Abstract
Heritage Science has a lot to gain from the Open Science movement but faces major challenges due to the interdisciplinary nature of the field, as a vast array of technological and scientific methods can be applied to any imaginable material. Historical and cultural contexts are as significant as the methods and material properties, which is something the scientific templates for research data management rarely take into account. While the FAIR data principles are a good foundation, they do not offer enough practical help to researchers facing increasing demands from funders and collaborators. In order to identify the issues and needs that arise “on the ground floor”, the staff at the Heritage Laboratory at the Swedish National Heritage Board took part in a series of workshops with case studies. The results were used to develop guides for good data practices and a list of recommended online vocabularies for standardised descriptions, necessary for findable and interoperable data. However, the project also identified areas where there is a lack of useful vocabularies and the consequences this could have for discoverability of heritage studies on materials from areas of the world that have historically been marginalised by Western culture. If Heritage Science as a global field of study is to reach its full potential this must be addressed.
Introduction
The Open Science movement has gone from informal recommendations to mandatory requirements in many countries and among the major public funders for research (e.g. EU Directive 2019/1024). It has expanded beyond calls for Open Access publications to demands for Open Data preservation and accessibility. The FAIR data principles (Findable, Accessible, Interoperable, Reusable) have become the standards to which researchers, scientists and specialists must rise. The system in which today’s researchers are expected to function is digital and global, meaning laboratory practices must develop as well.
The scientific method is about testing and building upon previous results. For centuries, this has been a time-consuming process, but in the digital age, new tools are at our disposal, at the same time as the production of research results is increasing at an exponential rate. Digital infrastructures are therefore being developed to aggregate scientific data on an international scale (e.g. EOSC, GBIF), and conceptual reference models are used by computers to enable the multidisciplinary and multilingual data in these to become searchable. CIDOC CRM is one such reference model, developed by ICOM specifically for data from cultural heritage and associated fields (archaeology, ancient texts, buildings) and it is often used for infrastructures and applications geared towards these communities1,2,3,4: e.g. SSHOC, Ariadne RI, INFN CHNet THESPIAN and Arches for Science. Only some attempts in implementing such infrastructures have been successful enough to last beyond the initial project phase, as seen in the examples presented in the two special issues from 2017 on Digital Infrastructure for Cultural Heritage in the Journal on Computing and Cultural Heritage5,6. Still, Heritage Science as a global multidisciplinary field is a vital part of the Open Science movement. In 2022 the EU Commission decided to grant substantial long-term financing via the Horizon Europe programme for the development of a European Collaborative Cloud for Cultural Heritage (ECCCH)7. In short, regardless of what individual researchers may feel comfortable with, the future of financing is already tied to the ability to produce open and FAIR research data.
Unfortunately, researchers and specialists producing said data, especially those working in institutions not associated with a university, have not received the same support as infrastructure developers. Furthermore, they rarely have the support or technical know-how to install applications and adapt the open-source code to their requirements. The FAIR principles and recommendations are mostly too general to be directly applicable to specific research practices. There is a growing realisation that successful implementation requires adjustments to the very process of doing research, as well as guides grounded in communities of practice8,9. Interdisciplinary subjects like Heritage Science, which applies scientific methodologies to culture-historical objects, are doubly challenged to make the data findable and accessible to a wide variety of potential users.
The authors undertook a research-and-development project in order to examine the challenges faced specifically by Heritage Science practitioners in implementing FAIR data principles and to produce more useful instructions based on actual practices. The project was primarily aimed at helping individual specialists and smaller laboratories without the benefit of access to larger institutional infrastructures for data management. The Heritage Laboratory at the Swedish National Heritage Board was used for a case study (see below).
We will show that the FAIR principles are not so much about technical proficiency and far more about good practices, which is why successful implementation of Open Science requires more than just infrastructure and software development. We will also highlight how implementing good practices can generate benefits beyond making research data accessible, by bridging the divide between collection management and academic research. However, our investigation also revealed major challenges that require more than the effort of individuals to overcome—such as the lack of user-friendly controlled vocabularies that reflect the breadth and depth of human histories and cultures. If not dealt with, we risk recreating historical structural inequalities in our digital present.
Heritage Laboratory of the Swedish National Heritage Board—national and international context
The Heritage Laboratory of the Swedish National Heritage Board is a central provider for heritage science, including conservation science, archaeometry and art historical research in Sweden. It is a partner in the Heritage Science Sweden (HSS) network that was established in 2017.
The Heritage Laboratory regularly hosts external guest researchers who, together with the staff scientists, employ analytical and experimental methods to study materials and their properties. These analyses span from minute samples of objects or individual materials to comprehensive investigations of collections and sites. Projects are usually run in collaboration with stakeholders from both the private and public sectors, including museums, universities, libraries, governmental or municipal bodies and other custodians of cultural heritage. The Swedish National Heritage Board is a publicly funded institution, and as such the data generated in its Heritage Laboratory is in the public domain. Since 2023, aiming for FAIR data, the laboratory started using the EU-funded repository Zenodo10.
Through the EU project IPERION HS, Integrating Platforms for the European Research Infrastructure on Heritage Science, the Heritage Laboratory’s resources, as well as three Swedish universities’ heritage science resources, were partially made available internationally. IPERION HS was a consortium of organisations from 23 countries. It provided training and access to a multitude of scientific knowledge, analytical instruments and associated data for Heritage Science, much of which will continue to be available through the forthcoming European Research Infrastructure for Heritage Science, E-RIHS.
Open Science and the FAIR data principles
In the contemporary scientific landscape, Open Science has emerged as a transformative paradigm embodying principles that challenge conventional modes of research conduct. While there are several schools of thought, in general, the movement is characterised by a departure from the historical proprietary nature of scientific endeavours, advocating for a collaborative, transparent and inclusive framework for knowledge creation and dissemination11. Open Science encapsulates a set of practices aimed at dismantling traditional barriers to access and promoting a culture of shared data, methodologies and findings. In this exposition, we delve into the inherent virtues of Open Science and elucidate the operational dynamics underpinning its manifestation in the scientific domain.
The European Commission issued recommendations on conservation and access to scientific information in 2012, and these are under implementation in the EU, affecting national funding requirements as well12,13. The European Union is actively working for and promoting open research data through its research and innovation programme Horizon Europe. The programme calls for European countries to “embrace open science as the modus operandi for all researchers”14. Better interoperability and sharing of FAIR data will be the focus of several Cluster, Partnerships and Missions. In order to facilitate this transition, the programme helps finance the European Open Science Cloud (EOSC), which will connect research data from various repositories and infrastructures, and the Marie Skłodowska-Curie Actions, which promote the diffusion of open science practices and support the development of these skills among researchers.
The fact that some research data may be subject to sharing restrictions does not mean that they do not have to comply with the retention and availability requirements. It should be possible to make them available under the right conditions, for example after an application and legal review. A good way to ensure that research data is shared in a way that complies with the above guidelines is to use the FAIR principles. The FAIR principles have become the most important templates and guidelines for good data management by government agencies and public funders15,16,17. The principles originated in a workshop held in Leiden in the Netherlands in 2014, and during and after the meeting, what would become “FAIR Guiding Principles for Scientific Data Management and stewardship” was eventually formulated18. Originally, the principles were developed as a rather technical requirement for data repositories and platforms, but many of them also serve as support for individual practices. A summary of the FAIR principles, adjusted from the point of view of a data producer, is shown in Fig. 1.
