Abstract
A reduction in animal-based diets has driven market demand for alternative meat products, currently raising a new generation of plant-based meat alternatives (PBMAs). It remains unclear whether these substitutes are a short-lived trend or become established in the long term. Over the last few years, the trend of increasing sales and diversifying product range has continued, but publication activities in this field are currently limited mainly to market research and food technology topics. As their popularity increases, questions emerge about the safety and nutritional risks of these novel products. Even though all the examined products must be heated before consumption, consumers lack experience with this type of product and thus further research into product safety, is desirable. To consider these issues, we examined 32 PBMAs from Austrian supermarkets. Based on 16S rRNA gene amplicon sequencing, the majority of the products were dominated by lactic acid bacteria (either Leuconostoc or Latilactobacillus), and generally had low alpha diversity. Pseudomonadota (like Pseudomonas and Shewanella) dominated the other part of the products. In addition to LABs, a high diversity of different Bacillus, but also some Enterobacteriaceae and potentially pathogenic species were isolated with the culturing approach. We assume that especially the dominance of heterofermentative LABs has high relevance for the product stability and quality with the potential to increase shelf life of the products. The number of isolated Enterobacteriaceae and potential pathogens were low, but they still demonstrated that these products are suitable for their presence.
Similar content being viewed by others
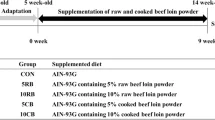
Comparative analysis of the gut microbiota of mice fed a diet supplemented with raw and cooked beef loin powder
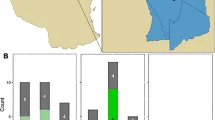
Microbial Diversity in Bushmeat Samples Recovered from the Serengeti Ecosystem in Tanzania
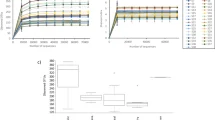
Comparison of the microbial composition of African fermented foods using amplicon sequencing
Introduction
For most of the populations in industrialized countries, meat consumption is an integral part of the diet. In 2020, the average U.S. American and European Union citizens consumed 102 and 69 kg of meat per capita, respectively1,2. The global meat consumption increased from 24 kg per year and capita in 1990 to 34 kg in 20202,3. Although the OECD (Organisation for Economic Co-operation and Development) estimates that consumption will level off at around 35 kg per year and capita by 2030, the total meat consumption will further increase with population growth3. This globally growing meat consumption plays a major role in the ecological issues we currently face, including land degradation, climate change, water pollution and loss of biodiversity4,5. Additionally, common industrial animal husbandry practices influence public health by facilitating the spread of antimicrobial resistances and vector-borne diseases5,6,7. Transition to plant-based diets has been identified as an effective way to tackle some of these food system’s challenges8. Food production needs to become a net carbon sink from 2040 onwards to reach the goal of global negative emissions9. The Paris Agreement predicted that the most needed changes include at least doubling the consumption of plant-based foods, including fruits, vegetables, legumes and nuts, and halving the consumption of red meat10. Consumers’ acceptance of the transition to a more plant-based diet is steadily growing11,12, and health benefits, including a lowered risk of type 2 diabetes, cardiovascular diseases and metabolic syndrome, are widely recognized13. In a 2021 survey conducted in ten different European countries, 2% of the participants referred to themselves as vegans, 5% as vegetarians, 3% as pescetarians and 30% as flexitarians14. The last group’s eating habits are focused on plant foods with the occasional inclusion of meat products. As the main target group, flexitarians account for about 90% of the sales of plant-based meat alternatives (PBMAs)15. Market research conducted as part of “The Smart Protein Project” noted a sales value increase of 82% for plant-based meat (vegan and vegetarian) between 2018 and 2020 for Austria16. The sales of this product group and the number of different products have increased strongly over the last few years16,17. Similar trends are recognized in other countries of the European Union16. Given the surging popularity of PBMAs, it is imperative to acknowledge the inherent product-specific hazards associated with their consumption. These hazards vary with the composition of ingredients, and encompass a range of contaminants including pesticides, mycotoxins, toxicologically significant plant compounds, heavy metals, and mineral oil hydrocarbons18,19,20. Despite the potential risks posed by these contaminants, research on this subject has been relatively limited. However, there is a shifting trend, with an increasing number of studies now addressing these concerns. Just recently, Mihalache et al. demonstrated the presence of significant levels of mycotoxins in the majority of the plant-based meat alternatives they analyzed. Although legal limits exist for these contaminants, they are deemed inadequate as they fail to encompass all pertinent mycotoxins and do not adequately consider current consumer behaviors21. Furthermore, the microbiological properties of these products have been similarly little studied to date, resulting in a corresponding lack of detailed data. This gap is particularly notable for PBMAs not classified as ready-to-eat foods, for which specific legal standards or microbiological safety criteria have yet to be established. This scenario underscores the need for enhanced research efforts to elucidate the microbiological risk profile of PBMAs, thereby informing the development of targeted safety regulations and recommendations, but also for addressing sustainability questions. Since two of the UN’s sustainability goals (goal 2 – zero hunger and 12 – responsible consumption and production) affect our eating habits, increased attention should be paid to reducing food loss and waste. A solid concept for preventing food loss and waste conveys multiple benefits: it saves food for human consumption, brings savings for primary producers, companies and consumers, as well as lowering the environmental and climate impact of food production and consumption. As 30% of food products in primary processing do not even reach the consumer, mainly because of microbial spoilage or pathogen contamination, it is essential to improve the knowledge of the microbial communities of our food, which could help to increase the shelf life, reduce the contamination with pathogens, but also to optimize packaging conditions which should be tailored for the suppression of product-specific microbiota. Still largely unanswered is the question if and how a high microbial diversity in food (consisting of living and dead microbiota) can have a positive effect on consumer’s health. It was also supposed recently that the loss of microbial diversity including the disappearance of ancestral indigenous microbiota, which is currently happening in western countries, affects human health and contributes to post-modern conditions such as obesity and asthma22,23. For all these research questions, fundamental knowledge on the microbial compositions on food are necessary, but still lacking. With limited knowledge regarding the microbiological properties of these products, we collected a variety of PBMAs from Austrian supermarkets to investigate their overall microbial community compositions. Additionally, we aimed to characterize the microbial profiles and compare four distinct groups of the most prevalent product types (pea and soybean based products with either “minced” (minced meat, burgers, etc.) or “fibrous” (meat chunks, fillet-like, etc.) texture). We hypothesized that products within one group have more similar communities than between groups, as a result of similar protein processing.
Results
Product descriptions
In total, 32 PBMA products from four groups (pea or soybean-based and minced or fibrous) were collected and summarized in Table 1. Beside these four main properties, the products were quite diverse in terms of composition and handling procedures. Particularly noteworthy was the number of ingredients used per product, ranging from 4 to 27 ingredients per product, resulting in a total of about 120 different ingredients overall (Table 1). Additionally, there was a wide range of shelf life, spanning from products with an expiry date to be consumed within a few days, to products with a best-before date, offering a shelf life of several months. The products were also different on factors that might influence the bacterial composition such as pre-heating or freezing steps or packing in modified atmosphere (MAP). Most of the products had clear cooking instructions on the labels, including the recommendation for thorough cooking (Table 1). In total, 27 samples were packed under modified atmosphere with unknown composition. Thirty samples were sold refrigerated, the other two were frozen. Additionally, according to the label, 7 of the 30 refrigerated products were frozen at any time point during the retail chain (Table 1).
Cultivable microbial communities in PBMA products
In total, 470 colonies were picked and selected for 16S rRNA gene or ITS2 region (ITS3/ITS4) Sanger sequencing. Among these, 447 (95.1%) were classified as bacteria. Of those isolates 431 (91.7% overall) were successfully classified to genus level, representing 38 genera from four different phyla (Fig. 1). Isolates classified as Enterobacteriaceae (n = 16, 3.4%) failed to reveal taxonomic classification below family level. The twenty fungal isolates (4.3% of all isolates) were classified into five different genera: Wickerhamomyces (n = 7), Pichia (n = 6), Yarrowia (n = 3), Kurtzmaniella (n = 3), Geotrichum (n = 1). Three isolates failed to retrieve any taxonomic assignment. In the initial cultivation trial, we successfully isolated most of the targeted genera. Subsequently, in the second round of cultivation, we were able to isolate Brochothrix, Weissella, and Psychrobacter. Despite their high relative abundances in the 16S rRNA amplicon sequencing data, Myroides, Pediococcus, Xanthomonas, and Shewanella remained elusive and could not be isolated from any of the samples. We found Bacillus, Leuconostoc, Enterococcus and Latilactobacillus very prevalent in PBMA samples, as they were isolated from 19 (59.4% from the total number of samples), 18 (56.3%), 12 (37.5%) and 10 (31.3%) samples, respectively. Moreover, species from Bacillus, Leuconostoc and Latilactobacillus were isolated from each of the four sample groups. Enterobacteriaceae, which are usually surveyed as an additional hygiene criterion, were only found in the pea protein products of a single manufacturer (Fig. 1). A selection of these Enterobacteriaceae, of the dominant lactic acid bacteria and potential pathogens as classified by the RDP Classifier (i.e. Staphylococcus aureus, Bacillus cereus group, Klebsiella sp.) were subjected to long-read WGS (Table 2, see next results section). In addition to the classical microbiology and sequencing analyses, we performed qPCR to quantify microbial DNA in the PBMA products. Given the methodical challenges and the heterogeneity of the sample types, we have detailed these qPCR results and the associated methodological considerations in the supplementary materials (Supplementary Notes 1).
