Abstract
How complex phenotypes emerge from intricate gene expression patterns is a fundamental question in biology. Integrating high-content genotyping approaches such as single-cell RNA sequencing and advanced learning methods such as language models offers an opportunity for dissecting this complex relationship. Here we present a computational integrated genetics framework designed to analyze and interpret the high-dimensional landscape of genotypes and their associated phenotypes simultaneously. We applied this approach to develop a multimodal foundation model to explore the genotype–phenotype relationship manifold for human transcriptomics at the cellular level. Analyzing this joint manifold showed a refined resolution of cellular heterogeneity, uncovered potential cross-tissue biomarkers and provided contextualized embeddings to investigate the polyfunctionality of genes shown for the von Willebrand factor (VWF) gene in endothelial cells. Overall, this study advances our understanding of the dynamic interplay between gene expression and phenotypic manifestation and demonstrates the potential of integrated genetics in uncovering new dimensions of cellular function and complexity.
This is a preview of subscription content, access via your institution
Access options
/* style specs end */
Access Nature and 54 other Nature Portfolio journals
Get Nature+, our best-value online-access subscription
$29.99 / 30 days
cancel any time
Subscribe to this journal
Receive 12 digital issues and online access to articles
$99.00 per year
only $8.25 per issue
Buy this article
- Purchase on SpringerLink
- Instant access to full article PDF
Prices may be subject to local taxes which are calculated during checkout
/* style specs start */
style {
display: none !important;
}
.LiveAreaSection * {
align-content: stretch;
align-items: stretch;
align-self: auto;
animation-delay: 0s;
animation-direction: normal;
animation-duration: 0s;
animation-fill-mode: none;
animation-iteration-count: 1;
animation-name: none;
animation-play-state: running;
animation-timing-function: ease;
azimuth: center;
backface-visibility: visible;
background-attachment: scroll;
background-blend-mode: normal;
background-clip: borderBox;
background-color: transparent;
background-image: none;
background-origin: paddingBox;
background-position: 0 0;
background-repeat: repeat;
background-size: auto auto;
block-size: auto;
border-block-end-color: currentcolor;
border-block-end-style: none;
border-block-end-width: medium;
border-block-start-color: currentcolor;
border-block-start-style: none;
border-block-start-width: medium;
border-bottom-color: currentcolor;
border-bottom-left-radius: 0;
border-bottom-right-radius: 0;
border-bottom-style: none;
border-bottom-width: medium;
border-collapse: separate;
border-image-outset: 0s;
border-image-repeat: stretch;
border-image-slice: 100%;
border-image-source: none;
border-image-width: 1;
border-inline-end-color: currentcolor;
border-inline-end-style: none;
border-inline-end-width: medium;
border-inline-start-color: currentcolor;
border-inline-start-style: none;
border-inline-start-width: medium;
border-left-color: currentcolor;
border-left-style: none;
border-left-width: medium;
border-right-color: currentcolor;
border-right-style: none;
border-right-width: medium;
border-spacing: 0;
border-top-color: currentcolor;
border-top-left-radius: 0;
border-top-right-radius: 0;
border-top-style: none;
border-top-width: medium;