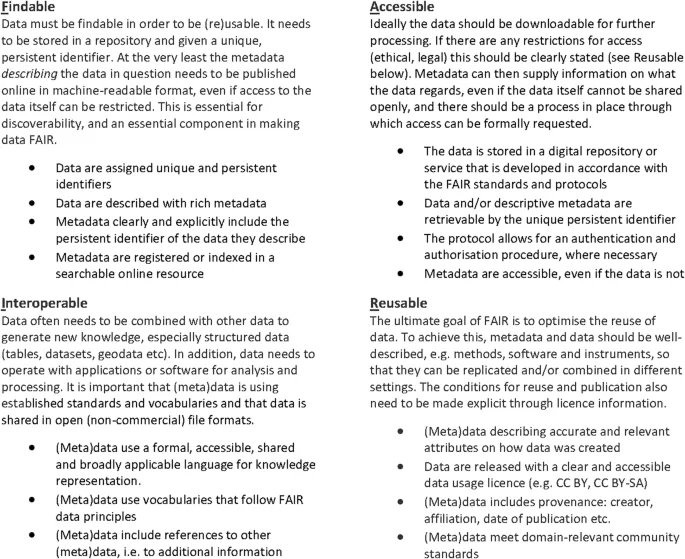
Findable and accessible mainly comes down to selecting a repository that offers the functions listed here. Interoperable and reusable are more about what the producers must take into consideration when creating and sharing their data.
While this paper will be focusing on the FAIR principles, which mainly concern technical aspects, it is important to highlight that there are also principles for the ethical production and use of research data. Especially regarding Indigenous Peoples’ rights and interests, which is highly relevant in many heritage studies. The CARE principles, which have been formulated by the Global Indigenous Data Alliance are a vital complement to the FAIR principles: Collective benefit, Authority to control, Responsibility and Ethics19,20. Proper implementation of FAIR principles can help ensure that communities are able to access information about their own history and that sensitive data is not made accessible in ways that can cause further harm or grief to these communities. This is becoming even more important in the age of data mining and AI.
Despite the demands for Open Science, and requirements by funders and journals alike to publish the data that underpins interpretations, it is still far too rare for researchers to make their data available, especially in the Humanities and Social Sciences. Partly due to a lack of oversight and control21, partly due to a lack of proper support and infrastructures which result in additional workloads22,23. Underlying both is a general uncertainty among researchers on how to apply the general principles and guidelines to their practical work. They have been taught how to write papers for publications but rarely how to create and organise their data so that it is interoperable and even less how to go about publishing said data. Researchers also tend to miss that “data” does not equal “databases”. Data is simply any kind of information on which interpretations are based. A diagram or distribution map shared only as an image in a publication can neither be tested nor reproduced.
This paper sets out to offer concrete recommendations and instructions based on experiences gained from projects at the Heritage Laboratory at the Swedish National Heritage Board, which spanned from South American textiles to prehistoric Scandinavian metals to modern artworks. We will start by demystifying some of the FAIR principles and explain why these are useful and powerful tools for humans as well as machines. What it mostly comes down to is terminology: the words we use to identify and explain our research.
Controlled vocabularies and linked data
The FAIR principles can seem quite daunting when one looks at the long list of specifics. However, many of them are primarily meant for developers of digital services. Complying with standard communication protocols, authentication, indexing and long-term availability, is resolved simply by choosing a digital repository that observes the FAIR principles. For the individual researcher, implementing FAIR principles mainly comes down to two things:
-
Data practice
-
Metadata
We will cover good data practices below, but metadata is essential to every aspect of FAIR and often requires active human participation. Metadata is data that provides information about data. A dataset can contain a lot of metadata, e.g. properties, contextual, technical and methodological information. The more standardised this metadata is, the easier it is for both humans and machines to understand the content. Research data in the form of a computer file, whether photograph, text, diagram, spreadsheet, or database, should come with descriptive metadata that gives a shorthand explanation of the content of the file, just as the keywords selected for an article. Descriptive metadata is essential for a computer to be able to find what you are searching for—especially if the file itself does not contain textual or structured data (e.g. images) (see Fig. 2). Some may be automatically included in file properties, but most must be added by a human being through conscious selection.
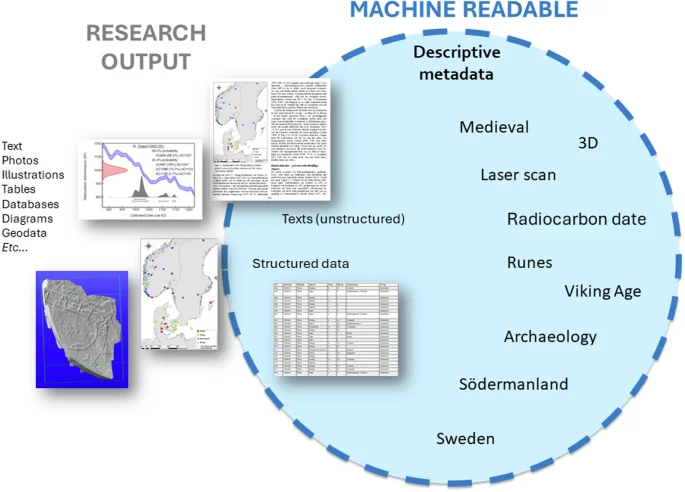
Adding metadata to describe the files makes the content immensely more findable and interoperable.
The concept of descriptive metadata is, of course, much older than computers, but it has become far more important and powerful as a tool in the digital age. Computers can do things quickly that are difficult for humans, so we tend to forget that we can do things that are still beyond most computers’ abilities, even with advances in Artificial Intelligence. The keywords chosen to describe this article are a classic example of metadata selected to help both computers and humans. As a human, you may use these terms in a search engine, and the computer uses them to find matching candidates. Depending on the level of sophistication of the search platform, variations in spelling or expressions can mean viable options are missed. Software is only as smart as it is programmed to be.
The best way to ensure the use of good metadata, whether in a dataset or as a description of a file, is to make use of a thesaurus or vocabulary, i.e. a collection of standardised terms within a specific context. A thesaurus is technically just a collection of words and their synonyms, whereas a vocabulary should contain definitions as well. In reality, these tend to overlap, and the terms are often used interchangeably (thereby being an example of the very issue they are meant to resolve). In this article we will be using the term vocabulary.
Vocabularies are essential since variations in terminologies and practices lead to misunderstandings. Homographs are words with identical spelling but different meanings, and synonyms are words with similar meaning despite completely different spelling. A human can often grasp the meaning through context, but machines need even more help. For a computer to be able to understand these properly, developers make use of controlled vocabularies and predefined lists of terms in order to discern between homographs and to group synonyms24. When you choose a word from a list in a database, or when a web interface suggests alternative spellings as you type, you are benefiting from a controlled vocabulary. While vocabularies can feel restrictive, they are indispensable for machine interoperability. Anyone who has ever tried to search a dataset created with free text registration or to combine different datasets knows this all too well.
