Abstract
The rapid advancement of artificial intelligence (AI) is poised to reshape almost every line of work. Despite enormous efforts devoted to understanding AI’s economic impacts, we lack a systematic understanding of the benefits to scientific research associated with the use of AI. Here we develop a measurement framework to estimate the direct use of AI and associated benefits in science. We find that the use and benefits of AI appear widespread throughout the sciences, growing especially rapidly since 2015. However, there is a substantial gap between AI education and its application in research, highlighting a misalignment between AI expertise supply and demand. Our analysis also reveals demographic disparities, with disciplines with higher proportions of women or Black scientists reaping fewer benefits from AI, potentially exacerbating existing inequalities in science. These findings have implications for the equity and sustainability of the research enterprise, especially as the integration of AI with science continues to deepen.
This is a preview of subscription content, access via your institution
Access options
style{display:none!important}.LiveAreaSection-193358632 *{align-content:stretch;align-items:stretch;align-self:auto;animation-delay:0s;animation-direction:normal;animation-duration:0s;animation-fill-mode:none;animation-iteration-count:1;animation-name:none;animation-play-state:running;animation-timing-function:ease;azimuth:center;backface-visibility:visible;background-attachment:scroll;background-blend-mode:normal;background-clip:borderBox;background-color:transparent;background-image:none;background-origin:paddingBox;background-position:0 0;background-repeat:repeat;background-size:auto auto;block-size:auto;border-block-end-color:currentcolor;border-block-end-style:none;border-block-end-width:medium;border-block-start-color:currentcolor;border-block-start-style:none;border-block-start-width:medium;border-bottom-color:currentcolor;border-bottom-left-radius:0;border-bottom-right-radius:0;border-bottom-style:none;border-bottom-width:medium;border-collapse:separate;border-image-outset:0s;border-image-repeat:stretch;border-image-slice:100%;border-image-source:none;border-image-width:1;border-inline-end-color:currentcolor;border-inline-end-style:none;border-inline-end-width:medium;border-inline-start-color:currentcolor;border-inline-start-style:none;border-inline-start-width:medium;border-left-color:currentcolor;border-left-style:none;border-left-width:medium;border-right-color:currentcolor;border-right-style:none;border-right-width:medium;border-spacing:0;border-top-color:currentcolor;border-top-left-radius:0;border-top-right-radius:0;border-top-style:none;border-top-width:medium;bottom:auto;box-decoration-break:slice;box-shadow:none;box-sizing:border-box;break-after:auto;break-before:auto;break-inside:auto;caption-side:top;caret-color:auto;clear:none;clip:auto;clip-path:none;color:initial;column-count:auto;column-fill:balance;column-gap:normal;column-rule-color:currentcolor;column-rule-style:none;column-rule-width:medium;column-span:none;column-width:auto;content:normal;counter-increment:none;counter-reset:none;cursor:auto;display:inline;empty-cells:show;filter:none;flex-basis:auto;flex-direction:row;flex-grow:0;flex-shrink:1;flex-wrap:nowrap;float:none;font-family:initial;font-feature-settings:normal;font-kerning:auto;font-language-override:normal;font-size:medium;font-size-adjust:none;font-stretch:normal;font-style:normal;font-synthesis:weight style;font-variant:normal;font-variant-alternates:normal;font-variant-caps:normal;font-variant-east-asian:normal;font-variant-ligatures:normal;font-variant-numeric:normal;font-variant-position:normal;font-weight:400;grid-auto-columns:auto;grid-auto-flow:row;grid-auto-rows:auto;grid-column-end:auto;grid-column-gap:0;grid-column-start:auto;grid-row-end:auto;grid-row-gap:0;grid-row-start:auto;grid-template-areas:none;grid-template-columns:none;grid-template-rows:none;height:auto;hyphens:manual;image-orientation:0deg;image-rendering:auto;image-resolution:1dppx;ime-mode:auto;inline-size:auto;isolation:auto;justify-content:flexStart;left:auto;letter-spacing:normal;line-break:auto;line-height:normal;list-style-image:none;list-style-position:outside;list-style-type:disc;margin-block-end:0;margin-block-start:0;margin-bottom:0;margin-inline-end:0;margin-inline-start:0;margin-left:0;margin-right:0;margin-top:0;mask-clip:borderBox;mask-composite:add;mask-image:none;mask-mode:matchSource;mask-origin:borderBox;mask-position:0 0;mask-repeat:repeat;mask-size:auto;mask-type:luminance;max-height:none;max-width:none;min-block-size:0;min-height:0;min-inline-size:0;min-width:0;mix-blend-mode:normal;object-fit:fill;object-position:50% 