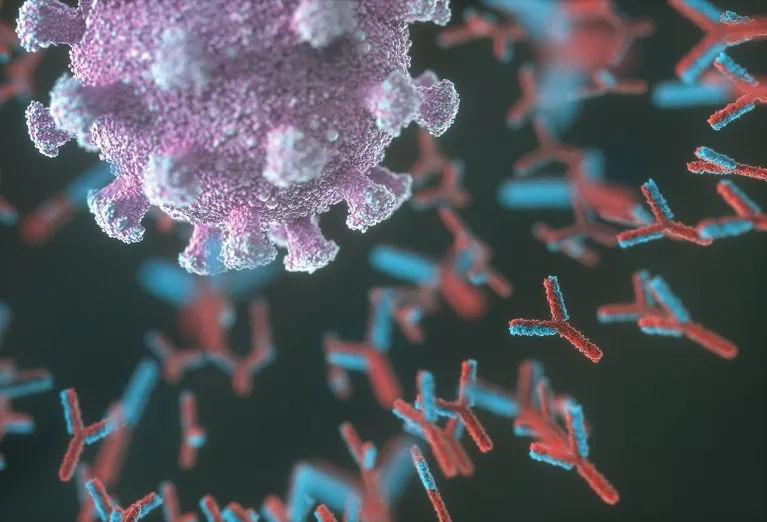
The virtual lab set-up used several LLMs to design antibody fragments that could bind to SARS-CoV-2.Credit: KTSDESIGN/Science Photo Library via Getty
In an effort to automate scientific discovery using artificial intelligence (AI), researchers have created a virtual laboratory that combines several ‘AI scientists’ — large language models with defined scientific roles — that can collaborate to achieve goals set by human researchers.
The system, described in a preprint posted on bioRxiv last month1, was able to design antibody fragments called nanobodies that can bind to the virus that causes COVID-19, proposing nearly 100 of these structures in a fraction of the time it would take an all-human research group.
Researchers built an ‘AI Scientist’ — what can it do?
“These virtual-lab AI agents have shown to be quite capable at doing a lot of tasks,” says study co-author James Zou, a computational biologist at Stanford University in California. “We’re quite excited about exploring the potential of the virtual lab across different scientific domains.”
The experiment “represents a new paradigm of taking AI as collaborators, not just tools”, says Yanjun Gao, who researches the health-care applications of AI at the University of Colorado Anschutz Medical Campus in Aurora. But she adds that human input and oversight are still crucial. “I don’t think at this stage we can fully trust the AI to make decisions.”
Interdisciplinary AI
Scientists worldwide have explored the potential of large language models (LLMs) to speed up research — including creating an ‘AI scientist’ that can carry out parts of the scientific process, from generating hypotheses and designing experiments to drafting papers. But Zou says that most studies have focused on the application of LLMs for experiments with a narrow scope, rather than exploring their potential in interdisciplinary research. He and his colleagues set up the virtual lab to combine expertise from different fields.
They began by training two LLMs for their virtual team: the team-leading principal investigator (PI), which has expertise in AI for research, and a ‘scientific critic’ to catch errors and oversights from other LLMs throughout the process. The authors gave these LLMs a goal — designing new nanobodies to target the virus SARS-CoV-2 — and instructed them to develop other LLMs that could achieve it.
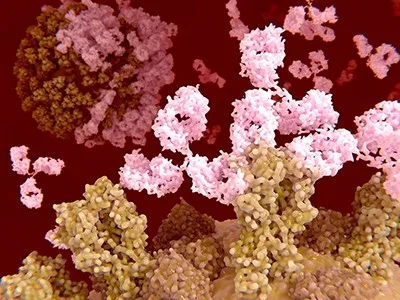
‘A landmark moment’: scientists use AI to design antibodies from scratch
The PI then created and trained three further AI scientist agents to support the research efforts. Each of these ‘scientists’ was trained in a particular discipline — immunology, computational biology or machine learning. “These different agents would have different expertise, and they would work together in solving different kinds of scientific problems,” says Zou.
The AI agents worked independently on tasks allocated by the virtual PI, such as calculating parameters or writing code for a new machine-learning model. They could also make use of other AI research tools, such as the protein-design tools AlphaFold and Rosetta. A human researcher guided the LLMs through regular ‘team meetings’ to evaluate their progress.
“The virtual lab is designed to be mostly autonomous, so the agents discuss with each other. They decide what problem to solve and what approach to take and how to implement those approaches,” says Zou. “The human researchers focus on providing more high-level feedback to guide the direction of the virtual lab.” Team meetings involved several rounds of ‘discussion’, but took only 5–10 minutes each.
Versatile system
The agents ultimately designed 92 nanobodies, more than 90% of which were shown to bind to the original variant of SARS-CoV-2 in validation studies. Two of the nanobodies also showed promise in targeting newer variants of the virus.
The researchers are optimistic that their system could help to enhance multiple fields of scientific research. “We designed it to be a very versatile platform. So in principle, we can use these virtual lab agents and ask them to solve different scientific problems,” Zou says. He stresses that human intervention and feedback is key to the virtual lab’s success. “We still need to verify and validate those hypotheses; this is where it’s important to still have the real-world experiment.”
Gao says future studies should further evaluate responses generated by the AI scientists, to understand why the LLMs make mistakes or disagree with one another. “Safety and evaluation is something that I really hope to see more in the future of human–AI collaborations,” Gao says.