Terms in a controlled vocabulary are only unique within that context. For instance, a vocabulary for animals can contain the term “bat”, but so can a vocabulary for sports equipment. A data management system can contain multiple controlled vocabularies with seemingly identical terms, differentiating between them using unique identifiers that are rarely visible to the users. But how is a web application able to correctly interpret homographs and synonyms deriving from many different controlled vocabularies? The way to deal with this is through so-called authorities. An authority is a term in a controlled vocabulary that has a globally unique identifier (GUID), not just unique within a software system (Fig. 3). The GUID should preferably be in the form of a persistent URL, a web link that is created in a way that minimises the risk it will be broken. Unique and durable identifiers make it possible for computers to discern the meaning of a specific term, i.e. it is “machine readable”. By also including alternative spellings or aliases for an authority term it is possible for machines to find correct information despite variations in human input. This is how we can search in Wikipedia for Kopernik, Koppernigk or Copernicus and still find the same article.
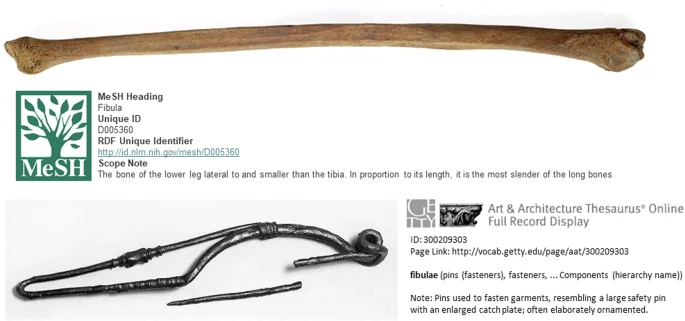
Searching for “fibula” in one will yield the definition of a bone element and, in the other, the description of a pin used to fasten garments. Since they have globally unique identifiers, it is possible to make use of the same terms in a computer system so that they are not confused with each other.
An authority can be used to describe a term with structured metadata, as well as links to other authorities, increasing its usefulness exponentially. In this way a single authority identifier can be used to retrieve information about when and where John III was born, who he married, letters he wrote and to whom, museum objects and artworks associated with him, and in which collections these can be found. An authority connects a term, or an object, to the information super-infrastructure that is the World Wide Web. By including machine-actionable identifiers to describe your data, information from many different sources can be discovered and visualised via computer applications.
Below, we will show how making use of controlled vocabularies and authorities for terms is a very effective way to ensure your research data meets the FAIR criteria, especially in making them findable and interoperable. We will also recommend publicly accessible controlled vocabularies that are a good starting point for Heritage Science, and which are easy for individuals to use.
Method: Workshopping how to publish data
During the project, proposals for tutoring and examples of solutions were workshopped to get feedback on content and working methods. This is to ensure that the deliveries of the project results correspond to the objectives that have existed, as well as to capture the requirements that came up during both group and individual meetings with the laboratory staff. After the initial period of information collection, when curated lists and practice documents began to take shape, the first round of workshops was held in the form of individual sessions with the investigators in the lab. The respective cases were reviewed, and the questions raised at the beginning of the project were again raised and answered to the extent possible. Proposals for relevant vocabulary and authorities were discussed, and the content of the document on good data management practices was reviewed.
A group workshop was then held where the laboratory investigators were given a chance to test the curated lists and try to upload data into Zenodo and enrich it with relevant metadata. It provided good information on what needed to be clarified, improved, and changed in the curated lists and where, in the process, more support was needed.
The practical workshops highlighted the parts of the process that generated the most questions among the laboratory staff, and which tasks were considered the most difficult to resolve on one’s own:
-
Identifying what data to share: Most projects result in a lot of output, including versions of images and datasets, and it is often not immediately evident which will be used in the end in publications, tables, diagrams, etc. Who is the intended user, does anyone even want this data?
-
Preparing data for sharing: A laboratory uses instruments that often generate file formats that are very useful for analysis, provided one has the prerequisite software. But is it this version that should be shared, or a version exported into open source format? The latter may lose some of the functionality, so is it worth it? Can datasets and tables be shared “as is”, or must they be adapted to be useful?
-
Describing data: The point of descriptive metadata for research data is not evident to most people and had to be explained using examples. Even more difficult is choosing the few terms that will sum up the data to make it findable online. Especially in an interdisciplinary field like Heritage Science where interest may come from both the technical and methodological aspects of a project, as well as the cultural and historical aspects of the materials. What will people be searching for? And since descriptions can theoretically be very extensive, what is “good enough”?
-
Responsibility: Who is responsible for ensuring that data is prepared, shared, and described respectively? The main researcher? The project leader? The data creator? A data manager? These are questions that need to be resolved early in a project, for all involved.
-
Workload priorities: It takes time to sort, prepare and publish data. Committing to this is not easy when the usefulness is rarely immediately apparent. Incentives to spend time making data FAIR and open are still not firmly established, and there are few cases of reuse which can be used as inspiration.
The experiences and feedback from the workshop participants were used to put together guides, instructions, and recommendations, which are summarised below.
Results
Good data practices
Good practices when creating and recording data are the basic prerequisite for the result to meet the FAIR principles in the end. Practices that were acceptable (although not optimal) for analogue data have become the biggest obstacle to reaching the full potential of digital data. Machines require a higher degree of rigour to understand data than humans do. Those working in laboratory operations are skilled at learning new instruments and methods, but they have not always been given the opportunity to learn how to ensure that their output is machine-readable and interoperable. It is not certain that the instruments and software used are optimised for those objectives, especially when it comes to commercial products where manufacturers lack incentives to make formats open source.
Basically, good data practices involve four main components:
-
1.
Data content (interoperability)
-
2.
Data description (findability)
-
3.
Data file formats (accessibility)
-
4.
Data licensing (reusability)
Good data content is about using standardised terms whenever possible (from acknowledged vocabularies—see below), especially for the column headings. Do not use abbreviations (unless internationally recognised), variations of spelling (e.g. singular and plural) or special signs in the cells (e.g. “?”). Each cell should also contain only one single observation value (e.g. “silver”, not “silver, lead”). Clarifications and qualifications should be restricted to free text fields.
Data description is about making sure others can identify and understand the relevant source material you are producing. A simple step is naming the files in ways that are descriptive and logical (e.g. not just “File1” or “Report”). Ensure that there is good documentation about the output created within a project, e.g. tables or datasets that list photo descriptions or sample provenances. For instance, a project may have included various types of analysis made on several paintings, resulting in multiple images and datasets. Is it possible for an outsider to understand which files are the results of what type of analysis on which painting, once they are uploaded to a repository and removed from the project folder on your computer? Is there a file that contains the necessary contextual information about your data output, which you can include or link to? Descriptive metadata for the files stored in a repository is also a very important step in ensuring findability and efficiently communicating what the data are about. We will go deeper into how to do this in the section below, on vocabularies for metadata to be used both for data content and description.
