Dr. AI is setting up practice in a medical setting near you. And experts say your health care is likely to improve in multiple ways with that new attention to detail. But in some areas, you might want to steer clear.
Artificial intelligence is quickly becoming a staple in growing segments of health care, but it’s not ready in others. Still, experts say you need not worry that you’ll lose the personal touch if you’ve been getting that from a medical provider: Humans are as important as ever in the practice of medicine. You might even find care providers have more time to address your needs.
The National Institutes of Health notes artificial intelligence tools are driving widespread change across medical disciplines including research, diagnosis and treatment. Concurrent advancements in computing power and the proliferation of massive, health-related data sets are setting the stage for new approaches in the research field as scientists increasingly employ AI software and its powerful information processing capabilities to advance their work at an increasingly rapid pace.
“I am excited about the technology,” said attorney Daniel J. Gilman, senior scholar at the International Center for Law and Economics, a nonpartisan, nonprofit research center based in Portland, Oregon. “I think we’ve seen that as long as it is introduced and used in a careful and responsible fashion, AI seems to have tremendous promise.”
From experiment to problem solver
Dr. Yves Lussier is both physician and engineer — and an unabashed AI enthusiast. At the University of Utah School of Medicine, he’s department chair of biomedical informatics — the founding department of that field in the U.S. and maybe the world, dating from the late 1950s, he said.
Lussier traces the roots of AI to the 1940s, when neural networks were developed to “reason with uncertainty.” Later, AI advanced to reason with certainty. The pace of each AI breakthrough has been faster than the one before. By the mid-1970s, software from Stanford could reason with both certainty and uncertainty — “expert systems,” he said. Since, Depp learning (15 years ago) and “transformers” (seven years ago) have led to the emerging conversational AIs called “generative AI,” such as ChatGPT.
AI types abound. Most people don’t realize the voice recognition that’s an open sesame to your bank account is AI. What Lussier calls the “game changer” came seven years ago with generative AI, which can be prompted to create texts, images, videos and other data. You can push a transcript into a generative AI and retrieve plainspoken words or technical terms depending on your audience.
The biggest impact, perhaps, is helping solve problems that have been largely intractable.
Dr. Nathan Blue is an obstetrician and assistant professor at the University of Utah School of Medicine’s Department of Obstetrics and Gynecology. Blue has been involved with research efforts for over a decade, working to develop new clinical diagnostic strategies that can identify early signs of pregnancy complications that arise from deficiencies in placental material.
Those deficiencies, Blue said, can lead to fetal growth restrictions, complications involving bleeding, preeclampsia and stillbirths. The traditional research strategies to quantify risk associated with placental deficiencies have a lot of pitfalls, he said, and can be crude and inflexible.
New research techniques that incorporate AI systems are showing promise for overcoming some of the inefficiencies of previous strategies and could lead to new clinical practices that will, Blue said, lower stress for expectant mothers, help reduce uncertainty about medical intervention decisions and lead to better use of resources.
“In the last couple of years, we’ve started working with the bioinformatics and genomics group here at the U.,” Blue said. “These senior thought leaders and investigators have helped us leverage more computationally advanced approaches, including artificial intelligence, to better quantify risk.”
Part of the research work includes applying AI tools to large data troves, including anonymized genomic profiles of more than 10,000 obstetrics patients, and zeroing in on diagnostic markers that may become part of new clinical applications to help more accurately predict future at-risk pregnancies.
“On the research and investigation side, what is really exciting about what the AI-based tools and approach can offer is, until now, we’ve been trying different versions of the same thing,” Blue said. “Using pretty old-fashioned tools to find factors, but really we’re mucking about in the same sandbox, so to speak.
“What I’m super excited about in the AI-based strategy is it’s helping us bypass a lot of the pitfalls to analysis but boosting how we conceptualize how we use information. In that sense, the progress is really accelerating risk for pregnancy work and the application and accuracy of those tools is better than what we were getting before.”
Professor Xiaondong Ma from the University of Utah School of Medicine’s Department of Radiology and Imaging Sciences is a member of the Medical Imaging and Computational Analysis Lab, a research team working to develop advanced techniques, including AI in image acquisition, analysis and quantification for clinical and research applications.
