by the model, it is checking off all of the properties that seem to apply. For example, if you provide GPT-4 with a description of a chessboard and ask it to make a move, the model might check off properties indicating that the input is about a game, that the game is chess, and that the user is asking for a move. Some properties might be related to more specific information, such as the fact that the board described in the input has a white knight on space E3; others might encode abstract observations, like the role that the white knight in space E3 is playing in protecting its king.
Once the input has been processed, the model must now apply what it’s learned to help select its next word. Here, it’s useful to extend our checklist metaphor to include a vast collection of guidelines for linking checked-off properties to specific words. In the newest generation of large language models, these guidelines can become impressively complicated. A model, upon learning that it’s dealing with a chess game, might consult its guidelines for chess, finding suggestions about how it can translate the locations of specific pieces into a pool of words that describe possible legal moves for those pieces. These potential moves might then be combined with guidelines based on other properties of the input, in order to identify which moves might be particularly good. For example, if the model has to choose whether to move a knight or a pawn, and it’s previously recognized that the knight is protecting the king, then a more general defense-minded guideline might be invoked, pushing the model toward moving the pawn.
In reality, of course, these A.I. systems don’t literally consult checklists or guidelines. They process data using billions of training-derived “parameters” organized into mathematical abstractions with names like “transformers” and “neural networks.” Still, these metaphors are close enough to serve our purposes; among other things, they explain why the best large language models can do more than simply mix and match existing text. When GPT-4 plays chess, it’s not blindly spitting out moves from vaguely similar boards encountered during its training. Instead, it has captured within its networks abstract ideas about how chess works, and it then uses the patterns implicit in these ideas to select its output. This approach allows the model not just to talk about chess but, in a crude sense, to actually play it. “Really, you shouldn’t think about it as pattern-matching and just trying to produce the next word,” Bubeck explained, in his M.I.T. talk. “Yes, it was trained to predict the next word—but what’s emerged out of this is a lot more than just a statistical pattern-matching object.”
Yet, for all their complexity, these language models still can’t handle basic planning tasks. Our metaphors can help us to resolve this paradox, too. The checklists and guidelines that define these models might be huge and intricate, but they are also static. They’re drawn up and optimized during expensive and time-consuming training processes, and can’t be modified in real time while they’re being pressed into action. This presents a problem for planning, because in order to plan you need to simulate futures relevant to the specific situation you’re currently facing. When you ask an L.L.M. to solve a Towers of Hanoi puzzle, it can recognize the question and produce a solution that looks good; its rule book might even have guidelines about specific sequences of moves that tend to work well. But, unless the model has been trained on this specific scenario, it cannot, as a human might, look ahead to eliminate the moves available now that will create a dead end later.
Chess poses a similar challenge. A closer look reveals that GPT-4’s performance tends to drop precipitously around twenty to thirty moves into the game—roughly the point in a standard chess match at which the board clears out and the position evolves into something novel. A human player tackles this middle part of a chess game through planning—simulating potential next moves, guessing what countermoves they might generate, and so on, in search of something that makes sense strategically. These are the stretches when chess masters stare intently at the board, sifting through various possible gambits in their minds. GPT-4, which cannot simulate the future, is left with a vast collection of heuristics for good chess moves that don’t apply directly to the current, unique board. As a result, it reverts to more haphazard play.
Just because large language models can’t simulate the future doesn’t mean that no computational system can. In 1996, when I.B.M.’s Deep Blue supercomputer first defeated the chess grandmaster Garry Kasparov, it relied heavily on its ability to evaluate hundreds of millions of potential future moves for every game position that it encountered. Twenty years later, DeepMind’s AlphaGo system defeated the champion Go player Lee Sedol, a victory that likewise depended on the system’s ability to consider vast numbers of possible upcoming moves. Models like GPT-4 might be static, but well-known game-playing systems such as Deep Blue and AlphaGo have always been fundamentally interactive.
A natural question is whether these two distinct competencies—understanding and imagination—could be combined. Not long ago, a team from Meta’s A.I. division, co-led by a precocious computer scientist named Noam Brown, set out to attempt exactly this. Brown first achieved prominence in the A.I. community for Libratus, a poker bot that he co-created with Tuomas Sandholm, his doctoral adviser at Carnegie Mellon. In 2017, Libratus became the first computer to beat professional poker players in two-player competition, earning Brown and Sandholm the Marvin Minsky Medal for Outstanding Achievements in A.I. for their efforts. In 2019, Brown and Sandholm, now working with engineers at Meta, surpassed this feat with the introduction of Pluribus, the first poker bot to win against professionals in multiplayer poker.
Fresh off the success of Pluribus, Brown went searching for a harder challenge—one that would require not just the abstract strategic thinking needed to win a game like poker but also the ability to excel in the messier world of unstructured human interaction. He soon turned his attention to Diplomacy, a cult-classic strategy game. Diplomacy is a turn-based war simulation in the style of Risk; it unfolds on a game board depicting Europe before the First World War, divided into unique land and sea territories. Players control armies and naval fleets, which they can move around the board, attacking other players’ forces and attempting to conquer nearby territories. The game ends when either one player controls a majority of the map’s supply centers or all the remaining players agree on a draw.
