Abstract
Neural networks find widespread use in scientific and technological applications, yet their implementations in conventional computers have encountered bottlenecks due to ever-expanding computational needs. Photonic computing is a promising neuromorphic platform with potential advantages of massive parallelism, ultralow latency and reduced energy consumption but mostly for computing linear operations. Here we demonstrate a large-scale, high-performance nonlinear photonic neural system based on a disordered polycrystalline slab composed of lithium niobate nanocrystals. Mediated by random quasi-phase-matching and multiple scattering, linear and nonlinear optical speckle features are generated as the interplay between the simultaneous linear random scattering and the second-harmonic generation, defining a complex neural network in which the second-order nonlinearity acts as internal nonlinear activation functions. Benchmarked against linear random projection, such nonlinear mapping embedded with rich physical computational operations shows improved performance across a large collection of machine learning tasks in image classification, regression and graph classification. Demonstrating up to 27,648 input and 3,500 nonlinear output nodes, the combination of optical nonlinearity and random scattering serves as a scalable computing engine for diverse applications.
This is a preview of subscription content, access via your institution
Access options
style{display:none!important}.LiveAreaSection-193358632 *{align-content:stretch;align-items:stretch;align-self:auto;animation-delay:0s;animation-direction:normal;animation-duration:0s;animation-fill-mode:none;animation-iteration-count:1;animation-name:none;animation-play-state:running;animation-timing-function:ease;azimuth:center;backface-visibility:visible;background-attachment:scroll;background-blend-mode:normal;background-clip:borderBox;background-color:transparent;background-image:none;background-origin:paddingBox;background-position:0 0;background-repeat:repeat;background-size:auto auto;block-size:auto;border-block-end-color:currentcolor;border-block-end-style:none;border-block-end-width:medium;border-block-start-color:currentcolor;border-block-start-style:none;border-block-start-width:medium;border-bottom-color:currentcolor;border-bottom-left-radius:0;border-bottom-right-radius:0;border-bottom-style:none;border-bottom-width:medium;border-collapse:separate;border-image-outset:0s;border-image-repeat:stretch;border-image-slice:100%;border-image-source:none;border-image-width:1;border-inline-end-color:currentcolor;border-inline-end-style:none;border-inline-end-width:medium;border-inline-start-color:currentcolor;border-inline-start-style:none;border-inline-start-width:medium;border-left-color:currentcolor;border-left-style:none;border-left-width:medium;border-right-color:currentcolor;border-right-style:none;border-right-width:medium;border-spacing:0;border-top-color:currentcolor;border-top-left-radius:0;border-top-right-radius:0;border-top-style:none;border-top-width:medium;bottom:auto;box-decoration-break:slice;box-shadow:none;box-sizing:border-box;break-after:auto;break-before:auto;break-inside:auto;caption-side:top;caret-color:auto;clear:none;clip:auto;clip-path:none;color:initial;column-count:auto;column-fill:balance;column-gap:normal;column-rule-color:currentcolor;column-rule-style:none;column-rule-width:medium;column-span:none;column-width:auto;content:normal;counter-increment:none;counter-reset:none;cursor:auto;display:inline;empty-cells:show;filter:none;flex-basis:auto;flex-direction:row;flex-grow:0;flex-shrink:1;flex-wrap:nowrap;float:none;font-family:initial;font-feature-settings:normal;font-kerning:auto;font-language-override:normal;font-size:medium;font-size-adjust:none;font-stretch:normal;font-style:normal;font-synthesis:weight