As far as possible data should be preserved in open-source file formats to ensure that the content can be accessed. Recommendations for file formats for archiving purposes are published by most national archives, and the US Library of Congress has an online “Recommended Formats Statement” that is continuously updated with information about viable file formats for text, images, 3D, audio, datasets, table data, GIS, etc. which is very much recommended. However, laboratory instruments often deliver results in specialised proprietary file formats that cannot be converted into open versions without losing many valuable properties. A solution in those cases can be to publish both the original data file as well as an export of the most important data in an open file format, thereby ensuring both immediate and long-term usability. Scientific communities are also taking an increasingly active role in developing good practices for preserving and sharing data within specific fields, including those relevant to Heritage Science (e.g. refs. 25,26,27). The goal is not to be perfect in all your data practices but to at least take some basic steps ensuring the results can be found, accessed and used by your peers.
When research data is published online, it should be complemented with information that outlines the terms for reuse, preferably in a short, easy-to-understand format. There are a number of different licenses available, the most common ones being the licences maintained by Creative Commons, an international nonprofit organization (https://creativecommons.org/share-your-work/cclicenses/). The one most commonly used in scientific publications is CC BY, which enables others to distribute, remix, adapt, and build upon the material in any medium or format, so long as attribution is given to the creator—i.e. a citation. This is very much in accordance with long-established practices around the reuse of published research, and it is the default license for data uploaded to EU platforms like Zenodo. While it can be tempting to add extra restrictions, this is generally not a good idea if you want your work to be properly used and cited. Many publishers of research in journals and books are commercial ventures, meaning a BY-NC (non-commercial) or even a BY-SA (share alike) suffix causes problems. Non-derivative (ND) means no changes can be made to the digital object, such as cropping part of an image or combining it with another. It may also be going against funders’ requirements to use other licenses than CC BY or CC0 (public domain) as these are the only two CC licenses considered truly open.
The CC BY license ensures that you maintain the right to be cited when someone else uses your data in their own research. As for misuse of research results, that can, of course, always happen. There are additional rules and regulations that govern acceptable practices in research. By having a public record of all your work, and clear rules for its reuse, you are better protected in such cases than if you only stored it on your own computer.
Vocabularies and metadata for Heritage Science
As mentioned above, descriptive metadata is essential for making your data discoverable on the internet and an essential part of the FAIR principles. However, as became very clear during the workshops, this is an area where the general recommendations available often leave people struggling with where to start and what to focus on. Disciplines have different requirements and priorities, and there is a growing awareness that guidelines must be tailored with that in mind26,28,29. Heritage Science faces an additional challenge due to its inherent interdisciplinarity. Recommendations aimed at laboratory disciplines tend to forget that cultural, spatial, and temporal contexts are a vital part of heritage objects. At the same time, researchers within the cultural heritage field can miss that chemists and physicists will be looking for research based on instruments used and types of material components being analysed. It became quite clear during the workshops and afterward during follow-up meetings that many felt overwhelmed when faced with all the possible metadata that could theoretically be used to describe a set of research data. However, the point of descriptive metadata is not to cover every possible description but to create a good foundation for discoverability.
Using finished and ongoing projects selected by the participants as case studies we identified categories of metadata that are relevant for many Heritage Science projects. These cases covered everything from modern art to historic and prehistoric materials from different parts of the world (weapons, coins, textiles, manuscripts, etc.), as well as modern materials used in museum exhibits. Instruments and methods available at the Heritage Laboratory include radiography, Raman, infrared and UV–Vis spectroscopy, X-ray fluorescence mapping, electron and optical microscopy and various advanced imaging capabilities, as well as instruments for climate measurement, accelerated ageing tests and tensile and compression testing. Based on this combination of a variety of materials and methods we were able to identify and test a number of available vocabularies and test their usability with the participants both during group workshops and individually as the data was uploaded to Zenodo. The process allowed us to identify some main categories of metadata that are useful as a starting point for descriptive metadata in most heritage science projects, though not all of them will be relevant in every case.
By adding descriptive metadata for at least some of the categories listed below, researchers will have made a good effort to make their data discoverable. We will start with the categories themselves, and then we will present the controlled vocabularies that we recommend you use for specific categories. However, as discussed below there are areas where these vocabularies fall short. Finding georeferenced authorities is especially difficult, as geographical entities vary over time and disappear (e.g. Roman Empire) or are culturally or ethnically defined rather than politically (e.g. Sápmi).
Recommended categories of metadata for Heritage Science
Use standardised terms in vocabularies within these categories (if relevant) when describing your research data in a repository.
-
Subjects (e.g. Heritage Science, Archaeology, Art History, Literature, etc.)
-
Materials (e.g. copper, bone, vellum, pigment, silk, paper)
-
Method/Instrument (e.g. microscopy, photogrammetry, SEM-EDS, X-ray)
-
Geographical context (e.g. Country, Region and/or Site name)
-
Time or style period (e.g. European Bronze Age, Tang dynasty, Olmec, Expressionism)
-
Object type (e.g. brooch, coin, flute, oil painting, missal, temple)
-
Person (e.g. name of Artist or the name of a person featured in a work of art or literature)
-
Object identifier (collection identifiers for the artefacts, artworks, samples, or unique identifiers for sites or buildings)
Object identifiers in the form of authority for a specific artefact, artwork or archival object are becoming more common as digitalised collections are made available online (Fig. 4). Collection databases contain rich metadata about an item, meaning a lot more information can be communicated in a machine-actionable manner just by including the object identifier as liked data in your dataset. If the items analysed in a project do not have globally unique identifiers (as compared to inventory numbers which are not unique), consult with the collection caretaker to see if it is possible to generate them. Using these will make it easier to pinpoint the exact item that was analysed helping curators and researchers to keep track of what types of analyses have been done on items and where the results have been published.
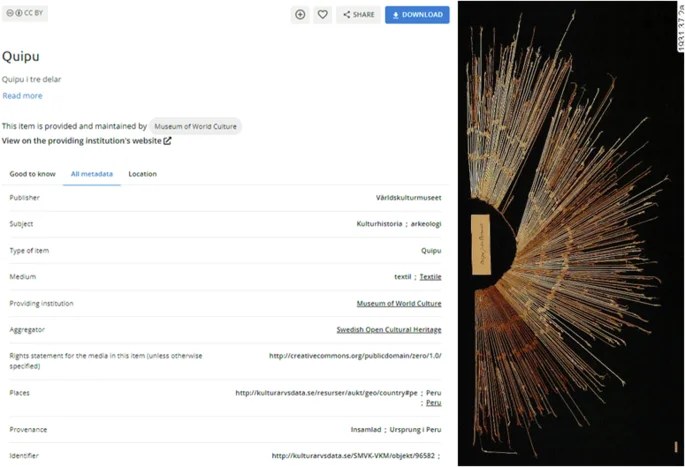
Metadata is harvested using linked data published by the collections, and some of the terms are machine-translated, making the items searchable in other languages. https://www.europeana.eu/en/item/91608/SMVK_VKM_objekt_96582 (captured 2024-05-18, edited for publication).
The forthcoming European Research Infrastructure for Heritage Science, E-RIHS, and its precursor project IPERION HS, have initiated a pilot collaboration with OpenAIRE to implement Open Science in the field of heritage science30. The IPERION HS data management plan summarises the types of relevant data for heritage science projects as well as how such data shall be preserved and published adhering to FAIR principles31,32. While the implementation of open access is still in its infancy in the field of Heritage Science, the Natural Science museums in Europe have progressed to develop an international research infrastructure called DiSSCo, where scientific data and images are linked to specific museum specimens to create so-called “FAIR Digital Objects” (FOD)33.
