Abstract
The placenta plays a crucial role in protecting the fetus from environmental harm and supports the development of its brain. In fact, compromised placental function could predispose an individual to neurodevelopmental disorders. Placental epigenetic modifications, including DNA methylation, could be considered a proxy of placental function and thus plausible mediators of the association between intrauterine environmental exposures and genetics, and childhood and adult mental health. Although neurodevelopmental disorders such as autism spectrum disorder have been investigated in relation to placenta DNA methylation, no studies have addressed the association between placenta DNA methylation and child’s cognitive functions. Thus, our goal here was to investigate whether the placental DNA methylation profile measured using the Illumina EPIC array is associated with three different cognitive domains (namely verbal score, perceptive performance score, and general cognitive score) assessed by the McCarthy Scales of Children’s functions in childhood at age 4. To this end, we conducted epigenome-wide association analyses, including data from 255 mother-child pairs within the INMA project, and performed a follow-up functional analysis to help the interpretation of the findings. After multiple-testing correction, we found that methylation at 4 CpGs (cg1548200, cg02986379, cg00866476, and cg14113931) was significantly associated with the general cognitive score, and 2 distinct differentially methylated regions (DMRs) (including 27 CpGs) were significantly associated with each cognitive dimension. Interestingly, the genes annotated to these CpGs, such as DAB2, CEP76, PSMG2, or MECOM, are involved in placenta, fetal, and brain development. Moreover, functional enrichment analyses of suggestive CpGs (p < 1 × 10−4) revealed gene sets involved in placenta development, fetus formation, and brain growth. These findings suggest that placental DNA methylation could be a mechanism contributing to the alteration of important pathways in the placenta that have a consequence on the offspring’s brain development and cognitive function.
Introduction
The process of the human brain developing is a protracted process that starts in the third week of gestation with the differentiation of the neural progenitor cells and lasts at least until late adolescence, and possibly throughout life [1]. Early gene expression changes and environmental factors play a crucial role in the normal development of the brain [2]. In particular, placenta is posited as a critical determinant of immediate and long-term neurodevelopmental outcomes in children [3]. The placenta–brain axis refers to this close relationship between the placenta and the brain [4], and, as recently reviewed by Kratimenos et al. [5], compromised placental function can predispose an individual to psychiatric disorders. During pregnancy, placenta is the organ that transfers oxygen and nutrients from the mother’s blood to the fetal brain. Throughout the whole pregnancy, placenta plays a crucial role as it protects the fetus from environmental harm and supports the development of its brain. Additionally, the placenta aids in immune system control and functions as a neuroendocrine organ, generating hormones that act as growth factors and neuropeptides that operate as neurotransmitters [6].
Genomic and epigenomic changes in the placenta have been linked to neurodevelopmental abnormalities and typical neurobehavioral development in epidemiological and animal studies [7]. In particular, the epigenome can be considered a proxy of placental function during pregnancy and a plausible mediator of the association between intrauterine environmental exposures and genetics, and neurodevelopment and brain health trajectories [8]. DNA methylation (DNAm) on cytosine nucleotides followed by guanine (CpG) across the genome is the most studied epigenetic mechanism, having an important role in regulating gene expression [9]. For example, some authors have suggested that differences in placental methylation patterns are associated with differences in brain development, potentially through mechanisms such as the reprogramming of gene expression involved in the hypothalamic-pituitary-adrenal axis [10].
Previous research has revealed that a child’s cognitive capacity is not just influenced by genetics and that there are a number of other factors associated, such as socioeconomic status [11], the mother’s gestational age [12], the mother’s intelligence [13], family context [14], and, most importantly for this study, prenatal exposure to detrimental environmental factors such as cigarette smoke, organochlorine pollutants, maternal malnutrition, obesity, or stress, among others [15,16,17,18,19]. Thus, it is thought that placental function, and in particular placenta DNAm, could be a plausible mechanism contributing to this prenatal environmental influence on cognitive functions later in life. In fact, neurodevelopmental disorders or traits such as autism spectrum disorder or neurobehavior have been investigated in relation to placenta DNAm [19,20,21].
However, to the best of our knowledge, the association between placenta DNAm and child’s cognitive functions has not been addressed before. Notably, a recent epigenome-wide association study (EWAS) in older-aged adults (N = 6809) reported associations of blood DNAm with global cognitive function at one intergenic CpG site on chromosome 12 and with phonemic verbal fluency at one CpG site on chromosome 10 in the INPP5A gene [22]. Moreover, using cord blood data, the Pregnancy and Childhood Epigenetics (PACE) consortium has recently conducted an epigenome-wide association study for cognitive functioning in childhood (N = 3300) [23]. Overall, they did not find robust evidence that cord blood DNAm at the single CpGs investigated could be associated with cognitive functions, either overall, verbal, or perceptive performance. On the other hand, with a much smaller sample size (N = 112) but different neurodevelopmental outcome, Abrishamcar et al. [24] found that methylation at several CpGs in cord blood was significantly mediating the effect of prenatal tobacco exposure and prenatal alcohol exposure on cognition and motor skills at 6 months of age in a south-african cohort. In the same cohort, but another study, the authors found that methylation at 3 CpGs in cord blood was associated with severe neurodevelopmental delay at 2 years of age [25]. In the context of adverse birth outcomes, Camerota et al. [26] assessed neonatal DNAm from buccal swabs, such as in children born very preterm, and found that methylation at 309 CpGs mediated the association between cumulative prenatal risk and child cognitive ability at 3 years of age. The same authors also explored attention problems in another study and found that DNAm at 33 CpG sites was associated with this outcome at 2 years of age [27]. In line with this, other studies have focused more on behavioural traits instead of cognition. Notable contributions, again within the PACE consortium, include work by Neumann et al. [28], who reported that DNAm at 9 CpGs in cord blood were associated with later ADHD symptoms, and by Rijlaarsdam et al. [29] who found that DNAm at 1 CpG and 1 differentially methylated region (DMR) in child blood was associated with general psychopathology factor at school-age.
Because of all the exposed above, the placenta is probably an appropriate organ for studying the early mechanisms and determinants of cognition. Thus, our goal here is to investigate whether differences in placenta DNAm profile are associated with three different cognitive domains (namely verbal score, perceptive performance score, and general cognitive score) in childhood, accounting for relevant covariates. We also assess the effects of maternal cognitive function, gestational age, and pregnancy complications and adverse birth outcomes on this association. To do this, we conducted epigenome-wide association analyses including data from mother-child pairs from 3 subcohorts within the INMA project (INfancia y Medio Ambiente, acronism of Spanish “Chilhood and Environment) and performed a follow-up functional analysis to help the interpretation of the findings.
Materials/subjects and methods
Study participants
The study population belongs to the INMA project (INfancia y Medio Ambiente-Environment and Childhood), which is a network of birth cohorts in Spain created with the aim to study the role of environmental pollutants in air, water and diet during pregnancy and early childhood in relation to child growth and development [30]. In our study, we used data from participants within the subcohorts of Gipuzkoa, Sabadell and Valencia due to data availability. Of the 2506 mother-child pairs that were followed, a sub-sample of 255 mothers-child pairs with complete data on placenta genome-wide methylation and child’s general cognitive functions at 4 years of age were selected for this study. Mother-child pairs of multiple births or mother-child pairs with a non-European ancestry were excluded. For more details, please see Supplementary Methods.
Placental biopsy, DNA extraction and processing
In INMA, 2506 mother-child pairs were followed until birth and a random selection of 489 placentas were collected. Genomic DNA from placenta was isolated using the DNAeasy® Blood and Tissue Kit, (Qiagen, CA, USA) and stored at −20 °C until further processing. DNAm was assessed with the Infinium MethylationEPIC BeadChip from Illumina, following the manufacturer’s protocol in the Erasmus Medical Centre core facility. For more details on the specific protocols followed, please see Supplementary Methods.
