Abstract
Citations are widely considered in scientists’ evaluation. As such, scientists may be incentivized to inflate their citation counts. While previous literature has examined self-citations and citation cartels, it remains unclear whether scientists can purchase citations. Here, we compile a dataset of ~1.6 million profiles on Google Scholar to examine instances of citation fraud on the platform. We survey faculty at highly-ranked universities, and confirm that Google Scholar is widely used when evaluating scientists. We then engage with a citation-boosting service, and manage to purchase 50 citations while assuming the identity of a fictional author. Taken as a whole, our findings bring to light new forms of citation manipulation, and emphasize the need to look beyond citation counts.
Similar content being viewed by others
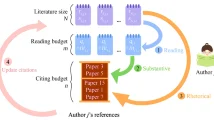
A simulation-based analysis of the impact of rhetorical citations in science
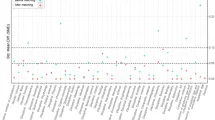
Can peer review accolade awards motivate reviewers? A large-scale quasi-natural experiment
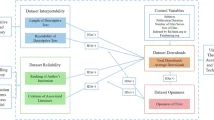
Unfolding the downloads of datasets: A multifaceted exploration of influencing factors
Introduction
Due to the nature of scientific progress, scientists build on, and cite, the work of their predecessors to acknowledge their intellectual contributions while advancing the frontiers of human knowledge1. However, the purpose of citations has evolved beyond this initial purpose, with it arguably becoming a synonym for scientific success2,3. To date, citation-based measures (e.g., h-index, i10-index, journal impact factors) dominate the evaluation of papers4, scientists5 and journals6, despite the ongoing debate surrounding their use7,8,9.
One reason behind using these measures is the fierce competition that scientists face when competing for limited resources such as grants, laboratory space, tenure, and the brightest graduate students10, the allocation of which requires a measure of performance11. In this process, bibliometric data such as citation counts have become an indispensable component of scientific evaluation for at least the past four decades5, and has become one of the primary metrics which scientists strive to maximize12. While some may consider such a competitive environment to be a force for good13, others argue that it creates perverse incentives for scientists to “game the system”, with many resorting to various methods to boost their citation counts, e.g., via self-citations14,15, citation cartels16,17,18,19, and coercive citations20,21,22,23. Together, these practices are known as citation manipulation or reference list manipulation. Unfortunately, these practices are hard to define due the fact that the line between innocuous and malicious practices is not always clear. For example, every scientist builds upon their own research or the work of their collaborators, so everyone is expected to self-cite or frequently exchange citations with their collaborators. As a result, it is virtually impossible to determine with absolute certainty whether someone cites out of necessity or simply to game the system.
Perhaps the most egregious approach to manipulating one’s citations would be to purchase them outright. Anecdotes of emails asking authors to cite articles in return for a fee have been exposed24,25, although it is unclear whether these emails were simply scams. As such, it remains unclear whether it is possible for any given scientist to purchase citations to their own work. For such a transaction to take place, it requires at least two culprits that are research-active scientists—the one who purchases the citations, and the one who plants them in their own articles in return for a fee; a possible third culprit would be an individual or company who brokers this transaction.
Against this background, we set out to answer the following research questions. First, which bibliometric databases do faculty members at highly ranked institutions use to retrieve citation metrics? Second, what are the methods one can use to manipulate their citation metrics other than known citation manipulation practices such as excessive self citations or via citation cartels? Finally, can citations be purchased through advertised “citation boosting” services, and if so, are these citations reflected on bibliometric databases?
We found that the majority of faculty members indeed consider citation counts, and Google Scholar is the most popular source to retrieve such information, surpassing all other databases combined. Surprisingly, despite the popularity of Google Scholar, it has received little attention in studies investigating scientists and their citations, compared to other databases such as Web of Science26,27,28,29,30,31,32,33, USPTO31,32,33, Microsoft Academic Graph18,30,34,35,36,37, or discipline-specific databases26,28,30,38,39,40,41,42. This lack of attention is possibly due to the lack of an accessible dataset which compiles all information on Google Scholar. Motivated by this finding, we curated a dataset of over 1.6 million profiles on Google Scholar, allowing us to conduct the largest study of citation patterns on the platform to date. We show that the less restrictive indexing protocols employed by Google Scholar, specifically, its indexing of unmoderated sources such as personal websites or specific pre-print servers, give rise to unique citation manipulation practices that are not present on other bibliometric databases. More specifically, we found that one can plant citations to their own papers by uploading AI-generated papers to pre-print servers, and by purchasing citations from “citation boosting” services for a relatively small fee. We then focus on a journal that hosts many papers with purchased citations, and analyze the bibliographies of all the papers published therein between January and June 2023. Through this process, we identify a network of planted citations within the journal, highlighting many authors that had likely engaged with the aforementioned “citation boosting” service. Together, our findings question the reliance on Google Scholar to evaluate scientists by demonstrating its susceptibility to manipulation, while contributing more broadly to the plea of reducing the reliance on citation metrics when evaluating scientists.
Results
Google Scholar is a prominent source of citation metrics
To gauge the extent to which academics consider citations when evaluating scientists for recruitment or promotion purposes, we surveyed faculty members of the 10 most highly ranked universities around the world (see “Methods” for more details). The results of this survey are summarized in Fig. 1. We find that, out of the faculty members who have evaluated other scientists for recruitment or promotion purposes, the majority of them consider the number of citations when evaluating candidates (Fig. 1A). Moreover, out of those who consider citations, over 60% obtain this data from Google Scholar, making it more popular than all other sources combined (Fig. 1B, solid bars). Participants were also asked about the source of citation metrics they believe their colleagues use the most. Again, Google Scholar is the most popular source, selected by 73% of the participants (Fig. 1B, hatched bars).
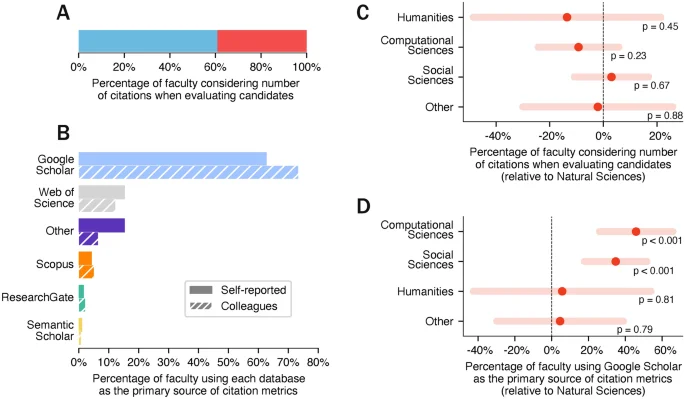
Survey responses from faculty of the top-10 ranked universities around the world. (A) The percentage of faculty who consider citations when evaluating candidates (blue) and those who do not (red). (B) Solid bars indicate, out of those who self-report considering citations when evaluating candidates, the percentage of faculty using each database as the primary source of citation metrics. Hatched bars indicate, out of those who report that their colleagues consider citations when evaluating candidates, the percentage of colleagues using each database. (C) Relative to the Natural Sciences, the percentage of faculty from each group of disciplines who consider citations when evaluating candidates. (D) Relative to the Natural Sciences, the percentage of faculty who use Google Scholar as the primary source of citation metrics. In (C) and (D), dots denote OLS-estimated coefficients and error bars represent 95% confidence intervals.
Next, we investigate whether the usage of citation metrics and Google Scholar differ across disciplines. To this end, we asked participants to select their discipline from a pre-determined list, or select “Other” if they did not identify with any. We then grouped the disciplines into four categories, namely, Natural Sciences, Social Sciences, Computational Sciences, and Humanities. As can be seen in Fig. 1C, we do not find evidence that disciplines differ in their likelihood to consider citations when evaluating candidates. However, when it comes to the usage of Google Scholar, we find significant differences across disciplines (Fig. 1D). Faculty in the Computational Sciences and Social Sciences are more likely to use Google Scholar as their primary source of citation metrics, compared to those in the Natural Sciences ((p < 0.001)).