style;font-variant:normal;font-variant-alternates:normal;font-variant-caps:normal;font-variant-east-asian:normal;font-variant-ligatures:normal;font-variant-numeric:normal;font-variant-position:normal;font-weight:400;grid-auto-columns:auto;grid-auto-flow:row;grid-auto-rows:auto;grid-column-end:auto;grid-column-gap:0;grid-column-start:auto;grid-row-end:auto;grid-row-gap:0;grid-row-start:auto;grid-template-areas:none;grid-template-columns:none;grid-template-rows:none;height:auto;hyphens:manual;image-orientation:0deg;image-rendering:auto;image-resolution:1dppx;ime-mode:auto;inline-size:auto;isolation:auto;justify-content:flexStart;left:auto;letter-spacing:normal;line-break:auto;line-height:normal;list-style-image:none;list-style-position:outside;list-style-type:disc;margin-block-end:0;margin-block-start:0;margin-bottom:0;margin-inline-end:0;margin-inline-start:0;margin-left:0;margin-right:0;margin-top:0;mask-clip:borderBox;mask-composite:add;mask-image:none;mask-mode:matchSource;mask-origin:borderBox;mask-position:0 0;mask-repeat:repeat;mask-size:auto;mask-type:luminance;max-height:none;max-width:none;min-block-size:0;min-height:0;min-inline-size:0;min-width:0;mix-blend-mode:normal;object-fit:fill;object-position:50% 50%;offset-block-end:auto;offset-block-start:auto;offset-inline-end:auto;offset-inline-start:auto;opacity:1;order:0;orphans:2;outline-color:initial;outline-offset:0;outline-style:none;outline-width:medium;overflow:visible;overflow-wrap:normal;overflow-x:visible;overflow-y:visible;padding-block-end:0;padding-block-start:0;padding-bottom:0;padding-inline-end:0;padding-inline-start:0;padding-left:0;padding-right:0;padding-top:0;page-break-after:auto;page-break-before:auto;page-break-inside:auto;perspective:none;perspective-origin:50% 50%;pointer-events:auto;position:static;quotes:initial;resize:none;right:auto;ruby-align:spaceAround;ruby-merge:separate;ruby-position:over;scroll-behavior:auto;scroll-snap-coordinate:none;scroll-snap-destination:0 0;scroll-snap-points-x:none;scroll-snap-points-y:none;scroll-snap-type:none;shape-image-threshold:0;shape-margin:0;shape-outside:none;tab-size:8;table-layout:auto;text-align:initial;text-align-last:auto;text-combine-upright:none;text-decoration-color:currentcolor;text-decoration-line:none;text-decoration-style:solid;text-emphasis-color:currentcolor;text-emphasis-position:over right;text-emphasis-style:none;text-indent:0;text-justify:auto;text-orientation:mixed;text-overflow:clip;text-rendering:auto;text-shadow:none;text-transform:none;text-underline-position:auto;top:auto;touch-action:auto;transform:none;transform-box:borderBox;transform-origin:50% 50%0;transform-style:flat;transition-delay:0s;transition-duration:0s;transition-property:all;transition-timing-function:ease;vertical-align:baseline;visibility:visible;white-space:normal;widows:2;width:auto;will-change:auto;word-break:normal;word-spacing:normal;word-wrap:normal;writing-mode:horizontalTb;z-index:auto;-webkit-appearance:none;-moz-appearance:none;-ms-appearance:none;appearance:none;margin:0}.LiveAreaSection-193358632{width:100%}.LiveAreaSection-193358632 .login-option-buybox{display:block;width:100%;font-size:17px;line-height:30px;color:#222;padding-top:30px;font-family:Harding,Palatino,serif}.LiveAreaSection-193358632 .additional-access-options{display:block;font-weight:700;font-size:17px;line-height:30px;color:#222;font-family:Harding,Palatino,serif}.LiveAreaSection-193358632 .additional-login>li:not(:first-child)::before{transform:translateY(-50%);content:””;height:1rem;position:absolute;top:50%;left:0;border-left:2px solid #999}.LiveAreaSection-193358632 .additional-login>li:not(:first-child){padding-left:10px}.LiveAreaSection-193358632 .additional-login>li{display:inline-block;position:relative;vertical-align:middle;padding-right:10px}.BuyBoxSection-683559780{display:flex;flex-wrap:wrap;flex:1;flex-direction:row-reverse;margin:-30px -15px 0}.BuyBoxSection-683559780 .box-inner{width:100%;height:100%;padding:30px 5px;display:flex;flex-direction:column;justify-content:space-between}.BuyBoxSection-683559780 p{margin:0}.BuyBoxSection-683559780 .readcube-buybox{background-color:#f3f3f3;flex-shrink:1;flex-grow:1;flex-basis:255px;background-clip:content-box;padding:0 15px;margin-top:30px}.BuyBoxSection-683559780 .subscribe-buybox{background-color:#f3f3f3;flex-shrink:1;flex-grow:4;flex-basis:300px;background-clip:content-box;padding:0 15px;margin-top:30px}.BuyBoxSection-683559780 .subscribe-buybox-nature-plus{background-color:#f3f3f3;flex-shrink:1;flex-grow:4;flex-basis:100%;background-clip:content-box;padding:0 15px;margin-top:30px}.BuyBoxSection-683559780 .title-readcube,.BuyBoxSection-683559780 .title-buybox{display:block;margin:0;margin-right:10%;margin-left:10%;font-size:24px;line-height:32px;color:#222;text-align:center;font-family:Harding,Palatino,serif}.BuyBoxSection-683559780 .title-asia-buybox{display:block;margin:0;margin-right:5%;margin-left:5%;font-size:24px;line-height:32px;color:#222;text-align:center;font-family:Harding,Palatino,serif}.BuyBoxSection-683559780 .asia-link{color:#069;cursor:pointer;text-decoration:none;font-size:1.05em;font-family:-apple-system,BlinkMacSystemFont,”Segoe UI”,Roboto,Oxygen-Sans,Ubuntu,Cantarell,”Helvetica Neue”,sans-serif;line-height:1.05em6}.BuyBoxSection-683559780 .access-readcube{display:block;margin:0;margin-right:10%;margin-left:10%;font-size:14px;color:#222;padding-top:10px;text-align:center;font-family:-apple-system,BlinkMacSystemFont,”Segoe UI”,Roboto,Oxygen-Sans,Ubuntu,Cantarell,”Helvetica Neue”,sans-serif;line-height:20px}.BuyBoxSection-683559780 ul{margin:0}.BuyBoxSection-683559780 .link-usp{display:list-item;margin:0;margin-left:20px;padding-top:6px;list-style-position:inside}.BuyBoxSection-683559780 .link-usp span{font-size:14px;color:#222;font-family:-apple-system,BlinkMacSystemFont,”Segoe UI”,Roboto,Oxygen-Sans,Ubuntu,Cantarell,”Helvetica Neue”,sans-serif;line-height:20px}.BuyBoxSection-683559780 .access-asia-buybox{display:block;margin:0;margin-right:5%;margin-left:5%;font-size:14px;color:#222;padding-top:10px;text-align:center;font-family:-apple-system,BlinkMacSystemFont,”Segoe UI”,Roboto,Oxygen-Sans,Ubuntu,Cantarell,”Helvetica Neue”,sans-serif;line-height:20px}.BuyBoxSection-683559780 .access-buybox{display:block;margin:0;margin-right:10%;margin-left:10%;font-size:14px;color:#222;opacity:.8px;padding-top:10px;text-align:center;font-family:-apple-system,BlinkMacSystemFont,”Segoe UI”,Roboto,Oxygen-Sans,Ubuntu,Cantarell,”Helvetica Neue”,sans-serif;line-height:20px}.BuyBoxSection-683559780 .price-buybox{display:block;font-size:30px;color:#222;font-family:-apple-system,BlinkMacSystemFont,”Segoe UI”,Roboto,Oxygen-Sans,Ubuntu,Cantarell,”Helvetica Neue”,sans-serif;padding-top:30px;text-align:center}.BuyBoxSection-683559780 .price-buybox-to{display:block;font-size:30px;color:#222;font-family:-apple-system,BlinkMacSystemFont,”Segoe UI”,Roboto,Oxygen-Sans,Ubuntu,Cantarell,”Helvetica Neue”,sans-serif;text-align:center}.BuyBoxSection-683559780 .price-info-text{font-size:16px;padding-right:10px;color:#222;font-family:-apple-system,BlinkMacSystemFont,”Segoe UI”,Roboto,Oxygen-Sans,Ubuntu,Cantarell,”Helvetica Neue”,sans-serif}.BuyBoxSection-683559780 .price-value{font-size:30px;font-family:-apple-system,BlinkMacSystemFont,”Segoe UI”,Roboto,Oxygen-Sans,Ubuntu,Cantarell,”Helvetica Neue”,sans-serif}.BuyBoxSection-683559780 .price-per-period{font-family:-apple-system,BlinkMacSystemFont,”Segoe UI”,Roboto,Oxygen-Sans,Ubuntu,Cantarell,”Helvetica Neue”,sans-serif}.BuyBoxSection-683559780 .price-from{font-size:14px;padding-right:10px;color:#222;font-family:-apple-system,BlinkMacSystemFont,”Segoe UI”,Roboto,Oxygen-Sans,Ubuntu,Cantarell,”Helvetica Neue”,sans-serif;line-height:20px}.BuyBoxSection-683559780 .issue-buybox{display:block;font-size:13px;text-align:center;color:#222;font-family:-apple-system,BlinkMacSystemFont,”Segoe UI”,Roboto,Oxygen-Sans,Ubuntu,Cantarell,”Helvetica Neue”,sans-serif;line-height:19px}.BuyBoxSection-683559780 .no-price-buybox{display:block;font-size:13px;line-height:18px;text-align:center;padding-right:10%;padding-left:10%;padding-bottom:20px;padding-top:30px;color:#222;font-family:-apple-system,BlinkMacSystemFont,”Segoe UI”,Roboto,Oxygen-Sans,Ubuntu,Cantarell,”Helvetica Neue”,sans-serif}.BuyBoxSection-683559780 .vat-buybox{display:block;margin-top:5px;margin-right:20%;margin-left:20%;font-size:11px;color:#222;padding-top:10px;padding-bottom:15px;text-align:center;font-family:-apple-system,BlinkMacSystemFont,”Segoe UI”,Roboto,Oxygen-Sans,Ubuntu,Cantarell,”Helvetica Neue”,sans-serif;line-height:17px}.BuyBoxSection-683559780 .tax-buybox{display:block;width:100%;color:#222;padding:20px 16px;text-align:center;font-family:-apple-system,BlinkMacSystemFont,”Segoe UI”,Roboto,Oxygen-Sans,Ubuntu,Cantarell,”Helvetica Neue”,sans-serif;line-height:NaNpx}.BuyBoxSection-683559780 .button-container{display:flex;padding-right:20px;padding-left:20px;justify-content:center}.BuyBoxSection-683559780 .button-container>*{flex:1px}.BuyBoxSection-683559780 .button-container>a:hover,.Button-505204839:hover,.Button-1078489254:hover,.Button-2737859108:hover{text-decoration:none}.BuyBoxSection-683559780 .btn-secondary{background:#fff}.BuyBoxSection-683559780 .button-asia{background:#069;border:1px solid #069;border-radius:0;cursor:pointer;display:block;padding:9px;outline:0;text-align:center;text-decoration:none;min-width:80px;margin-top:75px}.BuyBoxSection-683559780 .button-label-asia,.ButtonLabel-3869432492,.ButtonLabel-3296148077,.ButtonLabel-1636778223{display:block;color:#fff;font-size:17px;line-height:20px;font-family:-apple-system,BlinkMacSystemFont,”Segoe UI”,Roboto,Oxygen-Sans,Ubuntu,Cantarell,”Helvetica Neue”,sans-serif;text-align:center;text-decoration:none;cursor:pointer}.Button-505204839,.Button-1078489254,.Button-2737859108{background:#069;border:1px solid #069;border-radius:0;cursor:pointer;display:block;padding:9px;outline:0;text-align:center;text-decoration:none;min-width:80px;max-width:320px;margin-top:20px}.Button-505204839 .btn-secondary-label,.Button-1078489254 .btn-secondary-label,.Button-2737859108 .btn-secondary-label{color:#069}
/* style specs end */
Access Nature and 54 other Nature Portfolio journals
Get Nature+, our best-value online-access subscription
$29.99 / 30 days
cancel any time
Subscribe to this journal
Receive 12 digital issues and online access to articles
$99.00 per year
only $8.25 per issue
Buy this article
- Purchase on Springer Link
- Instant access to full article PDF
Prices may be subject to local taxes which are calculated during checkout
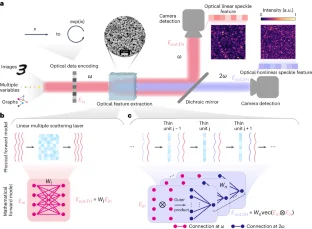
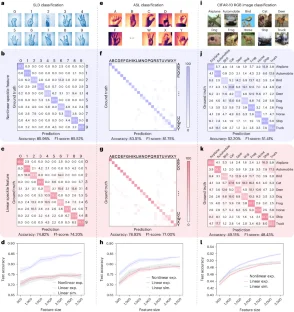

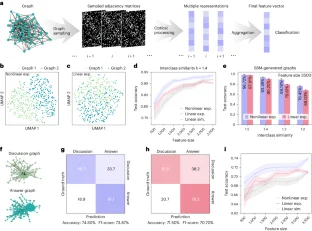
Data availability
The raw datasets before optical processing are all publicly available. Specifically, the raw data for SLDs dataset is downloaded from ref. 47, ASL alphabet dataset is downloaded at https://www.kaggle.com/datasets/datamunge/sign-language-mnist, CIFAR-10 dataset is downloaded from ref. 53, yacht hydrodynamics dataset is downloaded from ref. 56, concrete compressive strength dataset is downloaded from ref. 57, graph dataset generated by the SBM is proposed by ref. 64, Reddit-binary graph dataset is downloaded from ref. 65, MNIST dataset is downloaded from ref. 49, FashionMNIST dataset is downloaded from ref. 50 and STL-10 dataset is downloaded from ref. 54. The recorded experimental speckle feature datasets are available at https://doi.org/10.5281/zenodo.10799862 and https://doi.org/10.5281/zenodo.8392103 (refs. 71,72). Source data for Figs. 2, 3 and 4 are provided with this paper.