Methylation data acquisition, quality control and normalisation
The methylation data was pre-processed using the PACEAnalysis R package (v.0.1.7) (https.www.epicenteredresearch). The pre-processing pipeline consists of sample quality control, probe quality control, normalisation, batch correction, estimation of cell type proportions, and winsorization of outlier values (the extreme values to the 1% percentile–with 0.5% each at upper and lower ends of the distribution- were winsorized, where percentiles were estimated with the empirical beta-distribution). The final dataset consists of 379 samples (before merging with phenotypic data) and 811,990 probes. DNAm values are expressed as beta values, where 0 means un-methylation and 1 complete methylation. Cell type proportions of six populations (trophoblasts, syncytiotrophoblast, nucleated red blood cell, Hofbauer cells, and endothelial cells) were estimated from DNAm using the placenta reference panel from term placentas implemented in the planet R package [31]. Additional details regarding pre-processing can be found in the supplementary Methods.
Child’s general cognitive functions assessment
A standardised version of the McCarthy Scales of Children’s Functions (MSCA) adapted to the Spanish population by TEA Editions S.A. (official editorial company for adapting tests in Spain) was used to evaluate motor and cognitive functions [32]. In this particular study, we used three out of the five sub-area scales from the original MSCA: verbal scale, perceptive performance scale, and general cognitive scale. The three cognitive continuous scores (verbal, perceptive performance, and general cognitive scores) were standardised across the entire cohort (N = 2150) so that mean = 100 and SD = 15, to facilitate comparison among the different subcohorts and to align with conventional cognitive test scoring [30] (Fig. 1; Table 1). Participants were included if they had replied to all the tests. For more details on this assessment please see Supplementary Methods.
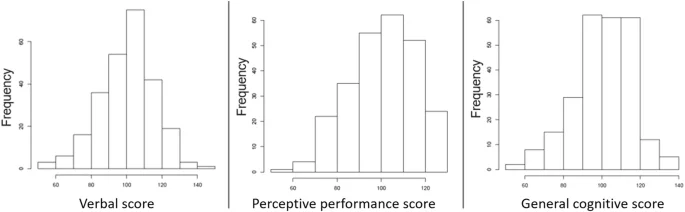
Histograms of the cognitive outcome variables verbal score, perceptive performance score, and general cognitive score.
Statistical analysis
In this study, we conducted EWAS to test the association between the normalised beta-value at each CpG (dependent variable) and the verbal score, perceptive performance score or general cognitive scores in children (independent variable). For this, we conducted multivariable robust linear regression models to account for potential heteroscedasticity and non-normality, using the R package PACEAnalysis (https.www.epicenteredresearch). We have modelled DNAm as the dependent variable to control for extreme values (with high leverage or influential points) that could skew the association [33], particularly in placental tissue where skewed methylation patterns are common [34]. The main models included the following set of covariates selected among a list of potential a priori confounders: subcohort (Gipuzkoa, Valencia or Sabadell); child’s sex (female/male), obtained from clinical records; gestational age, calculated by ultrasounds; maternal age; maternal education (primary or without education /secondary /university); parity (primiparous vs multiparous); child’s age at MSCA assesment; maternal smoking status during pregnancy (non-smoker /non-sustained smoker – quit smoking in early pregnancy, before the end of first trimester-/sustained smoker throughout pregnancy), obtained through questionnaires administered face to face by trained interviewers in first and third trimester of pregnancy; and five cell type proportions (stromal cells, which corresponds to the lowest proportion of cell types, was removed from the models to avoid collinearity issues). Further, the models were adjusted for contamination score which was created by the PACEanalysis R package, as mentioned in the Supplementary Methods [35]. Multiple testing was corrected using the False discovery rate (FDR) through Benjamini–Hochberg (BH) procedure. CpGs with a nominal p < 1 × 10−4 were considered to show suggestive statistically significant association with the cognitive scores and they were analysed in the downstream analysis. The association coefficients were transformed so that they reflect the difference in DNAm level associated with an interquartile range (IQR) increase in cognitive scores.
Several sensitivity analyses were run consisting of: (1) additionally adjusting for maternal cognitive function score (WAIS-III Similarity subtest conducted on the participant mothers when the child was 4 years old); (2) unadjusting for gestational age; and (3) excluding infants that were born preterm (gestational age < 37 weeks), small for gestational age (neonate’s birth weight below the percentile 10 taking into account the reference table of the nearest week of gestation [36]), and/or mother-child pairs that experienced pregnancy complications (preeclampsia, gestational diabetes). A summary of the models and the corresponding sample sizes is available in Supplementary Table 1. Pearson’s correlation and GGally R package were used to compare the resulting estimates between models. The difference in the effect size among main models and alternative models was calculated as (effect size main model − effect size alternative model)/effect size main model × 100. Venn diagrams to visualise the overlap between CpGs identified in the different models were conducted using the webtool https://bioinformatics.psb.ugent.be/webtools/Venn/. We used the Quality Control module from the EASIER R package to perform the quality control of EWAS results, annotate CpGs, and estimate FDRs to account for multiple testing [37].
Differentially methylated regions
DMRs were identified using the dmrff package in R [38]. This method first identifies candidate DMRs by screening the meta-level EWAS results for genomic regions each covered by a sequence of CpG sites with EWAS effects in the same direction, EWAS p < 0.05, and <500 bp gaps between consecutive CpG sites. Then, summary statistics are calculated for each candidate DMR by meta-analysing the EWAS summary statistics of the CpG sites in the region (regions were considered DMRs if containing more than 1 CpG site). Multiple testing was corrected using the FDR.
Biological interpretation
Individual probes and probes included in the DMRs showing FDR significance were looked up in the EWAS catalogue [39] and EWAS atlas [40] to examine potential associations with exposures and health outcomes based on existing studies. To further characterise potential genetic influences on these sites, we used the placenta mQTL lists available from Delahaye et al. [41], the fetal brain mQTL list from Hannon et al. [42] (http://epigenetics.essex.ac.uk/mQTL/), the brain mQTL resource from the Brain xQTL Serve (https://mostafavilab.stat.ubc.ca/xqtl/) [43], and the whole blood mQTL list from GoDMC (http://mqtldb.godmc.org.uk/) [44]. To assess whether methylation levels of CpGs were associated with the expression levels of nearby genes, we consulted the placenta Expression Quantitative Trait Methylation (eQTM) lists available from Delahaye et al. [41], and Deyssenroth et al. [45], and the blood eQTM list from the eQTM HELIX catalogue (https://helixomics.isglobal.org/), generated from childhood blood samples.
Then, we did several functional enrichment analyses with the suggestive CpGs (p < 1 × 10−4), using the Functional Enrichment module of the EASIER R package [37]. The University of California-Santa Cruz (UCSC) genome browser was used to further explore the genomic context of the FDR-significant CpGs and DMRs. For more details on the functional enrichment analyses performed, please see Supplementary Methods.
Finally, we also explored whether genomic regions of the identified DMRs overlap with the 242, 226, and 1271 genome-wide significant loci previously reported in GWAS on intelligence [46], cognitive performance, and educational attainment [47] (0.5 Mb window centred to the genomic 250 locus indicated in the original studies).
Results
Study descriptives
Our study includes 255 mother-child pairs from three subcohorts of the INMA project. Among the participant 255 mother-child pairs, 42 were from INMA Valencia, 111 from INMA Sabadell and 102 from INMA Gipuzkoa subcohorts. Table 1 shows the main sociodemographic characteristics, reproductive/birth and cognitive scores of the included mother-child pairs. Around half of the children were females and half were males (49.4% and 50.6%), and all were of European ancestry. For half of the mothers, it was their first pregnancy (56.86%), and the majority of them had a secondary education or superior. Almost 75% of the mothers did not smoke throughout the whole pregnancy and 14.12% smoked only during the first trimester. Mean age of the mother at the delivery date was 31.34 years (SD = 3.88) and the mean gestational age was 39.72 weeks (SD = 1.31). Average cell type proportions were similar to those reported by previous authors [48].
The child’s cognitive scores were assessed through the MSCA test at 4 years of age (mean = 4.68, SD = 0.49). The mean of the main study variables (verbal score, perceptive performance score, and general cognitive score) is close to 100 because they were standardised in the whole cohort population (N = 2150) to facilitate comparison among the different subcohorts [30] (Fig. 1; Table 1). Verbal and perceptive performance score weakly correlated with each other (r = 0.366), whereas general cognitive score strongly correlated with both verbal (r = 0.848) and perceptive performance score (r = 0.769) (Fig. 2). This is not unexpected since general cognitive score is calculated using the cognitive tasks included in both the verbal score and the perceptive performance score, while verbal score and perceptive performance score do not share any MSCA cognitive subtest.