Among other projects, he and his team are investigating vascular issues — particularly how abnormalities in the carotid artery could serve as indicators of more serious vascular pathologies.
Ma said analyzing images captured by MRI and/or CT scans has traditionally been a manual, time-consuming process. Thanks to an AI-powered, semiautomatic image analysis technique being developed by the MICA lab, the work to develop new diagnostic strategies has accelerated.
Another MICA project that’s leveraging AI-based image analysis tools is looking at the connection between calcification that occurs in the brain and its relationship to the ravages of aging, he said.
“We have the potential to predict vascular disease and diseases associated with aging, like Alzheimer’s,” Ma said. “Our hope is that AI can help us screen these images and define which patients may be of high risk.”
Workload triage
Women who’ve had a pap smear are already part of AI’s story in medicine. AI has been used for 30 years to sort through millions of exams annually to determine which need special attention, proving its worth as triage there and in radiology, among others. AI may spot the earliest signs of unhealthy tissue change that the naked eye could miss, saving time, money and suffering.
The AI is designed to have many false positives and no false negatives. “It doesn’t make an error of forgetting a cancer. But it claims 10 times more often that there’s cancer when there is none,” which a pathology expert sorts out, Lussier said. But how fast AI runs through images makes cervical cancer screening manageable and affordable.
Lussier said it took a decade or more to design that kind of artificial intelligence long years ago. Now, given the pace of advances, such a program could probably be created in weeks or months.
Would a redesign improve results? “No, it’s highly accurate. But it would cost a lot less now because it would take less time and be done with better tools. That’s the game changer,” said Lussier. Faster design using fewer resources means lower costs, accessible for more users within industries like health care, providing greater benefit for consumers.
AI takes a star turn
AI shines especially bright finding abnormalities in radiology images.
Gilman said AI imaging refinements now do a more sophisticated job than human eyes alone to discern noise in an image without introducing artifacts or losing information. Another strength is “signal detection — finding things with better images that a time-pressed radiologist might miss with a quick scan and the naked eye.”
But while AI can call something to an expert’s attention, it cannot diagnose. Radiologists check AI results because they have the experience and knowledge.
It’s hard to overestimate the value, though, of AI trained on millions of images to free up the physician’s time by going through the entire image workload to flag those needing attention. And AI can regularly review to see what might have been missed. “The radiologist, the physician, the oncologist is not eliminated. What’s eliminated is a big pile of time they spend staring at these things. They’re still going to stare at images, but they’re going to stare at the ones that really need attention,” Gilman said. “A considerable amount of the workload is shifted so the practitioner’s involvement is much more efficient.”
Dr. Christoph Wald, an American College of Radiology spokesman, believes AI and radiology are especially compatible because radiology is digital from image through answer. “We’re the first digital specialty that exists.”
But perhaps the greatest benefit for patients is making good images with less information, which means lower-dose radiation or shorter tests without sacrificing quality.
The FDA has approved AI in radiology for triage. What AI can say amounts to, “‘I am reasonably certain this case is positive for the finding that I was trained on,’” said Wald. “It doesn’t say the disease is present, but it flags it so the human expert can make the call.”
AI is trained to find a little black line, for instance, not diagnose a broken neck. Other things can create that line, including “things on the image that aren’t real. The radiologist will say, ‘I know why you’re saying that. But that’s not a break.’ It’s really important to understand that distinction because AI doesn’t try to diagnose.”
Quantitative AI is another bit of magic in radiology. On the lung CT of a longtime smoker with emphysema, quantitative AI measures what portion of the lung is diseased. A human cannot do that. That information helps decide how intensive therapy should be, said Wald, so AI impacts treatment. Differently trained AIs can quantify fat, muscle mass, calcium in arteries, even brain thickness for patients with neurodegenerative disease. AI’s measuring capacity keeps growing.
Certain patterns — lots of fat, little muscle, bone calcium that’s not dense — could signal a patient at great risk for metabolic disease, creating opportunistic screening that improves care. It’s not feasible to have a human do that, but when AI can — quickly, at scale — it becomes feasible, said Wald.
AI can also help a radiologist by zipping through the electronic health record to see what’s known about the patient, summarizing large chunks of information to help physicians reach correct conclusions.