bottom: auto;
box-decoration-break: slice;
box-shadow: none;
box-sizing: border-box;
break-after: auto;
break-before: auto;
break-inside: auto;
caption-side: top;
caret-color: auto;
clear: none;
clip: auto;
clip-path: none;
color: initial;
column-count: auto;
column-fill: balance;
column-gap: normal;
column-rule-color: currentcolor;
column-rule-style: none;
column-rule-width: medium;
column-span: none;
column-width: auto;
content: normal;
counter-increment: none;
counter-reset: none;
cursor: auto;
display: inline;
empty-cells: show;
filter: none;
flex-basis: auto;
flex-direction: row;
flex-grow: 0;
flex-shrink: 1;
flex-wrap: nowrap;
float: none;
font-family: initial;
font-feature-settings: normal;
font-kerning: auto;
font-language-override: normal;
font-size: medium;
font-size-adjust: none;
font-stretch: normal;
font-style: normal;
font-synthesis: weight style;
font-variant: normal;
font-variant-alternates: normal;
font-variant-caps: normal;
font-variant-east-asian: normal;
font-variant-ligatures: normal;
font-variant-numeric: normal;
font-variant-position: normal;
font-weight: 400;
grid-auto-columns: auto;
grid-auto-flow: row;
grid-auto-rows: auto;
grid-column-end: auto;
grid-column-gap: 0;
grid-column-start: auto;
grid-row-end: auto;
grid-row-gap: 0;
grid-row-start: auto;
grid-template-areas: none;
grid-template-columns: none;
grid-template-rows: none;
height: auto;
hyphens: manual;
image-orientation: 0deg;
image-rendering: auto;
image-resolution: 1dppx;
ime-mode: auto;
inline-size: auto;
isolation: auto;
justify-content: flexStart;
left: auto;
letter-spacing: normal;
line-break: auto;
line-height: normal;
list-style-image: none;
list-style-position: outside;
list-style-type: disc;
margin-block-end: 0;
margin-block-start: 0;
margin-bottom: 0;
margin-inline-end: 0;
margin-inline-start: 0;
margin-left: 0;
margin-right: 0;
margin-top: 0;
mask-clip: borderBox;
mask-composite: add;
mask-image: none;
mask-mode: matchSource;
mask-origin: borderBox;
mask-position: 0 0;
mask-repeat: repeat;
mask-size: auto;
mask-type: luminance;
max-height: none;
max-width: none;
min-block-size: 0;
min-height: 0;
min-inline-size: 0;
min-width: 0;
mix-blend-mode: normal;
object-fit: fill;
object-position: 50% 50%;
offset-block-end: auto;
offset-block-start: auto;
offset-inline-end: auto;
offset-inline-start: auto;
opacity: 1;
order: 0;
orphans: 2;
outline-color: initial;
outline-offset: 0;
outline-style: none;
outline-width: medium;
overflow: visible;
overflow-wrap: normal;
overflow-x: visible;
overflow-y: visible;
padding-block-end: 0;
padding-block-start: 0;
padding-bottom: 0;
padding-inline-end: 0;
padding-inline-start: 0;
padding-left: 0;
padding-right: 0;
padding-top: 0;
page-break-after: auto;
page-break-before: auto;
page-break-inside: auto;
perspective: none;
perspective-origin: 50% 50%;
pointer-events: auto;
position: static;
quotes: initial;
resize: none;
right: auto;
ruby-align: spaceAround;
ruby-merge: separate;
ruby-position: over;
scroll-behavior: auto;
scroll-snap-coordinate: none;
scroll-snap-destination: 0 0;
scroll-snap-points-x: none;
scroll-snap-points-y: none;
scroll-snap-type: none;
shape-image-threshold: 0;
shape-margin: 0;
shape-outside: none;
tab-size: 8;
table-layout: auto;
text-align: initial;
text-align-last: auto;
text-combine-upright: none;
text-decoration-color: currentcolor;
text-decoration-line: none;
text-decoration-style: solid;
text-emphasis-color: currentcolor;
text-emphasis-position: over right;