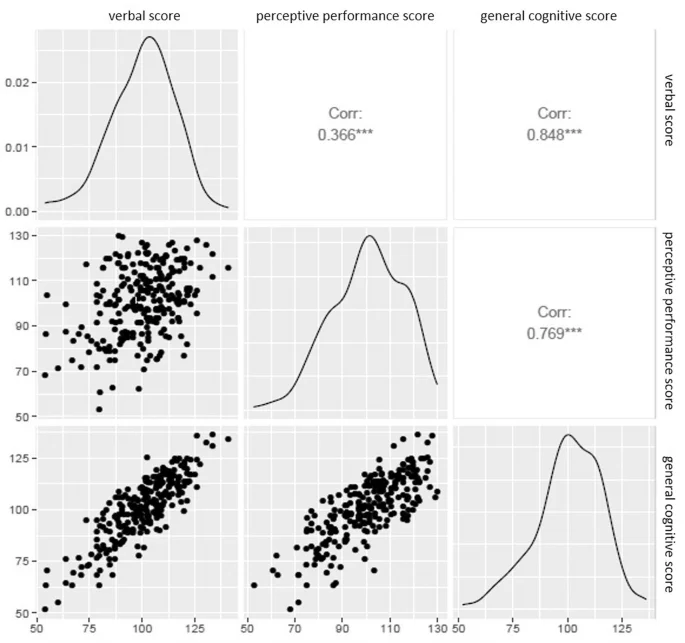
Correlation between the verbal score, perceptive performance score, and general cognitive score.
Epigenome-wide association studies
We run three separate EWAS, one for each cognitive domain, adjusting for covariates. We did not observe any relevant genomic inflation in the main three models: lambdas were 0.89, 0.97, and 0.92 for general cognitive score, verbal score, and perceptive performance score models, respectively. QQplots are shown in Supplementary Fig. 1. The analysis assessing the association between placenta DNAm and general cognitive score revealed 4 significant CpGs after correcting for FDR (p < 7.0611 × 10−8): cg00866476, which was negatively associated with the general cognitive score, and cg15480200, cg02986379, and cg14113931, which were positively associated with the general cognitive score (Fig. 3). Cg1548200 is annotated to an open sea region within chromosome 10, cg02986379 to a south shore region annotated to the DAB2 gene in chromosome 5, cg00866476 to a north shore region not annotated to any gene in chromosome 2, and cg14113931 to a north shore region annotated to CEP76 and PSMG2 genes in chromosome 18 (Table 2). When running the analysis separately by subcohort, we observed a consistent effect in all subcohorts (see Supplementary Fig. 2 for forest plots), showing heterogeneity (i2) values ranging from 0 to 0.2 and p > 0.05. None of the probes reached statistical significance in the analysis assessing the other two cognitive domains.
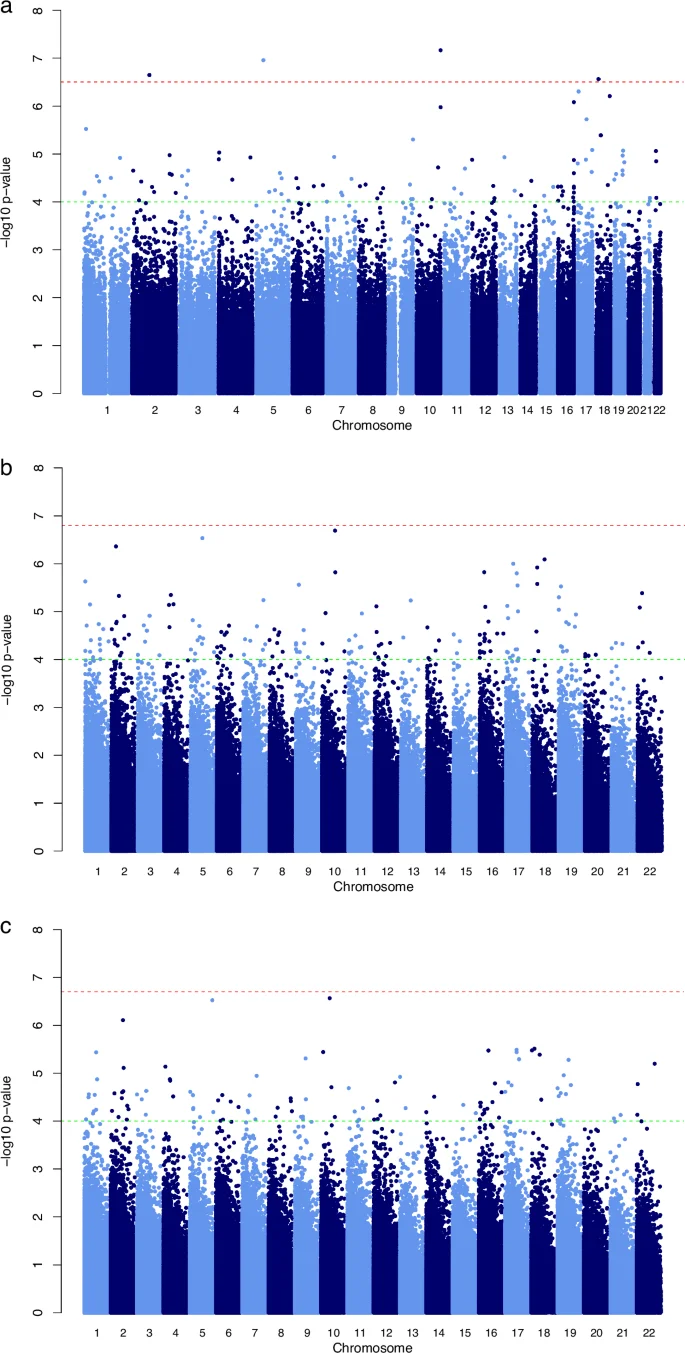
a general cognitive score; b verbal score; and c perceptive performance score. Each dot represents one of the 708,105 CpGs. The y axis represents the negative logarithm of the association p values, and the x axis shows the genomic coordinates of the CpGs. The red abline indicates the FDR significance, while green abline indicates a suggestive cutoff of p < 1 × 10–4.
When exploring results at a suggestive significance threshold of p < 1 × 10−4, methylation at 103, 172, and 112 CpGs was associated with the general cognitive, the verbal, and the perceptive performance score scores, respectively (Fig. 3). See the annotated results of all probes that reached a significance of p < 1 × 10−4 or each of the main models (Supplementary Table 2). Complete results are available at Zenodo platform (https://zenodo.org/records/13379121).
When comparing all the CpGs that where significant at a suggestive p-value (p < 1 × 10−4) in the three main models, we observed that of the 172 suggestive CpGs for verbal score, 33 were common with the suggestive CpGs for general cognition. Of the 112 suggestive CpGs for perceptive performance score, 22 were common with CpGs for the general cognitive score. None of the suggestive CpGs were shared between models assessing the perceptive performance score and the verbal score. A Venn diagram summarising these findings is shown in Supplementary Fig. 3.
Results of the sensitivity analyses without adjusting for gestational age indicate that gestational age is not significantly affecting our main findings, which is consistent with the fact that gestational age is not associated with any of the 3 cognitive domains in our dataset (p > 0.05) (Lambdas ranging from 0.89 to 0.97) (summary statistics are shown in Supplementary Table 3, correlation between models is shown in Supplementary Fig. 4). Results of the sensitivity analyses with additional adjusting for maternal cognitive function indicate that the main findings are not influenced by the cognitive function of the mother (Lambdas ranging from 0.88 to 0.97) (descriptives are shown in Supplementary Table 4, correlation between models is shown in Supplementary Fig. 4, summary statitics are shown in Supplementary Table 5). Finally, results of the sensitivity analyses excluding pregnancy complications and adverse birth outcomes indicate that the main findings are not driven by the subset of mother-child pairs that experienced pregnancy complications and adverse birth outcomes (Lambdas ranging from 1.0 to 1.1) (descriptives are shown in Supplementary Table 6, correlation between models is shown in Supplementary Fig. 5, summary statitics are shown in Supplementary Table 7). Hence, when looking at the FDR-significant CpGs for the general cognitive score in the main model, we found that direction and magnitude of the effect was rather consistent between main and all the sensitivity models (with % change ranging from 0.1 to 10.18%).
