During her PhD training years a decade ago, Kathleen Elison would ride her purple Huffy cruiser bike from her laboratory, housed at the Flower Building at City of Hope, to a neighboring microscopy facility. With her advisor, Jacob Berlin, PhD, associate professor in the department of molecular medicine, Elison was developing an ultradense microarray chip for measuring small molecule interactions with the goal of informing therapeutic applications, such as drug discovery. A key step for prepping the chip for her screening experiments involved using a microscope to collect the sequencing information of a combinatorial library, a process termed “decoding.”
“The trip was one mile one way and I would go three times a day to image our chips,” Elison recalled.
At that time, decoding a maximum of five fields of view manually would require four to five days. A big step toward increasing that throughput came after the group received funding for their own microscope. With new pipelines for automated image processing and data extraction, the scale-up snowballed from there.
Today, Elison is a senior scientist at Terray Therapeutics, a biotech company based in Monrovia, California (Los Angeles), whereas Berlin is the founding CEO. The company’s core technology combines Elison’s ultraminiaturized chip (about the size of a nickel) with artificial intelligence (AI) tools for drug discovery. What started as manual imaging of five fields of view on a single chip over several days has now escalated to imaging and decoding entire chips within 24 hours using Terray’s automated platform.
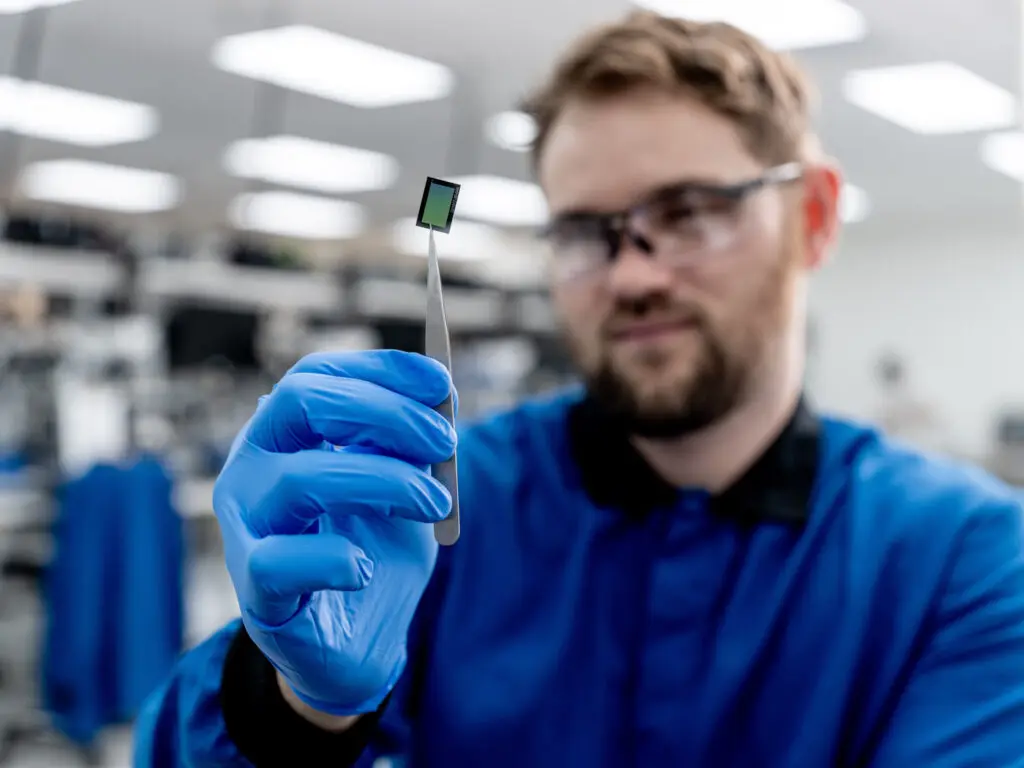
“That’s an astronomical gain of 7,000 times more space in a fifth of the time,” Elison told me on a recent visit to Terray’s headquarters.
Elison’s chip was taken to the company context in late 2018. In February 2022, Terray announced their $60 million Series A, which followed a previously unannounced $20 million seed round. Since that time, the company has grown into a team of 100 employees and a 52,000 square foot facility that neighbors the City of Hope campus in Duarte, California. During my on-site visit, I spotted a few stuffed animal coatis, a member of the racoon family, a hat tip to the company’s foundational model of chemical space, contrastive optimization for accelerated therapeutic inference (COATI).
Terray has also established a handful of promising partnerships. In October 2022, the company announced a collaboration with Calico Life Sciences to discover small-molecule therapeutics for age-related diseases. A multi-target collaboration with Bristol Myers Squibb was announced in December 2023.