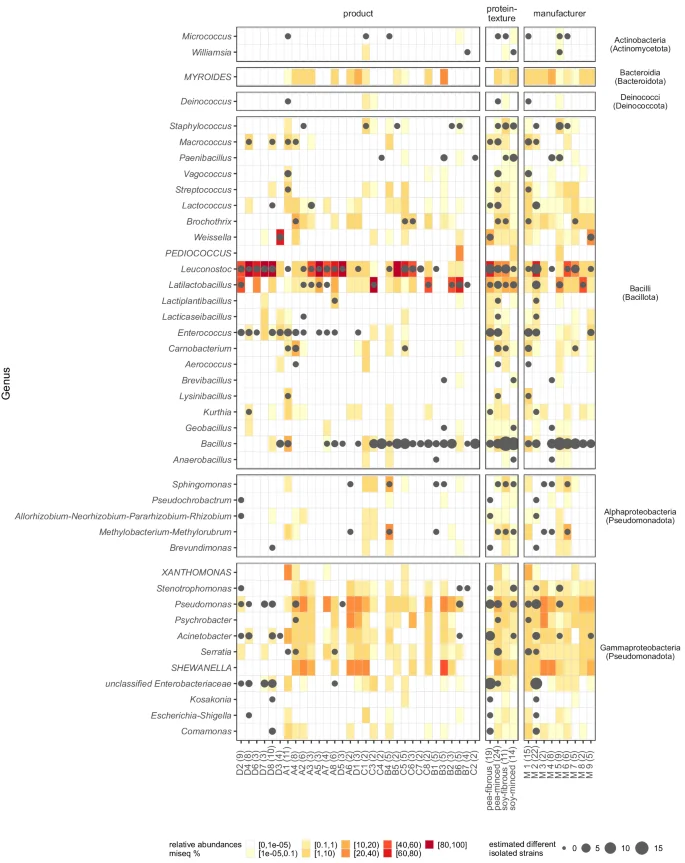
The 16S rRNA gene sequences of isolates belonging to a particular genus were aligned to each other, and were clustered based on their sequence similarity. Consequently, different clusters represents different strains or species. The higher the number of clusters within a genus, the larger the plotted dot in the figure. The surrounding area is shaded according to the relative abundances in the 16S rRNA gene amplicon high-throughput sequencing (for the group and manufacturer summary, the mean relative abundance of each included sample is used). Additionally, genera, that were highly abundant in some samples, but had no corresponding isolates, were added to the figure denoted capitalized letters.
Lactic acid bacteria and Gammaproteobacteria dominate PBMA products
In total, 27 samples (883,427 sequences; median frequency per sample: 26,083) sequenced by 16S rRNA gene amplicon HTS surpassed the quality criteria and proceeded to further downstream analysis. ASVs were assigned to 25 different phyla. Three of the samples showed > 3% relative abundance of at least one phylum (i.e. Bacillota 0.00-94.74%, Pseudomonadota 0.00–30.85%, Bacteroidota 0.00–10.84%). In total, 18 samples were dominated by Bacillota (10 samples with > 90% abundance) and the other 9 were dominated by Pseudomonadota. The most common genera were Leuconostoc (detected in 25 samples; ranging 0.03–100.00% relative abundance in the sample), Latilactobacillus (21 samples; 0.02-86.38%), Pseudomonas (20 samples; 0.36–35.25%), Serratia (19 samples; 0.03–8.92%), and Acinetobacter (17 samples; 0.09–15.40%). Leuconostoc was the most abundant genus in 13 samples, followed by Latilactobacillus (4 products), and Shewanella (4 products) (Fig. 2). Strikingly, some genera that showed a high relative abundance ( > 10%) in one or more sample were not able to be isolated by culture-dependent methods (i.e. Shewanella, Xanthomonas, Photobacterium, Myroides, Pediococcus).
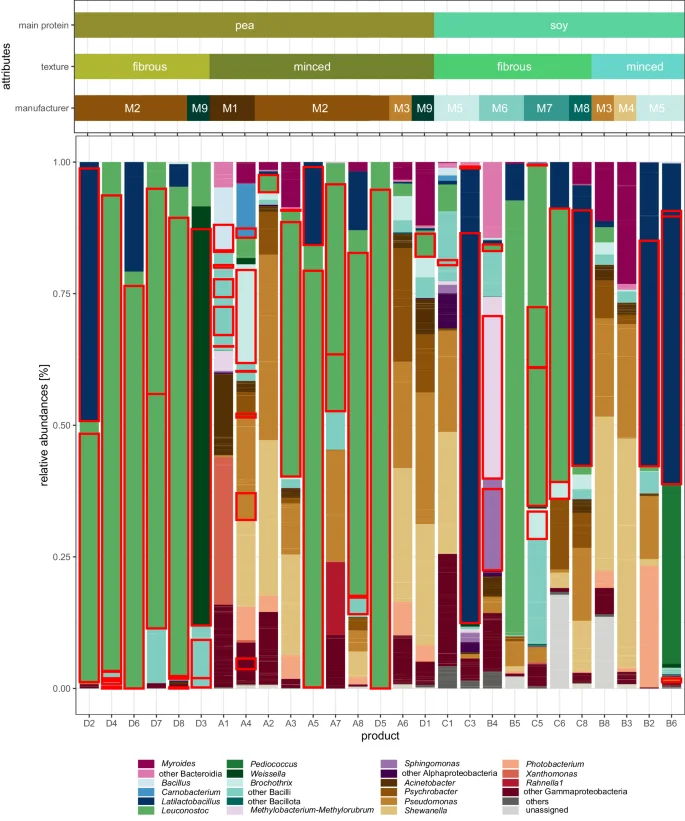
The underlying relative frequencies of the ASVs are recognizable as pale yellow lines within a genus. Genera with a maximum value of 10% across all samples were subsumed by color in the next higher taxonomic level. The samples are ordered by main protein source, texture and manufacturer. ASVs with matching isolates ( > 99% identity), were outlined in red.
The tSNE plot clearly showed three distinct clusters (Fig. 3a), which we described based on the most abundant taxa as Leuconostocaceae-, Latilactobacillus– and Pseudomonadota-profiles. The clustering was comprehensible, when comparing the similarity of the relative abundance patterns of the samples within each cluster. However, there was no clear separation based on the examined variables (main protein source, status, manufacturer). A Kruskal-Wallis test comparing the samples of the three clusters showed a significant difference for Hill-Simpson (p < 0.01) and Hill-Shannon (p < 0.01) diversity (Fig. 3b, c).
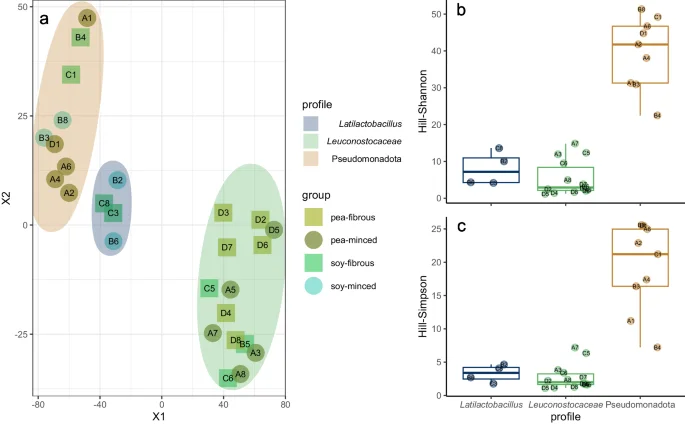
a tSNE plot clustered samples to different profiles based on Bray-Curtis dissimilarity (Similar clusters were also found with other distance matrices – see Supplementary Fig. 2). b Hill-Shannon diversity and c: Hill-Simpson diversity comparing these profiles. Box plots show the median with hinges that correspond to the 25th and 75th percentiles. The whiskers extend from the hinge to the largest and smallest value no further than 1.5 multiplied by the inter-quartile range. Individual data points are overlaid.
Protein source and texture are not the main drivers for the microbial community pattern
The Kruskal-Wallis test comparing the Hill-Shannon index between the four groups (based on proteins source and texture) was significant (p-value = 0.02), while there was no significant difference comparing the group-wise Hill-Simpson index (p = 0.05). Post-hoc Dunn’s testing with Bonferroni alpha adjustment showed that when comparing group-wise Hill-Shannon index only the groups “pea-fibrous” and “pea-minced” differed significantly (p = 0.02 – Supplementary Fig. 1). The group dispersions were homogenous in all examined distance methods (i.e. Bray-Curtis, Jaccard, JSD). The PERMANOVA showed that texture and protein source significantly affected the microbial composition (Supplementary Table 1), but explained only between 15.94% and 23.44% of the total variance. The variance explanation by the PERMANOVA would increase, if the manufacturer as variable was added to the model, but as the sampling was very unbalanced, it was renounced. LEfSe identified several predictive characteristic genomic features for product categories with a log10 LDA score > 4.0. For “pea-fibrous” products the occurrence and abundance of Leuconostoc and Enterococcus were characteristic as well as the corresponding superordinate phylogeny levels. For “pea-minced” products it was mainly Pseudomonas. In “soy-fibrous” products two low abundant orders (i.e. Sphingomonadales and Burkholderiales) had a log10 LDA score > 4.0. The orders Enterobacterales and Flavobacteriales (Phylum Bacteroidota) were identified to explain microbial characteristics in this group (Fig. 4).
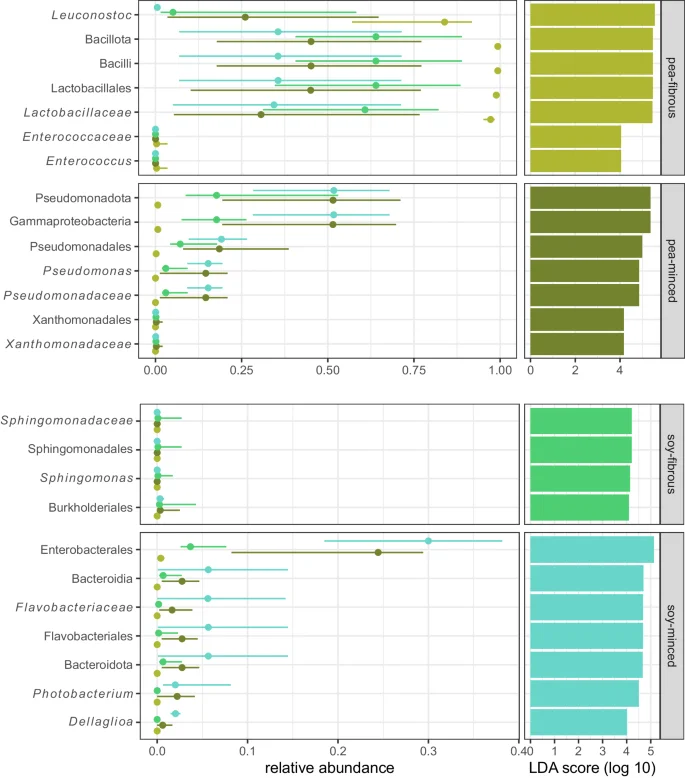
Additionally illustrated are the medians of the relative abundances as a point, with lines representing the first and third quartiles. Additionally, LEfSe per community profiles are displayed in Supplementary Fig. 3.