50%;offset-block-end:auto;offset-block-start:auto;offset-inline-end:auto;offset-inline-start:auto;opacity:1;order:0;orphans:2;outline-color:initial;outline-offset:0;outline-style:none;outline-width:medium;overflow:visible;overflow-wrap:normal;overflow-x:visible;overflow-y:visible;padding-block-end:0;padding-block-start:0;padding-bottom:0;padding-inline-end:0;padding-inline-start:0;padding-left:0;padding-right:0;padding-top:0;page-break-after:auto;page-break-before:auto;page-break-inside:auto;perspective:none;perspective-origin:50% 50%;pointer-events:auto;position:static;quotes:initial;resize:none;right:auto;ruby-align:spaceAround;ruby-merge:separate;ruby-position:over;scroll-behavior:auto;scroll-snap-coordinate:none;scroll-snap-destination:0 0;scroll-snap-points-x:none;scroll-snap-points-y:none;scroll-snap-type:none;shape-image-threshold:0;shape-margin:0;shape-outside:none;tab-size:8;table-layout:auto;text-align:initial;text-align-last:auto;text-combine-upright:none;text-decoration-color:currentcolor;text-decoration-line:none;text-decoration-style:solid;text-emphasis-color:currentcolor;text-emphasis-position:over right;text-emphasis-style:none;text-indent:0;text-justify:auto;text-orientation:mixed;text-overflow:clip;text-rendering:auto;text-shadow:none;text-transform:none;text-underline-position:auto;top:auto;touch-action:auto;transform:none;transform-box:borderBox;transform-origin:50% 50%0;transform-style:flat;transition-delay:0s;transition-duration:0s;transition-property:all;transition-timing-function:ease;vertical-align:baseline;visibility:visible;white-space:normal;widows:2;width:auto;will-change:auto;word-break:normal;word-spacing:normal;word-wrap:normal;writing-mode:horizontalTb;z-index:auto;-webkit-appearance:none;-moz-appearance:none;-ms-appearance:none;appearance:none;margin:0}.LiveAreaSection-193358632{width:100%}.LiveAreaSection-193358632 .login-option-buybox{display:block;width:100%;font-size:17px;line-height:30px;color:#222;padding-top:30px;font-family:Harding,Palatino,serif}.LiveAreaSection-193358632 .additional-access-options{display:block;font-weight:700;font-size:17px;line-height:30px;color:#222;font-family:Harding,Palatino,serif}.LiveAreaSection-193358632 .additional-login>li:not(:first-child)::before{transform:translateY(-50%);content:””;height:1rem;position:absolute;top:50%;left:0;border-left:2px solid #999}.LiveAreaSection-193358632 .additional-login>li:not(:first-child){padding-left:10px}.LiveAreaSection-193358632 .additional-login>li{display:inline-block;position:relative;vertical-align:middle;padding-right:10px}.BuyBoxSection-683559780{display:flex;flex-wrap:wrap;flex:1;flex-direction:row-reverse;margin:-30px -15px 0}.BuyBoxSection-683559780 .box-inner{width:100%;height:100%;padding:30px 5px;display:flex;flex-direction:column;justify-content:space-between}.BuyBoxSection-683559780 p{margin:0}.BuyBoxSection-683559780 .readcube-buybox{background-color:#f3f3f3;flex-shrink:1;flex-grow:1;flex-basis:255px;background-clip:content-box;padding:0 15px;margin-top:30px}.BuyBoxSection-683559780 .subscribe-buybox{background-color:#f3f3f3;flex-shrink:1;flex-grow:4;flex-basis:300px;background-clip:content-box;padding:0 15px;margin-top:30px}.BuyBoxSection-683559780 .subscribe-buybox-nature-plus{background-color:#f3f3f3;flex-shrink:1;flex-grow:4;flex-basis:100%;background-clip:content-box;padding:0 15px;margin-top:30px}.BuyBoxSection-683559780 .title-readcube,.BuyBoxSection-683559780 .title-buybox{display:block;margin:0;margin-right:10%;margin-left:10%;font-size:24px;line-height:32px;color:#222;text-align:center;font-family:Harding,Palatino,serif}.BuyBoxSection-683559780 .title-asia-buybox{display:block;margin:0;margin-right:5%;margin-left:5%;font-size:24px;line-height:32px;color:#222;text-align:center;font-family:Harding,Palatino,serif}.BuyBoxSection-683559780 .asia-link{color:#069;cursor:pointer;text-decoration:none;font-size:1.05em;font-family:-apple-system,BlinkMacSystemFont,”Segoe UI”,Roboto,Oxygen-Sans,Ubuntu,Cantarell,”Helvetica Neue”,sans-serif;line-height:1.05em6}.BuyBoxSection-683559780 .access-readcube{display:block;margin:0;margin-right:10%;margin-left:10%;font-size:14px;color:#222;padding-top:10px;text-align:center;font-family:-apple-system,BlinkMacSystemFont,”Segoe UI”,Roboto,Oxygen-Sans,Ubuntu,Cantarell,”Helvetica Neue”,sans-serif;line-height:20px}.BuyBoxSection-683559780 ul{margin:0}.BuyBoxSection-683559780 .link-usp{display:list-item;margin:0;margin-left:20px;padding-top:6px;list-style-position:inside}.BuyBoxSection-683559780 .link-usp span{font-size:14px;color:#222;font-family:-apple-system,BlinkMacSystemFont,”Segoe UI”,Roboto,Oxygen-Sans,Ubuntu,Cantarell,”Helvetica Neue”,sans-serif;line-height:20px}.BuyBoxSection-683559780 .access-asia-buybox{display:block;margin:0;margin-right:5%;margin-left:5%;font-size:14px;color:#222;padding-top:10px;text-align:center;font-family:-apple-system,BlinkMacSystemFont,”Segoe UI”,Roboto,Oxygen-Sans,Ubuntu,Cantarell,”Helvetica Neue”,sans-serif;line-height:20px}.BuyBoxSection-683559780 .access-buybox{display:block;margin:0;margin-right:10%;margin-left:10%;font-size:14px;color:#222;opacity:.8px;padding-top:10px;text-align:center;font-family:-apple-system,BlinkMacSystemFont,”Segoe