Code availability
The code used to produce the results within this work is openly available at https://doi.org/10.5281/zenodo.10799862 (ref. 71).
References
-
Wang, Y. E., Wei, G.-Y. & Brooks, D. Benchmarking TPU, GPU, and CPU platforms for deep learning. Preprint at arXiv https://doi.org/10.48550/arXiv.1907.10701 (2019).
-
Wright, L. G. et al. Deep physical neural networks trained with backpropagation. Nature 601, 549–555 (2022).
Google Scholar
-
Wetzstein, G. et al. Inference in artificial intelligence with deep optics and photonics. Nature 588, 39–47 (2020).
Google Scholar
-
Shastri, B. J. et al. Photonics for artificial intelligence and neuromorphic computing. Nat. Photonics 15, 102–114 (2021).
Google Scholar
-
Yao, P. et al. Fully hardware-implemented memristor convolutional neural network. Nature 577, 641–646 (2020).
Google Scholar
-
Okumura, S. et al. Nonlinear decision-making with enzymatic neural networks. Nature 610, 496–501 (2022).
Google Scholar
-
Mehonic, A. & Kenyon, A. J. Brain-inspired computing needs a master plan. Nature 604, 255–260 (2022).
Google Scholar
-
Farhat, N. H., Psaltis, D., Prata, A. & Paek, E. Optical implementation of the Hopfield model. Appl. Opt. 24, 1469–1475 (1985).
Google Scholar
-
Weaver, C. & Goodman, J. W. A technique for optically convolving two functions. Appl. Opt. 5, 1248–1249 (1966).
Google Scholar
-
Shen, Y. et al. Deep learning with coherent nanophotonic circuits. Nat. Photonics 11, 441–446 (2017).
Google Scholar
-
Tait, A. N. et al. Neuromorphic photonic networks using silicon photonic weight banks. Sci. Rep. 7, 7430 (2017).
Google Scholar
-
Feldmann, J. et al. Parallel convolutional processing using an integrated photonic tensor core. Nature 589, 52–58 (2021).
Google Scholar
-
Xu, X. et al. 11 TOPS photonic convolutional accelerator for optical neural networks. Nature 589, 44–51 (2021).
Google Scholar
-
Anderson, M. G., Ma, S.-Y., Wang, T., Wright, L. G. & McMahon, P. L. Optical transformers. Preprint at arXiv https://doi.org/10.48550/arXiv.2302.10360 (2023).
-
Lin, X. et al. All-optical machine learning using diffractive deep neural networks. Science 361, 1004–1008 (2018).
Google Scholar
-
Chang, J., Sitzmann, V., Dun, X., Heidrich, W. & Wetzstein, G. Hybrid optical-electronic convolutional neural networks with optimized diffractive optics for image classification. Sci. Rep. 8, 12324 (2018).
Google Scholar
-
Miscuglio, M. et al. Massively parallel amplitude-only fourier neural network. Optica 7, 1812–1819 (2020).
Google Scholar
-
Gigan, S. Imaging and computing with disorder. Nat. Phys. 18, 980–985 (2022).