Couldn’t insurance companies use the same tools to exclude people from coverage? Probably, he said. But they already analyze data to risk-stratify premiums. “They know a lot of that already. The fact we’re using AI inside the electronic health record does not mean we’re revealing more about you to the outer world. It’s a processing tool,” Wald said.
AI gets an enthusiastic high-five from care providers for summarizing the latest from ever-growing knowledge in medical subspecialties. “We’re hopeful with AI it will become easier to discover relevant developments that no single human can possibly constantly monitor,” Wald said.
Radiology AI is narrowly focused, so multiple products may be strung together. His department has AI that looks for pulmonary embolism and another scanning for preincidental pulmonary embolism. Yet another AI looks for rib fractures. “That’s three AIs we have to license to get a not even comprehensive assessment of a chest CT for a couple of important findings.” Looking for other things requires differently trained AI.
More time for you
Helping care providers manage a time crunch is a major expected AI benefit in health care, experts told Deseret News. Electronic health records have made it easy to share medical information with other professionals, but building those records takes a couple of hours a day writing notes, leading to an “epidemic of burnout among nurses and physicians because it adds too much of a burden on every (patient) visit,” said Lussier.
“We’re gonna hope (AI) will reduce that.”
Fortunately, many of AI’s advantages reduce both time drag and administrative costs, “which have become staggering in health care,” per Gilman.
Some AI applications figure out complicated scheduling. Since most imaging providers are overloaded with patients — Wald’s practice has a six-week wait for a non-urgent MRI — AI can help make use of the machine’s every moment. Duration for exams varies: A cardiac MRI could take two hours, a knee MRI 15 minutes. The variety makes it tough to efficiently slot everyone in. AI is unfazed. “It can put these complex requests in a pattern shown to work well,” Wald said.
AI could figure out how long operations take and whether some surgeons are faster on average to schedule assets like operating rooms efficiently. It could reduce time waiting for an appointment as well as waits at the clinic.
Lussier said if AI improves scheduling or otherwise frees up time, it could be used for patient care.
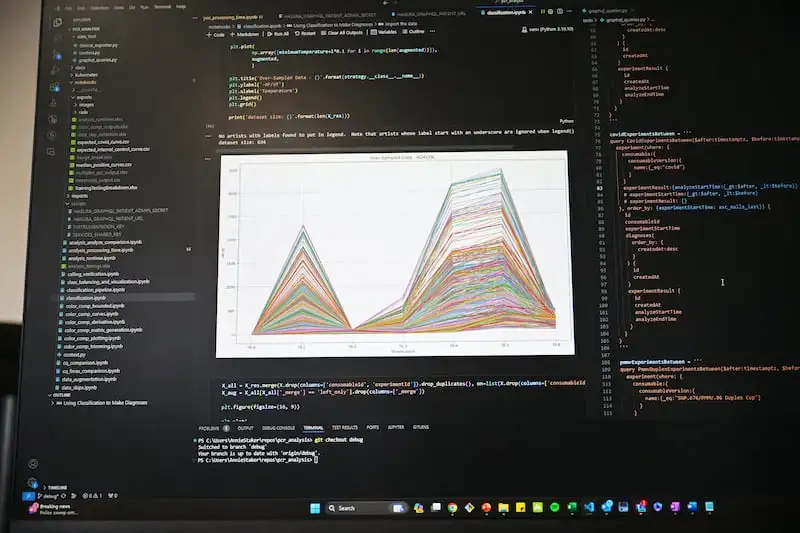
An elusive diagnosis
When a patient has a complaint, the option is some combination of a physical exam, lab work or imaging. AI can help the doctor figure out what kind of imaging to order. It helps patients, too. “If you were to go to Bing, Copilot or ChatGPT and say, ‘I’ve had a big headache for four weeks, what’s the best test to do?’ you’d get a pretty good answer,” said Wald. You could see if your doctor agreed.
“AI is really good at navigating a large body of insight and distilling it down to a reasonable recommendation,” he said.
Lussier tells the story of a mom who took her young son to 17 doctors in three years seeking the cause of his constant pain. She told Today that each specialist would address symptoms within their own area of expertise, but no true answer emerged. Frustrated, the mom typed his symptoms and every bit of his MRI notes into ChatGPT, which suggested tethered cord syndrome, an invisible condition associated with spina bifida. She’d never heard of it in all those doctor visits, but the AI suggested consulting a neurosurgeon. Her son was finally helped.