Differentially methylated regions analysis
In the DMRs analysis, two DMRs containing 5 and 2 CpGs were associated with general cognitive score, two DMRs containing 7 and 3 CpGs were associated with perceptive performance score, and two other DMRs containing 8 and 2 CpGs were associated with verbal score (Table 3). One of the DMRs (chr18:71879836–71879850) was associated with both verbal and general cognitive scores. Notably, none of the DMRs included the CpGs that were significantly associated with the general cognitive score after FDR correction. As shown in Table 3 and Supplementary Fig. 6, DMR on chromosome 3 (chr3:168864101–168864417) and DMR on chromosome 6 (chr6:31803111–31803199), both associated with perceptive performance score, were annotated to a CpG island and a south shore within the MDS1 and EVI1 Complex Locus (MECOM) and Small Nucleolar RNA Host Gene 32 (SNHG32/C6orf48) genes, respectively; DMR on chromosome 19 (chr19:9785727–9786077), associated with verbal score, was annotated to a CpG island and a south shore within the Zinc Finger Protein 562 (ZNF562) gene. Moreover, according to ENCODE data on several cell lines, including different blood and embryonic stem cell types, these three DMRs present H3K27Ac histone marks and overlap with DNAse hypersensitive areas, which are usually associated to active regulatory elements. DMRs on chr6 and 19 overlap with active chromatin states (active promoters). In contrast, DMR on chr3 overlaps with polycomb-repressed states. The rest of DMRs were annotated to open sea regions in the genome.
Follow-up analysis
We conducted a set of downstream analysis aimed to facilitate biological interpretation of the main findings. First, we searched the FDR-significant CpGs of the general cognitive score single-probe analysis and CpGs located within the FDR-significant DMRs in the EWAS catalogue and EWAS atlas (see Supplementary Table 8a). According to these databases, methylation at 3 of the 4 CpGs associated with the general cognitive score have been previously associated with gestational age (in cord blood and fetal brain), age (in blood), exposure to environmental factors (smoking, high maternal plasma glucose, in blood), or associated to traits or health conditions (preterm birth, eosinophilia, in blood). Regarding the CpGs included in the DMRs (27 CpGs), several of them have been associated with age in blood (only one with gestational age), and traits or health conditions (rheumatoid arthritis, B acute lymphoblastic leukaemia, type 2 diabetes—in blood-, or renal, colorectal or oral cancer—in tumoral tissues) (Supplementary Table 8b).
Of the top FDR-significant CpGs, cg14113931, corresponds to a brain and blood methylation quantitative trailt locus (mQTL), and cg00866476 to a blood mQTL (Supplementary Table 8a). Notably, none of these 4 top CpGs were identified as FDR-significant in previous studies exploring the association between DNAm in cord blood or whole blood and cognitive functions in childhood or adulthood [22,23,24,25,26]. To further explore these tissue differences, we run the same analyses with cord blood DNAm data in the INMA cohort individuals that have methylation data on both placenta and cord blood (n = 148). They showed very different effect directions/sizes across the two tissues. Hence, methylation level means were also very different across the two tissues for cg15480200 and cg14113931, which were hemymethylated in placenta (Supplementary Table 9).
Of the FDR-significant DMRs CpGs, cg07751698 and cg22034155, associated with perceptive performance, correspond to fetal brain mQTLs (and a blood mQTL in the case of the latter). Four CpGs from the same DMR associated with general cognitive score (cg02712303, cg23190366, cg09433114, cg05720891), correspond to 4 blood mQTLs, one of them also being a blood eQTL regulating Brain-specific angiogenesis inhibitor 1-associated protein 2 –BAIAP2-AS1- gene expression (cg02712303), and another (cg23190366) also being a brain mQTL. Finally, regarding the longest DMR associated with verbal score, 3 out of the 8 included CpGs (cg24874111, cg20631204, and cg14073063) correspond to fetal brain mQTLs (cg24874111 and cg14073063 also being blood eQTLS regulating Zinc Finger Protein 562 –ZNF562- gene expression), while another CpG (cg17704570) corresponds to a blood mQTL (Supplementary Table 8b).
We also explored whether genomic regions of the FDR-significant hits overlapped with the 242, 226, and 1271 genome-wide significant loci previously reported in GWAS of intelligence [46], cognitive performance, and educational attainment [47] (0.5 Mb window centred to the CpG position). We found that the genomic regions of CpGs cg15480200 and cg14113931 include SNPs previously associated with educational attainment (rs12765185, located on genomic coordinate chr10: 134977077, and rs8097125, located on genomic coordinate chr18: 13005473, respectively [47]).
Hence, the genomic regions of the two DMRs associated with perceptive performance score include SNPs previously associated with educational attainment (rs13099165, located on genomic coordinate chr3: 168740425; rs9267658, located on genomic coordinate chr6: 31845985; rs9267677, located on genomic coordinate chr6: 31892641 [47]) and with cognitive performance (rs707938, located on genomic coordinate chr6: 31729359 [47]); and the genomic region of the previously mentioned DMR associated with verbal score includes SNPs previously associated with educational attainment (rs2287838, located on genomic coordinate chr19: 9959014 [47]).
The gene-set enrichment analysis of the suggestive CpGs (p < 1 × 10−4) conducted with Missmethyl did not show any significant results after multiple-testing correction (Supplementary Table 10; Supplementary Table 11). However, within the top biological pathways and processes there were some related with the formation of the nervous system or with the development of the fetus, as for example, the ventral spinal cord formation or the Notch pathway, and others related to the immune system, reparation, or biosynthesis. The enrichment analysis using the Consensus Path tool revealed an enrichment of 19, 9, and 5 gene-sets significant at FDR (p < 0.05) for the general cognitive score, verbal score, and perceptive performance score, respectively (Supplementary Table 12). The most recurrent gene-sets were related to Hedgehog and Notch signalling pathways, both involved in placenta development, fetus formation, and brain growth [49,50,51].
Finally, we checked whether the suggestive CpGs were over-represented in the placenta germline DMRs (gDMRs) (regions of the genome that show differential methylation according to the parental origin of the genome, associated with the expression of imprinted genes, which are expressed in a parent-of-origin-specific manner) [52], or in placenta partially methylated domains (PMDs) (large regions that cover ~37% of the human genome, which are partially methylated and contain placenta-specific repressed genes) [53] (Supplementary Table 13). Notably, there was a highly significant enrichment of suggestive CpGs of the general cognitive score in both gDMRs and PMDs, while there was not an enrichment of suggestive CpGs from the verbal score and perceptive performance score scores in these regions.
Discussion
In this study, we conducted, to the best of our knowledge, the first epigenome-wide-analysis investigating whether placenta DNAm profile is associated with childhood cognitive functions measured in three main domains of the McCarthy Scales, such as verbal score, perceptive performance score, and general cognitive score, at the age of 4 years. Moreover, we adjusted the models for confounders and explored the effect of maternal cognitive function, gestational age, pregnancy complications, and adverse birth outcomes in this association. The main analysis, at a single-probe level, did not identify significant associations between placenta DNAm and perceptive performance score or verbal score. However, we did find some evidence of FDR-corrected significant association between DNAm and the general cognitive score in 4 CpGs. Overall, the magnitude of the beta coefficients of the top CpGs associated with the general cognitive score is rather small (the difference in DNAm level % associated with an IQR increase in cognitive scores ranges from −0.8 to 1), and therefore, its functional implications remain unclear. Nonetheless, there are examples in the literature (for example, in blood samples) where small effects persist and have been replicated across populations and across time, as summarised by Breton and Marsit [54]. These small effect sizes, while challenging to interpret in isolation, may collectively contribute to significant biological and clinical outcomes. Another explanation for these small changes could be that DNAm is altered only in a specific cell type of the placenta, and this effect is diluted when analysing the bulk tissue.