Google Scholar
-
Saade, A. et al. in 2016 IEEE International Conference on Acoustics, Speech and Signal Processing 6215–6219 (IEEE, 2016). https://doi.org/10.1109/ICASSP.2016.7472872
-
Larger, L. et al. Photonic information processing beyond turing: an optoelectronic implementation of reservoir computing. Opt. Express 20, 3241–3249 (2012).
Google Scholar
-
Brunner, D., Soriano, M. C., Mirasso, C. R. & Fischer, I. Parallel photonic information processing at gigabyte per second data rates using transient states. Nat. Commun. 4, 1364 (2013).
Google Scholar
-
Vandoorne, K. et al. Experimental demonstration of reservoir computing on a silicon photonics chip. Nat. Commun. 5, 3541 (2014).
Google Scholar
-
Larger, L. et al. High-speed photonic reservoir computing using a time-delay-based architecture: million words per second classification. Phys. Rev. X 7, 011015 (2017).
-
Rafayelyan, M., Dong, J., Tan, Y., Krzakala, F. & Gigan, S. Large-scale optical reservoir computing for spatiotemporal chaotic systems prediction. Phys. Rev. X 10, 041037 (2020).
-
Pierangeli, D., Marcucci, G. & Conti, C. Photonic extreme learning machine by free-space optical propagation. Photonics Res. 9, 1446–1454 (2021).
Google Scholar
-
Van der Sande, G., Brunner, D. & Soriano, M. C. Advances in photonic reservoir computing. Nanophotonics 6, 561–576 (2017).
Google Scholar
-
Spall, J., Guo, X. & Lvovsky, A. I. Hybrid training of optical neural networks. Optica 9, 803–811 (2022).
Google Scholar
-
Zuo, Y. et al. All-optical neural network with nonlinear activation functions. Optica 6, 1132–1137 (2019).
Google Scholar
-
Ryou, A. et al. Free-space optical neural network based on thermal atomic nonlinearity. Photonics Res. 9, B128–B134 (2021).
Google Scholar
-
Feldmann, J., Youngblood, N., Wright, C. D., Bhaskaran, H. & Pernice, W. H. All-optical spiking neurosynaptic networks with self-learning capabilities. Nature 569, 208–214 (2019).
Google Scholar
-
Tait, A. N. et al. Silicon photonic modulator neuron. Phys. Rev. Appl. 11, 064043 (2019).
Google Scholar
-
Ashtiani, F., Geers, A. J. & Aflatouni, F. An on-chip photonic deep neural network for image classification. Nature 606, 501–506 (2022).
Google Scholar
-
Wang, T. et al. Image sensing with multilayer nonlinear optical neural networks. Nat. Photonics 17, 408–415 (2023).
Google Scholar
-
Williamson, I. A. D. et al. Reprogrammable electro-optic nonlinear activation functions for optical neural networks. IEEE J. Sel. Top. Quantum Electron. 26, 1–12 (2020).
Google Scholar
-
Li, H.-Y. S., Qiao, Y. & Psaltis, D. Optical network for real-time face recognition. Appl. Opt. 32, 5026–5035 (1993).
Google Scholar
-
Wagner, K. & Psaltis, D. Multilayer optical learning networks. Appl. Opt. 26, 5061–5076 (1987).
Google Scholar
-
Marcucci, G., Pierangeli, D. & Conti, C. Theory of neuromorphic computing by waves: machine learning by rogue waves, dispersive shocks, and solitons. Phys. Rev. Lett. 125, 093901 (2020).
Google Scholar
-
Nakajima, M., Tanaka, K. & Hashimoto, T. Neural Schrödinger equation: physical law as deep neural network. IEEE Trans. Neural Netw. Learn. Syst. 33, 2686–2700 (2021).
Google Scholar
-
Zhou, T., Scalzo, F. & Jalali, B. Nonlinear Schrödinger kernel for hardware acceleration of machine learning. J. Light. Technol. 40, 1308–1319 (2022).
Google Scholar
-
Teğin, U., Yıldırım, M., Oğuz, İ., Moser, C. & Psaltis, D. Scalable optical learning operator. Nature Comp. Sci. 1, 542–549 (2021).
Google Scholar
-
Morandi, A., Savo, R., Müller, J. S., Reichen, S. & Grange, R. Multiple scattering and random quasi-phase-matching in disordered assemblies of LiNbO3 nanocubes. ACS Photonics 9, 1882–1888 (2022).