UI”,Roboto,Oxygen-Sans,Ubuntu,Cantarell,”Helvetica Neue”,sans-serif;line-height:20px}.BuyBoxSection-683559780 .price-buybox{display:block;font-size:30px;color:#222;font-family:-apple-system,BlinkMacSystemFont,”Segoe UI”,Roboto,Oxygen-Sans,Ubuntu,Cantarell,”Helvetica Neue”,sans-serif;padding-top:30px;text-align:center}.BuyBoxSection-683559780 .price-buybox-to{display:block;font-size:30px;color:#222;font-family:-apple-system,BlinkMacSystemFont,”Segoe UI”,Roboto,Oxygen-Sans,Ubuntu,Cantarell,”Helvetica Neue”,sans-serif;text-align:center}.BuyBoxSection-683559780 .price-info-text{font-size:16px;padding-right:10px;color:#222;font-family:-apple-system,BlinkMacSystemFont,”Segoe UI”,Roboto,Oxygen-Sans,Ubuntu,Cantarell,”Helvetica Neue”,sans-serif}.BuyBoxSection-683559780 .price-value{font-size:30px;font-family:-apple-system,BlinkMacSystemFont,”Segoe UI”,Roboto,Oxygen-Sans,Ubuntu,Cantarell,”Helvetica Neue”,sans-serif}.BuyBoxSection-683559780 .price-per-period{font-family:-apple-system,BlinkMacSystemFont,”Segoe UI”,Roboto,Oxygen-Sans,Ubuntu,Cantarell,”Helvetica Neue”,sans-serif}.BuyBoxSection-683559780 .price-from{font-size:14px;padding-right:10px;color:#222;font-family:-apple-system,BlinkMacSystemFont,”Segoe UI”,Roboto,Oxygen-Sans,Ubuntu,Cantarell,”Helvetica Neue”,sans-serif;line-height:20px}.BuyBoxSection-683559780 .issue-buybox{display:block;font-size:13px;text-align:center;color:#222;font-family:-apple-system,BlinkMacSystemFont,”Segoe UI”,Roboto,Oxygen-Sans,Ubuntu,Cantarell,”Helvetica Neue”,sans-serif;line-height:19px}.BuyBoxSection-683559780 .no-price-buybox{display:block;font-size:13px;line-height:18px;text-align:center;padding-right:10%;padding-left:10%;padding-bottom:20px;padding-top:30px;color:#222;font-family:-apple-system,BlinkMacSystemFont,”Segoe UI”,Roboto,Oxygen-Sans,Ubuntu,Cantarell,”Helvetica Neue”,sans-serif}.BuyBoxSection-683559780 .vat-buybox{display:block;margin-top:5px;margin-right:20%;margin-left:20%;font-size:11px;color:#222;padding-top:10px;padding-bottom:15px;text-align:center;font-family:-apple-system,BlinkMacSystemFont,”Segoe UI”,Roboto,Oxygen-Sans,Ubuntu,Cantarell,”Helvetica Neue”,sans-serif;line-height:17px}.BuyBoxSection-683559780 .tax-buybox{display:block;width:100%;color:#222;padding:20px 16px;text-align:center;font-family:-apple-system,BlinkMacSystemFont,”Segoe UI”,Roboto,Oxygen-Sans,Ubuntu,Cantarell,”Helvetica Neue”,sans-serif;line-height:NaNpx}.BuyBoxSection-683559780 .button-container{display:flex;padding-right:20px;padding-left:20px;justify-content:center}.BuyBoxSection-683559780 .button-container>*{flex:1px}.BuyBoxSection-683559780 .button-container>a:hover,.Button-505204839:hover,.Button-1078489254:hover,.Button-2737859108:hover{text-decoration:none}.BuyBoxSection-683559780 .btn-secondary{background:#fff}.BuyBoxSection-683559780 .button-asia{background:#069;border:1px solid #069;border-radius:0;cursor:pointer;display:block;padding:9px;outline:0;text-align:center;text-decoration:none;min-width:80px;margin-top:75px}.BuyBoxSection-683559780 .button-label-asia,.ButtonLabel-3869432492,.ButtonLabel-3296148077,.ButtonLabel-1636778223{display:block;color:#fff;font-size:17px;line-height:20px;font-family:-apple-system,BlinkMacSystemFont,”Segoe UI”,Roboto,Oxygen-Sans,Ubuntu,Cantarell,”Helvetica Neue”,sans-serif;text-align:center;text-decoration:none;cursor:pointer}.Button-505204839,.Button-1078489254,.Button-2737859108{background:#069;border:1px solid #069;border-radius:0;cursor:pointer;display:block;padding:9px;outline:0;text-align:center;text-decoration:none;min-width:80px;max-width:320px;margin-top:20px}.Button-505204839 .btn-secondary-label,.Button-1078489254 .btn-secondary-label,.Button-2737859108 .btn-secondary-label{color:#069}
/* style specs end */
Access Nature and 54 other Nature Portfolio journals
Get Nature+, our best-value online-access subscription
$29.99 / 30 days
cancel any time
Subscribe to this journal
Receive 12 digital issues and online access to articles
$119.00 per year
only $9.92 per issue
Buy this article
- Purchase on SpringerLink
- Instant access to full article PDF
Prices may be subject to local taxes which are calculated during checkout
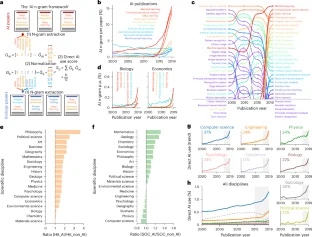
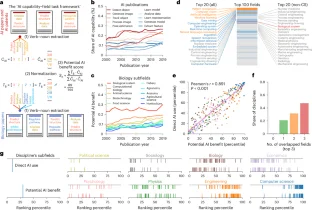

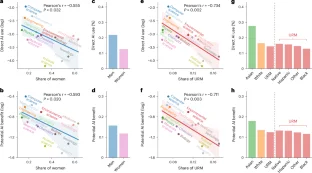
Data availability
The MAG data are available at https://doi.org/10.5281/zenodo.6511057 (ref. 131) and ref. 132. The USPTO patent data are available at https://patentsview.org. The OSP dataset is available from the paper at https://doi.org/10.1073/pnas.1804247115. The SDR data are available at https://www.nsf.gov/statistics/srvydoctoratework, and the datasets used in this study are de-identified, containing only summary statistics for each discipline. The data met the assumption of tests in the analysis. The data necessary to reproduce all main plots in this paper are available at https://kellogg-cssi.github.io/ai4science.