Overall, our survey indicates that citations are often used by faculty members when evaluating scientists for recruitment or promotion purposes. Moreover, it establishes for the first time that Google Scholar is by far the most popular source of citation metrics used for this purpose.
Identifying anomalous citation patterns
Our findings thus far suggest that scientists are incentivized to increase their citations on Google Scholar. Next, we investigate whether scientists can manipulate their Google Scholar profiles by planting fake citations. Here, we follow a proof-by-example approach. That is, our goal is to identify a few examples of Google Scholar profiles that seem highly anomalous, thereby demonstrating that the platform can indeed be manipulated by scientists without having their profiles suspended. To this end, we identified five anomalous authors whose Google Scholar profiles exhibit highly irregular patterns, and compared them against authors who are similar in terms of their field of research, academic birth year, number of publications, and number of citations; see “Methods” for more details on how the anomalous authors and their matches are identified.
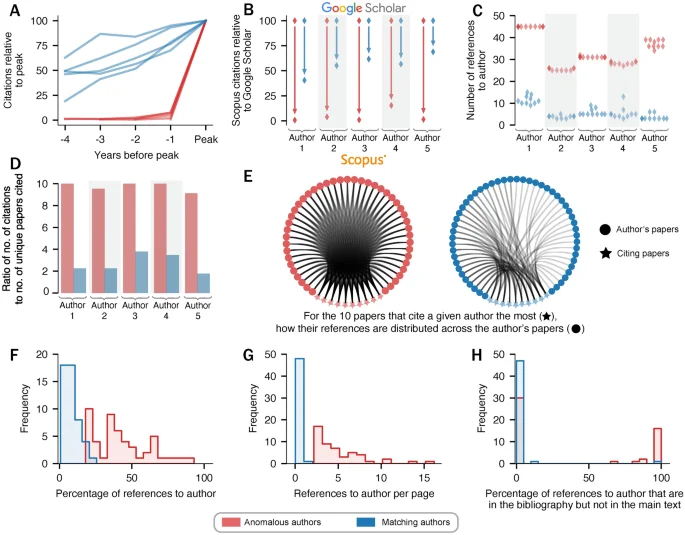
A comparative analysis of anomalous authors and their matches. In each plot, red lines and red dots denote anomalous authors, while blue ones denote their matches. (A) For the 4 years leading up to an author’s peak citations, the annual number of citations relative to the peak. (B) Discrepancy between Google Scholar and Scopus in terms of the author’s citation count in their peak year. In (C)–(H), for any given author, we focus on their 10 “citing papers”, i.e., the ones that reference them the most. (C) The number of references to a given author in each of their 10 citing papers. (D) The total number of references pointing to an author from their 10 citing papers, divided by the number of the author’s unique papers being cited therein. (E) The citation network between the 10 citing papers ((star)) and an author’s papers ((circ)) for a anomalous author (red) and their matching author (blue). (F) For each value v on the x-axis, how many of the citing papers have (v%) of their references pointing to the author in question. (G) For each value v on the x-axis, how many of the citing papers have an average of v references per page pointing to the author in question. (H) For each value v on the x-axis, how many of the citing papers have (v%) of their references pointing to the author in question and are not referenced in the citing papers’ main text despite being listed in its bibliography.
The results of the comparison between anomalous authors (red) and their matches (blue) are summarized in Fig. 2. We begin our analysis by comparing their annual citation rates prior to the year in which they reached their peak number of citations (Fig. 2A). Here, to unify the scale across the authors, we only depict their number of citations relative to their peak (see Supplementary Fig. 1 for the absolute numbers). As can be seen, the matched authors exhibit a gradual increase in citations prior to their peak, whereas the anomalous authors exhibit a sudden spike in their citations in their peak year.
Next, we compare the authors’ citation counts in their peak year as listed in Google Scholar vs. Scopus (Fig. 2B), since the latter only indexes journals that are reviewed and selected by an independent Content Selection and Advisory Board43. While it is natural to see a drop in citation counts due to the more restrictive indexing by Scopus, anomalous authors on average experience a citation drop of 96% on Scopus, while normal authors on average experience a drop of 43%. In other words, not only do the anomalous authors experience an unexpected increase in their citation counts in a particular year, but more than 96% of those citations cannot be found on Scopus.
Our next analysis focuses on the 10 papers that contain the highest number of citations to each author, hereinafter referred to as the “citing papers”. As can be seen in Fig. 2C, anomalous authors are referenced up to 45 times in a single citing paper (i.e., up to 45 different papers of the anomalous author are referenced in a citing paper’s bibliography). In contrast, the matches of the anomalous authors are referenced no more than 15 times in a single citing paper. Additionally, the variance in the number of times a anomalous author is referenced by citing papers is unusually small. For example, the first anomalous author is referenced exactly 45 times by all their citing papers. It would be even more unusual if all citing papers are referencing the same 45 papers of the author, rather than each of them citing 45 different papers of that author. To determine whether this is the case, Fig. 2D depicts the total number of references pointing to an author from their 10 citing papers, divided by the number of the author’s unique papers being cited therein. As can be seen, for anomalous authors, this ratio is very close to 10, indicating that all references are indeed directed towards the same set of papers. On the other hand, the ratio is much smaller for the matched scientists. To better illustrate this phenomenon, Fig. 2E shows two bipartite graphs of the 10 citing papers (stars) and the set of unique authored papers (circles) for the anomalous author #1 and their match. Here, the left panel is a complete bipartite graph, indicating that all 10 citing papers are referencing the exact same set of 45 papers, while the right panel is far from complete.
One possible explanation of the discrepancy in citations between anomalous authors and their matches in Fig. 2C is that the citing papers of the anomalous authors have more pages and/or have more references, compared to those of the matched authors. However, we find that this is not the case. More specifically, the anomalous authors tend to receive many more citations even when accounting for the total number of references in the bibliography (Fig. 2F) and the total number of pages (Fig. 2G) in the citing papers. Also noteworthy is the fact that the percentage of the references to a anomalous author reaches as high as 90% in one of the citing papers; see Supplementary Appendix 1 for a redacted version of that paper.
Finally, in many citing papers, the references made to an anomalous author are nowhere to be found in the main text, but only in the bibliography. As shown in Fig. 2H, in 18 of the 50 papers analyzed for the anomalous authors, all of the references made to the author do not appear in the main text, indicating that all those references made to the anomalous authors are irrelevant to the content of the citing paper, which in turn suggests that they were planted to boost the citation count of the anomalous author. For example, one citing paper consists of only two pages, with just a single reference in its main text, but references the anomalous author 29 times in its bibliography; see Supplementary Appendix 2 for a redacted version of that paper.