Google Scholar
-
Savo, R. et al. Broadband Mie driven random quasi-phase-matching. Nat. Photonics 14, 740–747 (2020).
Google Scholar
-
Moon, J., Cho, Y.- C., Kang, S., Jang, M. & Choi, W. Measuring the scattering tensor of a disordered nonlinear medium. Nat. Phys. 19, 1709–1718 (2023).
-
Antonik, P., Marsal, N., Brunner, D. & Rontani, D. Human action recognition with a large-scale brain-inspired photonic computer. Nat. Mach. Intell. 1, 530–537 (2019).
Google Scholar
-
Mounaix, M. et al. Spatiotemporal coherent control of light through a multiple scattering medium with the multispectral transmission matrix. Phys. Rev. Lett. 116, 253901 (2016).
Google Scholar
-
Popoff, S. M. et al. Measuring the transmission matrix in optics: an approach to the study and control of light propagation in disordered media. Phys. Rev. Lett. 104, 100601 (2010).
Google Scholar
-
Mavi, A. A new dataset and proposed convolutional neural network architecture for classification of American Sign Language digits. Preprint at arXiv https://doi.org/10.48550/arXiv.2011.08927 (2020).
-
tecperson. Sign language MNIST. kaggle https://www.kaggle.com/datasets/datamunge/sign-language-mnist (2017).
-
LeCun, Y., Bottou, L., Bengio, Y. & Haffner, P. Gradient-based learning applied to document recognition. Proc. IEEE 86, 2278–2324 (1998).
Google Scholar
-
Xiao, H., Rasul, K. & Vollgraf, R. Fashion-MNIST: a novel image dataset for benchmarking machine learning algorithms. Preprint at arXiv https://doi.org/10.48550/arXiv.1708.07747 (2017).
-
Oguz, I. et al. Programming nonlinear propagation for efficient optical learning machines. Adv. Photonics 6, 016002–016002 (2024).
Google Scholar
-
Momeni, A. & Fleury, R. Electromagnetic wave-based extreme deep learning with nonlinear time-floquet entanglement. Nat. Commun. 13, 2651 (2022).
Google Scholar
-
Krizhevsky, A. et al. Learning multiple layers of features from tiny images (2009).
-
Coates, A., Ng, A. & Lee, H. in Proc. Fourteenth International Conference on Artificial Intelligence and Statistics 215–223 (JMLR Workshop and Conference Proc., 2011).
-
Pierangeli, D., Rafayelyan, M., Conti, C. & Gigan, S. Scalable spin-glass optical simulator. Phys. Rev. Appl. 15, 034087 (2021).
Google Scholar
-
Gerritsma, J., Onnink, R. & Versluis, A. Yacht hydrodynamics. UCI Mach. Learn. Reposit. https://doi.org/10.24432/C5XG7R(2013).
-
Yeh, I.- C. Concrete compressive strength. UCI Mach. Learn. Reposit. https://doi.org/10.24432/C5PK67 (2007).
-
Yan, X. & Han, J. in Proc. 2002 IEEE International Conference on Data Mining, 2002 721–724 (IEEE, 2002). https://doi.org/10.1109/ICDM.2002.1184038
-
Bronstein, M. M., Bruna, J., LeCun, Y., Szlam, A. & Vandergheynst, P. Geometric deep learning: going beyond euclidean data. IEEE Signal Process. Mag. 34, 18–42 (2017).
Google Scholar
-
Yanardag, P. & Vishwanathan, S. in Proc. 21th ACM SIGKDD International Conference on Knowledge Discovery and Data Mining 1365–1374 (2015). https://doi.org/10.1145/2783258.2783417
-
Shervashidze, N., Vishwanathan, S., Petri, T., Mehlhorn, K. & Borgwardt, K. in Artificial Intelligence and Statistics 488–495 (PMLR, 2009).
-
Yan, T. et al. All-optical graph representation learning using integrated diffractive photonic computing units. Sci. Adv. 8, eabn7630 (2022).
Google Scholar
-
Ghanem, H., Keriven, N. & Tremblay, N. in ICASSP 2021–2021 IEEE International Conference on Acoustics, Speech and Signal Processing 3575–3579 (IEEE, 2021). https://doi.org/10.1109/ICASSP39728.2021.9413614
-
Lee, C. & Wilkinson, D. J. A review of stochastic block models and extensions for graph clustering. Appl. Netw. Sci. 4, 1–50 (2019).