Genomic features of isolates reveal potential microbiological risk
Most of the sequenced Enterobacteriaceae were described as established human pathogens according to Bartlett et al.24. Among these Escherichia coli was the only species, associated with foodborne diseases. The genome of the isolated E. coli strain revealed several virulence factors and antimicrobial resistance genes (AMRG) according to VFDB and CARD databases, respectively (Supplementary Table 2). This includes virulence factors like fimH (Type 1 fimbriae) and fyuA (ferric yersiniabactin uptake), which are associated with Adherent-Invasive E. coli (AIEC)25, or AMRG such as mdfA (associated with multidrug resistance) or ampH (beta-lactamase resistance). The three Klebsiella isolates belonged to the K. oxytoca species complex (KoSC) and were classified as K. oxytoca, K. grimontii and K.pasteurii. Different class A beta-lactamase genes were detected in the isolates, including blaOXY-2 in K. oxytoca, blaOXY-6 in K. grimontii and blaOXY-4 in K. pasteurii. Extended spectrum beta-lactamase (ESBL)- and carbapenemase-producing isolates of this species complex are associated with nosocomial infections26. The other Enterobacteriaceae isolates were classified as Citrobacter braakii, Leclercia adecarboxylata and Lelliottia amnigena A. Within the Bacillus genus, the 13 sequenced isolates were classified as B. licheniformis (n = 4), B. pumilus (n = 3), B. paralicheniformis (n = 1), B. subtilis (n = 1), B. velezensis (n = 1) and B. paranthracis (n = 3). Since B. paranthracis can cause foodborne illnesses27, their genomes were additionally analyzed with BTyper3 (Supplementary Table 2), which predicted the presence of the relevant non-hemolytic enterotoxin (Nhe) complex, which is associated with diarrhea, as well as other virulence factors. The Staphyloccous aureus genome harbored several virulence genes (Supplementary Table 2). The analysis of the lactic acid bacteria focused on spoilage potential (enzymatic repertoire for metabolite production) and preservative aspects. The sequenced Leuconostoc and Latilactobacillus isolates possessed genes to produce metabolites associated with meat spoilage, like butane-2,3-dione (diacetyl), 3-hydroxybutan-2-one (acetoin), 2-hydroxypropanoate (lactate), acetate and ethanol. One of the Ln. mesenteroides isolates harbored genes for a leucocin A/sakacin P family class II bacteriocin and the associated relevant transport proteins, while in L. sakei isolates only the transporter genes were detectable (antiSMASH hits and belonging blastp best hits in Supplementary Table 2). Ln. mesenteroides showed greater abilities for carbohydrate utilization in terms of glycoside hydrolases (26 GHs) in comparison to L. sakei (14 GHs). Further, Ln. mesenteroides harbored genes coding for enzymes in charge of synthesizing a broad range of amino acids (i.e. Threonine, Cysteine, Methionine, Valin, Isoleucin, Leucin, Arginin, Tryptophane) and menaquinone (Supplementary Table 2).
Discussion
There is a lack of microbiological investigations for novel PBMAs, and although nutritional consequences, sustainability gains and sociotechnological pathways for transition to meat alternatives have been extensively reviewed (e.g. van der Weele et al. 201928), currently microbiology, shelf-life optimization and risk assessments are currently lagging behind, considering the high variety of novel products in this category. Highly processed food, like the PBMAs examined in this study, present challenges to microbiologists. The broad range of ingredients (~120 in the 32 selected products), different processing steps, and a variety of production equipment may lead to many potential sources for spurious bacterial contamination. The product specific production processes were not available, but up to the present low (LMEC) and high moisture extrusion cooking (HMEC) are the most common commercially used technologies to produce meat textured plant proteins29. Both methods are based on an interaction of heat, shear force and pressure, but the conditions for the extrusion process depend on the original protein source and the desired final protein structure30,31,32. Looking at the survival abilities of microorganisms throughout this process, the crucial factors are temperature, pressure, and time. These variables are closely tied to the process configurations; hence, the following can merely serve as general guide. Specifically, within HMEC, the extruder barrels feature distinct temperature zones, with the high-temperature melting zone reaching approximately 150–170 °C33. However, the temperature at the extruder die is notably lower, being around 15–25 °C cooler than the barrel34. The barrel temperature for LMEC is general lower with 120–150 °C35. The pressures in these barrels is between 1 and 4 MPa in HMEC and up to 13 MPa in LMEC35,36,37. Yu et al.38 showed in different LMEC settings fastest particle residence times (first signs of the tracer) from 10 to 40 s and extrudate collection times (complete tracer passed) from 60 to 120 s, resulting in mean residence times of 35–87 s, depending on feed moisture, screw speed and die diameter. So far it is described that vegetative forms of bacteria are inactivated effectively by this process and only spores of bacteria like Bacilli and Clostridia would survive the extrusion process39,40. However, reactivation of spores during further processing is possible41. This could be one explanation why Bacillus was isolated from most of the samples (19/32), while the relative abundance of 16S rRNA gene DNA is relatively low, compared to other genera. Based on our results and the initial extrusion procedure, we can infer that the main protein would not be the primary source for the majority of the living bacterial cells we isolated from the products. The most likely causes of contamination are the addition of ingredients, specifically spices and herbs42, as well as the production environment, which includes the selection of operational equipment and handling procedures. In this context, future studies must focus on examining raw materials and production environments to confirm these findings and to adjust the production towards higher security and safety.
Based on the microbial distribution patterns from the 16S rRNA gene amplicon HTS data, we roughly can define three different bacterial community profiles. Lactic acid bacteria (LAB) dominated two of them (14/27 Leuconostocaceae dominated samples, 4/27 Latilactobacillus dominated samples). Interestingly, the majority of these samples, were dominated by one single ASV of these genera, which corresponded with the ones isolated. Based on WGS, the LAB representative isolates were classified as Ln. mesenteroides, Ln. citreum and L. sakei. Ln. mesenteroides and Ln. citreum are usually found in plants and plant-based foods43. Ln. mesenteroides is an important fermenter for products like kimchi, sauerkraut44, and soybean paste45. Additionally, they have shown antimicrobial activities against pathogenic bacteria, such as Salmonella enterica subsp. enterica serovar Typhimurium and Listeria monocytogenes46,47. On the other hand, Ln. mesenteroides has been also associated with meat spoilage, and the production of off-odors, off-flavours, slime and gas production48,49,50, particularly in combination with modified atmosphere packaging and cold storage51,52,53. Similar properties were attributed to L. sakei. It was described as fermenters particularly for sausages54, and vegetable products55, as producer of bacteriocins56, and as probiotics57. The detection of genes associated with the production of metabolites such as butane-2,3-dione (diacetyl), 3-hydroxybutan-2-one (acetoin), 2-hydroxypropanoate (lactate), acetate, and ethanol in the sequenced Leuconostoc and Latilactobacillus isolates might have important implications in the final organoleptic properties of this specific food products. Diacetyl and acetoin are volatile compounds that contribute to off-flavors and off-aromas in food products like meat when present in high concentrations. For example, these compounds impart buttery or metallic flavors and are often associated with undesirable sensory characteristics in spoiled meats58. The production of lactate, acetate, and ethanol indicates metabolic activities and also leads to changes in pH, acidity, and alcohol content59. These alterations affect the sensory attributes and quality, all potentially leading to spoilage. Metabolites produced by Leuconostoc and Latilactobacillus isolates, particularly diacetyl and acetoin, are also known to accelerate the deterioration of meat products, contributing to a shortened shelf life and reduced consumer acceptability, what could also be true for PBMAs, although further research is needed.
For kimchi and other plant products is described, that Leuconostoc, Enterococcus and Lactococcus act as initial colonizers, succeeded by Lactobacillus, Pediococcus and Weissella43. This can be explained by a better adaptability to environmental conditions (e.g. through a large number of accessory genes), but also by the already high population density on the ingredients, while in later stages decreasing pH values inhibits Leuconostoc species, which are sensitive to acids43. However, the examined products were very diverse in their shelf life, so it was difficult to conclude, whether Latilactobacillus dominated samples, were in a later stage of shelf life than the Leuconostoc dominated samples. In vegan meat alternatives, the drop in pH should not be comparable to kimchi (pH 4.0)60. It ranks in the range of 5.4–6.661,62, and thus should not favor L. sakei. In general, in both species there are strains able to grow at 4 °C51,55,63, although Ln. mesenteroides strains examined by Comi and Iacumin grew faster at 4 °C than L. sakei64. Leuconostoc spp., among these Ln. mesenteroides and Ln. citreum are described to inhibit the growth of L. sakei strains65, but it was also demonstrated, that L. sakei is able to inhibit the growth of Ln. mesenteroides, when they were inoculated equally to cooked bacon66. However, L.sakei is highly adapted to protein rich meat and fish environments and so has reduced abilities for amino acid biosynthesis67. The L. sakei isolates from this study lack eight important amino acid biosynthesis pathways. However, these pathways were present in the Ln. mesenteroides isolates. Since most of these amino acids have lower concentrations in plant proteins compared to muscle proteins68, Ln. mesenteroides might have some growth advantages in PBMAs. Apart from this, the investigated Ln. mesenteroides isolates had more genes associated to the utilization of different carbohydrate sources (i.e. maltose, dextrins, isomaltose), compared to L. sakei (26 vs. 14 GHs). Additionally, the examined Ln. leuconostoc isolate had the ability to synthesise menaquinone (vitamin K2), which may mean better handling of oxidative and other environmental stressors and an increase in growth rate69,70.
Overall, we examined nine samples with high relative abundances of Pseudomonadota, mainly Pseudomonas, Psychrobacter and Shewanella. These three genera were described as common spoilers in meat and fish products71. Plant-based meat products are usually slightly higher in pH than their animal-derived counterparts62. Among the genera found in the 16S rRNA gene amplicon HTS, at least 13 of them are described as biofilm builders in food processing environments72, including Pseudomonas, Psychrobacter and Shewanella. The possibility of biofilm formation on the processing equipment is high, given that many of the machines used are difficult to clean. However, this notion is inconsistent with the fact that among the nine Pseudomonadota-dominated samples, seven exhibit very similar pattern, despite originating from six different producers. To our knowledge, there are no available studies on the microbial communities of raw soybean or pea proteins, but 16S rRNA gene patterns of peas or the phyllosphere of soy do not support the hypothesis that this kind of contamination is associated with the main protein source. Only sample B4, which is the sole sample dominated by Alphaproteobacteria, had high similarities to the relative abundances of the microbial community of the soy phyllosphere73. However, some of the producers just have a few products in this food segment, what could imply that they purchase already extruded proteins from a large distributor for use in their products. We assume that bacteria, which are metabolically active at some point during the process, contribute to the development of (off-)odors and (off-)tastes, and on the other hand, can provide information on whether there are contamination inputs from the ingredients or process areas that could be considered in the HACCP concept in the longer term (e.g. preventing biofilm formations).