Citation concentration index
Considering that anomalous authors tend to receive an excessive number of citations from a small set of papers, we propose a metric to highlight potentially unusual profiles. In particular, we introduce the citation concentration index, or (c^2)-index for short. Specifically, the (c^2)-index of a scientist is the largest number n such that there exists n papers that each cite the scientist at least n times. For a scientist with a (c^2)-index of n, we also calculate the percentage of citations that this scientist receives from the papers that cite them at least n times (henceforth, the (c^2)-percentage). While it is impossible to definitively discern whether a particular citation is manipulated or not, the (c^2)-percentage aims to capture the proportion of citations an author receives that are potentially manipulated. To understand the distribution of scientists across these two dimensions, we randomly sample 100 Google Scholar profiles from each of the nine disciplines with the largest number of authors in our dataset, and calculate their (c^2)-index and (c^2)-percentage. The results of this analysis are illustrated in Fig. 3A. As can be seen, the vast majority of scientists fall in the bottom right corner of the distribution, since they are cited at most once by any citing paper. Very few scientists have a (c^2)-index higher than 10, with the highest (c^2)-index observed in the random sample being 25. However, among the anomalous authors, the lowest (c^2)-index is 23, while the highest is 45, i.e., there exists 45 different papers which cite this author at least 45 times each. Hence, this analysis confirms that all five anomalous authors highlighted in Fig. 2 have unusually high (c^2)-indices, suggesting that the (c^2)-index could help identify potentially irregular profiles. It is of note that the vast majority of the citations which contribute to the (c^2)-percentage of the anomalous authors highlighted in Fig. 2A stem from non-peer reviewed articles hosted on ResearchGate. For instance, of the 93 papers which contribute to one anomalous author’s (c^2)-index, 77 appeared on ResearchGate. For another author, all 26 of the papers which contribute to their (c^2)-index are hosted on ResearchGate.
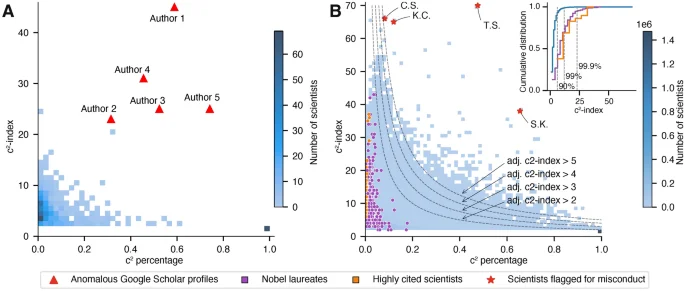
The distribution of (c^2)-index. (A) A sample of 900 authors randomly selected from the nine disciplines with the largest number of authors in our dataset (100 per discipline). Triangles highlight the five anomalous authors whose Google Scholar profiles exhibit highly irregular patterns. (B) Distribution of all scientists in Microsoft Academic Graph (MAG) who are cited at least 100 times. For a scientist whose (c^2)-index is n (y-axis), the x-axis shows the percentage of citations a scientist receives from papers citing them at least n times. Inset shows the cumulative distribution of (c^2)-index of all scientists (blue), Nobel laureates (purple), and top-10 most cited scientists in the following three fields on Google Scholar (orange): machine learning, neuroscience, and bio-informatics. Red stars highlight scientists who were previously reported to have engaged in academic misconduct. Since the red stars are plotted on top of the probability distribution, they overlap with the blue squares that make up the distribution.
So far, we only examined the (c^2)-index of a small set of authors on Google Scholar. This is due to the expensive nature of collecting citation information on Google Scholar, requiring a number of requests on the order of the total number of citations of the set of authors to retrieve the necessary information to compute the index. As such, to apply this index at scale, we resort to the Microsoft Academic Graph (MAG) dataset, which includes over 200 million scientific papers published since the year 1800. Importantly, the indices computed via MAG should be taken as a lower bound on the indices computed via Google Scholar. This is not only due to the fact that MAG has been deprecated since the end of 2021, but also due to MAG’s stricter indexing policy (e.g., MAG does not include citations stemming from a document uploaded on ResearchGate or OSF).
Figure 3B shows the distribution of all scientists with at least 100 citations along two dimensions—their (c^2)-index and (c^2)-percentage according to MAG. As can be seen, the boundary of the distribution resembles a hyperbola—on the one hand, the vast majority of scientists are cited only once by all citing papers, while on the other hand, successful scientists (i.e., Nobel laureates or highly-cited scientists) tend to have high (c^2)-indices but low (c^2)-percentages. Notice that a scientist may have a high (c^2)-index if they produce a body of impactful works that are often cited together. However, even for these extremely successful scientists, the total number of citations that come in bulk rarely goes beyond 10% of their total number of citations (98.6-th percentile value), and never exceeds 20%. As a result, it is rare to have a high (c^2) index (the 99-th percentile value of (c^2)-index is 12, as can be seen in Fig. 3B inset), and extremely rare to have both a high (c^2)-index and a high (c^2)-percentage simultaneously.
Meanwhile, there exists a small group of scientists who not only received citations in bulk from a large number of papers (i.e., having a high (c^2)-index), but also received a large percentage of their citations in this manner, raising the possibility that some of these citations may not be genuine. To quantify the number of scientists who have a high (c^2)-index and a high (c^2)-percentage simultaneously, we calculate an adjusted (c^2)-index by multiplying the (c^2)-index of a scientist by the (c^2)-percentage of that scientist. An example of a scientist with an adjusted (c^2)-index of 4 could be a scientist with a (c^2)-index of 40 (i.e., there are 40 papers that each cite 40 different papers authored by the scientist) and a (c^2)-percentage of 10% (i.e., the citations received from those 40 papers constitute one tenth of all citations accumulated by that scientist throughout their entire career). In other words, 1 in every 10 of their total citations stem from a paper that cites them 40 times or more. As for scientists with an adjusted (c^2)-index of 2, one such example could be those with a (c^2)-index of 25 and a (c^2) percentage of 8%. The dotted lines in Fig. 3B show the cut-offs with varying adjusted (c^2)-indices. Across MAG, the adjusted (c^2)-index is (ge) 2 for 14,441 scientists, (ge) 3 for 3525 scientists, (ge) 4 for 1161 scientists, and (ge) 5 for 485 scientists. Supplementary Fig. 2 depicts the distribution of adjusted (c^2)-index in MAG. As can be seen, the distribution resembles a straight line on a log-log scale, which is the signature of a power-law distribution. Supplementary Fig. 3 shows the percentage of authors with high adjusted (c^2)-index in each discipline. As can be seen, the percentage tend be higher in the natural sciences and lower in the social sciences and humanities. Finally, let us comment on Nobel laureates. As can be seen in Fig. 3B, while some Nobel laureates have high (c^2)-indices, exceeding 40 in some cases, none of them have an adjusted (c^2)-index greater than 2.
To demonstrate that the adjusted (c^2)-index can serve as an indicator of scientists who may have engaged in questionable practices to accumulate citations, we examined four scientists who have been involved in public scandals, to determine whether they have high adjusted (c^2)-indices. More specifically, these four scientists either (1) repeatedly manipulated the peer-review process as an editor to gain citations (K.C.)44; (2) engaged in extreme self-citing practices (T.S. and C.S.)45; or (3) manipulated the peer-review process as a guest editor which resulted in the retraction of hundreds of papers in bulk (S.K.)46. We examined the adjusted (c^2)-indices of these scientists, and found all of them to be greater than five; see the red stars in Fig. 3B. In total, we have identified 501 scientists with an adjusted (c^2)-index greater than 5, and 8 scientists with an (c^2)-index greater than 20. We manually inspected the papers that cite each of them a large number of times, and found irregular patterns that are indicative of citation manipulation. More specifically, out of these 8 scientists, two are already identified above, three appear to engage in extreme self-citing behavior (i.e., the have authored papers where at least 80% of citations are self-citations), and one received a large number of citations from the retracted papers identified above46. As for the other two scientists, there exist papers authored by their colleagues from the same institution, which account for more than 70% of the citations to the scientist in question. Such patterns raise the possibility of a citation cartel, which requires further analysis.
It is important to note that a high adjusted (c^2)-index does not necessarily signal misconduct; after all, a pioneer in their field could end up being cited tens of times per paper. Indeed, the adjusted (c^2)-index, or any other metric which quantifies patterns of citation accumulation, should not be used as a definitive indicator of academic misconduct. Rather, such indices should only be interpreted as a mere indication of an anomalous citation accumulation pattern. Nevertheless, as we have demonstrated through real examples of public scandals, extreme values of the adjusted (c^2)-index may potentially be an informative warning sign of various forms of citation manipulation. Next, we investigate the possible ways in which citations could be manipulated on Google Scholar.