And in November 2023, Terray announced an equity investment by NVentures, NVIDIA’s venture capital arm. In this collaboration, Terray leverages NVIDIA DGX Cloud to develop comprehensive chemistry foundation models for small molecules, some of which will be available on the NVIDIA BioNeMo cloud service for generative AI in drug discovery.
Berlin describes the timeline of the company as a multistage journey through the hardware revolution (scaling up chip manufacturing), to the data revolution (collecting quality data with speed and precision), to the compute revolution (building a drug discovery platform). As insufficient or unclear data are one of the biggest bottlenecks for AI approaches to drug discovery, Terray’s ultraminiaturized chip aims to address this gap by generating quality chemical data at unprecedented scale. Over the past 18 months, Terray has measured more than 2.6 billion small molecule/target interactions to fuel their generative AI drug design.
Ultimately, Berlin emphasizes that AI and data are both useless without the other. “No human is going to look at 2.6 billion data points and do anything really exciting with them. You have to have the computational side as well. The difference is the data, but the value is the interwoven half-and-half approach from Day 1 that the computation and data go together always,” Berlin told GEN.
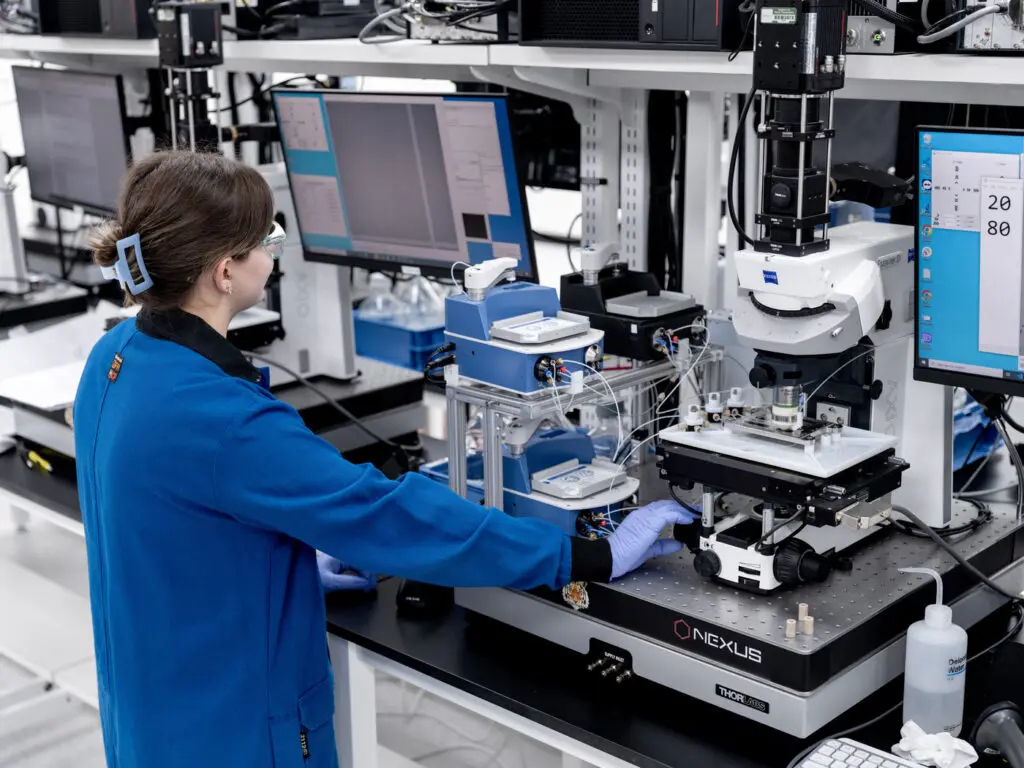
Embracing the Black Box
Terray is one of literally dozens of biotech companies currently riding the “AI revolution” in drug discovery. Big players, such as Exscientia and Recursion Pharmaceuticals, two now public companies founded in 2012 and 2013, respectively, were followed by an explosion of new drug discovery start-ups leveraging AI in the decade ahead. But what exactly does this AI black box entail?
On a broad level, AI in drug discovery is characterized by new data-intensive platforms that provide improved speed and failure rate reductions. These platforms incorporate AI tools, such as machine learning (ML), to learn biological patterns from the data, make predictions to direct next experimental steps, and test those predictions in the wet laboratory.
In a recent article published in Nature Biotechnology, scientists from Insilico Medicine, a drug discovery company headquartered in Hong Kong and New York City, used AI-driven methodology to identify a small molecule inhibitor for the fatal lung condition, idiopathic pulmonary fibrosis. The work was completed in roughly 18 months from target discovery to preclinical candidate nomination.