Interestingly, one of the FDR-significant CpGs, cg02986379, is located in the promoter region of the DAB2 gene, which encodes a clathrin and cargo binding endocytic adaptor protein, playing a role in cellular trafficking of a number of transmembrane receptors and signalling proteins, cell differentiation, and proliferation. This gene is highly expressed in the placenta, where it seems to play a role in placenta growth and development [55], and its compromised abundance is associated with fetal growth restriction and low birth weight pathology. In the nervous system, DAB2 seems to have a role in neuronal development and nerve growth factor-mediated neurite outgrowth [56, 57]. Similarly, another of the FDR-significant CpGs, cg14113931, is located in the body and promoter of CEP76 and PSMG2 genes, respectively. It has not been described a particular role for CEP76 gene in the placenta, but this gene encodes an important centrosomal protein that regulates cell cycle and mitotic progression [58, 59]. PSMG2 encodes an adaptor protein involved in chaperone-mediated protein complex assembly, with no specific function in placenta, but associated with cognitive impairment and Alzheimer’s disease [60]. The other 2 top CpGs indentified, cg15480200 and cg00866476, are annotated to genomic open sea regions. Notably, none of these 4 top CpGs were identified as FDR-significant in previous studies exploring the association between DNAm in cord blood or whole blood and cognitive functions in childhood or adulthood [22,23,24,25,26]. This discrepancy in findings could be attributed to differences in tissue type (supported by the very different effect directions/sizes and methylation level means across cord blood and placenta DNAm at these CpGs in the same individuals from the INMA cohort), as well as other factors such as study design, population characteristics, or sample variability. Interestingly, genomic regions of 2 of these 4 CpGs include SNPs previously associated with educational attainment, a trait highly associated with intelligence test scores [61]. According to EWAS atlas and EWAS catalogue, 3 of these 4 top CpGs (cg15480200, cg00866476, and cg14113931) have been associated with gestational age and preterm birth in whole blood or cord blood studies [62,63,64]. Since preterm birth and reduced gestational age are associated with cognitive functions later in life, this may explain an association between DNAm at these particular sites and child’s cognition if mediated by adverse birth outcomes such as preterm birth. However, this is not supported by the high correlation between the main models and models unadjusted by gestational age, or models excluding pregnancy complications and adverse birth outcomes (preterm). It might be that gestational age affects this association at earlier stages than 4 years of age, and further investigation is required.
Similarly, maternal heritability did not seem to influence our results, given that our models adjusted by maternal cognitive function showed a high correlation with the main models. On the other hand, 3 of these 4 CpGs (cg15480200, cg00866476, cg14113931) were associated with blood mQTLs according to GoDMC database [44], and cg14113931 corresponds to a brain mQTL according to Brain xQTL Serve resource [43]. None of the CpGs correspond to previously reported placenta mQTLs. However, mQTL studies in the placenta are scarce and smaller than in the other tissues, so it is unclear whether genetics might be, at least partially, underlying these associations.
When comparing all the suggestive CpGs in the three main models, we observed that a small proportion were common between general cognitive score and verbal score, a slightly smaller proportion were common between cognitive general score and perceptive performance score, and none of the suggestive CpGs were shared between models assessing the perceptive performance score and verbal score scores. This is not unexpected since the general cognitive score is calculated using the cognitive tasks included in both the verbal score and the perceptive performance score, while the verbal score and perceptive performance score do not share any MSCA cognitive subtests. Actually, verbal and perceptive performance scores are weakly correlated with each other, and general cognitive scores are strongly correlated with both verbal and perceptive performance scores. The fact that the general cognitive score includes more tasks than the other two cognitive domains could be underlying the more significant results identified in this model compared to the other 2 for the single-probe analyses.
Functional enrichment of the suggestive CpGs in the three main models revealed gene sets involved in placenta development, fetus formation, and brain growth, such as Hedgehog and Notch pathways [49,50,51]. Epigenetic changes in these pathways might be associated with changes in placental function, fetal development, and specifically brain development, having a potential impact on later-in-life cognitive functions. Finally, the significant enrichment of suggestive CpGs from the general cognitive score model in both PMDs and gDMRs regions, suggests a specific regulatory role of these CpGs on placenta genes that are usually repressed in this tissue [53], and a potential involvement of these CpGs in genomic imprinting [65]. In fact, imprinted genes are strongly over-represented in the placenta, and it has been stated that changes in their expression in this tissue may also have consequences for brain in both the offspring and the mother, possibly through an imbalance in nutrients supply/demand or by alterations in the endocrine signalling from the placenta to the mother [66, 67]. However, the functional implications of this need further exploration, and functional and genomic enrichment analysis have to be interpreted with caution given the relaxed suggestive p-value used in these particular analyses (p < 1 × 10−4).
When exploring the association between child cognitive functions and DMRs in the placenta, we found 2 DMRs negatively associated with general cognitive score, 2 DMRs negatively associated with perceptive performance score, and 2 DMRs negatively associated with verbal score. Interestingly, three of them are annotated to regulatory regions of the following genes: MECOM, C6orf48/SNHG32, and ZNF562. First, MECOM or EVI1 encodes a zinc finger transcription factor, expressed in the placenta among other tissues, known to be involved in hematopoiesis, cell cycle regulation, differentiation, proliferation, and embryogenesis [68, 69]. This gene is also known as a negative regulator of NF-κB-dependent inflammation [70]. Neurodevelopmental disorders such as ASDs or cognitive impairment are associated with inflammation during development [71, 72]. This can be, in part, caused by a deregulation of the maternal-fetal immune environment [73, 74]. Infections during pregnancy are one of the main triggers of maternal–fetal immune dysregulation [73, 74]. In line with this, methylation levels at three CpGs included in this DMR (cg07751698, cg06238409, and cg13147822) were previously associated with the presence of several microorganisms in the placenta [75]. Second, ZNF562 is involved in transcription regulation and, according to the STRING webtool [76], appears to interact with SMARCAD1 and TRIM28, both of which are involved in the regulation of the inflammatory response [77, 78]. Interestingly, two CpGs included in this DMR are reported fetal brain mQTLs and eQTMs in childhood blood that regulate ZNF562 gene expression. However, few data have been published to date regarding this gene, especially in relation to placenta or fetal development. Third, the non-coding small nucleolar RNA C6orf48/SNHG32, of unknown function, is downregulated in cytotrophoblasts from severe preeclampsia cases [79]. Interestingly, a DMR within this gene in the placenta was associated with following a vegetarian diet before pregnancy [80]. Moreover, eight CpGs within this region in the placenta were previously associated with fetal growth restriction in mothers with insufficient gestational weight gain [81]. Overall, the fact that these DMRs overlap with regulatory elements of the associated genes supports the potential functional relevance of these regions, especially regarding the 2 DMRs associated with inflammation-related genes. Hence, similar to what was found in the single-CpG analysis, genomic regions of 3 of these DMRs include SNP’s previously associated with cognitive performance and with educational attainment (which is highly associated with intelligence test scores [61]). Finally, even though the DMR in chromosome 17 associated with general cognitive score is located in an open sea region, it includes 4 CpGs reported as mQTLs in blood, 1 of which is also a reported brain mQTL, and another an eQTM in child blood that regulates BAIAP2-AS1 gene expression, a gene that is altered in the brain of schizophrenia patients [82].
The current findings should be interpreted in the context of several limitations. The reduced sample size warrants future genome-wide studies with larger sample sizes to replicate and extend our findings, and to explore sex and cell type influences on the assessed associations. Since this study is based on a European population sample, this should be replicated in other ancestries and in more diverse settings. Additionally, eventhough we used a genome-wide array to measure DNAm, this only accounts for only 2–3% of the CpG sites in the genome. We could only compare our results with the other two EWAS on cognitive functions performed in cord blood and whole blood (rather than placenta) because of the novelty of the study. Finally, although our findings are based on placenta tissue, we cannot discard the fact that the methylation status of these marks in the placenta could also reflect the same status in other target tissues, such as the fetal brain, which are, similarly, or even more relevant for this association. In line with this, we detected that 6 of the CpGs included in the FDR-significant DMRs correspond to mQTLs of adult or fetal brains [42, 43].
Strengths of this study include investigating the methylome in relation to future cognitive functions in a crucial tissue for embryonic fetal development, and in particular for brain development, such as the placenta; and the use of detailed information on factors that influence neurodevelopment to adjust the models and to perform sensitivity analyses. Moreover, to the best of our knowledge, this is the first time that a study on placenta has attempted to establish a link between DNAm and childhood cognitive function, which may provide new avenues for other researchers to investigate this topic in the future.