But AI doesn’t always get it right. Washington State University reported in the journal PLOS ONE recently that in a study with thousands of simulated cases of patients who had chest pain, ChatGPT’s suggestions were inconsistent. It came up with drastically different heart risk assessment levels when it was given the same patient information. In a news release, researchers said that’s “likely due to the level of randomness built into the current version of the software, ChatGPT4, which helps it vary its responses to simulate natural language. This same randomness, however, does not work well for health care uses that require a single, consistent answer,” per the lead researcher.
Gentle communicator
Lussier’s half joking when he notes that a kind, detailed missive from a physician was likely crafted by AI. There’s truth to it, because AI can be taught to send a detailed and humane message with information and recommendations that not all physicians have time to craft for every case.
“It generates notes that are, strangely enough, more compassionate to the patient, because physicians and nurses are under duress and on a very tight schedule,” said Lussier.
Those missives give patients basic information, answer common questions about diseases and procedures, and provide clear instructions. They don’t require a separate writing session for each patient, yet don’t read like a form letter. The tone is designed to be warm and reassure patients about whatever medical journey they’re on.
Humans vet the letters and brochures. AI just makes it easier. Lussier said that a blinded group of physicians compared letters made by ChatGPT and those by peers and could not tell the difference, though, exposed to the letters often enough, they began to spot AI.
AI can generate reports on the same case for two target readers: a technical one for medical staff and another for the patient, said Wald. AI can easily embed definitions, hyperlinks and other aids.
When it comes to language barriers, Wald said, “Seamless term translation; just absolutely fabulous.”
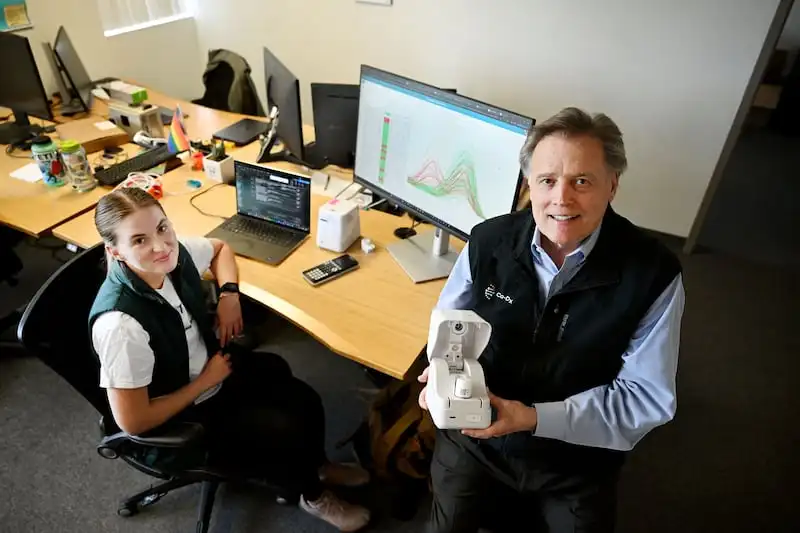
Peering into the future
Among AI’s promising areas is disease surveillance: spotting trends in public health. AI’s great at mining large datasets to find patterns, as it did during COVID-19, Lussier said. Researchers used a massive U.K. database to see if COVID-19 made people with certain cancers more likely to die, finding it severely complicated melanoma, but not breast cancer.
That’s not just for public health, but in ways that could change individual outcomes. Lussier said AI helps untangle health interplay, like whether using a drug to treat high cholesterol might prevent or delay Alzheimer’s or another drug might increase risk. Findings must be confirmed by clinical studies, but AI can help spot connections that elude clinicians. Ensuring it’s not “purely a spurious association” is vital.
Drug discovery is promising with AI. So is designing tests. AI helped Co-Diagnostics, a Salt Lake-based company that makes polymerase chain reaction, or PCR, diagnostic tests for conditions ranging from COVID-19 to tuberculosis, flu, strep and others. PCR makes a high number of copies of specific segments of DNA.
