This issue of Nature Computational Science features a Focus that highlights recent advancements, challenges, and opportunities in computational models for metamaterials design and manufacturing, as well as explores their potential promises in emerging information processors and computing technologies.
A metamaterial is a category of artificially structured material that is engineered to achieve properties that cannot be found in nature. Examples include mechanical metamaterials, which can exhibit a negative Poisson’s ratio — meaning that they can shrink in the direction perpendicular to a compression loading — and optical metamaterials, which can be designed to have a negative refractive index — meaning that they refract light in the direction opposite to their normal positive refractive properties. Such unique features, which are derived from specially engineered micro- or nano-scale structures, hold great potential for emerging applications across different domains, such as unconventional computing technologies, advanced imaging systems, and soft robotics.
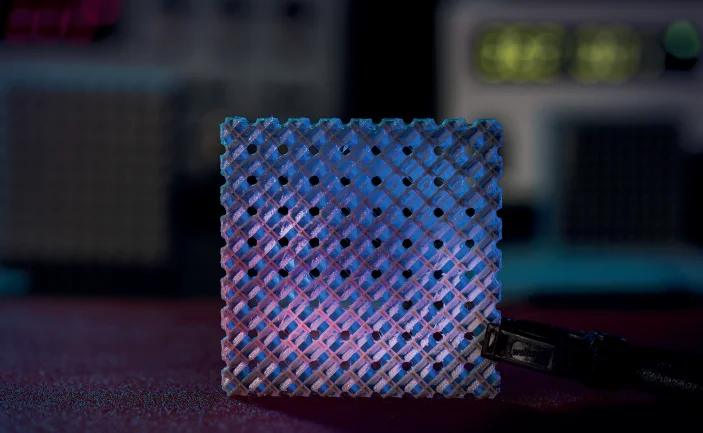
Credit: laboratory / Alamy Stock Photo
Nevertheless, the vast design space of metamaterials — given the sheer number of parameters such as geometric features, motifs, and constraints from additive manufacturing — has rendered human-guided heuristic approaches ineffective for meeting the needs of real-world applications, especially those with increasing complexity. As a result, there is a high demand in the community for advanced computational models and algorithms that can account for the various intricacies of the design process, from structural optimization to the final manufacturing stage. In order to provide the research community with a consolidated discussion on the recent developments, remaining challenges, and future opportunities in this burgeoning field, we present a Focus featuring experts’ views on the topic and on the potential applications of metamaterials to advance computing technologies, from mechanical to optical computing.
Physics-based models and methods play an important role in the design of metamaterials due to their high interpretability, as highlighted in a Q&A with Yongmin Liu, and there have been myriad developments in this direction. In a Perspective, Stefano Zapperi and colleagues provide an overview of optimization methods — more specifically, topology optimization (TO) and inverse homogenization — that have been widely used in the design of mechanical metamaterials. TO focuses on the distribution of materials to achieve desired macroscopic properties, while inverse homogenization emphasizes microstructure optimization. The authors stress the importance of integrating macro- and micro-structural optimization to enable the search for optimal materials across multiple length scales. Gary P. T. Choi explores in a Comment the recent developments in mathematical models and algorithms that help to build complex metamaterial structures based on origami and kirigami patterns, which are inspired by traditional paper folding and cutting art.
While physics-based models have been used to successfully navigate the extensive design space of metamaterials, they are often computationally expensive. As a result, it is challenging to apply these models to metamaterials with increasing complexity that may arise from intrinsic properties — such as complex three-dimensional structures, as highlighted by Liu — or from manufacturing constraints in the additive manufacturing (AM) process, including processing conditions and parameters, as noted by Zapperi and colleagues and in a Comment by Keith Brown and Grace X. Gu.
In order to overcome the challenges faced by physics-based models, machine learning (ML) and data-driven methods have emerged as effective alternatives for metamaterials design. Zapperi and colleagues state that ML-based methods can enhance conventional optimization approaches, for instance by reducing the number of iterations needed to obtain a final design and by replacing expensive numerical simulations. Brown and Gu argue that ML models, particularly multi-fidelity models, show promise in effectively integrating architectural design and AM process parameters. In addition, they highlight the potential of ML in self-driving labs, which can automate the design, manufacturing, and validation processes. When it comes to art-inspired metamaterial design, although the application of ML has been primarily focused on kirigami patterns, Choi points out that ML approaches can effectively handle the increased complexities of folds, cuts, and pattern geometries in both origami and kirigami metamaterials. Similarly, Liu brings up that ML can accelerate meta-optics design, for instance by bypassing expensive simulations.
Due to their unique and attractive properties, advanced metamaterials have found many potential applications. Notably, metamaterials can aid in the design of new computing technologies, enabling capabilities beyond those of conventional digital computers. In a Perspective, Jordan R. Raney and colleagues argue that programmable metamaterials — meaning, metamaterials with programmable shape changes and tunable mechanical properties — can be used in robotics to enable distributed intelligence, facilitating fast and efficient information processing. This approach can also address other limitations, such as poor scalability and vulnerability to harsh environments, which are often associated with centralized information processing in current electronic systems. Metamaterials and metasurfaces can also play an important role in optical computing, as highlighted by Yandong Li and Francesco Monticone in a Comment: these materials can offer high design flexibility and spatial parallelism, easy integration into more compact systems, and reduced latency, thus leading to improvements in energy efficiency, throughput, computing speed, and scalability compared to their digital counterparts. The success of such approaches is also demonstrated in a recent work (E. Choi et al. Nat. Photon. 18, 848–855; 2024) — which we discuss in a Research Highlight — where a computationally designed metasurface enabled 360° structured light, substantially enhancing performance in depth reconstruction for 3D imaging. Another noteworthy application — the concept of photonics for artificial intelligence — was underscored by Liu: this entails using optical metamaterials to build photonic neural networks and neuromorphic computing infrastructures, which has the potential to achieve excellent computational and energy efficiency, outperforming digital counterparts in various ML tasks.
Undoubtedly, there are still many remaining challenges to be addressed when it comes to the effective computational design of metamaterials. For instance, ML-based metamaterial models face several issues, such as data scarcity, low data quality, and the lack of generic computational software systems suitable for practical and industrial applications. Advancing this field requires a collaborative effort within the community to build better data infrastructure for data sharing, as highlighted by Liu, and to develop multi-fidelity models capable of handling both low- and high-quality data. With this Focus, we hope to inspire new ideas, foster further discussions, and encourage collaborations within the broader computational science and materials science communities.
Rights and permissions
Reprints and permissions
About this article
Cite this article
Metamaterials design via and for computation.
Nat Comput Sci 4, 543–544 (2024). https://doi.org/10.1038/s43588-024-00687-4
-
Published: 27 August 2024
-
Issue Date: August 2024
-
DOI: https://doi.org/10.1038/s43588-024-00687-4