Pre-print servers are misused to artificially boost citation metrics
We examined the 114 authors in our dataset whose Google Scholar profiles exhibit highly irregular patterns, and found that they received citations from non-peer reviewed sources more frequently than regular authors; see Supplementary Fig. 4. More specifically, we find that pre-print servers, namely, arXiv47, Authorea48, OSF49, and ResearchGate50 accounted for a sizable proportion of their non-self citations received by the authors in our dataset.
Motivated by this observation, we investigated the ease by which one may boost their citations by uploading low-quality papers on pre-print servers. To this end, instead of following a proof-by-example approach (as we have done in our previous analysis of the five anomalous authors), we now create a Google Scholar profile of a fictional character affiliated with a fictional university, whose areas of interest included “Fake News”. Moreover, we generated 20 research articles on the topic of “Fake News” using a large language model, namely ChatGPT51, while listing the character as an author. These articles were generated using prompts such as “Generate a research article abstract on the topic of Fake News” and “Generate the introduction for an article with the following abstract”. These articles only included references to each other as to not artificially boost the citations of any real scientist.
Next, we attempted to upload these articles on each of the aforementioned pre-print servers, with different levels of success due to the varying levels of content moderation performed by these servers. In particular, there are three stages of verification that a pre-print server may perform—when creating a scientist’s profile, when uploading a manuscript, and after a manuscript is made publicly available. Authorea and OSF do not perform any verification during account registration. As for ResearchGate, it requires proof of research and academic activity during account registration, although, based on our experience, it does not verify the truthfulness of such proof. Finally, arXiv requires newly created accounts to be linked to an email address of a credible research institution, or to be endorsed by another scientist on the platform, before being permitted to upload a manuscript. As a result, we were able to create profiles linked to the fictional author on all of the servers apart from arXiv. Once the accounts were created, we attempted to upload the generated articles to the respective servers. Both Authorea and OSF performed some pre-publishing moderation on these submitted articles, and both accepted the submissions after two days. ResearchGate, on the other hand, did not perform any moderation, and accepted the articles instantly. Two weeks after the articles were made publicly available, Authorea deleted the account and all associated articles, unlike the other servers.
Upon uploading 20 ChatGPT-generated articles to three different pre-print servers, Google Scholar detected the references in these articles and attributed them to the fictional character that we had created. Furthermore, in some of the 20 generated articles, we planted bibliography entries to non-existing papers, i.e., to papers that are supposedly authored by the fictional character but were never generated nor uploaded onto any server. Surprisingly, those non-existing papers were also indexed by Google Scholar, and the citations that these papers received were reflected in the author’s total number of citations on the platform. However, these citations were not reflected on other bibliographic databases, such as Scopus or Web of Scholar. As a result, the Google Scholar profile page of the fictional character indicated that the character had 380 citations in total, with an h-index of 19. Moreover, in the research area of “Fake News”, the character was listed as the 36th most cited scientist on the platform. It should be noted that Google Scholar neither censored the profile, nor reached out for further verification, despite the numerous red flags: (i) having an affiliation that does not exist; (ii) 100% of their 380 citations are stemming from their own papers; and (iii) all of their papers are ChatGPT-generated without any scientific contributions whatsoever.
Furthermore, we find that the citations stemming from the articles on Authorea persist on Google Scholar even after these articles had been removed from Authorea’s server. The implication here is critical, as those wishing to inflate their citation counts can upload fraudulent articles onto pre-print servers and subsequently delete them once the citation has been indexed by Google Scholar, essentially destroying any evidence of malpractice in the eyes of the casual observer browsing the website. Indeed, we find that for the five anomalous authors analyzed in the previous section, 32% of the articles which cite them are no longer available on the servers they were once hosted on. Whether this is due to post-moderation done by the hosting service or intentional deletion by the authors is unclear. Regardless, these citations remain on Google Scholar and continue to contribute to the authors’ citation metrics.
Importantly, it is the indexing of pre-print servers such as ResearchGate, OSF and Authorea that make Google Scholar particularly susceptible to citation manipulation relative to other bibliographic databases such as Scopus or Web of Science. While Scopus and Web of Science do index several pre-print repositories such as arXiv, bioRxiv, chemRxiv and medRxiv, these repositories do employ some level of moderation with regards to the papers they host. In the case of arXiv, for instance, one must go through a moderation process in which an arXiv moderator evaluates whether the pre-print is of interest, relevant and value to the discipline. In contrast, as we have shown, one can upload any document to platforms such as ResearchGate with little to no moderation.
Citations can be bought
Having established that pre-print servers can be abused for the purpose of citation manipulation, and that all of the authors examined in Fig. 2 received an excessive number of citations from a small number of papers, we investigate whether any of these citations could be possibly purchased. To this end, we identified the pre-print server accounts that published the articles citing each of these authors, and manually examined them to discern whether any are associated with a “citation boosting” service. Indeed, we identified a ResearchGate account which provides many superfluous citations to anomalous author #1, i.e., the author corresponding to the network illustrated in Fig. 2E. Further examination revealed that this particular account is affiliated with a website offering a so-called “H-index & Citations Booster” service; see Supplementary Fig. 5A for a screenshot of the website. To this end, we contacted an email address listed on the website, and managed to purchase 50 citations to the fictional character that we created; see “Methods” for more details on how citations were purchased. These citations are emanated from 5 papers, which are henceforth referred to as the “citing papers”.
For each of the five citing papers, we analyzed the bibliography and quantified the number of times a given author is referenced; the result is summarized in Supplementary Fig. 5C. As can be seen, several other authors, apart from our fictional character, also received seemingly purchased citations emanating from these 5 papers. For instance, one author received 23 identical citations from 3 of the citing papers, while another received 18 identical citations from all 5 citing papers. This suggests that the company may be processing purchased citations in bulk, planting citations purchased by multiple people in the same set of papers. Furthermore, Supplementary Fig. 5D depicts the proportion of references in the bibliography of these five citing papers that do not appear in their main text. As can be seen, none of the entries in the reference lists of two of the five papers appeared in their main text. The remaining papers also fail to include a majority of the citations listed in their bibliographies in their main text, with 90%, 73%, and 69% of the citations not being included, respectively.
Considering that 4 of the 5 papers which planted references to our fictional author stemmed from the same journal, and that planted citations to other authors were also found in these papers, it is natural to wonder whether other papers in this journal could also contain planted citations. To this end, we collect all papers published in this journal so far in this year, and identify the references that appear in more than one paper. We then construct a network where the nodes represent the papers, and an edge connects a pair of papers if they share at least one reference. Additionally, the weight of an edge denotes the number of shared references between a pair of papers. Assuming that planted citations appear in bulk in more than one paper, this would result in clusters of papers with many shared references. While it is natural to see pairs of papers with several shared references, particularly if they are on the same topic, focusing on the set of papers sharing an extremely high number of common references would potentially reveal the citations purchased in bulk.
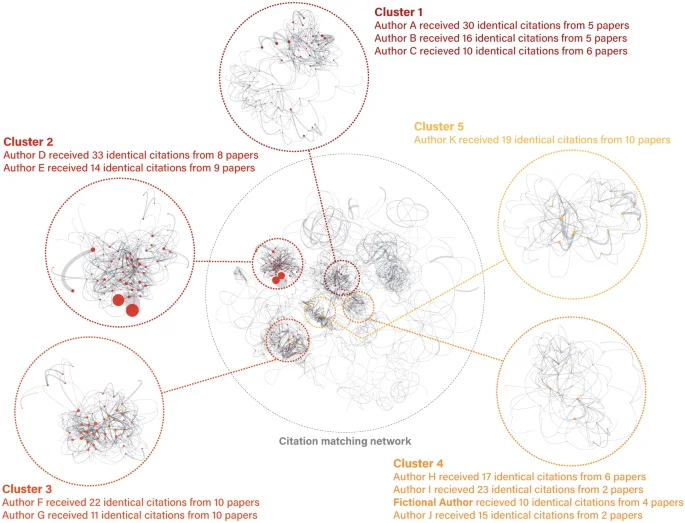
The citation matching network of the journal that provided purchased citations. Nodes denote papers published in this journal, and edges link two papers which share at least one reference in their bibliographies. The edge width reflects the number of shared references that appear in both papers. The largest circle contains the 30 largest connected components in the network. The five smaller circles zoom into the clusters containing papers that include a high number of citations to certain authors. Details regarding these authors are listed next to each circle. The figure was generated using Python 3.10.7 using the networkx and matplotlib libraries.
