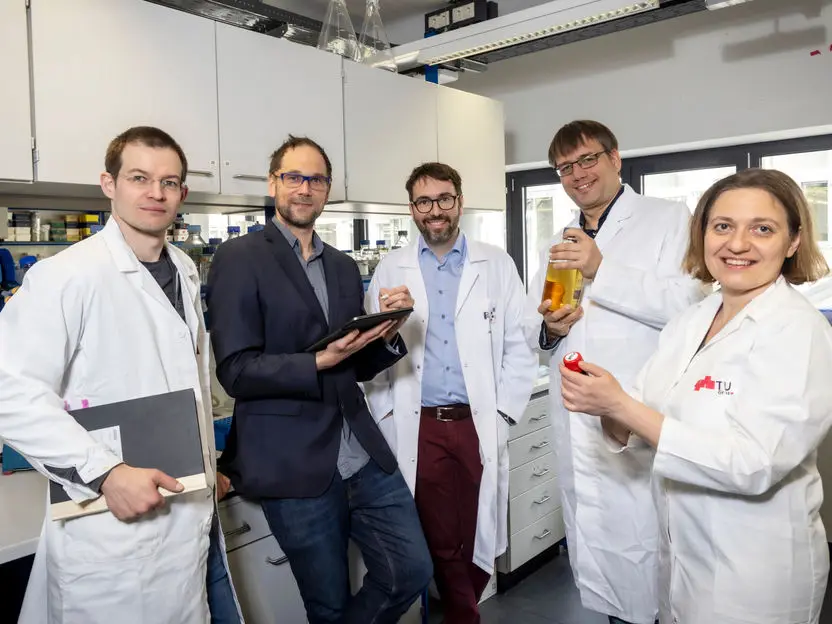
In a multidisciplinary research project, researchers from the biosciences, process engineering and computer science want to massively increase efficiency in the development of new enzymes and process optimization. This should even enable the breakdown of forever chemicals.
Graz University of Technology (TU Graz) is funding a new lead project called DigiBioTech, in which 17 scientists and ten doctoral students from the fields of biotechnology, biotechnological process engineering and computer science are working closely together to significantly improve the predictability and control of biochemical reactions and processes. By merging biotechnology, data science and artificial intelligence, the project team aims to efficiently produce novel enzymes. They will not only enable sustainable production processes, but some of these enzymes should be able to break down persistent environmental toxins such as perfluorinated and polyfluorinated alkyl compounds (PFAS).
1.96 million euros in funding
TU Graz is funding this fundamental research with 1.96Mio Euros over an initial period of three years. Funding can be extended after an interim evaluation. “TU Graz has outstanding international expertise in the fields of biotechnology and computer science. Combining the strengths of these two disciplines opens up fascinating prospects for basic research and the application of sustainable production processes,” says Andrea Höglinger, Vice-Rector for Research. “The DigiBioTech lead project is a perfect fit for the profile of TU Graz, whose researchers are working on solutions for the most pressing challenges of the present and future.”
Significant leaps in efficiency expected
“Biotechnology can make our society considerably more sustainable. To do this, we need to optimise biological systems, which we will be able to do much better and more reliably by integrating artificial intelligence,” says Robert Kourist from the Institute of Molecular Biotechnology, who heads the DigiBioTech project. If, for example, you want to change the reaction centre of an enzyme in several places at the same time to improve its efficiency, the resulting number of variants to test in the laboratory quickly exceeds a million. “Applying machine learning enables enormous leaps in efficiency here,” says Gustav Oberdorfer from the Institute of Biochemistry. “Our aim is to reduce the number of necessary experiments to just a handful.”
Digital twin of biochemical processes
Just as complex as the optimisation of the molecular design of enzymes is the design of the process technology, i.e. the targeted control of the physical and chemical environment so that a biological process runs optimally. This is particularly difficult when several enzymes work together. “We want to create digital twins of such biological and biochemical processes in order to predict and design the processes in all their complexity and to be able to control them on a large scale,” says Regina Kratzer from the Institute of Biotechnology and Biochemical Engineering.
Development of suitable machine learning methods
“Biotechnology and computer science are long-standing key research areas at TU Graz. In this lead project, we aim to develop a common language in order to match experimental data with recent machine learning technology and advance machine learning research in the area of biotechnology,” says Robert Peharz from the Institute of Theoretical Computer Science. So-called diffusion-based generative models, which are currently frequently used to generate images, are to be used in the development of new enzymes. Furthermore, according to Robert Peharz, probabilistic machine learning approaches such as Bayesian optimisation will be used to narrow down the huge space of enzyme candidates.
Breakdown of forever chemicals and production of bioplastics from CO2
The methods are being developed and applied in sub-projects dedicated to three main topics: the development of enzymes for the breakdown of per- and polyfluorinated alkyl compounds (PFAS), the production of bioplastics from CO2 and the prediction and automated control of the interaction of multiple enzymes. To this end, predictions from artificial intelligence are always verified in laboratory experiments and then fed back to the AI models for refinement.
“We are faced with the challenge that there are few publicly accessible data sets for enzyme activities and biotechnological process control,” says Gustav Oberdorfer. “We therefore have to generate these ourselves through experiments and keep the parameters absolutely constant so that they are as suitable as possible for the used machine learning models.” The data obtained within the framework of DigiBioTech will all be made publicly available. “With this project, we also want to contribute to democratisation in this area of biotechnology,” emphasises Gustav Oberdorfer.