text-emphasis-style: none;
text-indent: 0;
text-justify: auto;
text-orientation: mixed;
text-overflow: clip;
text-rendering: auto;
text-shadow: none;
text-transform: none;
text-underline-position: auto;
top: auto;
touch-action: auto;
transform: none;
transform-box: borderBox;
transform-origin: 50% 50%0;
transform-style: flat;
transition-delay: 0s;
transition-duration: 0s;
transition-property: all;
transition-timing-function: ease;
vertical-align: baseline;
visibility: visible;
white-space: normal;
widows: 2;
width: auto;
will-change: auto;
word-break: normal;
word-spacing: normal;
word-wrap: normal;
writing-mode: horizontalTb;
z-index: auto;
-webkit-appearance: none;
-moz-appearance: none;
-ms-appearance: none;
appearance: none;
margin: 0;
}
.LiveAreaSection {
width: 100%;
}
.LiveAreaSection .login-option-buybox {
display: block;
width: 100%;
font-size: 17px;
line-height: 30px;
color: #222;
padding-top: 30px;
font-family: Harding, Palatino, serif;
}
.LiveAreaSection .additional-access-options {
display: block;
font-weight: 700;
font-size: 17px;
line-height: 30px;
color: #222;
font-family: Harding, Palatino, serif;
}
.LiveAreaSection .additional-login > li:not(:first-child)::before {
transform: translateY(-50%);
content: “”;
height: 1rem;
position: absolute;
top: 50%;
left: 0;
border-left: 2px solid #999;
}
.LiveAreaSection .additional-login > li:not(:first-child) {
padding-left: 10px;
}
.LiveAreaSection .additional-login > li {
display: inline-block;
position: relative;
vertical-align: middle;
padding-right: 10px;
}
.BuyBoxSection {
display: flex;
flex-wrap: wrap;
flex: 1;
flex-direction: row-reverse;
margin: -30px -15px 0;
}
.BuyBoxSection .box-inner {
width: 100%;
height: 100%;
padding: 30px 5px;
display: flex;
flex-direction: column;
justify-content: space-between;
}
.BuyBoxSection p {
margin: 0;
}
.BuyBoxSection .readcube-buybox {
background-color: #f3f3f3;
flex-shrink: 1;
flex-grow: 1;
flex-basis: 255px;
background-clip: content-box;
padding: 0 15px;
margin-top: 30px;
}
.BuyBoxSection .subscribe-buybox {
background-color: #f3f3f3;
flex-shrink: 1;
flex-grow: 4;
flex-basis: 300px;
background-clip: content-box;
padding: 0 15px;
margin-top: 30px;
}
.BuyBoxSection .subscribe-buybox-nature-plus {
background-color: #f3f3f3;
flex-shrink: 1;
flex-grow: 4;
flex-basis: 100%;
background-clip: content-box;
padding: 0 15px;
margin-top: 30px;
}
.BuyBoxSection .title-readcube,
.BuyBoxSection .title-buybox {
display: block;
margin: 0;
margin-right: 10%;
margin-left: 10%;
font-size: 24px;
line-height: 32px;
color: #222;
text-align: center;
font-family: Harding, Palatino, serif;
}
.BuyBoxSection .title-asia-buybox {
display: block;
margin: 0;
margin-right: 5%;
margin-left: 5%;
font-size: 24px;
line-height: 32px;
color: #222;
text-align: center;
font-family: Harding, Palatino, serif;
}
.BuyBoxSection .asia-link,
.Link-328123652,
.Link-2926870917,
.Link-2291679238,
.Link-595459207 {
color: #069;
cursor: pointer;
text-decoration: none;
font-size: 1.05em;
font-family: -apple-system, BlinkMacSystemFont, “Segoe UI”, Roboto,
Oxygen-Sans, Ubuntu, Cantarell, “Helvetica Neue”, sans-serif;
line-height: 1.05em6;
}
.BuyBoxSection .access-readcube {
display: block;
margin: 0;
margin-right: 10%;
margin-left: 10%;
font-size: 14px;
color: #222;
padding-top: 10px;
text-align: center;
font-family: -apple-system, BlinkMacSystemFont, “Segoe UI”, Roboto,
Oxygen-Sans, Ubuntu, Cantarell, “Helvetica Neue”, sans-serif;
line-height: 20px;
}
.BuyBoxSection ul {