In summary, we found some evidence of placenta DNAm being associated with childhood cognitive functions. This association was not affected by maternal cognitive function, gestational age, pregnancy complications, and adverse birth outcomes in our cohort. Top CpGs and DMRs are mapped to genes involved in placenta fetal and brain development, and inflammation. Moreover, some are located close to loci of cognitive performance and educational attainment. These findings suggest that placental DNAm could be a mechanism contributing to the alteration of important pathways in the placenta that have a consequence on the offspring’s brain development and cognitive function. However, the limitations of the study, such as the reduced sample size, call for further research in other cohorts (including other ancestries, social contexts, sex-stratified analyses, etc), and in vivo and in vitro experiments to achieve a more comprehensive understanding of this association and its biological implications.
Data availability
Complete results are available at Zenodo platform (https://zenodo.org/records/13379121).
References
-
Spear LP. The adolescent brain and age-related behavioral manifestations. Neurosci Biobehav Rev 2000;24:417–63.
-
Stiles J, Jernigan TL. The basics of brain development. Neuropsychol Rev 2010;20:327.
Google Scholar
-
Hodyl NA, Aboustate N, Bianco-Miotto T, Roberts CT, Clifton VL, Stark MJ. Child neurodevelopmental outcomes following preterm and term birth: What can the placenta tell us? Placenta. 2017;57:79–86.
Google Scholar
-
Rosenfeld CS. The placenta-brain-axis. J Neurosci Res 2021;99:271–83.
Google Scholar
-
Kratimenos P, Penn AA. Placental programming of neuropsychiatric disease. Pediatr Res 2019;86:157–64.
-
Misra DP, Salafia CM, Charles AK, Miller RK. Placental measurements associated with intelligence quotient at age 7 years. J Dev Orig Health Dis 2012;3:190–7.
Google Scholar
-
Rosenfeld CS. Placental serotonin signaling, pregnancy outcomes, and regulation of fetal brain development. Biol Reprod 2020;102:532–8.
Google Scholar
-
Lapehn S, Paquette AG. The placental epigenome as a molecular link between prenatal exposures and fetal health outcomes through the DOHaD hypothesis. Curr Environ Health Rep. 2022;9:490–501.
Google Scholar
-
Jones PA. Functions of DNA methylation: islands, start sites, gene bodies and beyond. Nat Rev Genet. 2012;13:484–92.
Google Scholar
-
Meakin CJ, Martin EM, Santos HP, Mokrova I, Kuban K, O’Shea TM, et al. Placental CpG methylation of HPA-axis genes is associated with cognitive impairment at age 10 among children born extremely preterm. Horm Behav 2018;101:29–35.
Google Scholar
-
Hackman DA, Farah MJ, Meaney MJ. Socioeconomic status and the brain: mechanistic insights from human and animal research. Nat Rev Neurosci 2010;11:651–9.
Google Scholar
-
Yang S, Platt RW, Kramer MS. Original contribution variation in child cognitive ability by week of gestation among healthy term births. Am J Epidemiol. 2010;171:399–06.
-
Rahu K, Rahu M, Pullmann H, Allik J. Effect of birth weight, maternal education and prenatal smoking on offspring intelligence at school age. Early Hum Dev. 2010;86:493–97.
-
Barreto FB, Sánchez de Miguel M, Ibarluzea J, Andiarena A, Arranz E. Family context and cognitive development in early childhood: a longitudinal study. Intelligence. 2017;65:11–22.
Google Scholar
-
Kristjansson AL, Thomas S, Lilly CL, Thorisdottir IE, Allegrante JP, Sigfusdottir ID. Maternal smoking during pregnancy and academic achievement of offspring over time: A registry data-based cohort study. Prev Med. 2018;113:74.
Google Scholar
-
De Rooij SR, Wouters H, Yonker JE, Painter RC, Roseboom TJ. Prenatal undernutrition and cognitive function in late adulthood. Proc Natl Acad Sci USA. 2010;107:16881–6.
Google Scholar
-
Richetto J, Riva MA. Prenatal maternal factors in the development of cognitive impairments in the offspring. J Reprod Immunol 2014;104-105:20–5.
Google Scholar
-
Vermeir G, Covaci A, Van Larebeke N, Schoeters G, Nelen V, Koppen G, et al. Neurobehavioural and cognitive effects of prenatal exposure to organochlorine compounds in three year old children. BMC Pediatr 2021;21:1–15.
Google Scholar
-
Oken E, Thompson JW, Rifas-Shiman SL, Vilchuk K, Bogdanovich N, Hameza M, et al. Analysis of maternal prenatal weight and offspring cognition and behavior: results from the Promotion of Breastfeeding Intervention Trial (PROBIT) cohort. JAMA Netw Open. 2021;4:e2121429.
Google Scholar
-
Zhu Y, Gomez JA, Laufer BI, Mordaunt CE, Mouat JS, Soto DC, et al. Placental methylome reveals a 22q13.33 brain regulatory gene locus associated with autism. Genome Biol. 2022;23:46.
-
Bakulski KM, Dou JF, Feinberg JI, Aung MT, Ladd-Acosta C, Volk HE, et al. Autism-associated DNA methylation at birth from multiple tissues is enriched for autism genes in the early autism risk longitudinal investigation. Front Mol Neurosci 2021;14:288.
Google Scholar
-
Marioni RE, McRae AF, Bressler J, Colicino E, Hannon E, Li S, et al. Meta-analysis of epigenome-wide association studies of cognitive abilities. Mol Psychiatry. 2018;23:2133–44.
Google Scholar
-
Caramaschi D, Neumann A, Cardenas A, Tindula G, Alemany S, Zillich L, et al. Meta-analysis of epigenome-wide associations between DNA methylation at birth and childhood cognitive skills. Mol Psychiatry. 2022;27:2126–35.
Google Scholar
-
Abrishamcar S, Chen J, Feil D, Kilanowski A, Koen N, Vanker A, et al. DNA methylation as a potential mediator of the association between prenatal tobacco and alcohol exposure and child neurodevelopment in a South African birth cohort. Transl Psychiatry. 2022;12:418.
Google Scholar
-
Hüls A, Wedderburn CJ, Groenewold NA, Gladish N, Jones MJ, Koen N, et al. Newborn differential DNA methylation and subcortical brain volumes as early signs of severe neurodevelopmental delay in a South African Birth Cohort Study. World J Biol Psychiatry. 2022;23:601–12.
Google Scholar
-
Camerota M, Lester BM, McGowan EC, Carter BS, Check J, Dansereau LM, et al. Contributions of prenatal risk factors and neonatal epigenetics to cognitive outcome in children born very preterm. Dev Psychol. 2024;60:1606–19.
-
Camerota M, Lester BM, Castellanos FX, Carter BS, Check J, Helderman J, et al. Epigenome-wide association study identifies neonatal DNA methylation associated with two-year attention problems in children born very preterm. Transl Psychiatry. 2024;14:126.
Google Scholar
-
Neumann A, Walton E, Alemany S, Cecil C, González JR, Jima DD, et al. Association between DNA methylation and ADHD symptoms from birth to school age: a prospective meta-analysis. Transl Psychiatry. 2020;10:398.
Google Scholar
-
Rijlaarsdam J, Cosin-Tomas M, Schellhas L, Abrishamcar S, Malmberg A, Neumann A, et al. DNA methylation and general psychopathology in childhood: an epigenome-wide meta-analysis from the PACE consortium. Mol Psychiatry. 2023;28:1128–36.
Google Scholar
-
Guxens M, Ballester F, Espada M, Fernández MF, Grimalt JO, Ibarluzea J, et al. Cohort profile: the INMA—INfancia y medio ambiente—(environment and childhood) project. Int J Epidemiol 2012;41:930–40.
Google Scholar
-
Yuan V, Hui D, Yin Y, Peñaherrera MS, Beristain AG, Robinson WP. Cell-specific characterization of the placental methylome. BMC Genomics. 2021;22:1–20.
Google Scholar
-
McCarthy D, Cordero Pando A. Escalas McCarthy de aptitudes y psicomotricidad para niños. Pearson; 2011.
-
Rousseeuw PJ, Leroy AM. Robust regression and outlier detection. John Wiley & Sons; 2005.
-
Yuen RKC, Avila L, Peñaherrera MS, von Dadelszen P, Lefebvre L, Kobor MS, et al. Human placental-specific epipolymorphism and its association with adverse pregnancy outcomes. PLoS ONE. 2009;4:e7389.