Beside the dominant, spoilage associated genera, we also isolated different Enterobacteriaceae members including Leclercia, Atlantibacter, Citrobacter, Escherichia and Klebsiella. This goes in line with previous studies that showed the ability of certain bacteria to grow in vegan burgers74. The presence of Enterobacteriaceae in food samples is a hygiene indicator and usually associated with human handling and poor hygienic conditions. In this study, all Enterobacteriaceae isolates originated from products of the same manufacturer. 16S rRNA gene amplicon HTS revealed very low levels of Enterobacteriaceae in all products, suggesting that the heating processes during production effectively killed Enterobacteriaceae in these products, as previously reported74. All products were labelled with cooking instructions: most of them specifying cooking time in minutes, some only showing “heat through before consumption”. This final heating step by the consumer is considered as part of the HACCP concept of the manufacturer. While cooking time in minutes is a good guidance for the consumers, “heat through” is in our opinion too imprecise. First, there is a lack of experience with these kind of products, second in contrast to animal meat (products) there is no indicator like color change to determine a sufficiently cooked state. However, lack of experience with these products is not limited to preparation, but also to spoilage detection, handling of the raw product and shelf life or storage before and after preparation. In total, nine out of 32 samples had an expiry date, the others a best before date. Although the best-before date on these products is to be welcomed for reasons of sustainability, it is understandable that consumers are more inclined to discard the products once this date has passed, as they do not trust themselves to make an assessment. The odor of the examined products was not comparable to a corresponding meat product. Most of the products in this study were “ready-to-heat”, so there is no need for handling before the heating step. Additional preparation steps are most likely for vegan mince (mixing and forming steps). In this case, the same kind of kitchen hygiene is appropriate as is recommended for raw meat. Toth et al. concluded in their study that dishes with vegan meat substitutes spoiled faster than their meat counterparts when stored after preparation62. These findings, together with the generally higher refrigerator temperatures than recommended75, once again show the importance of increasing consumer awareness of food handling and storage. This is especially true, considering the potential pathogens isolated from the products. Taking into account the potential pathogens identified in the products, our research demonstrates that the detected E. coli strain carries virulence factors and antimicrobial resistance genes linked to AIEC, suggesting their potential to cause foodborne illnesses. For instance, the presence of fimH, responsible for encoding the FimH protein involved in bacterial adhesion, underscores the strain’s ability to adhere to host cells, facilitating infection establishment and biofilm formation76. Additionally, one strain harbored the multidrug resistance related gene mdfA, which might enhance the bacterium’s ability to withstand various antimicrobial agents77. Furthermore, the identification of ampH associated with resistance to beta-lactam antibiotics might limit treatment options in the case of potential colonization78. In summary, the presence of these virulence and antimicrobial resistance genes suggests that E. coli strains found in plant-based meat alternatives may possess heightened adhesive capabilities, increased survival mechanisms, resistance to multiple drugs, and resistance to specific classes of antibiotics, potentially posing challenges for infection treatment. Our detection of class A beta-lactamase genes, like blaOXY-2 in Klebsiella oxytoca, blaOXY-6 in K. grimontii, and blaOXY-4 in K. pasteurii, holds significant medical implications. These genes indicate the genetic capacity of the respective Klebsiella species to produce enzymes that can hydrolyze and inactivate beta-lactam antibiotics, such as penicillins and cephalosporins. This resistance mechanism poses a considerable challenge in treating infections caused by these bacteria, as beta-lactams are among the most used and prescribed antibiotics in clinical practice79. Consequently, healthcare providers are increasingly considering alternative antibiotic regimens or tailored therapies based on antimicrobial susceptibility testing results to ensure effective treatment80. The Staphylococcus aureus genome harbored several AMRG, such as norA (associated with resistance to quinolones) and tet(38) associated with resistance to tetracyclines), that contribute to their ability to survive in the presence of antimicrobial agents81,82. Other AMRG, such as mepA and lmrS, were also found in the S. aureus genomes, and have been previously reported to be part of the host innate immune defenses83,84. The targeted WGS analysis performed here gave a first direct hint to the potential of some PBMA products for causing foodborne diseases.
Regarding the potential drivers of microbial composition in PBMAs we showed that main protein source and texture were significant variables in the PERMANOVA, but they only explained about 18% of the model’s variance. Adding the manufacturer as additional variable to the PERMANOVA increased the explained variance to 53%. Even if our sample selection was too unbalanced in terms of manufacturers to be able to make valid statistic statements we assume that the production plant has an non-negligible effect on the product’s microbial community. The common perception of the public that plant-based is inherently healthy, must be seen critically. As with any other food product, the macro-nutrient profile and the level of processing are important factors to be considered when determining how healthy a product is. It’s important for producers to label carefully and with accessible wording (as explanations are read by the general public), so that consumers can understand what they are purchasing and consuming. However, balancing plant-based meat alternatives with whole, minimally processed foods like vegetables, fruits, grains, nuts, and legumes optimizes nutritional value, and this message should be communicated as well.
A combination of culture techniques and various sequencing methods served to provide insights into the microbiology of the product group of PBMAs that were hardly available until now. The results show a clear dominance of lactic acid bacteria (i.e. Leuconostoc mesenteroides and Latilactobacillus sakei) in most of the sampled products, whose genetic profile suggests that they are the main culprits for the spoilage of these products. In the long term, a more detailed study of these species on these products could lead to an improvement in shelf life. In addition, several species were isolated that may be associated with foodborne illnesses (e.g. non-hemolytic enterotoxin genes possessing Bacillus paranthracis, Staphylococcus aureus, Escherichia coli). The presence of these species in this relatively small set of samples shows that this product group may well serve as a medium for foodborne pathogens if kitchen hygiene is lacking or recommended heating steps are missing, imprecisely formulated, or overlooked. Further, targeted investigations of larger samples groups would be valuable.
Methods
Sample acquisition
We purchased 32 different PBMAs, between July 12 and July 14, 2021, from four leading supermarket chains in Vienna, Austria. The focus was on pea- and soybean- protein based products with either a minced or a fibrous texture, since they have been the most common representatives of PBMA in Austria. Additional criteria for the selection included that the products were entirely plant-based (vegan) and did not contain fermented products, like tofu. Beside these characteristics, the samples were different in their composition, packing, shelf life, etc. (Table 1). All samples were transported refrigerated and stored at 4 °C until their immediate processing.
Sample preparation
In total, 10 g of each sample were homogenized 120 s with 90 ml sterile 1 × phosphate buffered saline (PBS, Gibco, Bleiswijk, The Netherlands) in sterile Stomacher®400 classic strainer bags (Seward Ltd, Worthing, United Kingdom) using a BagMixer®400 CC (Interscience, Puycapel, France). To remove coarse food particles, the homogenates were centrifuged at 300 × rcf (relative centrifugal force) for 2 min at room temperature (RT) using an Eppendorf Centrifuge 5810R and an A-4-62 rotor (Eppendorf Corporate, Hamburg, Germany). The remaining supernatants were transferred to new tubes and centrifuged at 3000 × rcf (30 min at RT). The obtained cell pellets were diluted 1:10 (v/v) with sterile 1 × PBS and were used freshly for bacterial and fungal isolation or were frozen at −20 °C for later cultivation approaches and at −80 °C for DNA extraction.
Bacterial and fungal cultivation
We carried out two sets of cultivation experiments, i) broad range and ii) targeted cultivation. To get a broad range of isolates from the expected microbial communities, the first set was done with several non-selective and selective media (Columbia agar (BioMérieux, Marcy l’Etoile, France), violet red bile glucose agar (VRBG, Biokar diagnostics, Allonne, France), brain heart infusion agar (BHI broth (Biokar diagnostics) + 1.5% agar (bacteriological agar type E, Biokar diagnostics)), plate count agar (PCA, Biokar diagnostics), Rose Bengal chloramphenicol agar (Biokar diagnostics), and Baird Parker agar (GranuCult™, Merck, Darmstadt, Germany)). The plates were inoculated with 100 μl of a 10−2 dilution of the sample and incubated at 37 °C both aerobically and semi-anaerobically (using GENbox anaer, BioMérieux, in BD BBL™ GasPak™ systems, Becton Dickinson, New Jersey, United States). In order to recover high amounts of different species, the plates were incubated for 16–68 h, depending on colonies’ sizes and growth densities. The primary goal of our cultivation process was to isolate a diverse range of microbial species, rather than to quantify or describe the microbial composition in detail. Consequently, when we encountered samples exhibiting overly dense growth after 16 h of incubation, which precluded the selection of individual colonies, we proceeded to further dilute the samples 10−3 or 10−4 in sterile 1 × PBS and plate these anew. Given our focus on maximizing species diversity, any potential changes in microbial community composition or growth patterns during this period were deemed secondary concerns. For the targeted cultivation, we leveraged the insights from 16S rRNA gene amplicon sequencing to tailor specific media and growth conditions aimed at isolating representatives of genera not captured under initial culturing conditions. This strategy was facilitated by utilizing cell pellets that had been preserved at −20 °C, enabling us to revisit and effectively target these genera several months after the initial sequencing efforts. Depending on the sample specific microbial composition, we used Luria Bertani agar (1% tryptone (Oxoid, Basingstoke, UK), 0.5% yeast extract (micro-granulated, Roth, Karlsruhe, Germany), 1% NaCl (Sigma-Aldrich, St. Louis, USA), 2% agar, pH 7.0), nutrient agar (0.5% casein peptone, tryptic digest (Roth), 0.3% beef extract powder (Fluka analytical, Seelze, Germany), 1.5% agar, pH 7.0), trypto-casein soy agar (TSA,Biokar diagnostics), marine agar (marine broth (Roth) + 1.5% agar), corynebacterium agar (1% casein peptone, tryptic digest, 0.5% yeast extract, 0.5% D(+)-glucose (Roth), 0.5% NaCl, 1.5% agar, pH 7.3), De-Man-Rogosa-Sharpe agar (MRS, Oxoid) and pseudomonas agar F (1% tryptone, 1% casein peptone, 0.15% K2HPO4, 0.15% (Roth), MgSO4 (Merck), 1% glycerol (Roth), 1.5% agar) and cultivated the samples (dilution 102–105) aerobically at 25 °C for 48 h.