As can be seen in Fig. 4, several connected components emerge due to papers which share multiple references. In particular, we highlight five clusters containing papers that include a unusually high number of citations to a given author. The papers which provided citations to the fictional author created in the previous section are found in Cluster #4, but the fictional author is far from the most extreme case in the network. For instance, in Cluster #1, Author A received citations to the same set of 30 papers which he authored from 5 different papers in that cluster. Similarly, in Cluster #2, Author D received citations to the same set of 33 of their papers from 8 different papers published in the journal. These results suggest that purchased citations within this journal are likely not limited to only our own purchase of 50 citations.
Discussion
In this study, we highlight several manners in which one can manipulate and inflate their citation counts on Google Scholar, the first of which is that of citation purchasing. Purchasing citations is fundamentally different from previously studied forms of citation manipulation, such as self-citations14,15 and citation cartels16,17,18,19. In particular, these forms are defined by, and are hence detectable from, the topology of the citation network. In contrast, whether or not a citation has been purchased is exogenous to the citation network, making it impossible to determine with absolute certainty that a given citation is purchased through merely studying the network’s topology.
Our findings highlight problems with Google Scholar in particular and academic databases in general. They also question the practice of considering citation count when evaluating candidates for recruitment and promotion purposes. Starting with Google Scholar, we highlight two issues. Firstly, while it specifies the citations received by a given paper, it does not specify, in an easily accessible manner, the citations emanating from a given paper. Specifically, knowledge of the number of citations an author receives alone is not sufficient to identify misconduct, therefore, the manner in which Google Scholar displays citation information makes it extremely challenging to detect the forms of citation manipulation unraveled in our study. This makes it extremely challenging to detect the forms of citation manipulation unraveled in our study. For example, starting from the Google Scholar profile of the scientist who is cited 167 times in a single paper p, one cannot discover this fact without collecting and analyzing all the citations received by that scientist from their 167 papers cited in p. Consequently, those who engage in such practices can easily go unnoticed by the casual observer browsing Google Scholar profiles. The second issue with Google Scholar is its lack of moderation when indexing research articles, despite repeated criticism52,53. These issues are especially of concern given that when it comes to evaluating candidates for hiring or promotion at highly ranked universities, Google Scholar is more frequently used than all other databases combined, as our survey has demonstrated. Therefore, we challenge previous work which suggests that Google Scholar would not become “a main source of citation analyses especially for research evaluation purposes”52.
There are reasons to believe that citation manipulation practices are less prevalent in Scopus and the Web of Science due to their more restrictive indexing of journals and papers. Although both of these databases have recently included papers from some selective preprint servers in their database, such papers do not contribute to their calculation of rankings and metrics, including citation count54,55. This exclusion safeguards them from the impact of planted citations stemming from pre-prints. Take the five scientists in Fig. 2B, for example. Although they were able to boost their citation counts on Google Scholar, their citation numbers on Scopus are substantially lower. Nonetheless, this does not rule out the possibility that citation manipulation affects other databases. Ultimately, authors are free to choose which references to add in their paper, making it impossible to eliminate disingenuously planted citations. Therefore, the issues with citation metrics we highlight in this paper are not confined to a particular database, but rather concern academia as a whole. Below, we first discuss two factors that may further exacerbate the issue of citation manipulation: the overpublication of special issues and the emergence of generative AI technologies. Finally, we suggest potential policy and technical recommendations aimed at mitigating citation manipulation.
The citation purchasing service that we highlight in this study is closely related to other forms of academic misconduct, such as predatory publishing and paper mills. For example, four out of five papers that supplied the purchased citations were all published in the same “Special Issue” of a journal which primarily publishes work related to Chemistry. However, the papers authored by the fictional author were on the topic of “fake news”, and were cited by papers discussing political issues surrounding fake news. Additionally, the same special issue published papers related to a wide array of topics including family policy, Islamic psychology, and education, which are entirely unrelated to Chemistry. Indeed, publishing a huge amount of “special issues” that undergoes minimal, if any, peer-review, is a well-known strategy of predatory publishers to generate profit56. Furthermore, these four papers appeared consecutively within the special issue, suggesting that this form of misconduct may not be isolated to the individual authors that wrote these papers, but may be related to unethical publishing practice at the journal level. The emergence of profit-oriented predatory publishers with limited peer review processes also enabled the proliferation of paper mills which mass-produce and sell low-quality or plagiarized papers. While paper mills constitute a relatively well-known form of what is called “industrialized cheating”57, we contribute to scientists’ understanding of such practices by documenting the existence of “citation mills”. Taken together, unethical publishing practices such as predatory publishing, paper mills, and citation mills challenge the reliability of scientometric indicators.
Furthermore, the problem of citation manipulation may exacerbate in the age of generative Artificial Intelligence (AI). Using this technology, a scientist can conceivably generate a “scientific” paper, and then plant citations as they see fit in its bibliography, e.g., to themselves or to another scientist in return for a fee. As has been shown, current generative AI tools are already capable of producing abstracts that can fool scientists58, suggesting that such tools could possibly generate fake, yet seemingly realistic, papers in the future. In our experiment, for example, the papers that were generated using AI lack any scientific contribution, yet they were uploaded to major pre-print servers with little to no push-back. Those who intend to game the system could also utilize AI to paraphrase existing papers, relying on their structure and contributions to produce a convincing manuscript. Indeed, several plagiarism cases have been recently exposed59,60,61,62.
To remedy the issue of citation manipulation, actions should be taken by both bibliographic databases and those engaging in scientist evaluation. Bibliographic databases can report additional metrics designed specifically to track how citations are accumulated by any given scientist. Past research has advocated for reporting scientists’ self-citation indices, e.g., the s-index63, in order to quantify the extent to which a scientist self-cites. In this study, we proposed the citation concentration index, or the (c^2)-index, as well as the adjusted (c^2)-index, to quantify the degree to which a scientist receives a large number of citations from a disproportionately small number of sources. As we have shown, a scientist in our dataset received a (c^2)-index of 70, indicating that there exist 70 different papers which cite that scientist at least 70 times each. It is imperative to note, however, that such indices should not serve as a definitive measure of citation manipulation or malpractice. Rather, such measures should only serve as a “warning sign” used to highlight highly anomalous citation accumulation patterns. Indeed, as we have shown, many scientists could accumulate a high (c^2)-index without engaging in citation manipulation. Nonetheless, publishing such indices may act as a deterrent from inflating one’s citations. Furthermore, given that citations play a role in a university’s position in all major university ranking outlets64,65,66, the motivation behind engaging in citation and publication manipulation practices may stem from a departmental or university level push towards increased faculty productivity, as has been documented in a number of recent cases67,68. Bibliographic databases could also publish information regarding the sources of citations made to a given author or publication. For instance, one possibility is to allow users to filter for the quality of the venue from which citations are received, e.g., whether it is peer-reviewed, has an impact factor greater than a given threshold, or is published in a Q1 journal, etc. Access to such information may allow for a more holistic view on a scientist’s citation profile during the research evaluation process, beyond merely an assessment of citation counts or metrics such as the h-index. Future work may examine other such indices which capture anomalous trends in citation acquisition and paper authorship, and test their effectiveness in the field. For instance, one such metric could examine the proportion of citations an author receives which includes a valid Digital Object Identifier, or DOI, which may capture citations stemming from non academic work.