Google Scholar
-
Kersting, K., Kriege, N. M., Morris, C., Mutzel, P. & Neumann, M. Benchmark data sets for graph kernels. TU Dortmund http://graphkernels.cs.tu-dortmund.de (2016).
-
Müller, J. S., Morandi, A., Grange, R. & Savo, R. Modeling of random quasi-phase-matching in birefringent disordered media. Phys. Rev. Appl. 15, 064070 (2021).
Google Scholar
-
Ni, F., Liu, H., Zheng, Y. & Chen, X. Nonlinear harmonic wave manipulation in nonlinear scattering medium via scattering-matrix method. Adv. Photonics 5, 046010–046010 (2023).
Google Scholar
-
Hinton, G. The forward-forward algorithm: some preliminary investigations. Preprint at arXiv https://doi.org/10.48550/arXiv.2212.13345 (2022).
-
Krastanov, S. et al. Room-temperature photonic logical qubits via second-order nonlinearities. Nat. Commun. 12, 191 (2021).
Google Scholar
-
Weis, R. S. & Gaylord, T. K. Lithium niobate: summary of physical properties and crystal structure. Appl. Phys. A 37, 191–203 (1985).
Google Scholar
-
Wang, H. Nonlinear optical computing with disordered media. Zenodo https://doi.org/10.5281/zenodo.10799862 (2024).
-
Wang, H. Nonlinear optical computing with disordered media—experimental data for results in supplementary information. Zenodo https://doi.org/10.5281/zenodo.8392103 (2023).
Acknowledgements
This work was supported by the Swiss National Science Foundation projects LION, APIC (TMCG-2_213713) and grant 179099, ERC SMARTIES, European Union’s Horizon 2020 research and innovation program from the European Research Council under the Grant Agreement No. 714837 (Chi2-nanooxides), and Institut Universitaire de France. H.W. acknowledges support from China Scholarship Council. J.H. acknowledges Swiss National Science Foundation fellowship (P2ELP2_199825). R.S. acknowledges support from European Union—NextGenerationEU, project Comp-SECOONDO (MSCA_0000079).
Author information
Authors and Affiliations
Contributions
J.H. and S.G. conceived the project. H.W. and J.H. developed the experimental setup with the assistance of F.X. H.W. and J.H. performed the experiments and simulations and processed and analyzed the results. A.M., A.N., X.L., R.S. and R.G. fabricated and characterized the LN samples and provided insights of the physical model. H.W. and J.H. wrote the paper with input from all authors. S.G., R.G., Q.L. and J.H. supervised the project.
Corresponding authors
Ethics declarations
Competing interests
The authors declare no competing interests.
Peer review
Peer review information
Nature Computational Science thanks Xing Lin, Thomas van Vaerenbergh and the other, anonymous, reviewer(s) for their contribution to the peer review of this work. Peer reviewer reports are available. Primary Handling Editor: Jie Pan, in collaboration with the Nature Computational Science team.
Additional information
Publisher’s note Springer Nature remains neutral with regard to jurisdictional claims in published maps and institutional affiliations.
Supplementary information
Supplementary Information
Supplementary Notes 1–5, Figs. 1–7 and Tables 1–7.
Peer Review File
Source data
Source Data Fig. 2
Source data for plots in Fig. 2b,c,d,f,g,h,j,k,l.
Source Data Fig. 3
Source data for plots in Fig. 3b,c,d,f,g,h,j,k,l.
Source Data Fig. 4
Source data for plots in Fig. 4d,e,g,h,i.
Rights and permissions
Springer Nature or its licensor (e.g. a society or other partner) holds exclusive rights to this article under a publishing agreement with the author(s) or other rightsholder(s); author self-archiving of the accepted manuscript version of this article is solely governed by the terms of such publishing agreement and applicable law.
Reprints and permissions
About this article
Cite this article
Wang, H., Hu, J., Morandi, A. et al. Large-scale photonic computing with nonlinear disordered media.
Nat Comput Sci (2024). https://doi.org/10.1038/s43588-024-00644-1
-
Received: 06 November 2023
-
Accepted: 14 May 2024
-
Published: 14 June 2024
-
DOI: https://doi.org/10.1038/s43588-024-00644-1