Isolate identification and whole genome sequencing
In both cultivation experiments, we selected morphologically unique, single colonies for re-cultivation followed by 16S rRNA gene Sanger sequencing of the pure cultures for identification. DNA was extracted, using a protocol modified after Walsh et al.85, by lysing pure cultures with 100 μl 0.01 M TRIS/HCl (Trizma®base, Sigma-Aldrich, St. Louis, United States) and 400 μl 2.5% Chelex®100 Resin solution (BioRad, Hercules, United States) at 95 °C for 10 min, followed by centrifugation with 15,000 × rcf for 3 s. The supernatants were subsequently used for 16S rRNA gene PCR, using a final concentration of 200 nM of each of the universal primers from LGC Genomics GmbH (Berlin, Germany; 27F – 5’-GAG TTT GAT CMT GGC TCA G-3’ and 1492R – 5’-GGY TAC CTT GTT ACG ACT T-3’), 0.025 U/μl Platinum™ Taq DNA-Polymerase (Invitrogen™, Vilnius, Lithuania), 1 × TaqMan PCR buffer, 2 mM MgCl2, and 250 μM dNTP Mix (Thermo Scientific™, Vilnius, Lithuania). For the PCR a protocol of 95 °C for 5 min (Taq activation) followed by 35 cycles of 40 s at 95 °C (denaturation) 40 s at 52 °C (annealing) and 1 min at 72 °C (elongation) was used. Negative extraction and PCR controls were included in the experiments. In-house Listeria monocytogenes DNA served as a positive control. All PCR products were checked with a QIAxcel DNA High Resolution Kit (Qiagen, Hilden, Germany) in the QIAxcel Advanced system (Qiagen). Samples without detectable amounts of PCR products were used for an ITS2 region PCR (200 nM of each of the primers ITS3 – 5’-GCA TCG ATG AAG AAC GCA GC-3’ and ITS4 – 5’-TCC TCC GCT TAT TGA TAT GC-3’ (White et al. 1990), 0.025 U/μl Platinum™ Taq DNA-Polymerase (Invitrogen™), 1 × TaqMan PCR buffer, 2 mM MgCl2, and 250 μM dNTP Mix (Thermo Scientific™), with a protocol of 95 °C for 5 min (Taq activation) followed by 30 cycles of 40 s at 94 °C, 40 s at 56 °C and 1 min at 72 °C). For cultures negative in 16S rRNA and ITS2 PCR, we repeated the extraction with the NucleoSpin™ Tissue kit (Machery-Nagel, Düren, Germany), using the manual in combination with the recommendations for hard-to-lyse bacteria. LGC Genomics GmbH purified and Sanger sequenced the PCR products in one direction (using 27F primer for 16S rRNA and ITS4 for the ITS2 region). Potential pathogens (n = 15) and unclassified Enterobacteriaceae (n = 6) were further subjected to whole genome sequencing (WGS) by using FLO-MIN106 flow cells on a MinION Mk1C (Oxford Nanopore Technologies, Oxford, UK). The library preparation for this approach was done according to the protocol of Oxford Nanopore Technologies (“Ligation sequencing gDNA – native barcoding (SQK-LSK109 with EXP-NBD196)”86) using the NEBNext® FFPE DNA repair kit (New England BioLabs® Inc., Ipswich, United States) for DNA repair and end-preparation, NEB Blunt/TA Ligase Master Mix (New England BioLabs® Inc.) and Native Barcoding Kit 96 (EXP-NBD196, Oxford Nanopore Technologies), for native barcode ligation, Adapter Mix II (Oxford Nanopore Technologies) and NEBNext® Quick Ligation Module (New England BioLabs® Inc.), for adapter ligation, Agencourt AMPure XP beads (Beckman Coulter™), for clean-up steps and SQK-LSK109 sequencing kit (Oxford Nanopore Technologies).
16S rRNA gene amplicon sequencing
For direct DNA extraction from PBMA samples the DNeasy® PowerFood® Microbial Kit (Qiagen) was used. For that purpose, the cell pellets stored in PBS (−80 °C) were thawed on ice, centrifuged (3000 × rcf, 30 min) and resuspended in 450 μl MBL buffer. Deviating from the DNeasy® PowerFood® Microbial Kit Handbook87, the lysis step was proceeded in Lysing Matrix A, 2 ml tubes (MP Biomedicals Germany GmbH, Eschwege, Germany). 16S rRNA gene amplicon library generation and sequencing was performed at the Vienna Biocenter Core Facilities NGS Unit (Vienna, Austria, www.vbcf.ac.at). Sequencing libraries of the 16S rRNA gene (V3/4 region) were prepared based on Illumina 16S rRNA Gene Amplicon Sequencing Library Preparation recommendations. Primers 341F (5’-CCT ACG GGN GGC WGC AG-3’) and 805R (5’-GAC TAC HVG GGT ATC TAA TCC-3’) (Klindworth et al. 2013) were used together with Illumina adapter sequences (5’-CGT CGG CAG CGT CAG ATG TGT ATA AGA GAC AG-3’ and 5’-GTC TCG TGG GCT CGG AGA TGT GTA TAA GAG ACA G-3’, respectively) for amplification. Libraries were constructed by ligating sequencing adapters and indices onto purified PCR products using the Nextera XT Sample Preparation Kit (Illumina). Equimolar amounts of each of the purified amplicons were pooled and sequenced on an Illumina MiSeq Sequencer with a 300 bp paired-end read protocol, yielding a median of 96,965 sequences per sample.
Sequence processing and statistics
The raw sequencing data obtained from the MiSeq platform was analyzed by using the QIIME 2 v2021.4.088 pipeline. The first step involved quality control, denoising, paired-end merging, chimera removal and inference of amplicon sequence variants (ASV) by using DADA289. The taxonomic classification was done with the Scikit-learn algorithm using a pre-trained full-length-uniform-classifier based on the SILVA 138.1 database, by using the q2-feature-classifier plugin90,91,92,93,94. After the removal of sequences classified as “mitochondria” or “chloroplast”, the resulting ASV table was imported into R v4.1.0 environment95 for further downstream analysis. To estimate the alpha diversities of the samples Hill-Simpson and Hill-Shannon diversity were calculated using the “iNEXT” v3.0.0 package96, based on 99.5% coverage rarefied samples97,98. Group comparisons were done with Kruskal-Wallis tests followed by Bonferroni-alpha-corrected Dunn’s tests for pairwise comparisons. For beta diversity analyses the samples were rarefied with 100 iterations depending on a coverage of 99.5% using the package “phyloseq” v1.38.099 and “metagMisc” v0.0.4100. Based on that we generated distance matrices with Bray-Curtis dissimilarities, Jaccard indices and Jensen-Shannon divergence (JSD) and use them for graphical (t-distributed stochastic neighbor embedding – tSNE) and statistical (PERMANOVA, LEfSe) analysis. For tSNE we used the “Rtsne” v0.16 package101 with a maximum of 999 iterations, a perplexity of 5 and two initial dimensions, as recommended by Oskolkov102. The high variability of the products and little knowledge on the underlying production conditions made it difficult to identify variables with adequate explanatory power for PERMANOVA, besides the main protein source and the texture of the PMBA products. The producing facility as additional variable would be meaningful, but the product assortment is dominated by one company, which made the model design very unbalanced. PERMANOVA was done with the “vegan” v2.6.4 package103 using “betadisp” to check for homogeneous dispersion and “adonis” functions for PERMANOVA with 999 iterations using main protein source and texture as explanation variables. Linear discriminant analysis Effect Size (LEfSe) was done with the relative abundance data of the coverage rarefied data in combination with “phyloseqCompanion” v1.1 package104 for data transformation, followed by the use of the LEfSe Bioconda tool by Segata et al.105, using the protein source and texture as class, a normalization of 106 and a log10 LDA score threshold of 4.0. In parallel, a group comparison for the same features, as examined with LEfSe, was done with Kruskal-Wallis tests followed by a Benjamini-Hochberg alpha correction.
The Sanger sequences from the isolates were trimmed using the “SangerRead” function within the “sangeranalyseR” v1.4.0 package106 with a Phred score mean quality cutoff of 40 and a sliding window size of 15 bp. Trimmed sequences, with > 100 bp length, were classified with the “assignTaxonomy” function of the “dada2” v1.22.0 package89 in R (with k-mer size 8 and 50 bootstrap replicates), based on an RDP (Ribosomal Database Project) Naïve Bayesian Classifier algorithm107. Further, the isolate sequences were assigned to a database generated from the MiSeq data set to connect the culture-based and culture-independent approaches. For Fig. 1, the isolate sequences of each genus were clustered within each sample, group and producer using the “IdClusters” function from the “DECIPHER” v2.22.0 package108 with a cutoff of 0.06. More clusters within each genus were interpreted as a higher species or strain diversity within each genus.
The WGS data produced with the MinION platform was trimmed and filtered with Filtlong v0.2.1109 and assembled with Flye v2.9110,111. Several polishing steps (four repetitions of Racon v1.5.0112 and a final step with Medaka v1.6.0113) followed, and the resulting genomes were analyzed by using the TORMES v1.3.0 114 pipeline with the default parameters as follows: Assembly statistics was performed by using QUAST115. The taxonomy of the genomes was inferred by using Kraken2116 and by extraction of the 16S rRNA genes by using Barrnap v0.9117 and further classified by using the RDP Classifier107. Multi-locus sequence typing (MLST) was performed by using mlst v2.19.0118. CDS prediction and protein annotation was performed by using Prodigal v2.6.3 119 and Prokka v1.14.6120. Antimicrobial resistance and virulence genes screening was performed by using Abricate v1.0.1121 against the comprehensive antibiotic resistance database (CARD)122 and the virulence factor database (VFBD)123, respectively. Further classification of the assembled genomes was performed by using the GTDB-Tk v2.2.0+124,125 and rMLST126. BTyper3127 was used for isolates classified with GTDB-Tk as Bacillus paranthracis. Lactic acid bacteria with good quality genomes were additionally examined with BlastKOALA v2.3128, dbCAN3129, combined with the databases KEGG (Kyoto Encyclopedia of Genes and Genomes https://www.kegg.jp/) or CAZymes (Carbohydrate Active Enzymes database http://www.cazy.org/)130, respectively, and antiSMASH v6.1.1131. The genes within clusters predicted with antiSMASH where then confirmed using protein-protein Basic Local Alignment Search Tool (BLAST®)132. All tools were used with default settings if not mentioned otherwise.