margin: 0;
}
.BuyBoxSection .link-usp {
display: list-item;
margin: 0;
margin-left: 20px;
padding-top: 6px;
list-style-position: inside;
}
.BuyBoxSection .link-usp span {
font-size: 14px;
color: #222;
font-family: -apple-system, BlinkMacSystemFont, “Segoe UI”, Roboto,
Oxygen-Sans, Ubuntu, Cantarell, “Helvetica Neue”, sans-serif;
line-height: 20px;
}
.BuyBoxSection .access-asia-buybox {
display: block;
margin: 0;
margin-right: 5%;
margin-left: 5%;
font-size: 14px;
color: #222;
padding-top: 10px;
text-align: center;
font-family: -apple-system, BlinkMacSystemFont, “Segoe UI”, Roboto,
Oxygen-Sans, Ubuntu, Cantarell, “Helvetica Neue”, sans-serif;
line-height: 20px;
}
.BuyBoxSection .access-buybox {
display: block;
margin: 0;
margin-right: 10%;
margin-left: 10%;
font-size: 14px;
color: #222;
opacity: 0.8px;
padding-top: 10px;
text-align: center;
font-family: -apple-system, BlinkMacSystemFont, “Segoe UI”, Roboto,
Oxygen-Sans, Ubuntu, Cantarell, “Helvetica Neue”, sans-serif;
line-height: 20px;
}
.BuyBoxSection .price-buybox {
display: block;
font-size: 30px;
color: #222;
font-family: -apple-system, BlinkMacSystemFont, “Segoe UI”, Roboto,
Oxygen-Sans, Ubuntu, Cantarell, “Helvetica Neue”, sans-serif;
padding-top: 30px;
text-align: center;
}
.BuyBoxSection .price-buybox-to {
display: block;
font-size: 30px;
color: #222;
font-family: -apple-system, BlinkMacSystemFont, “Segoe UI”, Roboto,
Oxygen-Sans, Ubuntu, Cantarell, “Helvetica Neue”, sans-serif;
text-align: center;
}
.BuyBoxSection .price-info-text {
font-size: 16px;
padding-right: 10px;
color: #222;
font-family: -apple-system, BlinkMacSystemFont, “Segoe UI”, Roboto,
Oxygen-Sans, Ubuntu, Cantarell, “Helvetica Neue”, sans-serif;
}
.BuyBoxSection .price-value {
font-size: 30px;
font-family: -apple-system, BlinkMacSystemFont, “Segoe UI”, Roboto,
Oxygen-Sans, Ubuntu, Cantarell, “Helvetica Neue”, sans-serif;
}
.BuyBoxSection .price-per-period {
font-family: -apple-system, BlinkMacSystemFont, “Segoe UI”, Roboto,
Oxygen-Sans, Ubuntu, Cantarell, “Helvetica Neue”, sans-serif;
}
.BuyBoxSection .price-from {
font-size: 14px;
padding-right: 10px;
color: #222;
font-family: -apple-system, BlinkMacSystemFont, “Segoe UI”, Roboto,
Oxygen-Sans, Ubuntu, Cantarell, “Helvetica Neue”, sans-serif;
line-height: 20px;
}
.BuyBoxSection .issue-buybox {
display: block;
font-size: 13px;
text-align: center;
color: #222;
font-family: -apple-system, BlinkMacSystemFont, “Segoe UI”, Roboto,
Oxygen-Sans, Ubuntu, Cantarell, “Helvetica Neue”, sans-serif;
line-height: 19px;
}
.BuyBoxSection .no-price-buybox {
display: block;
font-size: 13px;
line-height: 18px;
text-align: center;
padding-right: 10%;
padding-left: 10%;
padding-bottom: 20px;
padding-top: 30px;
color: #222;
font-family: -apple-system, BlinkMacSystemFont, “Segoe UI”, Roboto,
Oxygen-Sans, Ubuntu, Cantarell, “Helvetica Neue”, sans-serif;
}
.BuyBoxSection .vat-buybox {
display: block;
margin-top: 5px;
margin-right: 20%;
margin-left: 20%;
font-size: 11px;
color: #222;
padding-top: 10px;
padding-bottom: 15px;
text-align: center;
font-family: -apple-system, BlinkMacSystemFont, “Segoe UI”, Roboto,
Oxygen-Sans, Ubuntu, Cantarell, “Helvetica Neue”, sans-serif;
line-height: 17px;
}
.BuyBoxSection .tax-buybox {
display: block;
width: 100%;
color: #222;
padding: 20px 16px;
text-align: center;
font-family: -apple-system, BlinkMacSystemFont, “Segoe UI”, Roboto,
Oxygen-Sans, Ubuntu, Cantarell, “Helvetica Neue”, sans-serif;
line-height: NaNpx;
}