Code availability
Data are linked and analysed with customized code in Python 3 using standard software packages within these programmes, including pandas 1.3.5, numpy 1.21.5, scipy 1.7.3, matplotlib 3.5.1, seaborn 0.11.2, spacy 3.7.2, nomquamgender 0.1.0, demographicx 0.0.1 and others. The code necessary to reproduce all main plots and statistical analyses is available at https://kellogg-cssi.github.io/ai4science.
References
-
Herbert, A. S. The Sciences of the Artificial (MIT Press, 1969).
-
Brynjolfsson, E. & Mitchell, T. What can machine learning do? Workforce implications. Science 358, 1530–1534 (2017).
Google Scholar
-
Agrawal, A., Gans, J. & Goldfarb, A. The Economics of Artificial Intelligence: An Agenda (Univ. Chicago Press, 2019).
-
Autor, D., Mindell, D. A. & Reynolds, E. B. The Work of the Future: Shaping Technology and Institutions (MIT Task Force, 2019).
-
Acemoglu, D., Autor, D., Hazell, J. & Restrepo, P. Artificial intelligence and jobs: evidence from online vacancies. J. Labor Econ. 40, S293–S340 (2022).
Google Scholar
-
Aghion, P., Jones, B. F. & Jones, C. I. in The Economics of Artificial Intelligence: An Agenda Ch. 9, 237–290 (Univ. Chicago Press, 2019).
-
Cockburn, I. M., Henderson, R. & Stern, S. in The Economics of Artificial Intelligence: An Agenda Ch. 4, 115–148 (Univ. Chicago Press, 2019).
-
Tomasev, N. et al. AI for social good: unlocking the opportunity for positive impact. Nat. Commun. 11, 2468 (2020).
Google Scholar
-
Dwivedi, Y. K. et al. Artificial intelligence (AI): multidisciplinary perspectives on emerging challenges, opportunities, and agenda for research, practice and policy. Int. J. Inf. Manag. 57, 101994 (2021).
Google Scholar
-
Frey, C. B. & Osborne, M. A. The future of employment: how susceptible are jobs to computerisation? Technol. Forecast. Soc. Change 114, 254–280 (2017).
Google Scholar
-
Acemoglu, D. & Restrepo, P. The race between man and machine: implications of technology for growth, factor shares, and employment. Am. Econ. Rev. 108, 1488–1542 (2018).
Google Scholar
-
Khan, H. N., Hounshell, D. A. & Fuchs, E. R. H. Science and research policy at the end of Moore’s law. Nat. Electron. 1, 14–21 (2018).
Google Scholar
-
Iansiti, M. & Lakhani, K. R. Competing in the Age of AI: Strategy and Leadership When Algorithms and Networks Run the World (Harvard Business Press, 2020).
-
Eshraghian, J. K. Human ownership of artificial creativity. Nat. Mach. Intell. 2, 157–160 (2020).
Google Scholar
-
Marcus, G. & Davis, E. Rebooting AI: Building Artificial Intelligence We Can Trust (Pantheon Books, 2019).
-
Liang, W. et al. Advances, challenges and opportunities in creating data for trustworthy AI. Nat. Mach. Intell. 4, 669–677 (2022).
Google Scholar
-
Bengio, Y. et al. Managing extreme AI risks amid rapid progress. Science 384, 842–845 (2024).
Google Scholar
-
Frank, M. R. et al. Toward understanding the impact of artificial intelligence on labor. Proc. Natl Acad. Sci. USA 116, 6531–6539 (2019).
Google Scholar
-
Agrawal, A., Gans, J. S. & Goldfarb, A. Artificial intelligence: the ambiguous labor market impact of automating prediction. J. Econ. Perspect. 33, 31–50 (2019).
Google Scholar
-
Koebis, N., Starke, C. & Rahwan, I. The promise and perils of using artificial intelligence to fight corruption. Nat. Mach. Intell. 4, 418–424 (2022).
Google Scholar
-
Brynjolfsson, E., Li, D. & Raymond, L. R. Generative AI at Work NBER Working Paper No. w31161 (National Bureau of Economic Research, 2023).
-
Noy, S. & Zhang, W. Experimental evidence on the productivity effects of generative artificial intelligence. Science 381, 187–192 (2023).