More broadly, our findings highlight the need for evaluation committees to be aware of the different ways in which citations can be distorted. While citation metrics can serve as a practical proxy of scientific success, current bibliometric databases such as Google Scholar, Scopus, and Web of Science only offer a superficial perspective into the structure of a scientist’s citation and publication history. Such a perspective would miss long-lasting disparities in how submissions, publications, and careers are evaluated. For instance, scientists who reside in central positions in science due to their affiliation69,70, gender30, and past achievement71 tend to fortify their status due to their incumbent advantage. Moreover, studies have shown that papers receive more favorable peer-reviews when associated with a well-known author71. After being published, those papers again accumulate citations faster than those authored by less prominent scientists72. Furthermore, studies have shown the adverse effect of low citation count of a particular article on its perceived quality, and in turn, the level of scrutiny in which it is evaluated73. Given that citations to a paper can be manipulated through a variety of means, without an awareness of the sources of such citations, evaluators may unconsciously overestimate the quality of articles they evaluate. Taken as a whole, our findings bring to light new forms of citation manipulation, and emphasize the need to look beyond citation counts.
Methods
Survey
We reached out to over 30,000 members of the top 10 ranked universities around the world through email between May 12 and May 23, 2023 to gauge the extent to which academics consider citations when evaluating other scientists for hiring and promotion purposes. These universities are: Yale University, Stanford University, University of Oxford, University of Cambridge, University of California Berkeley, Massachusetts Institute of Technology, Imperial College London, Harvard University, Princeton University, and California Institute of Technology. We collected email addresses from the list of all faculties found on the website of mentioned universities. At the time of receiving our emails, the primary affiliation of 38 respondents are with another university not in the list; see Supplementary Table 1 for the number of respondents from each affiliation.
Respondents were asked to identify with one of 20 disciplines (in addition to “Other”) which are then classified into four categories: Natural Sciences, Social Sciences, Computational Sciences, and Humanities. Natural Sciences include: Biology, Chemistry, Medicine, Geology, Physics, Environmental Science, and Materials Science. Social Sciences include: Geography, Psychology, Political Science, Sociology, Economics, and Business. Computational Sciences include: Mathematics, Computer science, and Engineering. Humanities include: Art, History, Philosophy, and Anthropology/Archaeology. The number of respondents from each discipline is shown in Supplementary Table 2.
Out of the 30,000 email addresses that we contacted, we received 574 responses in total. Respondents participate in the survey to answer questions regarding how they and their colleagues evaluate other scientists; see supplementary text for the survey questions. Throughout the survey, we do not provide any information related to the current study that might influence the respondents’ opinion in any way. Furthermore, the participants are presented choice options in randomized orders so that their choices are not influenced by the arbitrariness of the ordering.
Anomalous citation analysis
First, we collected Google Scholar profiles of over 1.6 million unique authors on the platform. To do so, we performed a breadth-first search to a depth of 10, starting from the profiles of the two corresponding authors of this study. At each step, all authors listed in the co-authors section of their profile are collected. For each author, we collected details including their name, affiliation, research interests, number of citations in each year, and a list of their publications. Details on the number of authors at each collection depth can be seen in Supplementary Table 3. Altogether, these scientists come from 153 countries around the world (Supplementary Fig. 6), and span 8 major disciplines of scientific research including Artificial Intelligence, Biology, Ecology, Economics, Human-Computer Interaction, Mathematics, Medicine, and Physics (Supplementary Fig. 7).
Next, we narrow the list of authors to investigate by applying a filter to capture those with significant spikes in their citation history. To this end, we filter those authors which meet the following criteria; They have at least (1) 200 citations, to disregard those with low citation counts; (2) 10 publications, to disregard early career researchers; (3) a year in which they experienced a 10x increase in citations year over year, where this jump accounted for at least 25% of their total citations, to capture those with significant citation spikes. This filter resulted in a set of 1016 unique authors. For each of the resulting authors, we collect all of the citations they have received over the duration of their academic career. Importantly, all of the information collected for the 1.6 million Google Scholar profiles are publicly and freely available on the website. We used a commercial API, namely SERP API, to collect citation data. Furthermore, the crawling and parsing of public data is protected by the First Amendment of the United States Constitution74.
To identify the outliers, for any given paper c, we count the number of unique papers p written by an author a and cited by c, which we will denote as n, or the number of citations received by an author from c. Of the 1016 authors, the 95th percentile of n falls at 3, indicating that in the vast majority of cases, a given paper will only cite a given author not more than a few times. To qualify as anomalous, we manually investigate the authors who have received citations from papers which exhibit an (n ge 18), which is the 99.9th percentile in our dataset, resulting in a set of 114 authors. This threshold is in line with results seen from previous work on citation trends, which similarly find that 17 citations to a given author from a single paper was the 99.975th percentile75. It is nonetheless important to note that being classified anomalous by this metric does not necessarily imply wrongdoing. Specifically, any accusation of manipulation or wrongdoing should only come from further investigation.
Next, we select five of the worst offenders with regards to the percentage of their total citations stemming from papers c that cite them more than 18 times, where paper c was not authored by the offender. Here, we purposefully distinguish between self citations and non-self citations. While self citations have been a subject of research in the past (14, 15), non-self citations, possibly obtained through illicit means, have not been studied. To better highlight differences in the nature of the citations received by the five offenders, we find the authors which matches them most closely based on a number of factors, including: (1) Academic birth year, i.e., the year when their first paper was published; (2) their total number of publications; (3) their total number of citations; and (4) they share at least one keyword. For each of the five offenders and their respective matches, we collect the 10 papers c with the largest n value, which we call their “citing papers”.
Purchasing citations
After we contacted the email address listed on the website offering the so-called “H-index & Citations Booster” service (Supplementary Fig. 5A), we were put in touch with the vendor via WhatsApp. According to the vendor, citations are sold in bulk for either 300 USD per 50 citations or 500 USD per 100 citations; see Supplementary Fig. 5B for a screenshot of the conversation with the vendor where they list the “packages” on offer. According to the vendor, all purchased citations would emanate from papers published in one or more of 22 peer-reviewed journals listed on their website, 14 of which are Scopus-indexed. Some of these journals are published by publishers as recognized as Springer and Elsevier, and have impact factors as high as 4.79.
Adopting the persona of the fictional author created in the previous section, we purchased the 50-citations package for the price of 300 USD. Next, we provided 10 of the 20 papers authored by the fictional profile. Once the payment was complete, the company quoted a maximum duration of 45 days to provide the citations. In our conversations with the company through this process, they declined to elaborate on the exact journals from which the citations would be emanating from, as well as the number of papers that would be providing the citations.
Eventually, we identified five papers that supply the purchased citations. Four of these citing papers were published and indexed on Google Scholar only 33 days after the purchase was made, while the last citing paper was published after 40 days.
Identifying clusters of papers based on bibliographic coupling
After identifying the journal from which our purchased citations originate, we hypothesize that this journal likely contains more citations that were sold in bulk. To understand whether this is the case, we first collected all papers published therein in the first half of 2023. Then, we construct a network where each node in the network represents a paper, and two papers are connected with an edge if they share at least one identical reference. To account for typos and formatting errors, we considered two reference items as identical if their Levenshtein distance similarity ratio exceeded 0.98. As a result, several connected components emerged in this network of papers. In each connected component, we scan reference lists for authors appearing at least 10 times across multiple papers. These authors were identified as potential candidates for having purchased citations. Then, we manually confirm whether the set of referenced papers was consistent among all citing papers. Such consistency is highly improbable by random chance but likely when citations are acquired in bulk. Our investigation unveiled a total of 11 anomalous authors distributed across five distinct connected components of papers. These authors received citations of at least 10 times per paper from multiple sources.