Such infrastructures could decrease the need for costly and potentially destructive transportation of museum objects, specimens, and will potentially make it easier to build upon previous results. FAIR data practices can reduce the gap between researchers and collection managers, and highlight the ways in which collections in museums, archives and libraries continue to generate new knowledge.
Recommended controlled vocabularies for Heritage Science
Below is our selection of controlled vocabularies that cover categories relevant to Heritage Science. The common criteria are that they are maintained by stable organisations and have a fairly user-friendly web search. In Table 1, they are presented with the categories of metadata each is primarily useful for, with links to the web search and an example of an “authority”, i.e. a persistent URL (web link), for both human and machine-readable definitions. Unfortunately, the persistent URL is rarely the weblink one sees in the internet browser’s address field. To make matters worse, almost every vocabulary has a different way of referring to their authorities (e.g. “permalink”, “concept URI”, “RDF Unique Identifier”), the meanings of which are far from obvious to the average user. We have therefore made a notation of that as well in Table 1. It would certainly be beneficial to everyone if there was a more standardised terminology in this field as well.
The list of vocabularies is not meant to be exhaustive or definitive. As we will address in the final discussion there is a problematic lack of vocabularies necessary for the Humanities and Cultural Heritage disciplines. However, we believe these are a good starting point that will help make people more comfortable with using vocabularies and persistent identifiers. Some vocabularies have overlapping content, i.e. the same term or name appears in them, but there is no need to use multiple authorities for a term. There are digital services that specifically map authorities for terms to each other (e.g. Wikidata and VIAF—see below). This mapping of authorities works as an online thesaurus for web applications, meaning they can understand terms regardless of which controlled vocabulary is used. Just choose the authority (persistent unique identifier) for a term whose description is accurate enough to what you are referring to. There is no problem mixing authorities from different controlled vocabularies when describing your data. In fact, it will most likely be necessary, as there is no single controlled vocabulary that encompasses all terminologies (not even Wikidata—yet).
Getty vocabularies
Developed and published by The Getty Research Foundation, these are the most commonly used vocabularies in heritage studies. Many collection management systems are mapped to the Getty vocabularies, as are many other web and software applications in various languages, meaning they are linguistically interoperable to a very high degree. The vocabularies are hierarchically structured, meaning a term is linked to a more general and often a more specific term. This is a highly useful feature, as it means that a machine will understand that research data described with the metadata “earrings” is relevant when someone is looking for analyses made on jewellery (or “jewelry”).
Getty publishes a number of vocabularies, of which the Art & Architecture Thesaurus (AAT) is the most well-known and covers anything from archaeology to modern art. The Thesaurus of Geographic Names (TGN) has georeferenced places, including historical placenames. The Union List of Artist Names (ULAN) also includes names of organisations, repositories, etc. The Cultural Objects Name Authority (CONA) has names of artworks and architecturally significant places. The Iconography Authority (IA) lists religious terms and has a special focus on non-Western topics.
It should be noted that the Getty vocabularies mainly contain terms relevant to the Institute’s own extensive collections. Other museums, archives, etc., can contribute terms to expand them further, but this is only done occasionally. Consequently, the Getty vocabularies are mainly dominated by terminologies for objects that have found their way into Western collections or are part of the Western cultural canon. Something that will be discussed further below.
MesH
Medical Subject Headings (MeSH) is a controlled vocabulary produced by the US National Library of Medicine. Getty AAT contains a lot of terms for scientific instruments and methods that are relevant to Heritage Science, but MeSH contains practically all of them and is updated more regularly. It is a useful vocabulary if a scientific instrument or method is missing from Getty AAT, or if the definition there does not match what the instrument was used for (e.g. analyses of modern material properties).
MeSH also contains authorities for anatomical terms for bones, which can be useful when one wants to differentiate between an analysis made on a fibula (dress pin) or a fibula (shin bone) (see Fig. 3).
Gold Book (Compendium of Chemical Terminology)
The International Union of Pure and Applied Chemistry (IUPAC) publishes the Compendium of Chemical Terminology, popularly referred to as the Gold Book, as a controlled vocabulary online. It is a good complement to Getty AAT and MeSH when making sure to use standardised chemical terminologies and units of measurement or needing the authorities for these.
GeoNames
GeoNames is an open database containing georeferenced information about almost every type of geographical entity. There are often multilingual versions of place names, as well as links to Wikipedia, increasing the interoperability of its authorities. There can be issues about spatial accuracy in some cases34, especially for historical sites35. Pinpoint accuracy is mainly a problem when using the data for GIS analysis, not for someone who simply wants a unique identifier for a location. However, it is recommended to check on the map that the placement is fairly correct. It is possible for users to log in and correct errors or add additional information, such as alternate spellings, just as on Wikidata. This was done for one of the case studies at the Heritage Laboratory, which involved radiographic analysis on arrow points found at Låktatjåkko in northern Sweden36. As is the case with many Sami place names it has several alternative spellings (Låktatjåkka, Loktačohkka, Laktatjakko), one of which was added to GeoNames as a result of the workshop (Fig. 5).

The site Låktatjåkko has many alternative spellings, as seen below the main name. The identifier within the controlled vocabulary is the number 2697793, which, when paired with the domain name becomes a globally unique identifier (GUID): https://www.geonames.org/2697793. As seen in the image, the GUID (authority) also contains a lot of additional information, such as the coordinates, the region and country, etc.
VIAF
The Virtual International Authority File (VIAF) is a joint project for libraries and is maintained by the Online Computer Library Center (OCLC). This means that VIAF aggregates vocabularies from a large number of libraries across the world, and the authorities are mapped and linked back to these resources. The content is therefore highly trustworthy, as well as very multilingual and interoperable. VIAF contains names of people and organisations associated with published works, as well as titles of said works.
Wikidata
The most extensive and ubiquitous publisher of vocabularies on the internet is the Wikimedia Foundation. The service Wikidata contains all the items (terms, topics, concepts, objects) that have their own page in Wikipedia, as well as a huge number of aliases of said terms. As mentioned above, this is what allows users to find the correct page for terms with identical spelling, and to find the information online regardless of language and alphabet used during a search. Wikidata is readable by both machines and humans.
While using a Wikipedia article as a reference is problematic due to the anonymous and always-changing nature of the contributions, the same is not necessarily true for Wikidata identifiers. The former contains interpretations and opinions, whereas the latter contains mainly factual data in the form of structured metadata (which, of course, can be incorrect). As mentioned above, Wikidata authorities always have links to identifiers in other controlled vocabularies, as a way of reference. This means that Wikidata works as a global hub, mapping and connecting identifiers for terms across the internet. Like VIAF, but on a much greater scale. This is extremely useful for computer applications, which can use these connections to find, filter and visualise information from a combination of sources. It is also useful for humans. If you have been unable to find a term in any of the above vocabularies, looking it up in Wikidata will often yield a result, which in turn can help you discover additional useful controlled vocabularies if you are reluctant to use Wikidata as a reference.