.BuyBoxSection .button-container {
display: flex;
padding-right: 20px;
padding-left: 20px;
justify-content: center;
}
.BuyBoxSection .button-container > * {
flex: 1px;
}
.BuyBoxSection .button-container > a:hover,
.Button-505204839:hover,
.Button-1078489254:hover,
.Button-2737859108:hover {
text-decoration: none;
}
.BuyBoxSection .btn-secondary {
background: #fff;
}
.BuyBoxSection .button-asia {
background: #069;
border: 1px solid #069;
border-radius: 0;
cursor: pointer;
display: block;
padding: 9px;
outline: 0;
text-align: center;
text-decoration: none;
min-width: 80px;
margin-top: 75px;
}
.BuyBoxSection .button-label-asia,
.ButtonLabel-3869432492,
.ButtonLabel-3296148077,
.ButtonLabel-1636778223 {
display: block;
color: #fff;
font-size: 17px;
line-height: 20px;
font-family: -apple-system, BlinkMacSystemFont, “Segoe UI”, Roboto,
Oxygen-Sans, Ubuntu, Cantarell, “Helvetica Neue”, sans-serif;
text-align: center;
text-decoration: none;
cursor: pointer;
}
.Button-505204839,
.Button-1078489254,
.Button-2737859108 {
background: #069;
border: 1px solid #069;
border-radius: 0;
cursor: pointer;
display: block;
padding: 9px;
outline: 0;
text-align: center;
text-decoration: none;
min-width: 80px;
max-width: 320px;
margin-top: 20px;
}
.Button-505204839 .btn-secondary-label,
.Button-1078489254 .btn-secondary-label,
.Button-2737859108 .btn-secondary-label {
color: #069;
}
.uList-2102244549 {
list-style: none;
padding: 0;
margin: 0;
}
/* style specs end */
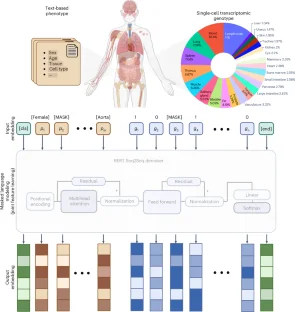
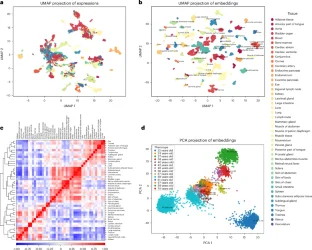
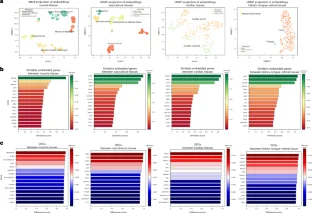
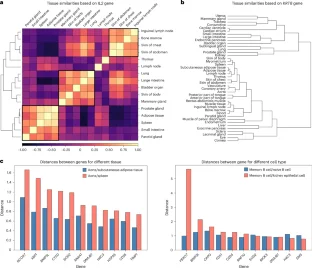
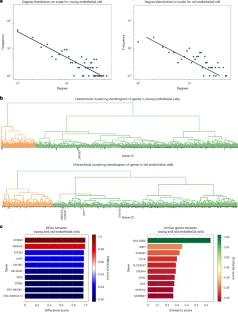
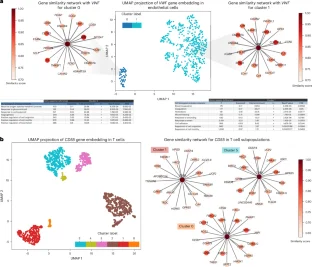
Data availability
We incorporated scRNA-seq data from the Tabula Sapiens study9, which provides manually annotated cell and tissue type and also includes metadata about each cell. The data include nearly 500,000 cells from 24 different tissues and organs, many from the same donor. Annotation of cell types shows more than 400 cell types of the human body in 14 different donors. The entire dataset can be downloaded at https://tabula-sapiens-portal.ds.czbiohub.org. Source data for Supplementary Fig. 1 are also available at this link. The Lung-liver atlas is an integration of the following datasets accessible through the Cellxgene platform at https://cellxgene.cziscience.com/collections/74e10dc4-cbb2-4605-a189-8a1cd8e44d8c, https://cellxgene.cziscience.com/collections/6f6d381a-7701-4781-935c-db10d30de293, https://cellxgene.cziscience.com/collections/c1241244-b22d-483d-875b-75699efb9f3c and https://cellxgene.cziscience.com/collections/edb893ee-4066-4128-9aec-5eb2b03f8287 and the Gene Expression Omnibus (GEO) accession number GSE136831. Source data for Figs. 2–6 are included to recreate these images.
Code availability
The training script for the PolyGene model, the transcriptome tokenizer and the code for preprocessing data and inference are publicly available via GitHub at https://github.com/roholazandie/polygene and via Zenodo at https://doi.org/10.5281/zenodo.14188129 (ref. 38).
References
-
Johannsen, W. Elemente der exakten Erblichkeitslehre (Gustav Fischer, 1909)
-
Norman, T. et al. Exploring genetic interaction manifolds constructed from rich single-cell phenotypes. Science 365, 786–793 (2019).
Google Scholar
-
Replogle, J. et al. Mapping information-rich genotype–phenotype landscapes with genome-scale Perturb-seq. Cell 185, 2559–2575.e28 (2022).