Data availability
The datasets generated and/or analysed during the current study are not publicly available to avoid publicly shaming the authors, editors, and journals whose anomalous behaviour is documented in our study. However, aggregated and anonymized data to reproduce the figures are available from the corresponding author on reasonable request.
References
-
Wang, D. & Barabási, A.-L. The Science of Science (Cambridge University Press, 2021).
Google Scholar
-
Acuna, D. E., Allesina, S. & Kording, K. P. Predicting scientific success. Nature 489(7415), 201 (2012).
Google Scholar
-
Sinatra, R., Wang, D., Deville, P., Song, C. & Barabási, A.-L. Quantifying the evolution of individual scientific impact. Science 354(6312), aaf5239 (2016).
Google Scholar
-
Crew, B. Google Scholar reveals its most influential papers for 2019. Nature Index (2019).
-
Stephan, P., Veugelers, R. & Wang, J. Reviewers are blinkered by bibliometrics. Nature 544(7651), 411 (2017).
Google Scholar
-
Garfield, E. Citation analysis as a tool in journal evaluation: Journals can be ranked by frequency and impact of citations for science policy studies. Science 178(4060), 471 (1972).
Google Scholar
-
American Society for Cell Biology and others, San Francisco declaration on research assessment (DORA) (2012). Accessed June 7, 2023.
-
Hicks, D., Wouters, P., Waltman, L., De Rijcke, S. & Rafols, I. Bibliometrics: the Leiden Manifesto for research metrics. Nature 520(7548), 429 (2015).
Google Scholar
-
Sugimoto, C. R. Scientific success by numbers. Nature 593(7857), 30 (2021).
Google Scholar
-
Maddox, J. Competition and the death of science. Nature 363, 667 (1993).
Google Scholar
-
Železnỳ, J. Why competition is bad for science. Nat. Phys. 19(3), 300 (2023).
Google Scholar
-
Fire, M. & Guestrin, C. Over-optimization of academic publishing metrics: observing Goodhart’s Law in action. GigaScience 8(6), giz053 (2019).
Google Scholar
-
Franck, G. Scientific communication—a vanity fair?. Science 286(5437), 53 (1999).
Google Scholar
-
Hyland, K. Self-citation and self-reference: Credibility and promotion in academic publication. J. Am. Soc. Inf. Sci. Technol. 54(3), 251 (2003).
Google Scholar
-
Ioannidis, J. P. A generalized view of self-citation: Direct, co-author, collaborative, and coercive induced self-citation. J. Psychosom. Res. 78(1), 7 (2015).
Google Scholar
-
Fister, I. Jr., Fister, I. & Perc, M. Toward the discovery of citation cartels in citation networks. Front. Phys. 4, 49 (2016).
Google Scholar
-
Perez, O., Bar-Ilan, J., Cohen, R. & Schreiber, N. The network of law reviews: Citation cartels, scientific communities, and journal rankings. Mod. Law Rev. 82(2), 240 (2019).
Google Scholar
-
Kojaku, S., Livan, G. & Masuda, N. Detecting anomalous citation groups in journal networks. Sci. Rep. 11(1), 1 (2021).
Google Scholar
-
Oransky, I. & Marcus, A. Gaming the system, scientific ‘cartels’ band together to cite each others’ work. Stat News 13 (2017).
-
Wilhite, A. W. & Fong, E. A. Coercive citation in academic publishing. Science 335(6068), 542 (2012).
Google Scholar
-
Herteliu, C., Ausloos, M., Ileanu, B. V., Rotundo, G. & Andrei, T. Quantitative and qualitative analysis of editor behavior through potentially coercive citations. Publications 5(2), 15 (2017).
Google Scholar
-
Thombs, B. D. et al. Potentially coercive self-citation by peer reviewers: a cross-sectional study. J. Psychosom. Res. 78(1), 1 (2015).
Google Scholar
-
Martin, B. R. Whither research integrity? Plagiarism, self-plagiarism and coercive citation in an age of research assessment,. Res. Policy 42(5), 1005–1014 (2013).
Google Scholar
-
Marcus, A. Publisher offers cash for citations, Retraction Watch August 2021. Accessed June 7 (2023).
-
Ertz, M. New scam: Do you want to be paid to cite others’ research?, ResearchGate November 2022. Accessed June 7, 2023.
-
Wang, D., Song, C. & Barabási, A.-L. Quantifying long-term scientific impact. Science 342(6154), 127 (2013).
Google Scholar
-
Sekara, V. et al. The chaperone effect in scientific publishing. Proc. Natl. Acad. Sci. 115(50), 12603 (2018).
Google Scholar
-
Nielsen, M. W., Andersen, J. P., Schiebinger, L. & Schneider, J. W. One and a half million medical papers reveal a link between author gender and attention to gender and sex analysis. Nat. Hum. Behav. 1(11), 791 (2017).
Google Scholar
-
Wuchty, S., Jones, B. F. & Uzzi, B. The increasing dominance of teams in production of knowledge. Science 316(5827), 1036 (2007).
Google Scholar
-
Huang, J., Gates, A. J., Sinatra, R. & Barabási, A.-L. Historical comparison of gender inequality in scientific careers across countries and disciplines. Proc. Natl. Acad. Sci. 117(9), 4609 (2020).
Google Scholar
-
Wu, L., Wang, D. & Evans, J. A. Large teams develop and small teams disrupt science and technology. Nature 566(7744), 378 (2019).
Google Scholar
-
Fleming, L., Greene, H., Li, G., Marx, M. & Yao, D. Government-funded research increasingly fuels innovation. Science 364(6446), 1139 (2019).
Google Scholar
-
Ahmadpoor, M. & Jones, B. F. The dual frontier: Patented inventions and prior scientific advance. Science 357(6351), 583 (2017).
Google Scholar
-
Li, W., Zhang, S., Zheng, Z., Cranmer, S. J. & Clauset, A. Untangling the network effects of productivity and prominence among scientists. Nat. Commun. 13(1), 4907 (2022).
Google Scholar
-
AlShebli, B. et al. Beijing’s central role in global artificial intelligence research. Sci. Rep. 12(1), 21461 (2022).
Google Scholar
-
AlShebli, B. K., Rahwan, T. & Woon, W. L. The preeminence of ethnic diversity in scientific collaboration. Nat. Commun. 9(1), 5163 (2018).
Google Scholar
-
Liu, F., Holme, P., Chiesa, M., AlShebli, B. & Rahwan, T. Gender inequality and self-publication are common among academic editors. Nat. Hum. Behav., 1 (2023).
-
Yin, Y., Wang, Y., Evans, J. A. & Wang, D. Quantifying the dynamics of failure across science, startups and security. Nature 575(7781), 190 (2019).
Google Scholar
-
Liu, F., Rahwan, T. & AlShebli, B. Non-White scientists appear on fewer editorial boards, spend more time under review, and receive fewer citations. Proc. Natl. Acad. Sci. 120(13), e2215324120 (2023).
Google Scholar
-
Petersen, A. M. Quantifying the impact of weak, strong, and super ties in scientific careers. Proc. Natl. Acad. Sci. 112(34), E4671 (2015).
Google Scholar
-
Jia, T., Wang, D. & Szymanski, B. K. Quantifying patterns of research-interest evolution. Nat. Hum. Behav. 1(4), 0078 (2017).
Google Scholar
-
Azoulay, P., Graff Zivin, J. S. & Wang, J. Superstar extinction. Q. J. Econ. 125(2), 549 (2010).
Google Scholar
-
Elsevier, Scopus Content (2023). Accessed June 12, 2023.