Google Scholar
-
Heiss JA, Just AC. Identifying mislabeled and contaminated DNA methylation microarray data: an extended quality control toolset with examples from GEO. Clin Epigenetics. 2018;10:73.
Google Scholar
-
Practice Bulletin No. 134: fetal growth restriction. Obstet Gynecol 2013;121:1122.
Google Scholar
-
isglobal-brge/EASIER: EwAS: quality control, meta-analysIs, and EnRichment version 0.1.2.8 from GitHub. https://rdrr.io/github/isglobal-brge/EASIER/. Accessed 30 May 2022.
-
Suderman M, Staley JR, French R, Arathimos R, Simpkin A, Tilling K. dmrff: identifying differentially methylated regions efficiently with power and control. bioRxiv 2018: https://www.biorxiv.org/content/10.1101/508556v1.
-
Battram T, Yousefi P, Crawford G, Prince C, Sheikhali Babaei M, Sharp G, et al. The EWAS Catalog: a database of epigenome-wide association studies. Wellcome Open Res. 2022;7:41.
Google Scholar
-
Li M, Zou D, Li Z, Gao R, Sang J, Zhang Y, et al. EWAS Atlas: a curated knowledgebase of epigenome-wide association studies. Nucleic Acids Res. 2019;47:D983–D988.
Google Scholar
-
Delahaye F, Do C, Kong Y, Ashkar R, Salas M, Tycko B, et al. Genetic variants influence on the placenta regulatory landscape. PLoS Genet. 2018;14:e1007785.
Google Scholar
-
Hannon E, Spiers H, Viana J, Pidsley R, Burrage J, Murphy TM, et al. Methylation QTLs in the developing brain and their enrichment in schizophrenia risk loci. Nat Neurosci 2016;19:48–54.
Google Scholar
-
Ng B, White CC, Klein H-U, Sieberts SK, McCabe C, Patrick E, et al. An xQTL map integrates the genetic architecture of the human brain’s transcriptome and epigenome. Nat Neurosci 2017;20:1418–26.
Google Scholar
-
Min JL, Hemani G, Hannon E, Dekkers KF, Castillo-Fernandez J, Luijk R, et al. Genomic and phenotypic insights from an atlas of genetic effects on DNA methylation. Nat Genet. 2021;53:1311–21.
Google Scholar
-
Deyssenroth MA, Marsit CJ, Chen J, Lambertini L. In-depth characterization of the placental imprintome reveals novel differentially methylated regions across birth weight categories. Epigenetics. 2020;15:47–60.
Google Scholar
-
Savage JE, Jansen PR, Stringer S, Watanabe K, Bryois J, de Leeuw CA, et al. Genome-wide association meta-analysis in 269,867 individuals identifies new genetic and functional links to intelligence. Nat Genet. 2018;50:912–9.
Google Scholar
-
Lee JJ, Wedow R, Okbay A, Kong E, Maghzian O, Zacher M, et al. Gene discovery and polygenic prediction from a genome-wide association study of educational attainment in 1.1 million individuals. Nat Genet. 2018;50:1112–21.
Google Scholar
-
Dieckmann L, Cruceanu C, Lahti-Pulkkinen M, Lahti J, Kvist T, Laivuori H, et al. Reliability of a novel approach for reference-based cell type estimation in human placental DNA methylation studies. Cell Mol Life Sci. 2022;79:115.
Google Scholar
-
Yang C, Qi Y, Sun Z. The role of sonic hedgehog pathway in the development of the central nervous system and aging-related neurodegenerative diseases. Front Mol Biosci 2021;8:605.
Google Scholar
-
Haider S, Pollheimer J, Knöfler M. Notch signalling in placental development and gestational diseases. Placenta. 2017;56:65–72.
Google Scholar
-
Takai H, Kondoh E, Mogami H, Kawasaki K, Chigusa Y, Sato M, et al. Placental sonic hedgehog pathway regulates fetal growth via the IGF axis in preeclampsia. J Clin Endocrinol Metab 2019;104:4239–52.
Google Scholar
-
Hamada H, Okae H, Toh H, Chiba H, Hiura H, Shirane K, et al. Allele-specific methylome and transcriptome analysis reveals widespread imprinting in the human placenta. Am J Hum Genet. 2016;99:1045–58.
Google Scholar
-
Schroeder DI, Blair JD, Lott P, Yu HOK, Hong D, Crary F, et al. The human placenta methylome. Proc Natl Acad Sci USA. 2013;110:6037–42.
Google Scholar
-
Breton CV, Marsit CJ, Faustman E, Nadeau K, Goodrich JM, Dolinoy DC, et al. Small-magnitude effect sizes in epigenetic end points are important in children’s environmental health studies: the Children’s environmental health and disease prevention research Center’s Epigenetics Working Group. Environ Health Perspect 2017;125:511–26.
Google Scholar
-
Lybbert J, Gullingsrud J, Chesnokov O, Turyakira E, Dhorda M, Guerin PJ, et al. Abundance of megalin and Dab2 is reduced in syncytiotrophoblast during placental malaria, which may contribute to low birth weight. Sci Rep. 2016;6:24508.
-
Cheung KK, Mok SC, Rezaie P, Chan WY. Dynamic expression of Dab2 in the mouse embryonic central nervous system. BMC Dev Biol. 2008;8:76.
-
Huang CH, Cheng JC, Chen JC, Tseng CP. Evaluation of the role of disabled-2 in nerve growth factor-mediated neurite outgrowth and cellular signalling. Cell Signal. 2007;19:1339–47.
Google Scholar
-
Tsang WY, Spektor A, Vijayakumar S, Bista BR, Li J, Sanchez I, et al. Cep76, a centrosomal protein that specifically restrains centriole re-duplication. Dev Cell. 2009;16:649.
Google Scholar
-
Takeda Y, Yamazaki K, Hashimoto K, Watanabe K, Chinen T, Kitagawa D. The centriole protein CEP76 negatively regulates PLK1 activity in the cytoplasm for proper mitotic progression. J Cell Sci. 2020;133:jcs241281.
-
Li P, Marshall L, Oh G, Jakubowski JL, Groot D, He Y, et al. Epigenetic dysregulation of enhancers in neurons is associated with Alzheimer’s disease pathology and cognitive symptoms. Nat Commun. 2019;10:2246.
-
Hegelund ER, Grønkjær M, Osler M, Dammeyer J, Flensborg-Madsen T, Mortensen EL. The influence of educational attainment on intelligence. Intelligence. 2020;78:101419.
Google Scholar
-
Bohlin J, Håberg SE, Magnus P, Reese SE, Gjessing HK, Magnus MC, et al. Prediction of gestational age based on genome-wide differentially methylated regions. Genome Biol 2016;17:1–9.
Google Scholar
-
de Goede OM, Lavoie PM, Robinson WP. Cord blood hematopoietic cells from preterm infants display altered DNA methylation patterns. Clin Epigenetics. 2017;9:1–13.
Google Scholar
-
Spiers H, Hannon E, Schalkwyk LC, Smith R, Wong CCY, O’Donovan MC, et al. Methylomic trajectories across human fetal brain development. Genome Res. 2015;25:338–52.
Google Scholar
-
Hanna CW. Placental imprinting: emerging mechanisms and functions. PLoS Genet. 2020;16:e1008709.
-
Isles AR. The contribution of imprinted genes to neurodevelopmental and neuropsychiatric disorders. Transl Psychiatry. 2022;12:210.
Google Scholar
-
Ursini G, Punzi G, Chen Q, Marenco S, Robinson JF, Porcelli A, et al. Convergence of placenta biology and genetic risk for schizophrenia. Nat Med. 2018;24:792–801.
Google Scholar
-
Hwang J-Y, Lee SH, Go MJ, Kim B-J, Kou I, Ikegawa S, et al. Meta-analysis identifies a MECOM gene as a novel predisposing factor of osteoporotic fracture. J Med Genet. 2013;50:212–9.
Google Scholar
-
Wieser R. The oncogene and developmental regulator EVI1: expression, biochemical properties, and biological functions. Gene. 2007;396:346–57.
Google Scholar
-
Xu X, Woo C-H, Steere RR, Lee BC, Huang Y, Wu J, et al. EVI1 acts as an inducible negative-feedback regulator of NF-κB by inhibiting p65 acetylation. J Immunol. 2012;188:6371–80.