Google Scholar
-
Silver, D. et al. Mastering the game of Go with deep neural networks and tree search. Nature 529, 484–489 (2016).
Google Scholar
-
Geirhos, R. et al. Generalisation in humans and deep neural networks. In Proc. Advances in Neural Information Processing Systems 7538–7550 (MIT Press, 2018).
-
Grace, K., Salvatier, J., Dafoe, A., Zhang, B. & Evans, O. When will AI exceed human performance? Evidence from AI experts. J. Artif. Intell. Res. 62, 729–754 (2018).
Google Scholar
-
Liu, X. et al. A comparison of deep learning performance against health-care professionals in detecting diseases from medical imaging: a systematic review and meta-analysis. Lancet Digit. Health 1, e271–e297 (2019).
Google Scholar
-
Ishowo-Oloko, F. et al. Behavioural evidence for a transparency-efficiency tradeoff in human-machine cooperation. Nat. Mach. Intell. 1, 517–521 (2019).
Google Scholar
-
Yang, Y., Youyou, W. & Uzzi, B. Estimating the deep replicability of scientific findings using human and artificial intelligence. Proc. Natl Acad. Sci. USA 117, 10762–10768 (2020).
Google Scholar
-
Wurman, P. R. et al. Outracing champion Gran Turismo drivers with deep reinforcement learning. Nature 602, 223–228 (2022).
Google Scholar
-
Maslej, N. et al. The AI Index 2024 Annual Report (AI Index Steering Committee, Institute for Human-Centered AI, Stanford Univ., 2024).
-
Gil, Y., Greaves, M., Hendler, J. & Hirsh, H. Amplify scientific discovery with artificial intelligence. Science 346, 171–172 (2014).
Google Scholar
-
Wang, H. et al. Scientific discovery in the age of artificial intelligence. Nature 620, 47–60 (2023).
Google Scholar
-
Carleo, G. et al. Machine learning and the physical sciences. Rev. Mod. Phys. 91, 045002 (2019).
Google Scholar
-
Rahwan, I. et al. Machine behaviour. Nature 568, 477–486 (2019).
Google Scholar
-
Jimenez-Luna, J., Grisoni, F. & Schneider, G. Drug discovery with explainable artificial intelligence. Nat. Mach. Intell. 2, 573–584 (2020).
Google Scholar
-
Xu, Y. et al. Artificial intelligence: a powerful paradigm for scientific research. Innovation 2, 100179 (2021).
Google Scholar
-
Davies, A. et al. Advancing mathematics by guiding human intuition with AI. Nature 600, 70–74 (2021).
Google Scholar
-
Peng, H., Ke, Q., Budak, C., Romero, D. M. & Ahn, Y.-Y. Neural embeddings of scholarly periodicals reveal complex disciplinary organizations. Sci. Adv. 7, eabb9004 (2021).
Google Scholar
-
Krenn, M. et al. On scientific understanding with artificial intelligence. Nat. Rev. Phys. 4, 761–769 (2022).
Google Scholar
-
Belikov, A. V., Rzhetsky, A. & Evans, J. A. Prediction of robust scientific facts from literature. Nat. Mach. Intell. 4, 445–454 (2022).
Google Scholar
-
Grossmann, I. et al. AI and the transformation of social science research. Science 380, 1108–1109 (2023).
Google Scholar
-
Groh, M. et al. Deep learning-aided decision support for diagnosis of skin disease across skin tones. Nat. Med. 30, 573–583 (2024).
Google Scholar
-
Bail, C. A. Can generative AI improve social science? Proc. Natl Acad. Sci. USA 121, e2314021121 (2024).
Google Scholar
-
Alvarez, A. et al. Science communication with generative AI. Nat. Hum. Behav. 8, 625–627 (2024).
Google Scholar
-
Senior, A. W. et al. Improved protein structure prediction using potentials from deep learning. Nature 577, 706–710 (2020).
Google Scholar
-
Jumper, J. et al. Highly accurate protein structure prediction with AlphaFold. Nature 596, 583–589 (2021).
Google Scholar
-
Baek, M. et al. Accurate prediction of protein structures and interactions using a three-track neural network. Science 373, 871–876 (2021).
Google Scholar
-
Popova, M., Isayev, O. & Tropsha, A. Deep reinforcement learning for de novo drug design. Sci. Adv. 4, aap7885 (2018).
Google Scholar
-
Zhavoronkov, A. et al. Deep learning enables rapid identification of potent DDR1 kinase inhibitors. Nat. Biotechnol. 37, 1038–1040 (2019).
Google Scholar
-
Schneider, P. et al. Rethinking drug design in the artificial intelligence era. Nat. Rev. Drug Discov. 19, 353–364 (2020).
Google Scholar
-
Sadybekov, A. V. & Katritch, V. Computational approaches streamlining drug discovery. Nature 616, 673–685 (2023).
Google Scholar
-
Iten, R., Metger, T., Wilming, H., Del Rio, L. D. & Renner, R. Discovering physical concepts with neural networks. Phys. Rev. Lett. 124, 010508 (2020).
Google Scholar
-
Seif, A., Hafezi, M. & Jarzynski, C. Machine learning the thermodynamic arrow of time. Nat. Phys. 17, 105–113 (2021).
Google Scholar
-
Wu, T. & Tegmark, M. Toward an artificial intelligence physicist for unsupervised learning. Phys. Rev. E 100, 033311 (2019).