Reporting summary
Further information on research design is available in the Nature Research Reporting Summary linked to this article.
Data availability
All sequencing data supporting the findings of this study are openly available in the following repositories: 16S rRNA gene amplicon sequencing data and MinION whole genome sequencing data of microbial isolates from food samples have been deposited in the European Nucleotide Archive (ENA) under the study accession number PRJEB73555. The Sanger sequencing data, presented in FASTA format, are available on GitHub at https://github.com/ffroch/veggiemeat.
Code availability
All used codes are available on GitHub at https://github.com/ffroch/veggiemeat. This repository includes detailed documentation on the data processing and analysis methods used in this study.
References
-
European Commission. Directorate-General for Agriculture and Rural Development. EU agricultural outlook for markets and income 2019-2030. Publications Office, https://doi.org/10.2762/904294 (2020).
-
OECD. Meat consumption (indicator). https://doi.org/10.1787/fa290fd0-en (2022).
-
OECD and Food and Agriculture Organization of the United Nations. OECD-FAO agricultural outlook 2022-2031. https://doi.org/10.1787/f1b0b29c-en (2022).
-
Steinfeld, H. Livestock’s long shadow: Environmental issues and options, Food and Agriculture Organization of the United Nations (2006).
-
Bianchi, F., Garnett, E., Dorsel, C., Aveyard, P. & Jebb, S. A. Restructuring physical micro-environments to reduce the demand for meat: A systematic review and qualitative comparative analysis. Lancet Planet. Health 2, e384–e397 (2018).
Google Scholar
-
Economou, V. & Gousia, P. Agriculture and food animals as a source of antimicrobial-resistant bacteria. Infect. Drug Resistance 8, 49–61 (2015).
Google Scholar
-
Watts, N. et al. The lancet countdown on health and climate change: From 25 years of inaction to a global transformation for public health. Lancet 391, 581–630 (2018).
Google Scholar
-
Scarborough, P. et al. Vegans, vegetarians, fish-eaters and meat-eaters in the UK show discrepant environmental impacts. Nat. Food 4, 565–574 (2023).
Google Scholar
-
United Nations Framework Convention on Climate Change. Paris agreement. https://unfccc.int/files/essential_background/convention/application/pdf/english_paris_agreement.pdf (2016).
-
Willett, W. et al. Food in the anthropocene: The EAT-lancet commission on healthy diets from sustainable food systems. Lancet 393, 447–492 (2019).
Google Scholar
-
Stoll-Kleemann, S. & Schmidt, U. J. Reducing meat consumption in developed and transition countries to counter climate change and biodiversity loss: A review of influence factors. Regional Environ. Change 17, 1261–1277 (2017).
Google Scholar
-
Ploll, U. & Stern, T. From diet to behaviour: Exploring environmental- and animal-conscious behaviour among austrian vegetarians and vegans. Br. Food J. 122, 3249–3265; (2020).
Google Scholar
-
World Health Organization, Regionale Office for Europe. Plant-based diets and their impact on health, sustainability and the environment: A review of the evidence: WHO european office for the prevention and control of noncommunicable diseases. https://apps.who.int/iris/handle/10665/349086 (2021).
-
European Union’s Horizon 2020 research and innovation programme. Plant-based foods in Europe: How big is the market? Smart protein plant-based food sector report. https://smartproteinproject.eu/plant-based-food-sector-report (2021).
-
Neuhofer, Z. T. & Lusk, J. L. Most plant-based meat alternative buyers also buy meat: An analysis of household demographics, habit formation, and buying behavior among meat alternative buyers. Sci. Rep. 12, 13062 (2022).
Google Scholar
-
European Union’s Horizon 2020 research and innovation programme. What consumers want: A survey on european consumer attitudes towards plant-based foods, with a focus on flexitarians: European union’s horizon 2020 research and innovation programme (no 862957). https://www.smartproteinproject.eu/consumer-attitudes-plant-based-food-report (2021).
-
Curtain, F. & Grafenauer, S. Plant-based meat substitutes in the flexitarian age: An audit of products on supermarket shelves. Nutrients 11, 2603 (2019).
Google Scholar
-
Hadi, J. & Brightwell, G. Safety of alternative proteins: Technological, environmental and regulatory aspects of cultured meat, plant-based meat, insect protein and single-cell protein. Foods 10, 1226 (2021).
Google Scholar
-
Augustin Mihalache, O., Dellafiora, L. & Dall’Asta, C. A systematic review of natural toxins occurrence in plant commodities used for plant-based meat alternatives production. Food Res. Int. 158, 111490 (2022).
Google Scholar
-
Bogueva, D. & McClements, D. J. Safety and nutritional risks associated with plant-based meat alternatives. Sustainability 15, 14336 (2023).
Google Scholar
-
Augustin Mihalache, O., Carbonell-Rozas, L., Cutroneo, S. & Dall’Asta, C. Multi-mycotoxin determination in plant-based meat alternatives and exposure assessment. Food Res. Int. 168, 112766 (2023).
Google Scholar
-
Blaser, M. J. & Falkow, S. What are the consequences of the disappearing human microbiota? Nat. Rev. Microbiol. 7, 887–894 (2009).
Google Scholar
-
Vangay, P. et al. US immigration westernizes the human gut microbiome. Cell 175, 962–972.e10 (2018).
Google Scholar
-
Bartlett, A., Padfield, D., Lear, L., Bendall, R. & Vos, M. A comprehensive list of bacterial pathogens infecting humans. Microbiology 168 (2022).
-
Pakbin, B., Brück, W. M. & Rossen, J. W. A. Virulence factors of enteric pathogenic Escherichia coli: A review. Int. J. Mol. Sci. 22, 9922 (2021).
Google Scholar
-
Cosic, A. et al. Variation in accessory genes within the Klebsiella oxytoca species complex delineates monophyletic members and simplifies coherent genotyping. Front. Microbiol. 12, 692453 (2021).
Google Scholar
-
Carroll, L. M. et al. Characterization of emetic and diarrheal Bacillus cereus strains from a 2016 foodborne outbreak using whole-genome sequencing: Addressing the microbiological, epidemiological, and bioinformatic challenges. Front. Microbiol. 10, 144 (2019).
Google Scholar
-
van der Weele, C., Feindt, P., van der Jan Goot, A., van Mierlo, B. & van Boekel, M. Meat alternatives: An integrative comparison. Trends Food Sci. Technol. 88, 505–512 (2019).
Google Scholar
-
Dekkers, B. L., Boom, R. M. & van der Goot, A. J. Structuring processes for meat analogues. Trends Food Sci. Technol. 81, 25–36 (2018).
Google Scholar
-
Lin, S., Huff, H. E. & Hsieh, F. Extrusion process parameters, sensory characteristics, and structural properties of a high moisture soy protein meat analog. J. Food Sci. 67, 1066–1072 (2002).
Google Scholar
-
Beniwal, A. S., Singh, J., Kaur, L., Hardacre, A. & Singh, H. Meat analogs: Protein restructuring during thermomechanical processing. Compr. Rev. Food Sci. Food Saf. 20, 1221–1249 (2021).
Google Scholar
-
Ferawati, F. et al. High-moisture meat analogues produced from yellow pea and faba bean protein isolates/concentrate: Effect of raw material composition and extrusion parameters on texture properties. Foods 10, 843 (2021).
Google Scholar
-
Schmid, E. M., Farahnaky, A., Adhikari, B. & Torley, P. J. High moisture extrusion cooking of meat analogs: A review of mechanisms of protein texturization. Compr. Rev. Food Sci. Food Saf. 21, 4573–4609 (2022).
Google Scholar
-
Lin, S., Huff, H. E. & Hsieh, F. Texture and chemical characteristics of soy protein meat analog extruded at high moisture. J. Food Sci. 65, 264–269 (2000).
Google Scholar
-
Guyony, V., Fayolle, F. & Jury, V. High moisture extrusion of vegetable proteins for making fibrous meat analogs: A review. Food Rev. Int. 39, 4262–4287 (2022).
Google Scholar
-
Kristiawan, M. et al. Multi-scale structural changes of starch and proteins during pea flour extrusion. Food Res. Int. 108, 203–215 (2018).
Google Scholar
-
Pietsch, V. L., Werner, R., Karbstein, H. P. & Emin, M. A. High moisture extrusion of wheat gluten: Relationship between process parameters, protein polymerization, and final product characteristics. J. Food Eng. 259, 3–11 (2019).
Google Scholar
-
Yu, L., Meng, Y., Ramaswamy, H. S. & Boye, J. Residence time distribution of soy protein isolate and corn flour feed mix in a twin-screw extruder. J. Food Process. Preserv. 38, 573–584 (2014).
Google Scholar
-
Mwangi, R. Inactivation of wild type Bacillus spores in a soy meat analog model by extrusion cooking. Masterthesis, University of Missouri, Columbia https://doi.org/10.32469/10355/5763 (2008).
-
Leutgeb, K. Microbial examination of raw and extruded products for production of a vegetarian meat analogue. Masterthesis, BOKU-University of natural resources and life sciences, Vienna; Institute of Food Science. https://forschung.boku.ac.at/fis/suchen.person_betreuungen?sprache_in=de&menue_id_in=107&id_in=3823 (2017).
-
Filho, G. C. S., Vessoni Penna, R. C. & Schaffner, D. W. Microbiological quality of vegetable proteins during the preparation of a meat analog. Ital. J. Food Sci. 17, 269–283 (2005).
-
Sagoo, S. K. et al. Assessment of the microbiological safety of dried spices and herbs from production and retail premises in the United Kingdom. Food Microbiol. 26, 39–43 (2009).
Google Scholar
-
Yu, A. O., Leveau, J. H. J. & Marco, M. L. Abundance, diversity and plant-specific adaptations of plant-associated lactic acid bacteria. Environ. Microbiol. Rep. 12, 16–29 (2020).