At Biocom California’s Global Life Science Partnering and Investor Conference, held in February at the famed Torrey Pines golf course in La Jolla, California, D.A. Wallach, general partner and cofounder of Time BioVentures, moderated a discussion entitled, “AI and Drug Discovery: Where’s the Beef?” to explore the role and advantages of AI tools in drug discovery pipelines (Fig. 3).
“‘Where is the beef?’ is a hard question to answer, partially because it’s distributed evenly everywhere. The industry has not seen the headline, ‘Here’s the commercial AI molecule that’s passed clinical testing and is now [one of the top] selling drugs in the world,’” said Nan Li, founder and managing partner at Dimension.
Indeed, today’s AI in drug discovery companies do not flaunt a “click button, get molecule” fully integrated product. Rather, AI tools provide improved efficiency to traditionally slow drug discovery pipelines, with in silico tools providing an efficient first place to search before heading to the wet laboratory.
Emma Parmee, global head therapeutics discovery at Johnson & Johnson, said that efficiency is not just defined by speed, but also includes enabling accessibility to targets that could not be drugged 5–10 years ago and identifying drug candidates that fail less in the clinic. In this vein, data-driven approaches have proven powerful to guide next research steps to facilitate the path to a successful drug.
“From a single starting point, we were using our generative tools [to output] tens of thousands of molecules, which were then triaged for our chemists to assess,” said Parmee. “One of the generated molecules actually inspired the chemist to make another [molecular change] that was really impactful for the program.”
She also emphasized that human knowledge, such as medicinal chemistry expertise and an understanding of synthetic accessibility, remains crucial to these pipelines, as AI models remain predictive.
Ben Sklaroff, CTO and cofounder at Genesis Therapeutics, described the computational chemist as a “strategic controller” of the AI. Instead of hand designing individual molecules, the chemists “point a searchlight in a particular direction.” AI can then generate a set of molecules within that area of chemical space. This searchlight can include identifying areas for molecular modification or defining parameters for absorption, distribution, metabolism, and excretion (ADME) to assist the pharmacology.
Genesis launched in 2019 as a Stanford spinout from the laboratory of Vijay Pande, founding general partner of Andreesen Horowitz’s (a16z) Bio+Health fund. The company focuses on generative and predictive AI for small molecule drug discovery using their deep learning platform, dubbed Genesis Exploration of Molecular Space (GEMS), Genesis has established partnerships with Genentech and Eli Lilly, and forged a $200 million venture capital round in August 2023, co-led by a16z.
Although scientists are on a mission to understand biology, Wallach posed the criticism that ML models tend to be “massive regression finders” and “big correlative inference machines” that may not provide answers to the mechanisms of biology. Should this be a concern for scientists?
“With a few exceptions, we hardly know how any drug actually works!” exclaimed Philip Tagari, CSO of insitro. “If the intent is to improve human health and ensure that effective therapies are in the hands of patients, developing a therapeutic without fully understanding the biology is par for the course. If it’s a black box, I embrace the black box!” Tagari continued.
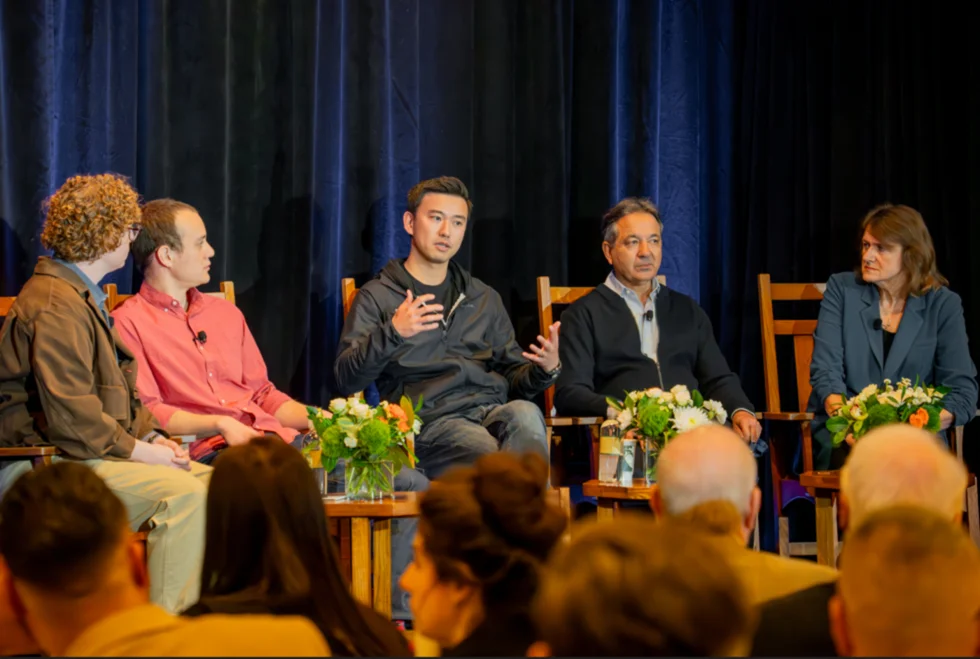
Taken together, applying AI to drug discovery remains a multifaceted feat. In my recent interview with Simon Barnett, research director at Dimension and former director of life science research at ARK Invest, on GEN‘s weekly podcast, Touching Base, Barnett described drug discovery not as a single massive problem that will see floodgates open with an AI or ML tool, but rather a series of small problems and decisions that, compounded together, creates a difficult problem in totality.