Dwight Egan, CEO, said AI sped and improved the tests’ development, proving invaluable in medical tool innovation, including for making a small testing unit called Co-Dx PCR Pro to enable home or clinical testing. You add a swabbed sample to a test-specific cartridge in the unit. The results come back in half an hour.
While they’re hoping for U.S. regulatory approval this year, the goal is to make the low-cost diagnostic tool available in countries where diagnoses are challenging, like India and Africa. Fifteen tests have been cleared by India’s government, where getting a speedy result is crucial, because people often travel far to see a clinician but can’t wait long for test results.
“We leverage AI daily in our toolsets,” Egan said.
Chris Thurston, Co-Diagnostics’ chief technology officer, hopes AI advances to the point one could feed it geographic prevalence of an illness and patient data like viral load to predict whether someone who tests positive is likely contagious. Right now, a specialist might say you’re positive but don’t seem too sick; you’re probably not contagious. “He makes the prediction. But I do see that in the near future the decision will be data driven.”
Check the work
Gilman warned it’s important to have a system on the back end in health care for “evaluation and scrutiny, to make sure things are going as they should and that there are ways to intervene if you’re getting anomalous results. I don’t think there’s anything fundamentally strange about that; you’re always balancing,” he added, noting that with drug approval, for instance, there’s a terrific amount of testing beforehand, but also ongoing surveillance so problems can be reported if they arise after a drug is approved.
“You want real and serious checks on the quality of the tools you’re using, whether those are medical devices or drugs or software,” said Gilman. “By the same token, you don’t want to be so careful you impede patient access to care. You have to balance being high quality and efficient.”
Spurious associations dot AI’s journey. Lussier smiles when he tells how good AI was at spotting the difference between dogs and wolves. It wasn’t flawless, but seemed to do pretty well until someone tested whether it was crying wolf based on background scenery. Turns out “it knew the one in snow was more likely to be a wolf.”
AI’s not good at explaining its findings and it can be overwhelmed. AI must do things systematically and if there’s a lot to consider — “like how 12 medications and 12 diseases interact with each other, for example — it just stops. It will find three or four of them. It doesn’t have the grit to go through them all,” Lussier said. AI thrives on short, simple commands.
You could ask AI to look at each of the pairs separately, with different prompts for each. That’s not very efficient.
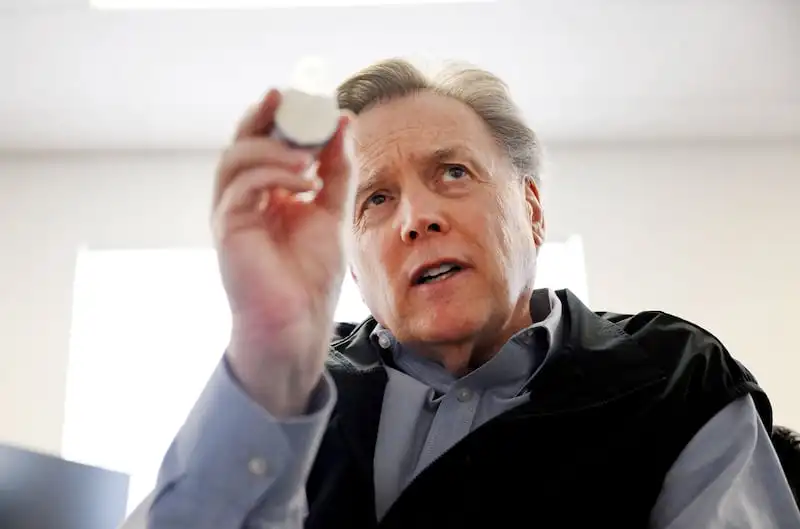
AI ‘hallucinations’
Ma and Blue both note that their respective research work relies on a hybrid approach that pairs high-powered AI tools with the expertise of scientists. While AI tools are accelerating scientific data processing and analysis, both researchers said AI-generated output comes with flaws that may include issues like bias and random conclusion errors, sometimes referred to as “hallucinations.”
AI hallucinations occur when the systems perceive patterns that don’t really exist and lead to nonsensical or inaccurate outputs. Bias can arise from the datasets used to train AI systems. If the underlying data is skewed, analysis and conclusions that arise from that data can reflect the same inaccuracies.