Google Scholar
-
Lu, L., Jin, P., Pang, G., Zhang, Z. & Karniadakis, G. E. Learning nonlinear operators via DeepONet based on the universal approximation theorem of operators. Nat. Mach. Intell. 3, 218–229 (2021).
Google Scholar
-
Han, J., Jentzen, A. & Weinan, E. Solving high-dimensional partial differential equations using deep learning. Proc. Natl Acad. Sci. USA 115, 8505–8510 (2018).
Google Scholar
-
Raayoni, G. et al. Generating conjectures on fundamental constants with the Ramanujan Machine. Nature 590, 67–73 (2021).
Google Scholar
-
Degrave, J. et al. Magnetic control of tokamak plasmas through deep reinforcement learning. Nature 602, 414–419 (2022).
Google Scholar
-
Tshitoyan, V. et al. Unsupervised word embeddings capture latent knowledge from materials science literature. Nature 571, 95–98 (2019).
Google Scholar
-
Sanchez-Lengeling, B. & Aspuru-Guzik, A. Inverse molecular design using machine learning: generative models for matter engineering. Science 361, 360–365 (2018).
Google Scholar
-
Chen, C. et al. A critical review of machine learning of energy materials. Adv. Energy Mater. 10, 1903242 (2020).
Google Scholar
-
Merchant, A. et al. Scaling deep learning for materials discovery. Nature 624, 80–85 (2023).
Google Scholar
-
Zheng, S. et al. The AI Economist: taxation policy design via two-level deep multiagent reinforcement learning. Sci. Adv. 8, eabk2607 (2022).
Google Scholar
-
Koster, R. et al. Human-centred mechanism design with Democratic AI. Nat. Hum. Behav. 6, 1398–1407 (2022).
Google Scholar
-
Dunjko, V. & Briegel, H. J. Machine learning & artificial intelligence in the quantum domain: a review of recent progress. Rep. Prog. Phys. 81, 074001 (2018).
Google Scholar
-
Sturm, B. L. et al. Machine learning research that matters for music creation: a case study. J. N. Music Res. 48, 36–55 (2019).
Google Scholar
-
Rajkomar, A., Dean, J. & Kohane, I. Machine learning in medicine. N. Engl. J. Med. 380, 1347–1358 (2019).
Google Scholar
-
Ramesh, A. et al. Zero-shot text-to-image generation. In Proc. 38th International Conference on Machine Learning 8821–8831 (ICML, 2021).
-
Epstein, Z., Hertzmann, A. & the Investigators of Human Creativity. Art and the science of generative AI. Science 380, 1110–1111 (2023).
Google Scholar
-
Swanson, K. et al. Generative AI for designing and validating easily synthesizable and structurally novel antibiotics. Nat. Mach. Intell. 6, 338–353 (2024).
Google Scholar
-
Crafts, N. Artificial intelligence as a general-purpose technology: an historical perspective. Oxf. Rev. Econ. Policy 37, 521–536 (2021).
Google Scholar
-
Bloom, N., Hassan, T. A., Kalyani, A., Lerner, J. & Tahoun, A. The Diffusion of New Technologies NBER Working Paper No. w28999 (National Bureau of Economic Research, 2021).
-
Caselli, F. & Coleman, W. J. Cross-country technology diffusion: the case of computers. Am. Econ. Rev. 91, 328–335 (2001).
Google Scholar
-
Comin, D. & Hobijn, B. An exploration of technology diffusion. Am. Econ. Rev. 100, 2031–2059 (2010).
Google Scholar
-
Zenil, H. et al. The future of fundamental science led by generative closed-loop artificial intelligence. Preprint at https://arxiv.org/abs/2307.07522 (2023).
-
Topol, E. J. High-performance medicine: the convergence of human and artificial intelligence. Nat. Med. 25, 44–56 (2019).
Google Scholar
-
Hidalgo, C. A., Orghian, D., Albo Canals, J., de Almeida, F. & Martín Cantero, N. How Humans Judge Machines (MIT Press, 2021).
-
Raisch, S. & Krakowski, S. Artificial intelligence and management: the automation–augmentation paradox. Acad. Manag. Rev. 46, 192–210 (2021).
Google Scholar
-
Fjelland, R. Why general artificial intelligence will not be realized. Humanit. Soc. Sci. Commun. 7, 10 (2020).
Google Scholar
-
Messeri, L. & Crockett, M. J. Artificial intelligence and illusions of understanding in scientific research. Nature 627, 49–58 (2024).
Google Scholar
-
Kanitscheider, I. & Fiete, I. Training recurrent networks to generate hypotheses about how the brain solves hard navigation problems. In Proc. Advances in Neural Information Processing Systems 4529–4538 (MIT Press, 2017).
-
Webb, M. The Impact of Artificial Intelligence on the Labor Market SSRN 3482150 (Social Science Research Network, 2019).
-
Kogan, L., Papanikolaou, D., Schmidt, L. D. & Seegmiller, B. Technology, Vintage-Specific Human Capital, and Labor Displacement: Evidence from Linking Patents with Occupations NBER Working Paper No. w29552 (National Bureau of Economic Research, 2022).
-
Atalay, E., Phongthiengtham, P., Sotelo, S. & Tannenbaum, D. The evolution of work in the United States. Am. Econ. J. Appl. Econ. 12, 1–34 (2020).