“A drug is not ‘designed with AI.’ [Rather], ML tools have continued to saturate the process of drug development over a period of years,” said Barnett. “You can build a great company around the right data and the right architecture applied to the right problem. If you line all those things up, I think there are really interesting outcomes.”
Big power of small molecules
Back at Terray, the “right” data, architecture, and problem feature a high-throughput platform to address the historical lack of data on small molecules for drug discovery.
From genomics, proteomics, siRNA, CRISPR knockouts, and patient databases, such as the UK Biobank, biology has seen an explosion in data streams that have fueled data-driven computational opportunities for therapeutics. Although data streams for biologics, such as antibodies and proteins, have had the advantage of leveraging natural factories, or cells, that can generate large libraries of candidates, small molecules were traditionally made by synthetic chemists with an average throughput of a few molecules a week. These approaches were both slow and expensive. As a solution, Terray’s chip provides data that are “huge, high quality, and iterates quickly.”
Vanessa Taylor, CSO of Terray, described that although the chip is target and disease agnostic, Terray maintains a therapeutic focus in immunology. Given that many biologics in the immunology space rely on patient injections, there is an unmet need for small molecule oral drugs.
“Patients don’t necessarily want to go into the clinic every six weeks to be injected with a drug. Plus, because of the long half-lives of these drugs, they don’t wash out fast. If a patient is massively immunosuppressed, they can be stuck for six weeks,” Taylor told GEN.
She also noted that Terray’s small molecule pursuit often follows pathways where drugs are already approved. “We know there’s a therapeutic benefit if we can target that pathway by a different mechanism, which is a small molecule going into the cell and hitting something in that pathway,” said Taylor.
“People don’t fully know where in those pathways to intervene. It’s a natural fit for [Terray’s approach] where you take many targets in parallel and you find that right point of intervention,” concurred Berlin.
Mapping mountains in chemical space
Traditional drug discovery followed a linear approach where individual targets are initially selected by humans based on known biology. In contrast, today’s AI tools present an inverted approach that samples large chemical space to inform a broad selection of targets that are worthy of consideration.
“Nobody knows at the outset where the right intersection of chemical discovery and biology is going to lead to the highest likelihood of success,” Berlin said. “The good news is the space is so big that the answer to almost anything is out there somewhere. But the opposite is true too. The space is so big, defining those answers is really hard.”
Terray’s chip technology evaluates the binding affinity of a protein of interest against millions of compounds on a microarray. Taylor noted that the screen is positioned to identify novel binding sites given that the platform is agnostic to how the protein binds. “It’s basically a massive high-throughput affinity platform with the potential to iterate. We focus on areas where we see binding and then look at how changing the structure of the molecule changes binding affinity,” Taylor told GEN.
Terray’s pipeline features three main steps: diversity library screening, focused library screening, and generative drug design. During the diversity library screen, tens of millions of compounds are added to the collection with a focus on increasing the coverage of chemical space, thereby constructing a map by first outlining the mountains.
The diversity screen pinpoints chemical areas of interest. Focused library screens then go forward to increase the chemical resolution around these areas. “You can think about the resolution that adds when you go from one dot way out in chemical space to entire cartoons of mountain mapping. You can then have molecules all around that space telling you, ‘a methyl here really matters!’ or ‘no, you’ve got to have this other ring system over here,’” Berlin said.
Lastly, the data from the diversity and focused library screening are combined with supplemental resources, such as ADME data sets, to assist medicinal chemists for generative drug design.
Ten years after her daily bike ride to the microscopy facility, Elison is struck by the interdisciplinary team pushing Terray’s research forward.
“[The computer data scientists] are very familiar with every aspect of what goes on in the wet lab since they are so intimate with the data that’s coming out of it,” said Elison. “Some of our best wet lab ideas come from computer scientists!”
Ultimately, the biggest reward for Elison has been the journey. “I won the lottery watching my grad school project evolve from a whiteboard concept to a full company,” she said.
A version of this article was published in the April 2024 issue of GEN‘s sister peer review journal, GEN Biotechnology.
Fay Lin, PhD, is senior editor for GEN Biotechnology.