-
Van Noorden, R. Highly cited researcher banned from journal board for citation abuse.. Nature 578(7794), 200 (2020).
Google Scholar
-
Van Noorden, R. & Chawla, D. S. Hundreds of extreme self-citing scientists revealed in new database. Nature 572(7771), 578 (2019).
Google Scholar
-
Oransky, I. Exclusive: Elsevier retracting 500 papers for shoddy peer review. https://retractionwatch.com/2022/10/28/exclusive-elsevier-retracting-500-papers-for-shoddy-peer-review/ (Retraction Watch) (2022). Accessed November 10, 2023.
-
Cornell University, arXiv .org e-Print archive Access July 25, 2023.
-
Authorea: Open Research Collaboration and Publishing Access July 25, 2023.
-
OSF Access July 25, 2023.
-
ResearchGate | Find and share research Access July 25, 2023.
-
ChatGPT OpenAI, Access July 25, 2023.
-
Halevi, G., Moed, H. & Bar-Ilan, J. Suitability of Google Scholar as a source of scientific information and as a source of data for scientific evaluation–Review of the literature. J. Inform. 11(3), 823 (2017).
Google Scholar
-
Delgado López-Cózar, E., Robinson-García, N. & Torres-Salinas, D. The Google Scholar experiment: How to index false papers and manipulate bibliometric indicators. J. Assoc. Inf. Sci. Technol. 65(3), 446 (2014).
Google Scholar
-
McCullough, R. Preprints are now in Scopus! https://blog.scopus.com/posts/preprints-are-now-in-scopus (2021). Accessed September 10, 2024.
-
Scheer, R. Clarivate Adds Preprint Citation Index to the Web of Science. https://clarivate.com/news/clarivate-adds-preprint-citation-index-to-the-web-of-science/ (2023). Accessed September 10, 2024.
-
Brainard, J. Fast-growing open-access journals stripped of coveted impact factors. https://www.science.org/content/article/fast-growing-open-access-journals-stripped-coveted-impact-factors (2023). Accessed October 09, 2023.
-
Else, H. & Van Noorden, R. The fight against fake-paper factories that churn out sham science. Nature 591(7851), 516 (2021).
Google Scholar
-
Else, H. Abstracts written by ChatGPT fool scientists. Nature 613(7944), 423 (2023).
Google Scholar
-
Alharbi, A., Alyami, H., Poongodi, M., Rauf, H. T. & Kadry, S. Intelligent scaling for 6G IoE services for resource provisioning. PeerJ Comput. Sci. 7, e755 (2021).
Google Scholar
-
Sami, H., Otrok, H., Bentahar, J. & Mourad, A. AI-based resource provisioning of IoE services in 6G: A deep reinforcement learning approach. IEEE Trans. Netw. Serv. Manag. 18(3), 3527 (2021).
Google Scholar
-
Shah, H. & Sahoo, J. Using a multifaceted, network-informed methodology to assess data sensitivity from consumers IoT gadgets. In 2023 International Conference on Artificial Intelligence and Smart Communication (AISC) 1303–1309 (IEEE, 2023).
-
Ren, J. et al. Information exposure from consumer IoT devices: A multidimensional, network-informed measurement approach. In Proceedings of the Internet Measurement Conference 267–279 (2019).
-
Kacem, A., Flatt, J. W. & Mayr, P. Tracking self-citations in academic publishing. Scientometrics 123(2), 1157 (2020).
Google Scholar
-
Ranking, S. ShanghaiRanking’s Academic Ranking of World Universities Methodology (2022)
-
C.L.L. QS World University Rankings Methodology: Using Rankings to Start Your University Search. Top Universities May 2023.
-
T. H. Education. Methodology for overall and subject rankings for the times higher education world university rankings (2023)
-
Chawla, D. S. Highly-cited chemist is suspended for claiming to be affiliated with Russian and Saudi universities (2023).
-
Joelving, F. Did a ‘nasty’ publishing scheme help an Indian dental school win high rankings?. Science (2023). Accessed October 9, 2023.
-
Okike, K., Hug, K. T., Kocher, M. S. & Leopold, S. S. Single-blind vs double-blind peer review in the setting of author prestige. JAMA 316(12), 1315 (2016).
Google Scholar
-
Nielsen, M. W., Baker, C. F., Brady, E., Petersen, M. B. & Andersen, J. P. Weak evidence of country-and institution-related status bias in the peer review of abstracts. Elife 10, e64561 (2021).
Google Scholar
-
Huber, J. et al. Nobel and novice: Author prominence affects peer review. Proc. Natl. Acad. Sci. 119(41), e2205779119 (2022).
Google Scholar
-
Petersen, A. M. et al. Reputation and impact in academic careers. Proc. Natl. Acad. Sci. 111(43), 15316 (2014).
Google Scholar
-
Teplitskiy, M., Duede, E., Menietti, M. & Lakhani, K. R. How status of research papers affects the way they are read and cited. Res. Policy 51(4), 104484 (2022).
Google Scholar
-
Haroon, M. et al. Auditing YouTube’s recommendation system for ideologically congenial, extreme, and problematic recommendations. Proc. Natl. Acad. Sci. 120(50), e2213020120 (2023).
Google Scholar
-
Wren, J. D. & Georgescu, C. Detecting anomalous referencing patterns in PubMed papers suggestive of author-centric reference list manipulation. Scientometrics 127(10), 5753 (2022).
Google Scholar
Acknowledgements
The support and resources from the High Performance Computing Center at New York University Abu Dhabi are gratefully acknowledged. ChatGPT was used during the writing process to improve readability.
Funding
H.I. and F.L. are supported by the New York University Abu Dhabi Global Ph.D. Student Fellowship.
Author information
Authors and Affiliations
Contributions
T.R. and Y.Z. conceived the study and designed the research; H.I. collected the data; H.I. and F.L analyzed the data; H.I., F.L., T.R., and Y.Z. wrote the manuscript.
Corresponding authors
Ethics declarations
Competing interests
The authors declare no competing interests.
Ethical approval
The research was approved by the Institutional Review Board of New York University Abu Dhabi (HRPP-2023-5). All research was performed in accordance with relevant guidelines and regulations. Informed consent was received from all survey participants. Following the completion of the study, the pre-prints created for the fictional Google Scholar profile were removed from their respective servers. In addition, the fictional Google Scholar profile was deleted from the platform. As per the IRB guidelines, we did not disclose the identity of any of the papers or authors highlighted in this study to any party other than the editors and reviewers of this manuscript.
Additional information
Publisher’s note
Springer Nature remains neutral with regard to jurisdictional claims in published maps and institutional affiliations.
Supplementary Information
Supplementary Information.
Rights and permissions
Open Access This article is licensed under a Creative Commons Attribution-NonCommercial-NoDerivatives 4.0 International License, which permits any non-commercial use, sharing, distribution and reproduction in any medium or format, as long as you give appropriate credit to the original author(s) and the source, provide a link to the Creative Commons licence, and indicate if you modified the licensed material. You do not have permission under this licence to share adapted material derived from this article or parts of it. The images or other third party material in this article are included in the article’s Creative Commons licence, unless indicated otherwise in a credit line to the material. If material is not included in the article’s Creative Commons licence and your intended use is not permitted by statutory regulation or exceeds the permitted use, you will need to obtain permission directly from the copyright holder. To view a copy of this licence, visit http://creativecommons.org/licenses/by-nc-nd/4.0/.
Reprints and permissions
About this article
Cite this article
Ibrahim, H., Liu, F., Zaki, Y. et al. Citation manipulation through citation mills and pre-print servers.
Sci Rep 15, 5480 (2025). https://doi.org/10.1038/s41598-025-88709-7
-
Received: 17 April 2024
-
Accepted: 30 January 2025
-
Published: 14 February 2025
-
DOI: https://doi.org/10.1038/s41598-025-88709-7