Google Scholar
-
Jiang NM, Cowan M, Moonah SN, Petri WA. The impact of systemic inflammation on neurodevelopment. Trends Mol Med. 2018;24:794–804.
Google Scholar
-
Deverman BE, Patterson PH. Cytokines and CNS development. Neuron. 2009;64:61–78.
Google Scholar
-
Schepanski S, Buss C, Hanganu-Opatz IL, Arck PC. Prenatal immune and endocrine modulators of offspring’s brain development and cognitive functions later in life. Front Immunol 2018;9:2186.
Google Scholar
-
Bauman MD, Van de Water J. Translational opportunities in the prenatal immune environment: promises and limitations of the maternal immune activation model. Neurobiol Dis 2020;141:104864.
Google Scholar
-
Tomlinson MS, Bommarito PA, Martin EM, Smeester L, Fichorova RN, Onderdonk AB, et al. Microorganisms in the human placenta are associated with altered CpG methylation of immune and inflammation-related genes. PloS One. 2017;12:e0188664.
Google Scholar
-
Szklarczyk D, Gable AL, Nastou KC, Lyon D, Kirsch R, Pyysalo S, et al. The STRING database in 2021: customizable protein–protein networks, and functional characterization of user-uploaded gene/measurement sets. Nucleic Acids Res. 2020;49:D605–D612.
Google Scholar
-
Kazakevych J, Denizot J, Liebert A, Portovedo M, Mosavie M, Jain P, et al. Smarcad1 mediates microbiota-induced inflammation in mouse and coordinates gene expression in the intestinal epithelium. Genome Biol 2020;21:64.
Google Scholar
-
Qin Y, Li Q, Liang W, Yan R, Tong L, Jia M, et al. TRIM28 SUMOylates and stabilizes NLRP3 to facilitate inflammasome activation. Nat Commun 2021;12:4794.
Google Scholar
-
Garrido-Gomez T, Ona K, Kapidzic M, Gormley M, Simón C, Genbacev O, et al. Severe pre-eclampsia is associated with alterations in cytotrophoblasts of the smooth chorion. Dev Camb Engl 2017;144:767–77.
Google Scholar
-
Lecorguillé M, Charles M-A, Lepeule J, Lioret S, de Lauzon-Guillain B, Forhan A, et al. Association between dietary patterns reflecting one-carbon metabolism nutrients intake before pregnancy and placental DNA methylation. Epigenetics. 2022;17:715–30.
Google Scholar
-
Kawai T, Yamada T, Abe K, Okamura K, Kamura H, Akaishi R, et al. Increased epigenetic alterations at the promoters of transcriptional regulators following inadequate maternal gestational weight gain. Sci Rep. 2015;5:14224.
Google Scholar
-
McKinney B, Ding Y, Lewis DA, Sweet RA. DNA methylation as a putative mechanism for reduced dendritic spine density in the superior temporal gyrus of subjects with schizophrenia. Transl Psychiatry. 2017;7:e1032.
Google Scholar
Acknowledgements
We acknowledge support from the grant CEX2018-000806-S funded by MCIN/AEI/10.13039/501100011033, from the Generalitat de Catalunya through the CERCA Programme; and from the European Union’s Horizon 2020 research and innovation programme-EU.3.1.2. (874583–ATHLETE project); grants from UE (FP7-ENV-2011 cod 282957 and HEALTH.2010.2.4.5-1), Spain: ISCIII (Red INMA G03/176, CB06/02/0041; FIS-FEDER: PI03/1615, PI04/1509, PI04/1112, PI04/1931, PI05/1079, PI05/1052, PI06/1213, PI07/0314, PI09/02647, PI11/01007, PI11/02591, PI11/02038, PI12/00610, PI13/1944, PI13/2032, PI14/00891, PI14/01687, PI16/1288, PI17/00663, PI16/1288 and PI1338;; Miguel Servet-FEDER CP11/00178, CP15/00025, MS20/0005, and CPII16/00051, G03/176; Generalitat Valenciana (CIAICO/2021/132): FISABIO (UGP-15-230, UGP-15-244, and UGP-15-249), Ministry of Universities (CAS21/00008 and NextGeneration EU), and Alicia Koplowitz Foundation 2017; grants from Instituto de Salud Carlos III (Red INMA G03/176; CB06/02/0041; PI041436; PI081151 incl. FEDER funds), Generalitat de Catalunya-CIRIT 1999SGR 00241, Fundació La marató de TV3 (090430); grants from Instituto de Salud Carlos III (PI06/0867, PI11/00610, FIS-PI09/00090, FIS-PI13/02187, FIS-PI13/02406, and FIS-PI18/01142 incl. FEDER funds), CIBERESP, Department of Health of the Basque Government (2005111093, 2009111069, 2013111089 and 2015111065), Provincial Government of Gipuzkoa (DFG06/002), and annual agreements with the municipalities of the study area (Zumarraga, Urretxu, Legazpi, Azkoitia y Azpeitia y Beasain). MG was funded by a Miguel Servet-II fellowship (CPII18/00018) awarded by the Spanish Institute of Health Carlos III. MCT is funded by a Beatriu de Pinós Postdoctoral Contract awarded by Generalitat de Catalunya-AGAUR and European Commission-Horizon 2020 (2019 BP 00107). JJ was funded by a Miguel Servet-II fellowship (CPII19/00015) awarded by the Instituto de Salud Carlos III (Co-funded by the European Union Social Fund “Investing in your future”). We acknowledge all volunteers participating in the study, and interviewers and psychologists.
Author information
Authors and Affiliations
Contributions
LD: formal analysis, writing—original draft, visualisation; ACP: methodology, resources, writing—review & editing; NF, MG, JJ: methodology, resources, funding acquisition, writing—review & editing; MF: data curation, resources, writing—review & editing; SL, MLE, ML, JI: resources, funding acquisition, writing—review & editing; MS: data curation, resources, funding acquisition, writing—review & editing; JS: conceptualisation, resources, funding acquisition, writing—review & editing, project administration, supervision; MB: conceptualisation, methodology, resources, writing—review & editing, supervision, project administration; MCT: conceptualisation, methodology, formal analysis, investigation, resources, writing—original draft, visualisation, supervision (corresponding author).
Corresponding author
Ethics declarations
Competing interests
The authors declare there are no competing financial interests in relation to the work described.
Ethics approval and consent to participate
The study was approved by the Ethical Committee of the Municipal Institute of Medical Investigation and by the Ethical Committee of the hospitals involved in the study. The pregnant women received information about the study, both written and oral. The informed consent of the participants was asked during each of the visits. A full roster of the INMA Project Investigators can be found at http://www.proyectoinma.org/presentacion-inma/listado-investigadores/en_listado-investigadores.html.
Additional information
Publisher’s note Springer Nature remains neutral with regard to jurisdictional claims in published maps and institutional affiliations.
Supplementary information
Supplementary Material
Rights and permissions
Open Access This article is licensed under a Creative Commons Attribution-NonCommercial-NoDerivatives 4.0 International License, which permits any non-commercial use, sharing, distribution and reproduction in any medium or format, as long as you give appropriate credit to the original author(s) and the source, provide a link to the Creative Commons licence, and indicate if you modified the licensed material. You do not have permission under this licence to share adapted material derived from this article or parts of it. The images or other third party material in this article are included in the article’s Creative Commons licence, unless indicated otherwise in a credit line to the material. If material is not included in the article’s Creative Commons licence and your intended use is not permitted by statutory regulation or exceeds the permitted use, you will need to obtain permission directly from the copyright holder. To view a copy of this licence, visit http://creativecommons.org/licenses/by-nc-nd/4.0/.
Reprints and permissions
About this article
Cite this article
Diez-Ahijado, L., Cilleros-Portet, A., Fernández-Jimenez, N. et al. Evaluating the association between placenta DNA methylation and cognitive functions in the offspring.
Transl Psychiatry 14, 383 (2024). https://doi.org/10.1038/s41398-024-03094-5
-
Received: 24 July 2023
-
Revised: 31 August 2024
-
Accepted: 04 September 2024
-
Published: 20 September 2024
-
DOI: https://doi.org/10.1038/s41398-024-03094-5