We present a Focus that calls attention to the current state of diversity, equity, and inclusion in computational science, including discussions on the challenges of improving equitable access and representation, as well as on strategies for improving computational tools to avoid contributing to inequalities.
At Nature Computational Science and the Nature Portfolio, one of our core values is ensuring diversity, equity, and inclusion (DEI), not only within our own teams but through our work as editors as well. We are committed to DEI within our internal practices and in our published content, and we strive to promote these values within the research communities that we serve. Of course, DEI has many dimensions — including gender, race/ethnicity, geographical origin, and educational experience — and is much more than just representation: it is also about addressing the sources of biases and discrimination, creating a sense of belonging for everyone through fair treatment and inclusive practices, and building communities to empower one another. Embracing DEI practices is the right thing to do, and it has also been proven to positively influence scientific performance: studies have underscored that more diverse research groups produce more novel and highly cited papers1,2. Nevertheless, there is still a long road ahead to improve DEI in science.
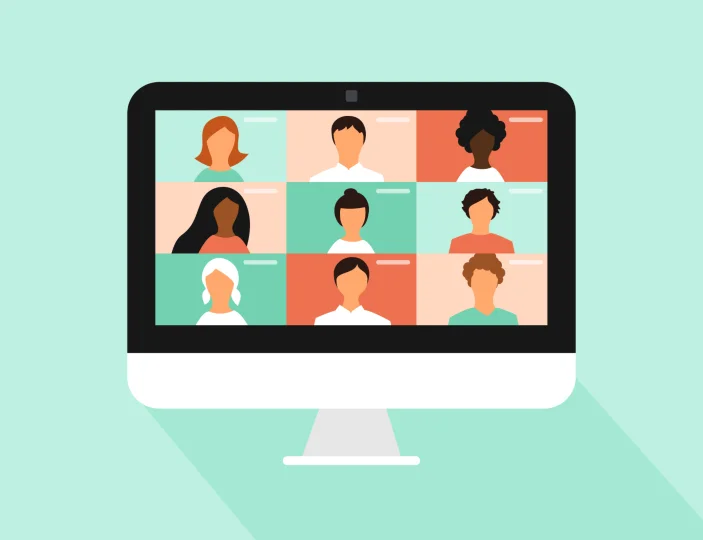
Credit: chuwy / DigitalVision Vectors / Getty Images
In this issue, we feature a Focus with the goal of starting a conversation about the current state of DEI in the broad field of computational science — and from the content presented within this collection, it is evident that a great deal still needs to be done moving forward.
In a Viewpoint, several scientists discuss strategies for increasing the presence of Black, Indigenous, and People of Color (BIPOC) researchers in computational science. Numerous contributors emphasize the importance of representation and building a sense of community, highlighting organizations and initiatives for this task. Christine Yifeng Chen notes the need to address funding disparities, as data shows that white applicants have received funding at higher rates than other groups, particularly Black and Asian applicants. “How do we break this cycle? Simply put, to eliminate inequalities, we must address the causes of inequality in the first place: unequal access to social prestige, insider knowledge, and most importantly, organizational resources,” she says. Some scientists argue for the importance of human-centered — or kanaka-centered — design approaches (‘kanaka’ means ‘human’ in the Hawaiian language), as well as for co-designing technologies with the community in order to improve equity for Native American and Indigenous researchers. The computational tools themselves are also discussed in this context, with Tai-Quan Peng and Karaitiana Taiuru underscoring the current role and potential of large language models in the field.
The importance of carefully and intentionally designed computational tools is echoed in a News Feature that explores the erasure of LGBTQ+ content from artificial intelligence (AI) training data. While AI companies have valid reasons for implementing safety systems, this does raise concerns about who decides which type of content is offensive. Though well intentioned, data shows that these safety filters have disproportionately removed LGBTQ+ content, which coincides with some of the efforts in the United States to remove queer content from public spaces, including book bans. As Sophia Chen puts it, “the loss of this data amounts to real-world consequences for LGBTQ+ people, as the models learn a patchy version of reality that obscures the full extent of their existence.”
When it comes to AI, the relationship between users and systems requires careful planning and consideration to empower users and avoid exacerbating existing inequalities. Siddharth Suri reflects in a Comment on what our relationship with AI should look like. In particular, he emphasizes the need for ethical and fair work environments for AI workers who may not have the power and autonomy to determine their relationships with AI systems. Vinod Namboodiri also notes in a Comment that AI tools can — and should — be used to empower users, especially persons with disabilities. Currently, there is limited mapping of indoor spaces, as traditional satellite-based positioning cannot be used, meaning that wayfinding typically occurs through visual signage. This approach may exclude persons who are blind or visually impaired, and may also suggest inconvenient routes to those with mobility impairments. Given that persons with disabilities represent a very diverse population, a user-centered design approach is particularly important in order to ensure that the tools best serve the intended users.
Inequality is also present when it comes to resource availability across different geographical regions. As an example, Joaquín Barroso-Flores discusses in a Comment the role of economic development in the supercomputing infrastructure in Latin America, as well as ongoing access challenges to such infrastructure. Despite a rich history of supercomputing in Latin America, including entries on the TOP500 list (which ranks the 500 most powerful supercomputers in the world), the region still falls behind the United States and many Asian and European countries, and several challenges remain for advancing infrastructure development and resource allocation. Notably, while financial resources are still needed, Barroso-Flores notes that a “throwing-money-at-the-problem” approach is not the way to narrow the gap between nations, but rather that there needs to be an emphasis on building alliances that make the most of the available infrastructure, ensuring international and interdisciplinary collaborations for sustaining and advancing the existing architectures.
Moving forward, computational tools need to be designed to better serve underrepresented communities and mitigate existing biases. For instance, in a Comment, Elaine O. Nsoesie argues that AI tools used in healthcare should have responsible use labels that mimic the Food and Drug Administration approved prescription labels. Such labels would provide information on approved and unapproved usages (for instance, use within specific populations and descriptions of known use cases), instances where potential issues may be encountered (such as hallucinations or misrepresentations of historical data), and so forth, in an effort to limit misuse of AI models in healthcare settings and to reduce their potential to worsen health inequities. In another Comment, Laetitia Gauvin reaffirms the need for addressing biases and data gaps within mobility data. She argues that we need a holistic approach based on knowledge of user diversity, mobility practices, and the needs of potentially vulnerable groups; furthermore, she states that results should be interpreted in the context of the social needs of each group.
Above all, we understand the importance of having a diverse, equitable and inclusive field of researchers. We believe that science is for everyone and we hope that this non-exhaustive exploration of DEI pushes the field to take a closer look at our method development practices, the potential harm that some AI tools may cause, our work and research environments, and the communities that we serve.
References
-
Yang, Y., Tian, T. Y., Woodruff, T. K., Jones, B. F. & Uzzi, B. PNAS 119, e2200841119 (2022).
Google Scholar
-
Powell, K. Nature 558, 19–22 (2018).
Google Scholar
Rights and permissions
Reprints and permissions
About this article
Cite this article
Putting a spotlight on diversity, equity, and inclusion.
Nat Comput Sci 4, 627–628 (2024). https://doi.org/10.1038/s43588-024-00702-8
-
Published: 24 September 2024
-
Issue Date: September 2024
-
DOI: https://doi.org/10.1038/s43588-024-00702-8