Finally, Wikidata allows for user-generated authorities. This can be a solution if there is a term, person, or object that you can define and describe, but which does not yet have a globally unique identifier. One of the projects at the Heritage Laboratory involved an oil painting by Picasso at the Swedish Museum of Modern Art, La Source, whose identifier was neither globally unique nor persistent. The name of the painting is identical to several other artworks, including a drawing by the same artist held by another museum. A Wikidata identifier was, therefore created as part of the project. This is not a viable solution for every collection item, but a painting by such a renowned artist met the criteria for relevance.
Additional vocabularies
The vocabularies listed above cover broad areas and have fairly user-friendly interfaces. There are three additional ones that we would like to highlight. Two of them are a bit more challenging in terms of interface and content, and one is geared towards a disciplinary niche.
PeriodO
One type of metadata that is very important to heritage studies is chronology. This is also one of the most complex to get right. Common concepts like “Neolithic” and “Middle Age” have different start and end dates depending on the region, just as there is a huge variety of names for prehistoric and historic periods and cultures in different countries.
PeriodO is a gazetteer, i.e. a geographical directory, with definitions of historical, art-historical, and archaeological periods. It is developed through both individual and organisational contributions, funded by the US National Endowment for the Humanities and the Institute of Museum and Library Services. It works similarly to VIAF in that it aggregates controlled vocabularies from various sources and creates authorities for them. The PeriodO web client allows users to search for periods broadly in all the controlled vocabularies, or to select a preferred vocabulary and search in that. The authorities do not just have a chronological definition but are also georeferenced, though sometimes only broadly through linking them to places as defined by Wikipedia (e.g. “France”).
The web client takes some getting used to, and the choices can feel a bit overwhelming as the vocabularies are a mix of project and organisational contributions. Getty AAT and Wikidata are often good enough for authorities on general chronological periods. However, we feel it is worth highlighting this service for those not finding what they need elsewhere, or who are looking for more advanced options.
FISH—Heritage Data
Forum on Information Standards in Heritage (FISH) is a British non-profit, whose membership includes many of the national and regional agencies for archaeology, history, and heritage in the UK. Despite what the acronym seems to suggest, the FISH vocabularies are not aimed at aquatic wildlife. Instead, they cover everything from archaeological sciences and objects to building materials to historical maritime and aircraft terms. These are published in several different formats, including as CSV and PDF, which are very helpful in finding standardised heritage terminologies in English just in general.
The vocabulary terms are also published as authorities in a web search. However it requires the user to first choose which one of the numerous vocabularies they want to search in. It is explained in the Schemes table what subjects each vocabulary covers, and choosing one of the FISH vocabularies by Historic England is generally a good starting point. There are other vocabularies specialised in Scottish, Welsh, and Irish heritage terminologies as well, which can be very useful.
Nomisma
Nomisma is a controlled vocabulary for concepts associated with numismatics, particularly coinage from the ancient Mediterranean cultures and historical European countries. It was started through individual initiatives but now has received funding from the International Numismatic Council and is overseen by a scientific steering committee. Apart from being helpful in finding standardised terminologies, there are geographical, multilingual, and linked data associated with many of the authorities, making them highly interoperable (Fig. 6).
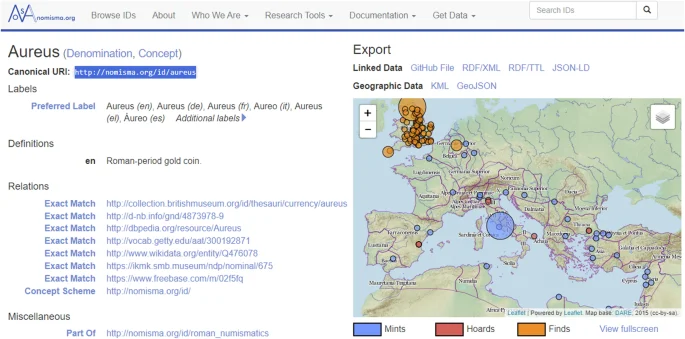
Example from Nomisma, which in addition to publishing a controlled vocabulary with unique identifiers for various terms, places and names associated with numismatics, also shows what can be accomplished in terms of visualisation when items are registered using these authorities (or “Canonical URI” as they are called here).
While the terms, people and places in Nomisma can often be found in the vocabularies mentioned above, it could be a good idea to use these authorities when describing research data that was generated analysing coins and medals. It will make the results more discoverable by a highly active research community.
Discussion
Hopefully the examples and recommendations outlined in this paper can help researchers within the field of Heritage Science to make their results more findable and useful to others. The long-term effects will include making the contributions of the field more evident to a wider audience, in addition to researchers receiving more credit for their work. When talking about the requirements for FAIR data there is often an undercurrent of worry that research results will be “stolen”, i.e. appropriated without credit, or even misrepresented. However, FAIRifying data is actually the best possible protection against plagiarism and unethical uses. By preserving your data in a trusted repository, and making it findable and accessible, you will have documented evidence of what you did and when. In short, it is in your own personal interest to publish your data before someone else does and claims it as theirs. This kind of official record-keeping will only become more important to the scientific community with the exponential development of Artificial Intelligence and Machine Learning algorithms. It is also possible to get credit for the time consuming work of building datasets with various types of analysis on materials and make them available for others27,37. The Open Science movement is essentially about improving the way we do science, enabling reproducibility and increasing transparency, but it is also about making more of the scientific process visible and rewarding more than the publication of articles, such as collaboration and knowledge sharing38,39.
A very clear result from the workshops with the Heritage Laboratory is how much more time-consuming it is to make data FAIR after a project has been completed. Not having a plan from the start of how to document and organise data results in having to find and rename files, create tables from scratch, and figure out which controlled vocabularies and metadata are relevant when too much time has passed. It is all too easy to push data delivery to the future but doing it concurrently with the project saves a lot of time in the end. Especially if your contributions will take place long before the completion of the main project. Having to sort and organise your data years later, when the results are finally being published in a journal, is both time-consuming and frustrating. Instead, you could have it all prepared and available in a repository under an embargo, able to be released with the press of a button. That way you can also include this work on your résumé at an earlier stage.
Interestingly, we found that perhaps the most challenging and fraught part of making data FAIR is simply settling on which descriptive metadata to use when uploading to a repository since it requires making choices that predict the needs and interests of other people. This supposedly simple task caused discomfort and anxiety among our participants, partly because there was no direct template available and partly because of the digital aspect. Some got stuck on finding the perfect definition of a specific term, feeling the one in a controlled vocabulary was not absolutely correct. Others worried about not including every possible term that someone might search for. “Good enough” was a mantra that had to be used over and over again, and it is something that can only really be overcome through repeated practice and becoming comfortable with the process.
The final challenge is something that unfortunately cannot be overcome by individual effort: accessibility to useful controlled vocabularies. As mentioned above, controlled vocabularies were initially created by and for software developers. While several are now published online, the user-friendliness of the web interface often leaves a lot to be desired. It is rarely easy to find the actual authority for a term in an online controlled vocabulary, i.e. the persistent and globally unique identifier. Not only are they almost never the same as the link shown in the web address field, but the terminologies vary so much that hardly a single one of the controlled vocabularies that we are recommending uses the same term to denote the persistent identifier. Ironically, controlled vocabularies (thesauri, ontologies, terminologies, etc.) could benefit from a bit more standardised vocabulary themselves.