Google Scholar
-
Freimer, N. & Sabatti, C. The human phenome project. Nat. Genet. 34, 15–21 (2003).
Google Scholar
-
Kiselev, V., Andrews, T. & Hemberg, M. Challenges in unsupervised clustering of single-cell RNA-seq data. Nat. Rev. Genet. 20, 273–282 (2019).
Google Scholar
-
Stegle, O., Teichmann, S. & Marioni, J. Computational and analytical challenges in single-cell transcriptomics. Nat. Rev. Genet. 16, 133–145 (2015).
Google Scholar
-
Theodoris, C. et al. Transfer learning enables predictions in network biology. Nature https://doi.org/10.1038/s41586-023-06139-9 (2023).
-
Cui, H. et al. scGPT: toward building a foundation model for single-cell multi-omics using generative AI. Nat. Methods https://doi.org/10.1038/s41592-024-02201-0 (2024).
-
Tabula Sapiens Consortium. The Tabula Sapiens: a multiple-organ, single-cell transcriptomic atlas of humans. Science 376, eabl4896 (2022).
-
Jayagopal, A., Aiswarya, A., Garg, A. & Nandakumar, S. Multimodal representation learning with text and images. Preprint at https://arxiv.org/abs/2205.00142 (2022).
-
Kline, A. et al. Multimodal machine learning in precision health: a scoping review. NPJ Digit. Med. 5, 171 (2022).
Google Scholar
-
Cheng, J. et al. On robustness in multimodal learning. Preprint at https://arxiv.org/abs/2304.04385 (2023).
-
Lin, Z., Yu, S., Kuang, Z., Pathak, D. & Ramanan, D. Proc. IEEE/CVF Conference on Computer Vision and Pattern Recognition (IEEE, 2023).
-
Chen, J. et al. The tongue features associated with chronic kidney disease. Medicine 100, e25037 (2021).
Google Scholar
-
Bemelman, F. & Verhoeven, C. A failing kidney and a burning tongue. Kidney Int. 86, 654 (2014).
Google Scholar
-
Guilliams, M. et al. Spatial proteogenomics reveals distinct and evolutionarily conserved hepatic macrophage niches. Cell 185, 379–396.e38 (2022).
Google Scholar
-
Salcher, S. et al. High-resolution single-cell atlas reveals diversity and plasticity of tissue-resident neutrophils in non-small cell lung cancer. Cancer Cell 40, 1503–1520.e8 (2022).
Google Scholar
-
Adams, T. et al. Single-cell RNA-seq reveals ectopic and aberrant lung-resident cell populations in idiopathic pulmonary fibrosis. Sci. Adv. 6, eaba1983 (2020).
Google Scholar
-
Sikkema, L. et al. An integrated cell atlas of the lung in health and disease. Nat. Med. 29, 1563–1577 (2023).
Google Scholar
-
Madissoon, E. et al. A spatially resolved atlas of the human lung characterizes a gland-associated immune niche. Nat. Genet. 55, 66–77 (2023).
Google Scholar
-
Marzluff, W., Gongidi, P., Woods, K., Jin, J. & Maltais, L. The human and mouse replication-dependent histone genes. Genomics 80, 487–498 (2002).
Google Scholar
-
Albig, W., Kioschis, P., Poustka, A., Meergans, K. & Doenecke, D. Human histone gene organization: nonregular arrangement within a large cluster. Genomics 40, 314–322 (1997).
Google Scholar
-
Lee, S. et al. Regulation of muscle mass by follistatin and activins. Mol. Endocrinol. 24, 1998–2008 (2010).
Google Scholar
-
Iyer, C. et al. Follistatin-induced muscle hypertrophy in aged mice improves neuromuscular junction innervation and function. Neurobiol. Aging 104, 32–41 (2021).
Google Scholar
-
Kota, J. et al. Follistatin gene delivery enhances muscle growth and strength in nonhuman primates. Sci. Transl. Med. 1, 6ra15 (2009).
Google Scholar
-
Jaijyan, D. et al. New intranasal and injectable gene therapy for healthy life extension. Proc. Natl Acad. Sci. USA 119, e2121499119 (2022).
Google Scholar
-
Boyman, O. & Sprent, J. The role of interleukin-2 during homeostasis and activation of the immune system. Nat. Rev. Immunol. 12, 180–190 (2012).