Google Scholar
-
Felten, E. W., Raj, M. & Seamans, R. A method to link advances in artificial intelligence to occupational abilities. AEA Pap. Proc. 108, 54–57 (2018).
Google Scholar
-
Wu, L., Hitt, L. & Lou, B. Data analytics, innovation, and firm productivity. Manag. Sci. 66, 2017–2039 (2020).
Google Scholar
-
Brynjolfsson, E., Mitchell, T. & Rock, D. What can machines learn, and what does it mean for occupations and the economy? AEA Pap. Proc. 108, 43–47 (2018).
Google Scholar
-
Wang, D. & Barabási, A.-L. The Science of Science (Cambridge Univ. Press, 2021).
-
Fortunato, S. et al. Science of science. Science 359, eaao0185 (2018).
Google Scholar
-
Zeng, A. et al. The science of science: from the perspective of complex systems. Phys. Rep. 714, 1–73 (2017).
Google Scholar
-
Frank, M. R., Wang, D., Cebrian, M. & Rahwan, I. The evolution of citation graphs in artificial intelligence research. Nat. Mach. Intell. 1, 79–85 (2019).
Google Scholar
-
Miao, L. et al. The latent structure of global scientific development. Nat. Hum. Behav. 6, 1206–1217 (2022).
Google Scholar
-
Liu, L., Jones, B. F., Uzzi, B. & Wang, D. Data, measurement and empirical methods in the science of science. Nat. Hum. Behav. 7, 1046–1058 (2023).
Google Scholar
-
Sourati, J. & Evans, J. A. Accelerating science with human-aware artificial intelligence. Nat. Hum. Behav. 7, 1682–1696 (2023).
Google Scholar
-
Murray, D. et al. Unsupervised embedding of trajectories captures the latent structure of scientific migration. Proc. Natl Acad. Sci. USA 120, e2305414120 (2023).
Google Scholar
-
Krenn, M. et al. Forecasting the future of artificial intelligence with machine learning-based link prediction in an exponentially growing knowledge network. Nat. Mach. Intell. 5, 1326–1335 (2023).
Google Scholar
-
Sinha, A. et al. An overview of Microsoft Academic Service (MAS) and applications. In Proc. 24th International Conference on World Wide Web 243–246 (WWW, 2015).
-
World Intellectual Property Organization (WIPO). WIPO Technology Trends 2019—Artificial Intelligence (WIPO, 2019).
-
Nivre, J. & Nilsson, J. Pseudo-projective dependency parsing. In Proc. 43rd Annual Meeting of the Association for Computational Linguistics 99–106 (ACL, 2005).
-
Honnibal, M. & Johnson, M. An improved non-monotonic transition system for dependency parsing. In Proc. 2015 Conference on Empirical Methods in Natural Language Processing 1373–1378 (ACL, 2015).
-
Benetka, J. R., Krumm, J. & Bennett, P. N. Understanding context for tasks and activities. In Proc. 2019 Conference on Human Information Interaction and Retrieval 133–142 (ACM, 2019).
-
Service, R. Science’s 2021 Breakthrough of the Year: protein structures for all. Science https://www.science.org/content/article/breakthrough-2021 (2021).
-
Börner, K. et al. Skill discrepancies between research, education, and jobs reveal the critical need to supply soft skills for the data economy. Proc. Natl Acad. Sci. USA 115, 12630–12637 (2018).
Google Scholar
-
Wuchty, S., Jones, B. F. & Uzzi, B. The increasing dominance of teams in production of knowledge. Science 316, 1036–1039 (2007).
Google Scholar
-
Wu, L., Wang, D. & Evans, J. A. Large teams develop and small teams disrupt science and technology. Nature 566, 378–382 (2019).
Google Scholar
-
Littmann, M. et al. Validity of machine learning in biology and medicine increased through collaborations across fields of expertise. Nat. Mach. Intell. 2, 18–24 (2020).
Google Scholar
-
Mehrabi, N., Morstatter, F., Saxena, N., Lerman, K. & Galstyan, A. A survey on bias and fairness in machine learning. ACM Comput. Surv. 54, 1–35 (2021).
Google Scholar
-
Young, E., Wajcman, J. & Sprejer, L. Where Are the Women? Mapping the Gender Job Gap in AI (The Alan Turing Institute, 2021).
-
Xie, Y. & Shauman, K. A. Women in Science: Career Processes and Outcomes (Harvard Univ. Press, 2003).
-
Hoppe, T. A. et al. Topic choice contributes to the lower rate of NIH awards to African-American/black scientists. Sci. Adv. 5, eaaw7238 (2019).
Google Scholar
-
Ginther, D. K. et al. Race, ethnicity, and NIH research awards. Science 333, 1015–1019 (2011).
Google Scholar
-
Larivière, V., Ni, C., Gingras, Y., Cronin, B. & Sugimoto, C. R. Bibliometrics: global gender disparities in science. Nature 504, 211–213 (2013).
Google Scholar
-
Huang, J., Gates, A. J., Sinatra, R. & Barabási, A.-L. Historical comparison of gender inequality in scientific careers across countries and disciplines. Proc. Natl Acad. Sci. USA 117, 4609–4616 (2020).
Google Scholar
-
The National Network for Critical Technology Assessment (NNCTA). Securing America’s Future: A Framework for Critical Technology Assessment (NNCTA, 2023).
-
Cachola, I., Lo, K., Cohan, A. & Weld, D. S. TLDR: extreme summarization of scientific documents. In Proc. 2020 Conference on Empirical Methods in Natural Language Processing 4766–4777 (ACL, 2020).