Ma said AI-generated data is scrutinized by scientists before being incorporated into research.
“We are very careful about how we process this kind of output because of the nature of our work: health care,” Ma said. “We need to ensure output is accurate and reliable.”
Still, experts who use AI see more benefits than problems. Gilman thinks “some of the dire worries that people talk about outside the health care sector seem to flirt with science fiction.” Inside the realm of health care, though, he has two concerns: quality control and data security. Being able to transmit data ever further offers advantages like connecting a physician in a very small town with a network of specialists at a big medical center. But that comes with security risks.
“To me, risks are risks to manage; they’re not big. I don’t have any grand science fiction fears for AI in health care. But that doesn’t mean I know what the future will look like in 20 years. Thus far, I can see some very useful applications,” he said.
Lussier thinks AI could pose problems if tasked with jobs for which it’s not fit. “I would reconsider using imaging in clinical care right now from generative AI, especially if there’s writing in it. I would be concerned having generative AI try to explain to a patient with multiple diseases and drugs that interact with one another. It’s not there yet.”
Generative AI doesn’t draw pictures, either, so it can’t illustrate that brochure it worded so beautifully to explain mechanics of a heart valve. It could create a conceptual illustration for the cover, probably. But a technically correct picture to explain anatomical anomaly? Nope.
AI’s red hot right now and there’s a temptation to use it everywhere, Thurston said. Sometimes it doesn’t add value to a traditional algorithmic approach, which they learned when they tried it for certain tasks. He said it hasn’t made its way into mechanical or engineering sides of his company, though the software team has adopted AI for many uses.
Where does Wald think AI should not go? “I think we must be very careful when we use AI on our individual patient populations. AI is typically trained on a relatively small number of patients. We absolutely need to make sure as local practitioners we validate that the technology is working as promised on our own patients. That’s currently not the case. Most practices do not have the wherewithal to actually monitor how well that stuff’s working. That’s a gap we need to close.”
He adds, “If you decide to use AI, make sure it works on your patients all the time and over time.”
Wald also strongly opposes autonomous AI, meaning AI that makes decisions without human oversight or validation. And he warns most AI in the U.S. is being trained on datasets from big academic medical centers, whose patient populations “are not necessarily representative of the population in the rest of the country,” which could build in bias unintentionally.
It’s vital to remember that AI makes mistakes in medicine, as it does in other realms. And if AI becomes an excuse to reduce staff, that’s no win for health providers or patients.
Blue said in spite of the fast-evolving usefulness of AI tools, he doesn’t foresee any near-term future where AI wholly replaces the work being done by medical researchers or health care clinicians.
“There’s a role for efficient processing and analysis of information but the end goal of both researchers and clinicians is to provide the best patient care attainable,” Blue said. “And that is work that requires the human traits of empathy, insight and perspective.”
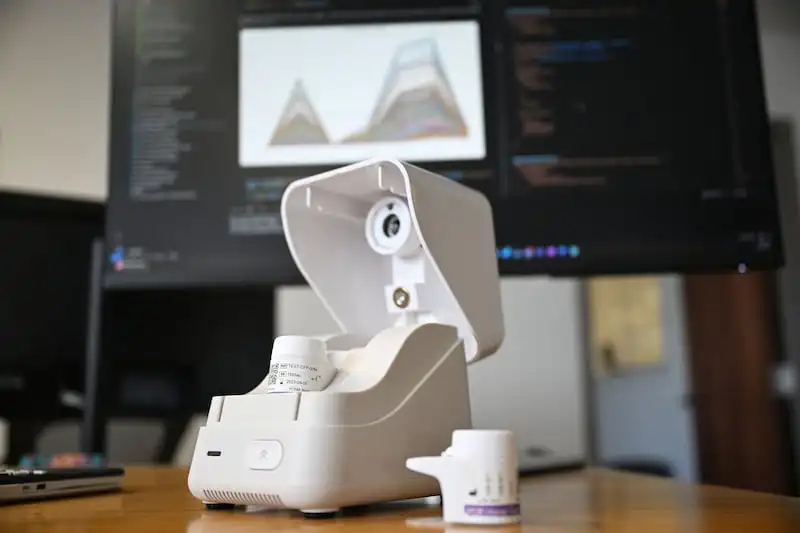