Google Scholar
-
Zhou, L. et al. Innate lymphoid cells support regulatory T cells in the intestine through interleukin-2. Nature 568, 405–409 (2019).
Google Scholar
-
Khanin, R. & Wit, E. How scale-free are biological networks. J. Comput. Biol. 13, 810–818 (2006).
Google Scholar
-
Arita, M. Scale-freeness and biological networks. J. Biochem. 138, 1–4 (2005).
Google Scholar
-
Yang, F. et al. scBERT as a large-scale pretrained deep language model for cell type annotation of single-cell RNA-seq data. Nat. Mach. Intell. 4, 852–866 (2022).
Google Scholar
-
Mikolov, T., Sutskever, I., Chen, K., Corrado, G. & Dean, J. Distributed representations of words and phrases and their compositionality. In Advances in Neural Information Processing Systems 26 (eds Burges, C. J.) 3111–3119 (Curran Associates, Inc., 2013).
-
Vaswani, A. et al. Attention is all you need. In Advances in Neural Information Processing Systems 30 (eds Guyon, I.) 5998–6008 (Curran Associates, Inc., 2017).
-
Devlin, J., Chang, M., Lee, K. & Toutanova, K. BERT: pre-training of deep bidirectional transformers for language understanding. Preprint at https://arxiv.org/abs/1810.04805 (2018).
-
Raffel, C. et al. Exploring the limits of transfer learning with a unified text-to-text transformer. J. Mach. Learn. Res. 21, 1–67 (2020).
Google Scholar
-
Wolf, F., Angerer, P. & Theis, F. SCANPY: large-scale single-cell gene expression data analysis. Genome Biol. 19, 15 (2018).
Google Scholar
-
Wolf, T. et al. Huggingface’s transformers: state-of-the-art natural language processing. Preprint at https://arxiv.org/abs/1910.03771 (2019).
-
Zandie, R. & Khodaee, F. roholazandie/polygene: polygene. Zenodo https://doi.org/10.5281/zenodo.14188129 (2024).
Acknowledgements
The work was supported in part by a grant from the National Institutes of Health R01 HL161069 awarded to E.R.E.
Author information
Authors and Affiliations
Contributions
F.K. and R.Z. conceptualized the study. F.K. led the design of the computational framework of the multimodal model. R.Z. implemented the code and trained the model. E.R.E. provided guidance and critical revisions throughout the research. Data preprocessing and model implementation were conducted by R.Z. F.K. analyzed the results and drafted the paper. All authors contributed to the review and editing of the paper and approved the final version.
Corresponding author
Ethics declarations
Competing interests
The authors declare no competing interests.
Peer review
Peer review information
Nature Computational Science thanks Zheng Xia and the other, anonymous, reviewer(s) for their contribution to the peer review of this work. Peer reviewer reports are available. Primary Handling Editor: Ananya Rastogi, in collaboration with the Nature Computational Science team.
Additional information
Publisher’s note Springer Nature remains neutral with regard to jurisdictional claims in published maps and institutional affiliations.
Supplementary information
Supplementary Information
Supplementary Figs. 1–8 and Supplementary Tables 1 and 2.
Reporting Summary
Peer Review File
Source data
Source Data Fig. 2
Source embedding data.
Source Data Fig. 3
Source embedding data for all the tissues, similarity and difference scores.
Source Data Fig. 4
Source embedding data for IL2 and KRT8, and distance data.
Source Data Fig. 5
Source embedding data for ECs in different ages.
Source Data Fig. 6
Source embedding data for both VWF and CD55.
Rights and permissions
Springer Nature or its licensor (e.g. a society or other partner) holds exclusive rights to this article under a publishing agreement with the author(s) or other rightsholder(s); author self-archiving of the accepted manuscript version of this article is solely governed by the terms of such publishing agreement and applicable law.
Reprints and permissions
About this article
Cite this article
Khodaee, F., Zandie, R. & Edelman, E.R. Multimodal learning for mapping genotype–phenotype dynamics.
Nat Comput Sci (2025). https://doi.org/10.1038/s43588-024-00765-7
-
Received: 01 May 2024
-
Accepted: 20 December 2024
-
Published: 28 January 2025
-
DOI: https://doi.org/10.1038/s43588-024-00765-7