To find unique and persistent identifiers for specific objects in collections is also complicated. Being able to accurately identify which particular artefact, sample or work of art that has been analysed should be of utmost importance for obvious reasons. While collections are now being digitalised into management systems, it requires a bit more to also generate identifiers that are globally unique outside of those systems. Even when such identifiers exist, they can be just as difficult to find as the authorities in controlled vocabularies. Collection curators should, in theory, be even more invested in ensuring it is easy to find results from research done on their objects, but they rarely know to insist on this when supporting a project, nor do researchers know to ask. Close collaboration and good communication between researchers and curators are important in bringing about change in this area.
However, the most challenging problem is perhaps the fact that available controlled vocabularies tend to favour the subjects that have historically received the greatest attention from Western societies, and thereby been given prominence in their collections. Not surprisingly, vocabularies describing events, places and items from the British Isles, Italy, Greece, France, and the United States are far more accessible online than those covering cultural-historical terminologies from other parts of the world. Online discoverability is a privilege that currently favours research on the areas that search engines and services can understand, and the problems this poses should not be underestimated. The creation and publication of vocabularies for historically under-represented regions, cultures and time-periods is something that should be given more funding and support going forward, or we will be recreating the same lopsided view of world events and human history digitally as well.
Conclusions
In conclusion, we find that there are real practical challenges to reach the goals set by Open Science and research funders, that will not be solved by waiting for the development of a perfect digital service or expecting an institution to take care of everything. Researchers and specialists need to learn the basic premises of good data management in order to be able to do their part. These basic premises are not nearly as complicated as one could think, reading through the technical jargon for the FAIR principles. That said, there is still a lack of support and services aimed at teaching researchers of all career stages how to create, name, and preserve their data in ways that follow the FAIR principles. Many university libraries are now taking up this challenge and are developing better support systems40. Unfortunately, many researchers and specialists no longer have access to the services provided by universities, as they are working at museums, archives or independent laboratories.
For the individual, implementing FAIR research data is not about learning complex digital technologies. However, it does require grappling with subjects that can be even more daunting and difficult: e.g. legal and ethical issues surrounding licensing and accessibility, security aspects on storage, formats for digital preservation, or simply just identifying what of all the data and information generated by a project should be preserved and published. What is “good enough”? The general principles put forth by funders and government agencies are not enough. We need handbooks and teaching materials that take into consideration the ways in which research is done by different kinds of disciplines. Especially for the Humanities and Social Sciences whose methods and types of data are even more varied than those in the Natural Sciences.
Finally, we want to end on a positive note. The development of infrastructures for preserving and sharing research data, not just articles or other forms of publication, is a genuinely good thing. It will help battle falsifications and plagiarism, and in the long run, it will increase the possibility of expanding our knowledge. For the individual researchers, it will not just be the texts they publish that will count towards their merits, but the actual data and information that they have produced—often the thing that took the most time and effort. There will be a record of that data, meaning there is a way to claim ownership and get proper accreditation. There will always be a period of frustration when having to learn new methods and change one’s routines, but most of what is asked in this case is not difficult to get started with and should be a natural part of any scientific project: good documentation and publication strategies.
Data availability
No datasets were generated or analysed during the current study.
Abbreviations
- CIDOC – CRM:
-
International Committee for Documentation—Conceptual Reference Model
- EOSC:
-
European Open Science Cloud
- E-RIHS:
-
European Research Infrastructure for Heritage Science
- FAIR:
-
Findable, Accessible, Interoperable, Reusable
- GBIF:
-
Global Biodiversity Information Facility
- GUID:
-
Global Unique Identifier
- ICOM:
-
International Council of Museums
- IPERION HS:
-
Integrating Platforms for the European Research Infrastructure on Heritage Science
- INFN-CHNet:
-
Istituto Nazionale di Fisica Nucleare—Cultural Heritage Network
- SSHOC:
-
Social Sciences & Humanities Open Cloud
References
-
Hermon, S. & Niccolucci, F. FAIR data and cultural heritage special issue editorial note. Int. J. Digit. Libr. 22, 251–255 (2021).
Google Scholar
-
Richards J. D. Joined up Thinking: aggregating archaeological datasets at an international scale. Internet Archaeol. http://intarch.ac.uk/journal/issue64/3/index.html (2023).
-
Castelli, L., Felicetti, A. & Proietti, F. Heritage Science and Cultural Heritage: standards and tools for establishing cross-domain data interoperability. Int. J. Digit. Libr. 22, 279–287 (2021).
Google Scholar
-
Bucciero, A. et al. DataSpace-ISPC: a semantic platform for Heritage Science. In EUROGRAPHICS Workshop on Graphics and Cultural Heritage 109–117 (The Eurographics Association, 2023). Available from: https://doi.org/10.2312/gch.20231166.
-
Santo, M. D., Niccolucci, F. & Richards, J. JOCCH Special Issue on Digital Infrastructures for Cultural Heritage (2nd part) Guest Editors: Massimo De Santo, Franco Niccolucci, and Julian Richards. J. Comput. Cult. Herit. 10, Artcle no. 13, 1 (2017).
-
Santo, M. D., Niccolucci, F. & Richards, J. Editorial: Special Issue on digital infrastructures for cultural heritage. J. Comput. Cult. Herit. 10, 1ee:1–1ee:2 (2017).
-
European Commission DG for R and I, Brunet, P., De Luca, L., Hyvönen, E., Joffres, A., Plassmeyer, P. et al. Report on a European Collaborative Cloud for Cultural Heritage: Ex-ante Impact Assessment 2022 (Publications Office of the European Union, accessed 25 July 2024); https://data.europa.eu/doi/10.2777/64014.
-
Boeckhout, M., Zielhuis, G. A. & Bredenoord, A. L. The FAIR guiding principles for data stewardship: fair enough? Eur. J. Hum. Genet. 26, 931–936 (2018).
Google Scholar
-
Jaunsen, A. O. et al. Nordic FAIR data collaboration opportunities. Zenodo https://doi.org/10.5281/zenodo.6476940 (2021).
-
Larsson, Å. M. & Bornsäter, B. FAIR data för Heritage Science: utvecklad praktik för öppna forskningsdata: rapport från ett FoU-projekt. Riksantikvarieämbetet http://urn.kb.se/resolve?urn=urn:nbn:se:raa:diva-8281 (2023).
-
Fecher, B. & Friesike, S. Open Science: one term, five schools of thought. In Opening Science: The Evolving Guide on How the Internet is Changing Research, Collaboration and Scholarly Publishing (eds Bartling, S. & Friesike, S.) 17–47 (Springer International Publishing, Cham, 2014).
-
EU Commission. 2012/417/EU: Commission Recommendation of 17 July 2012 on Access to and Preservation of Scientific Information. http://data.europa.eu/eli/reco/2012/417/oj/eng (2012).
-
Swedish Research Council. Proposal for National Guidelines for Open Access to Scientific Information. https://www.vr.se/english/analysis/reports/our-reports/2015-03-02-proposal-for-national-guidelines-for-open-access-to-scientific-information.html (2015).
-
Directorate-General for Research and Innovation (European Commission). Horizon Europe, Open Science: Early Knowledge and Data Sharing, and Open Collaboration (Publications Office of the European Union, 2021). https://data.europa.eu/doi/10.2777/18252.