Google Scholar
-
Chun, B. H., Kim, K. H., Jeon, H. H., Lee, S. H. & Jeon, C. O. Pan-genomic and transcriptomic analyses of Leuconostoc mesenteroides provide insights into its genomic and metabolic features and roles in kimchi fermentation. Sci. Rep. 7, 11504 (2017).
Google Scholar
-
Zhang, P., Zhang, P., Wu, J., Tao, D. & Wu, R. Effects of leuconostoc mesenteroides on physicochemical and microbial succession characterization of soybean paste, Da-jiang. LWT 115, 108028 (2019).
Google Scholar
-
de Paula, A. T., Jeronymo-Ceneviva, A. B., Todorov, S. D. & Penna, A. L. B. The two faces of Leuconostoc mesenteroides in food systems. Food Rev. Int. 31, 147–171 (2015).
Google Scholar
-
Thangavel, G. Thiruvengadam S. Curr. Pharm. Biotechnol. 20, 401–409 (2019).
Google Scholar
-
Björkroth, J. & Holzapfel, W. Genera Leuconostoc, Oenococcus and Weissella, in: Dworkin M., Falkow S.,Rosenberg E., Schleifer K.-H. & Stackebrandt E. (eds.): The Prokaryotes. Springer: pp. 267–319 (2006).
-
Casaburi, A., Piombino, P., Nychas, G. J., Villani, F. & Ercolini, D. Bacterial populations and the volatilome associated to meat spoilage. Food Microbiol. 45, 83–102 (2015).
Google Scholar
-
Lianou, A., Panagou, E.Z., Nychas, G. J. E. Microbiological spoilage of foods and beverages, in Subramaniam P. (ed.): The Stability and Shelf Life of Food, 6th ed. Elsevier: pp. 3–42 (2016).
-
Hamasaki, Y., Ayaki, M., Fuchu, H., Sugiyama, M. & Morita, H. Behavior of psychrotrophic lactic acid bacteria isolated from spoiling cooked meat products. Appl. Environ. Microbiol. 69, 3668–3671 (2003).
Google Scholar
-
Hultman, J., Rahkila, R., Ali, J., Rousu, J. & Björkroth, K. J. Meat processing plant microbiome and contamination patterns of cold-tolerant bacteria causing food safety and spoilage risks in the manufacture of vacuum-packaged cooked sausages. Appl. Environ. Microbiol. 81, 7088–7097 (2015).
Google Scholar
-
Pothakos, V., Snauwaert, C., de Vos, P., Huys, G. & Devlieghere, F. Psychrotrophic members of Leuconostoc gasicomitatum, Leuconostoc gelidum and Lactococcus piscium dominate at the end of shelf-life in packaged and chilled-stored food products in Belgium. Food Microbiol. 39, 61–67 (2014).
Google Scholar
-
Vinderola, G., Ouwehand, A. C., Salminen, S. & von Wright, A. Lactic acid bacteria: Microbiological and functional aspects, 5th ed. CRC Press (2019).
-
Jung, J. Y., Lee, S. H. & Jeon, C. O. Kimchi microflora: History, current status, and perspectives for industrial kimchi production. Appl. Microbiol. Biotechnol. 98, 2385–2393 (2014).
Google Scholar
-
Castellano, P., Pérez Ibarreche, M., Blanco Massani, M., Fontana, C. & Vignolo, G. M. Strategies for pathogen biocontrol using lactic acid bacteria and their metabolites: A focus on meat ecosystems and industrial environments. Microorganisms 5, 38 (2017).
Google Scholar
-
Park, C. W. et al. New functional probiotic Lactobacillus sakei Probio 65 alleviates atopic symptoms in the mouse. J. Medicinal Food 11, 405–412 (2008).
Google Scholar
-
Pellissery, A. J., Vinayamohan, P. G., Amalaradjou, M. A. R. & Venkitanarayanan, K. Spoilage bacteria and meat quality, in: Biswas A.K., Mandal P.K. (eds.) Meat Quality Analysis. Elsevier : pp. 307–334 (2020).
-
Poirier, S. et al. Holistic integration of omics data reveals the drivers that shape the ecology of microbial meat spoilage scenarios. Front. Microbiol. 14, 1286661 (2023).
Google Scholar
-
You, S. Y., Yang, J. S., Kim, S. H. & Hwang, I. M. Changes in the physicochemical quality characteristics of cabbage kimchi with respect to storage conditions. J. Food Qual. 2014, 9562981 (2017).
-
Geeraerts, W., de Vuyst, L. & Leroy, F. Ready-to-eat meat alternatives, a study of their associated bacterial communities. Food Biosci. 37, 100681 (2020).
Google Scholar
-
Tóth, A. J. et al. Microbial spoilage of plant-based meat analogues. Appl. Sci. 11, 8309 (2021).
Google Scholar
-
Zagorec, M. & Champomier-Vergès, M. C. Lactobacillus sakei: A starter for sausage fermentation, a protective culture for meat products. Microorganisms 5, 56 (2017).
Google Scholar
-
Comi, G. & Iacumin, L. dentification and process origin of bacteria responsible for cavities and volatile off-flavour compounds in artisan cooked ham. Int. J. Food Sci. Technol. 47, 114–121 (2012).
Google Scholar
-
Lee, M. E. et al. Starter cultures for kimchi fermentation. J. Microbiol. Biotechnol. 25, 559–568 (2015).
Google Scholar
-
Comi, G., Andyanto, D., Manzano, M. & Iacumin, L. Lactococcus lactis and Lactobacillus sakei as bio-protective culture to eliminate Leuconostoc mesenteroides spoilage and improve the shelf life and sensorial characteristics of commercial cooked bacon. Food Microbiol. 58, 16–22 (2016).
Google Scholar
-
Barbieri, F. et al. Insights into the metabolomic diversity of Latilactobacillus sakei. Foods 11, 477 (2022).
Google Scholar
-
Gorissen, S. H. M. et al. Protein content and amino acid composition of commercially available plant-based protein isolates. Amino Acids 50, 1685–1695 (2018).
Google Scholar
-
Vido, K. et al. Roles of thioredoxin reductase during the aerobic life of Lactococcus lactis. J. Bacteriol. 187, 601–610 (2005).
Google Scholar
-
Brooijmans, R. et al. Heme and menaquinone induced electron transport in lactic acid bacteria. Microb. Cell Factories 8, 28 (2009).
Google Scholar
-
Odeyemi, O. A., Burke, C. M., Bolch, C. C. J. & Stanley, R. Seafood spoilage microbiota and associated volatile organic compounds at different storage temperatures and packaging conditions. Int. J. Food Microbiol. 280, 87–99 (2018).
Google Scholar
-
Wagner, E. M., Thalguter, S., Wagner, M. & Rychli, K. Presence of microbial contamination and biofilms at a beer can filling production line. J. Food Prot. 84, 896–902 (2021).
Google Scholar
-
Vorholt, J. A. Microbial life in the phyllosphere. Nat. Rev. Microbiol. 10, 828–840 (2012).
Google Scholar
-
Luchansky, J. B. et al. Viability of shiga toxin–producing Escherichia coli, Salmonella, and Listeria monocytogenes within plant versus beef burgers during cold storage and following pan frying. J. Food Prot. 83, 434–442 (2020).
Google Scholar
-
James, C., Onarinde, B. A. & James, S. J. The use and performance of household refrigerators: A review. Compr. Rev. Food Sci. Food Saf. 16, 160–179 (2017).
Google Scholar
-
Foroogh, N., Rezvan, M., Ahmad, K. & Mahmood, S. Structural and functional characterization of the FimH adhesin of uropathogenic Escherichia coli and its novel applications. Microb. Pathogenesis 161, 105288 (2021).
Google Scholar
-
Edgar, R. & Bibi, E. MdfA, an Escherichia coli multidrug resistance protein with an extraordinarily broad spectrum of drug recognition. J. Bacteriol. 179, 2274–2280 (1997).
Google Scholar
-
Henderson, T. A., Young, K. D., Denome, S. A. & Elf, P. K. AmpC and AmpH, proteins related to the class c beta-lactamases, bind penicillin and contribute to the normal morphology of Escherichia coli. J. Bacteriol. 179, 6112–6121 (1997).
Google Scholar
-
Yang, J. et al. Klebsiella oxytoca complex: Update on taxonomy, antimicrobial resistance, and virulence. Clin. Microbiol. Rev. 35, e0000621 (2022).
Google Scholar
-
Galani, I., Karaiskos, I. & Giamarellou, H. Multidrug-resistant Klebsiella pneumoniae: Mechanisms of resistance including updated data for novel b-lactam-b-lactamase inhibitor combinations. Expert Rev. Anti-Infective Ther. 19, 1457–1468 (2021).
Google Scholar
-
de Gopegui, E. R., Juan, C., Zamorano, L., Pérez, J. L. & Oliver, A. Transferable multidrug resistance plasmid carrying cfr associated with tet(l), ant(4’)-ia, and dfrK genes from a clinical Methicillin-resistant Staphylococcus aureus ST125 strain. Antimicrob. Agents Chemother. 56, 2139–2142 (2012).
Google Scholar
-
Kosmidis, C. et al. Expression of multidrug resistance efflux pump genes in clinical and environmental isolates of Staphylococcus aureus. Int. J. Antimicrob. Agents 40, 204–209 (2012).
Google Scholar
-
Floyd, J. L., Smith, K. P., Kumar, S. H., Floyd, J. T. & Varela, M. F. LmrS is a multidrug efflux pump of the major facilitator superfamily from Staphylococcus aureus. Antimicrob. Agents Chemother. 54, 5406–5412 (2010).
Google Scholar
-
Xavier, M. R. et al. Anti-inflammatory effect, antibiotic potentiating activity against multidrug-resistant strains of Escherichia coli and Staphylococcus aureus, and evaluation of antibiotic resistance mechanisms by the ibuprofen derivative methyl 2-(-4-isobutylphenyl)propanoate. Microb. Pathogenesis 170, 105697 (2022).
Google Scholar
-
Walsh, P. S., Metzger, D. A. & Higushi, R. Chelex 100 as a medium for simple extraction of DNA for PCR-based typing from forensic material. BioTechniques 54, 134–139 (2013).
Google Scholar
-
Oxford Nanopore Technologies, Ligation sequencing gDNA – native barcoding (SQK-LSK109 with EXP-NBD196): Version: NBE_9121_v109_revE_19Jan2021. (2021).