What distinguishes Diplomacy from similar war games is how the rounds unfold. Before the players commit to their moves, they hold private one-on-one conversations with each of the other players. During this negotiation period, they can propose alliances, make threats, or hatch devilishly complicated feints and double crosses. Once the negotiations conclude, players record their moves for the given round and pass them to an arbiter who executes them, revealing who was true to their word and who has dealt a betrayal. In order to succeed, Brown has said, you have to recognize that players “might be lying to you when they say they’re going to support you”—and you must also sometimes lie. In this way, winning Diplomacy is not just about how you move your pieces but about how you navigate complicated relationships. This dual experience is so strategically realistic that it gave rise to a popular (though likely apocryphal) urban legend claiming that Henry Kissinger played Diplomacy in the Kennedy White House, to help train his statecraft.
To handle the communication-related aspects of Diplomacy, Brown’s team started with an off-the-shelf large language model called BART, which had been pre-trained on text sourced from the Internet. The researchers then refined this training with a large collection of player-to-player messages taken from real games of Diplomacy held online. The goal was to teach the model not only to speak in the lingo of the game but also to become more adept at understanding the sometimes hidden intentions of the players sending messages. Consider the following message, sent in a real game, from the player controlling Italy to the player controlling Russia: “Sounds good to me. How are you feeling about Turkey and Australia? If we decide to work against one of them first, I’m totally on board.” Brown’s team wanted their model to be able to succinctly summarize this text as a request by Italy for an alliance against either Turkey or Australia. Such comprehension is challenging—but it’s also exactly the type of challenge that language models have proved adept at conquering.
Though the research team trusted their L.L.M. to understand conversations with other Diplomacy players, they didn’t trust it to actually come up with smart strategies in response to these interactions. “The language model is doing fuzzy pattern-matching, trying to see things seen in training data, and then copying something similar to what was said,” Mike Lewis, a Meta engineer who worked on the project, told me. “It is not trying to predict good moves.” As his colleague Emily Dinan, who also worked on the project, put it, “We tried to relieve the language model of most of the responsibility of learning which moves in the game are strategically valuable, or even legal.” This responsibility was instead placed into a future-oriented planning engine of the sort more typically deployed in a poker or chess bot.
In the resulting system, the language model passes annotated versions of the messages it receives to the planning engine, which uses this information to help simulate possible strategies. Should it trust Italy’s suggestion to help it invade Turkey? Or is the suggestion to invade Australia better? What if Italy is being dishonest? The planning engine explores countless ways forward, integrating many different assumptions about the human players’ allegiances and potential for betrayal. Once it decides on a plan that maximizes its chance for success, it instructs the L.L.M. on what it wants from the other players; the model then turns these terse descriptions into convincing messages.
Brown’s team called their system Cicero, and tested its skill against real players on WebDiplomacy, a popular online game server. Their initial version of Cicero wasn’t superhuman in the manner of Pluribus, AlphaGo, or Deep Blue, but it more than held its own: in a two-month period, it participated in forty time-limited games, known as “blitz” matches, and ranked second out of nineteen participants who played in five or more games. Strikingly, the researchers couldn’t find any examples of in-game messages indicating that the human players suspected Cicero of being an A.I.
Today, for many people, L.L.M.-powered tools such as ChatGPT are synonymous with A.I. But Cicero suggests a broader reality. The future of artificial intelligence may not depend on the pursuit of increasingly complicated large language models but instead on the development of nuanced connections between these models and other types of A.I. Cicero combined language and game strategy, but future systems might draw on more general planning abilities, allowing a chatbot to create a smart plan for your week or navigate tricky interpersonal dynamics in responding to your e-mails. Stretch these possibilities further, and we might even arrive at a real-world HAL 9000, capable of pursuing goals in flexible (and perhaps terrifying) ways. The promise of this ensemble approach is reflected in the fact that the big players are already investing heavily in non-language-based forms of digital intelligence. Not long after Brown’s success with Cicero, for example, OpenAI hired him away from Meta to help integrate more planning into its popular language-model-based tools.
If 2023 was the year when we learned that language models could do more than simply mix and match existing text, then this year might be when we learn that the power of linguistic A.I. is nonetheless still limited. The idea that L.L.M.s can’t do everything might surprise many of us—especially after we’ve heard so many breathless prognostications about a GPT-powered future. But it’s well understood among researchers. In December, Yann LeCun, the head of A.I. at Meta, tweeted that language models “will disappear in a few years,” adding, “Future AI systems will use a different blueprint.” Many researchers believe that it may be a descendent of Cicero, rather than GPT-5, that sparks the next big disruptions.
How powerful might these combination systems be? Where we’ll end up on a scale from board games to HAL 9000 depends on how broad and how adaptable planning-oriented A.I. can become. At the moment, most of these systems are dedicated to winning games. Brown’s work on poker and Diplomacy was particularly impressive because his models take into account the beliefs and psychology of other players—but those players are still operating in a constrained setting, with clear rules. Strategizing about everyday life would require another leap in complexity. It may not even be possible. Mike Lewis, one of the Meta engineers who worked on Cicero, seemed skeptical when I asked about the possibility of a more generalized, human-style planning A.I. “These planning systems, like we used in Diplomacy, work very well in limited situations like games, and might also work for math reasoning,” he said. “They haven’t worked so well in general situations.” At the same time, however, he qualified his skepticism. “People disagree on this,” he said. Lewis’s uncertainty is typical. In our urgent study of how much machines can really know, it’s our own lack of knowledge that worries us most. ♦