-
Devarakonda, R., Prakash, G., Guntupally, K. & Kumar, J. Big Federal Data Centers implementing FAIR data principles: ARM Data Center example. In 2019 IEEE International Conference on Big Data (Big Data) 6033–6036 (The Institute of Electrical and Electronics Engineers, 2019).
-
European Parliament, Council of the European Union. Directive (EU) 2019/790 of the European Parliament and of the Council of 17 April 2019 on Copyright and Related Rights in the Digital Single Market and Amending Directives 96/9/EC and 2001/29/EC. L130/92. http://data.europa.eu/eli/dir/2019/790/oj (2019).
-
CESSDA Training Team. CESSDA Data Archiving Guide version 3.0. CESSDA ERIC. https://dag.cessda.eu/ (2024).
-
Wilkinson, M. D. et al. The FAIR Guiding Principles for scientific data management and stewardship. Sci. Data 3, 160018 (2016).
Google Scholar
-
Carroll, S. R., Herczog, E., Hudson, M., Russell, K. & Stall, S. Operationalizing the CARE and FAIR Principles for Indigenous data futures. Sci. Data 8, 108 (2021).
Google Scholar
-
Carroll, S. R. et al. Using indigenous standards to implement the CARE principles: setting expectations through tribal research codes. Front. Genet. 13, https://www.frontiersin.org/articles/10.3389/fgene.2022.823309 (2022).
-
Crawford, K. A. & Mazzilli, F. Challenges in open data: a TRAJ perspective. Theor. Roman Archaeol. J. 5, https://traj.openlibhums.org/article/id/9899/ (2022).
-
Bornsäter, B. The Road to Open Science: the Origin and Design of the Research Data Support at Six Swedish University Libraries (Vägen mot öppen vetenskap: Tillkomsten och utformningen av forskningsdatastödet vid sex svenska universitetsbibliotek). MA thesis. Uppsala universitet (2022) (in Swedish).
-
Hostler, T. J. The invisible workload of open research. J. Trial Error https://journal.trialanderror.org/pub/the-invisible-workload/release/1 (2023).
-
Harpring, P. Introduction to Controlled Vocabularies: Terminologies for Art, Architecture, and Other Cultural Works 1st edn (Getty Research Institute, Los Angeles, 2010).
-
McEwen, L., Martinsen, D., Lancashire, R., Lampend, P. & Davies, A. N. Are your spectroscopic data FAIR? Spectrosc. Eur. World https://www.spectroscopyeurope.com/td-column/are-your-spectroscopic-data-fair (2024).
-
Kemmer, I. et al. Building a FAIR image data ecosystem for microscopy communities. Histochem. Cell Biol. 160, 199–209 (2023).
Google Scholar
-
Cortea, I. M. Towards FAIR data management in heritage science research: updates and progress on the INFRA-ART spectral library. Heritage 7, 2569–2585 (2024).
Google Scholar
-
Devaraju, A. et al. FAIRsFAIR data object assessment metrics (0.5). Zenodo https://zenodo.org/record/6461229 (2022).
-
Huber, R. et al. D5.1 Implementing metrics for automated FAIR digital objects assessment in a disciplinary context. https://zenodo.org/record/7784120 (2023).
-
Bardi, A. & Benassi, L. Boosting Open Science in the IPERION HS research infrastructure with OpenAIRE. ERCIM News 133, 9–10 (2023).
-
Padfield J. D6.1 data management plan for IPERION HS. https://zenodo.org/record/5785981 (2021).
-
Benassi, L. IPERION HS how to do—open science: EU rules and tips. https://zenodo.org/record/4459152 (2021).
-
Koureas, D., Livermore, L., Alonso, E., Addink, W. & Casino, A. DiSSCo Prepare Project: increasing the implementation readiness levels of the European research infrastructure. Res. Ideas Outcomes 9, e107220 (2023).
Google Scholar
-
Ahlers, D. Assessment of the accuracy of GeoNames gazetteer data. In Proc. 7th Workshop on Geographic Information Retrieval (GIR ’13) 74–81 (Association for Computing Machinery, New York, NY, USA, 2013).
-
Harvey, F. & Doroshenko, I. Evaluating the use of gazetteer data for locating former concentration camp subcamps. GI_Forum 8, 3–13 (2020).
Google Scholar
-
Mårtensson, M. Radiography on Historical Arrows https://zenodo.org/record/7744059 (2015).
-
Plomp, E. et al. The IsoArcH initiative: working towards an open and collaborative isotope data culture in bioarchaeology. Data Brief. 45, 108595 (2022).
Google Scholar
-
Bertram, M. G. et al. Open science. Curr. Biol. 33, R792–R797 (2023).
Google Scholar
-
Umbach, G. Open Science and the impact of Open Access, Open Data, and FAIR publishing principles on data-driven academic research: towards ever more transparent, accessible, and reproducible academic output? Stat. J. IAOS 40, 59–70 (2024).
-
van der Graaf, M. Research Data Management Support Services by Libraries—A LIBER/ADBU Toolkit https://zenodo.org/record/8101818?mc_cid=8a251bc3d5&mc_eid=a7bcb8a274 (2023).
Acknowledgements
The project was made possible thanks to the support and encouragement of Head of Unit Stefan Nilsson, and the participation and contributions of the staff at the Heritage Laboratory: Elyse Conosa, Kathrin Hinrichs Degerblad, Magnus Mårtensson, Sara Norrehed, Tom Sandström and Kaj Thuresson. This investigation was funded by a Research & Development Grant (RAÄ-2022-85) from the Swedish National Heritage Board.
Author information
Authors and Affiliations
Contributions
Å.M.L. and B.B. wrote the main manuscript text. M.H. contributed with text on the Heritage Laboratory and helped with revisions of the full manuscript. ÅML prepared the figures. All authors reviewed the manuscript and made revisions after the reviewer’s response.
Corresponding author
Ethics declarations
Competing interests
The authors declare no competing interests.
Additional information
Publisher’s note Springer Nature remains neutral with regard to jurisdictional claims in published maps and institutional affiliations.
Rights and permissions
Open Access This article is licensed under a Creative Commons Attribution 4.0 International License, which permits use, sharing, adaptation, distribution and reproduction in any medium or format, as long as you give appropriate credit to the original author(s) and the source, provide a link to the Creative Commons licence, and indicate if changes were made. The images or other third party material in this article are included in the article’s Creative Commons licence, unless indicated otherwise in a credit line to the material. If material is not included in the article’s Creative Commons licence and your intended use is not permitted by statutory regulation or exceeds the permitted use, you will need to obtain permission directly from the copyright holder. To view a copy of this licence, visit http://creativecommons.org/licenses/by/4.0/.
Reprints and permissions
About this article
Cite this article
Larsson, Å.M., Bornsäter, B. & Hacke, M. Developing practices for FAIR and linked data in Heritage Science.
npj Herit. Sci. 13, 53 (2025). https://doi.org/10.1038/s40494-025-01598-x
-
Received: 18 May 2024
-
Accepted: 08 December 2024
-
Published: 01 March 2025
-
DOI: https://doi.org/10.1038/s40494-025-01598-x