-
Qiagen. DNeasy PowerFood microbial kit handbook. https://www.qiagen.com/at/resources/resourcedetail?id=55456cd5-2de4-43b2-8eaa-760d1371710a&lang=en (2017).
-
Bolyen, E. et al. Reproducible, interactive, scalable and extensible microbiome data science using QIIME2. Nat. Biotechnol. 37, 852–857 (2019).
Google Scholar
-
Callahan, B. J. et al. DADA2: High-resolution sample inference from Illumina amplicon data. Nat. Methods 13, 581–583 (2016).
Google Scholar
-
Quast, C. et al. The SILVA ribosomal RNA gene database project: Improved data processing and web-based tools. Nucleic Acids Res. 41, D590–6 (2013).
Google Scholar
-
Bokulich, N. A. et al. Optimizing taxonomic classification of marker-gene amplicon sequences with QIIME 2’s q2-feature-classifier plugin. Microbiome 6, 90 (2018).
Google Scholar
-
Kaehler, B. D. et al. Species abundance information improves sequence taxonomy classification accuracy. Nat. Commun. 10, 4643 (2019).
Google Scholar
-
Robeson, M. S. et al. RESCRIPt: Reproducible sequence taxonomy reference database management. PLoS Comput. Biol. 17, e1009581 (2021).
Google Scholar
-
Kaehler, B.D. et al. Silva 138.1 taxonomy classifiers for use with QIIME 2 q2-feature-classifier. https://doi.org/10.5281/zenodo.6395539 (2022).
-
R Core Team. R: A language and environment for statistical computing. https://www.R-project.org/ (2021).
-
Hsieh, T. C., Ma, K. H. & Chao, A. iNEXT: an R package for rarefaction and extrapolation of species diversity (Hill numbers). Methods Ecol. Evol. 7, 1451–1456 (2022).
Google Scholar
-
Chao, A. & Jost, L. Coverage-based rarefaction and extrapolation: Standardizing samples by completeness rather than size. Ecology 93, 2533–2547 (2012).
Google Scholar
-
Roswell, M., Dushoff, J. & Winfree, R. A conceptual guide to measuring species diversity. Oikos 130, 321–338 (2021).
Google Scholar
-
McMurdie, P. J. & Holmes, S. with contributions from Jordan G. PLOS ONE 8, e61217 (2021).
Google Scholar
-
Mikryukov, V. metagMisc: Miscellaneous functions for metagenomic analysis. https://github.com/vmikk/metagMisc (2022).
-
Krijthe, J. Rtsne: T-distributed stochastic neighbor embedding using a barnes-hut implementation. https://github.com/jkrijthe/Rtsne (2022).
-
Oskolkov, N. How to tune hyperparameters of tSNE: Three simple rules to make beautiful tSNE plots. https://towardsdatascience.com/how-to-tune-hyperparameters-of-tsne-7c0596a18868 (2019).
-
Oksanen, J. et al. Vegan: Community ecology package. https://github.com/vegandevs/vegan (2022).
-
Stagaman, K. phyloseqCompanion: Provides additional functions to work with phyloseq objects. https://github.com/kstagaman/phyloseqCompanion (2022).
-
Segata, N. et al. Metagenomic biomarker discovery and explanation. Genome Biol. 12, R60 (2011).
Google Scholar
-
Chao, K. H., Barton, K., Palmer, S. & Lanfear, R. sangeranalyseR: Simple and interactive analysis of sanger sequencing data in R. Genome Biol. Evol. 3, evab028 (2021).
Google Scholar
-
Wang, Q., Garrity, G. M., Tiedje, J. M. & Cole, J. R. Naive Bayesian classifier for rapid assignment of rRNA sequences into the new bacterial taxonomy. Appl. Environ. Microbiol. 73, 5261–5267 (2007).
Google Scholar
-
Wright, E. S. Using DECIPHER v2. R. J. 8, 352–359 (2016).
Google Scholar
-
Wick, R. Filtlong. https://github.com/rrwick/Filtlong (2021).
-
Kolmogorov, M., Yuan, J., Lin, Y. & Pevzner, P. A. Assembly of long, error-prone reads using repeat graphs. Nat. Biotechnol. 37, 540–546 (2019).
Google Scholar
-
Lin, Y. et al. Assembly of long error-prone reads using de Bruijn graphs. Proc. Natl Acad. Sci. USA 113, E8396–E8405 (2016).
Google Scholar
-
Vaser, R., Sović, I., Nagarajan, N. & Šikić, M. Fast and accurate de novo genome assembly from long uncorrected reads. Genome Res. 27, 737–746 (2017).
Google Scholar
-
Oxford Nanopore Technologies. Medaka. https://github.com/nanoporetech/medaka (2022).
-
Quijada, N. M., Rodríguez-Lázaro, D., Eiros, J. M. & Hernández, M. TORMES: An automated pipeline for whole bacterial genome analysis. Bioinformatics 35, 4207–4212 (2019).
Google Scholar
-
Gurevich, A., Saveliev, V., Vyahhi, N. & Tesler, G. QUAST: Quality assessment tool for genome assemblies. Bioinformatics 29, 1072–1075 (2013).
Google Scholar
-
Wood, D. E., Lu, J. & Langmead, B. Improved metagenomic analysis with Kraken 2. Genome Biol. 20, 257 (2019).
Google Scholar
-
Seemann, T. Barrnap. https://github.com/tseemann/barrnap (2018).
-
Seemann, T. Mlst. https://github.com/tseemann/mlst (2020).
-
Hyatt, D. et al. Prodigal: prokaryotic gene recognition and translation initiation site identification. Bioinformatics 11, 119 (2016).
-
Seemann, T. Prokka: Rapid prokaryotic genome annotation. Bioinformatics 30, 2068–2069 (2014).
Google Scholar
-
Seemann, T. Abricate. https://github.com/tseemann/abricate (2020).
-
McArthur, A. G. et al. The comprehensive antibiotic resistance database. Antimicrob. Agents Chemother. 57, 3348–3357 (2013).
Google Scholar
-
Chen, L. et al. VFDB: A reference database for bacterial virulence factors. Nucleic Acids Res. 33, D325–8 (2005).
Google Scholar
-
Chaumeil, P. A., Mussig, A. J., Hugenholtz, P. & Parks, D. H. GTDB-tk v2: Memory friendly classification with the genome taxonomy database. Bioinformatics 38, 5315–5316 (2022).
Google Scholar
-
Parks, D. H. et al. GTDB: An ongoing census of bacterial and archaeal diversity through a phylogenetically consistent, rank normalized and complete genome-based taxonomy. Nucleic Acids Res. 50, D785–D794 (2022).
Google Scholar
-
Jolley, K. A., Bray, J. E. & Maiden, M. C. J. Open-access bacterial population genomics: BIGSdb software, the PubMLST. Wellcome Open Res. 3, 124 (2018).
Google Scholar
-
Carroll, L. M., Cheng, R. A. & Kovac, J. No assembly required: Using BTyper3 to assess the congruency of a proposed taxonomic framework for the Bacillus cereus group with historical typing methods. Front. Microbiol. 11, 580691 (2020).
Google Scholar
-
Kanehisa, M., Sato, Y. & Morishima, K. BlastKOALA and GhostKOALA: KEGG tools for functional characterization of genome and metagenome sequences. J. Mol. Biol. 428, 726–731 (2016).
Google Scholar
-
Zhang, H. et al. dbCAN2: A meta server for automated carbohydrate-active enzyme annotation. Nucleic Acids Res. 46, W95–W101 (2018).
Google Scholar
-
Drula, E. et al. The carbohydrate-active enzyme database: Functions and literature. Nucleic Acids Res. 50, D571–D577 (2022).
Google Scholar
-
Blin, K. et al. antiSMASH 6. Nucleic Acids Res. 49, W29–W35 (2021).
Google Scholar
-
Altschul, S. F., Gish, W., Miller, W., Myers, E. W. & Lipman, D. J. Basic local alignment search tool. J. Mol. Biol. 215, 403–410 (1990).
Google Scholar
Acknowledgements
We would like to thank Birgit Bromberger and Christoph Eisenreich for their active support in conducting the experiments. N.M.Q. is currently funded by the European Union’s Horizon 2020 research and innovation programme under the Marie Skłodowska-Curie grant agreement No. 101034371. L.V.A. is currently funded by the European Union’s Horizon 2020 research and innovation programme under grant agreement No. 101060698. This work was supported by the Sparkling Science 2.0 grant (project ‘Micro-Tramper’) funded by the Austrian Federal Ministry of Science, Research and Economy (BMWFW).
Author information
Authors and Affiliations
Contributions
F-F.R. and E.S. conceived and planned the experiments. F-F.R. and M.D. carried out the experiments. F-F.R., N.M.Q. and E.S. performed the computations. F-F.R. and L.V.A. wrote the manuscript with input from all authors. All authors provided critical feedback on the research, analysis, and manuscript.
Corresponding author
Ethics declarations
Competing interests
The authors confirm that they have no conflicts of interest with respect to the work described in this manuscript.
Additional information
Publisher’s note Springer Nature remains neutral with regard to jurisdictional claims in published maps and institutional affiliations.
Supplementary information
Supplementary Information
Supplementary Table 2
Reporting Summary
Rights and permissions
Open Access This article is licensed under a Creative Commons Attribution 4.0 International License, which permits use, sharing, adaptation, distribution and reproduction in any medium or format, as long as you give appropriate credit to the original author(s) and the source, provide a link to the Creative Commons licence, and indicate if changes were made. The images or other third party material in this article are included in the article’s Creative Commons licence, unless indicated otherwise in a credit line to the material. If material is not included in the article’s Creative Commons licence and your intended use is not permitted by statutory regulation or exceeds the permitted use, you will need to obtain permission directly from the copyright holder. To view a copy of this licence, visit http://creativecommons.org/licenses/by/4.0/.
Reprints and permissions
About this article
Cite this article
Roch, FF., Dzieciol, M., Quijada, N.M. et al. Microbial community structure of plant-based meat alternatives.
npj Sci Food 8, 27 (2024). https://doi.org/10.1038/s41538-024-00269-8
-
Received: 07 September 2023
-
Accepted: 22 April 2024
-
Published: 13 May 2024
-
DOI: https://doi.org/10.1038/s41538-024-00269-8