-
Lew, A., Agrawal, M., Sontag, D. & Mansinghka, V. PClean: Bayesian data cleaning at scale with domain-specific probabilistic programming. In Proc. 24th International Conference on Artificial Intelligence and Statistics 1927–1935 (JMLR, 2021).
-
Bommasani, R. et al. On the opportunities and risks of foundation models. Preprint at https://arxiv.org/abs/2108.07258 (2021).
-
Wei, J. et al. Emergent abilities of large language models. Preprint at https://arxiv.org/abs/2206.07682 (2022).
-
Moor, M. et al. Foundation models for generalist medical artificial intelligence. Nature 616, 259–265 (2023).
Google Scholar
-
Huang, Z., Bianchi, F., Yuksekgonul, M., Montine, T. J. & Zou, J. A visual-language foundation model for pathology image analysis using medical Twitter. Nat. Med. 29, 2307–2316 (2023).
Google Scholar
-
Goldfarb, A., Taska, B. & Teodoridis, F. Could machine learning be a general purpose technology? A comparison of emerging technologies using data from online job postings. Res. Policy 52, 104653 (2023).
Google Scholar
-
Fawzi, A. et al. Discovering faster matrix multiplication algorithms with reinforcement learning. Nature 610, 47–53 (2022).
Google Scholar
-
Jobin, A., Ienca, M. & Vayena, E. The global landscape of AI ethics guidelines. Nat. Mach. Intell. 1, 389–399 (2019).
Google Scholar
-
Arrieta, A. B. et al. Explainable artificial intelligence (XAI): concepts, taxonomies, opportunities and challenges toward responsible AI. Inf. Fusion 58, 82–115 (2020).
Google Scholar
-
Lenharo, M. An AI revolution is brewing in medicine. What will it look like? Nature 622, 686–688 (2023).
Google Scholar
-
Bockting, C. L., van Dis, E. A. M., van Rooij, R., Zuidema, W. & Bollen, J. Living guidelines for generative AI—why scientists must oversee its use. Nature 622, 693–696 (2023).
Google Scholar
-
Schwartz, I. S., Link, K. E., Daneshjou, R. & Cortes-Penfield, N. Black box warning: large language models and the future of infectious diseases consultation. Clin. Infect. Dis. 78, 860–866 (2024).
Google Scholar
-
Ahmadpoor, M. & Jones, B. F. The dual frontier: patented inventions and prior scientific advance. Science 357, 583–587 (2017).
Google Scholar
-
Mukherjee, S., Romero, D. M., Jones, B. & Uzzi, B. The nearly universal link between the age of past knowledge and tomorrow’s breakthroughs in science and technology: the hotspot. Sci. Adv. 3, e1601315 (2017).
Google Scholar
-
Yin, Y., Dong, Y., Wang, K., Wang, D. & Jones, B. F. Public use and public funding of science. Nat. Hum. Behav. 6, 1344–1350 (2022).
Google Scholar
-
Microsoft Academic. Microsoft Academic Graph. Zenodo https://doi.org/10.5281/zenodo.6511057 (2022).
-
Lin, Z., Yin, Y., Liu, L. & Wang, D. SciSciNet: a large-scale open data lake for the science of science research. Sci. Data 10, 315 (2023).
Google Scholar
Acknowledgements
We thank Y. Yin, Y. Qian, B. Wang, N. Dehmamy, L. Varshney, L. Miao, L. Wu, A. Freilich and all members of the Center for Science of Science and Innovation (CSSI) at Northwestern University for helpful discussions. This work is supported by the Air Force Office of Scientific Research FA9550-19-1-0354 (D.W.), the National Science Foundation SBE 1829344, TIP 1123649-464363//2241237, and TIP 2404035 (D.W.), the Alfred P. Sloan Foundation G-2019-12485 (D.W.), and the Peter G. Peterson Foundation 21048 (D.W.). The funders had no role in study design, data collection and analysis, decision to publish or preparation of the paper.
Author information
Authors and Affiliations
Contributions
J.G. and D.W. conceived the idea. D.W. supervised the project. J.G. collected data and performed analyses. J.G. and D.W. analysed the results, interpreted the findings and wrote the paper.
Corresponding author
Ethics declarations
Competing interests
The authors declare no competing interests.
Peer review
Peer review information
Nature Human Behaviour thanks Alexander Gates and the other, anonymous, reviewer(s) for their contribution to the peer review of this work.
Additional information
Publisher’s note Springer Nature remains neutral with regard to jurisdictional claims in published maps and institutional affiliations.
Supplementary information
Supplementary Information
Supplementary Notes 1–9, Figs. 1–17, Tables 1 and 2, and references.
Reporting Summary
Rights and permissions
Springer Nature or its licensor (e.g. a society or other partner) holds exclusive rights to this article under a publishing agreement with the author(s) or other rightsholder(s); author self-archiving of the accepted manuscript version of this article is solely governed by the terms of such publishing agreement and applicable law.
Reprints and permissions
About this article
Cite this article
Gao, J., Wang, D. Quantifying the use and potential benefits of artificial intelligence in scientific research.
Nat Hum Behav (2024). https://doi.org/10.1038/s41562-024-02020-5
-
Received: 26 April 2023
-
Accepted: 12 September 2024
-
Published: 11 October 2024
-
DOI: https://doi.org/10.1038/s41562-024-02020-5